Book contents
- Frontmatter
- Contents
- Preface
- Introduction
- 1 Overview of count response models
- 2 Methods of estimation
- 3 Poisson regression
- 4 Overdispersion
- 5 Negative binomial regression
- 6 Negative binomial regression: modeling
- 7 Alternative variance parameterizations
- 8 Problems with zero counts
- 9 Negative binomial with censoring, truncation, and sample selection
- 10 Negative binomial panel models
- Appendix A Negative binomial log-likelihood functions
- Appendix B Deviance functions
- Appendix C Stata negative binominal – ML algorithm
- Appendix D Negative binomial variance functions
- Appendix E Data sets
- References
- Author Index
- Subject Index
- References
References
Published online by Cambridge University Press: 05 June 2012
- Frontmatter
- Contents
- Preface
- Introduction
- 1 Overview of count response models
- 2 Methods of estimation
- 3 Poisson regression
- 4 Overdispersion
- 5 Negative binomial regression
- 6 Negative binomial regression: modeling
- 7 Alternative variance parameterizations
- 8 Problems with zero counts
- 9 Negative binomial with censoring, truncation, and sample selection
- 10 Negative binomial panel models
- Appendix A Negative binomial log-likelihood functions
- Appendix B Deviance functions
- Appendix C Stata negative binominal – ML algorithm
- Appendix D Negative binomial variance functions
- Appendix E Data sets
- References
- Author Index
- Subject Index
- References
Summary
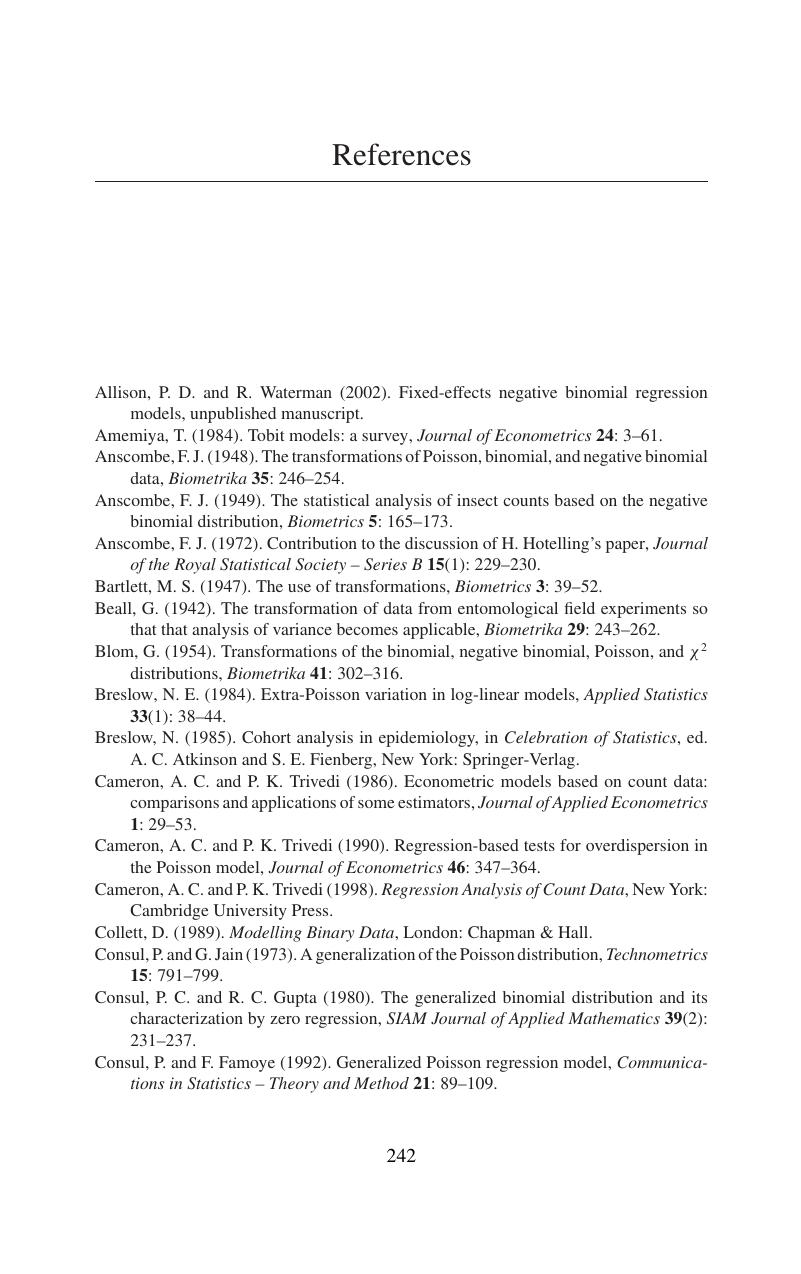
- Type
- Chapter
- Information
- Negative Binomial Regression , pp. 242 - 246Publisher: Cambridge University PressPrint publication year: 2007