1. Introduction
The goal of optimal (re)insurance is to strike the best balance between risk transfer and risk retention. Insurers transfer a portion of their risk exposures to reinsurers in order to reduce their exposure to large losses and to stabilize their financial positions. Reinsurers, on the other hand, assume these risks in exchange for premiums, diversify their risk portfolio, and generate additional income. The study of optimal reinsurance treaties has a rich history, with key contributions from researchers such as Borch (Reference Borch1960) and Arrow (Reference Arrow1963). In particular, Borch (Reference Borch1960) demonstrated that stop-loss treaties are effective in minimizing the variance of the insurer’s retained loss when the reinsurance premium is calculated by the expected value premium principle. Arrow (Reference Arrow1963) extended the analysis to incorporate risk-averse utility functions and concluded that stop-loss contracts not only minimize variance but also maximize the expected utility of the insurer’s terminal wealth. These findings highlight the value and effectiveness of stop-loss treaties in managing risk for insurers. Along with these two classical works, the research area on optimal (re)insurance has garnered significant attention from both academics and industry professionals and has grown into a vibrant field of study; see the inclusion of mean-variance preferences in Kaluszka (Reference Kaluszka2001), Li and Young (Reference Li and Young2021) and Boonen and Jiang (Reference Boonen and Jiang2022); the extension to general premium principles in Kaluszka (Reference Kaluszka2005) and Young (Reference Young1999); the implementation from the perspective of Stackelberg game in Li and Young (Reference Li and Young2021) and Boonen et al. (Reference Boonen, Cheung and Zhang2021a); the competition between one insurer and two or more reinsurers in Chi and Meng (Reference Chi and Meng2014) and Boonen et al. (Reference Boonen, Tan and Zhuang2021b). Interested readers can refer to Albrecher et al. (Reference Albrecher, Beirlant and Teugels2017) and Cai and Chi (Reference Cai and Chi2020) for a comprehensive review of the history and some recent developments on optimal insurance problems.
The recent regulatory developments in the insurance industry have emphasized the importance of using risk measures such as value-at-risk (VaR) and conditional-tail-expectationFootnote 1 (CTE) to assess and manage risk. In line with this, researchers have focused on incorporating these risk measures into the study of optimal reinsurance models. Cai and Tan (Reference Cai and Tan2007) specifically analyzed the optimal retention level of a stop-loss reinsurance treaty, aiming to minimize both the VaR or CTE of the insurer’s remaining risk exposure based on the expected value premium principle. From then on, the research on optimal reinsurance schemes has been expanded and refined by subsequent studies under the framework of risk measures. For example, Cai et al. (Reference Cai, Tan, Weng and Zhang2008) extended the analysis in Cai and Tan (Reference Cai and Tan2007) to include increasing convex functions and examined optimal reinsurance schemes within this class. Cheung (Reference Cheung2010) simplified the arguments in Cai et al. (Reference Cai, Tan, Weng and Zhang2008) using a geometric approach and showed that the stop-loss treaty remains optimal even when the expected value premium principle is replaced by Wang’s premium principle in the VaR-minimization problem. Cai and Tan (2011) further expanded the analysis by considering a larger class of admissible reinsurance schemes and determining the optimal reinsurance contracts for minimizing the VaR and CTE of the insurer’s total retained loss. These findings have been further extended and explored in subsequent studies by Chi (Reference Chi2012) and Chi and Tan (Reference Chi and Tan2013).
In practical insurance scenarios, insurance companies may default because of various reasons such as catastrophic events, underwriting losses, inadequate capitalization, or financial mismanagement. For example, the renowned finance and insurance tycoon American International Group (AIG) was a case in point. The company had written massive credit default swaps (CDS), which were essentially insurance contracts on mortgage-backed securities, prior to the financial crisis in 2008. As the housing market and mortgages deteriorated, AIG faced massive obligations under these CDS contracts, leading to significant losses that eroded its capital. In the end, the U.S. government intervened with a substantial bailout to avoid its default. From a prudent perspective, insurers and reinsurers may consider factors such as the probability of default, the potential size of the default, and the cost of capital when determining the optimal level of risk transfer. Therefore, it is essential to consider the problem of optimal reinsurance design in presence of counterparty’s default risk to achieve the best balance between risk transfer and risk retention subject to default risk.
When the size of a reinsurance company is small or when it has less diversified underwriting portfolios, the default risk is usually dependent on the issued risks. For instance, Scottish Re, which mainly operated life insurance business, eventually bankrupted in 2008 during the financial crisis. On the contrary, the default risk of the reinsurer can be assumed to be independent of the insurer’s loss in general when the reinsurer’s fiscal capacity is strong enough so that the default is generated due to the reinsurer’s other business lines. The story of the AIG meltdown is an evident example. Other examples are Munich Re and Swiss Re, both of which experienced severe suffering during the financial crisis, but the losses were mainly caused by investment risk, not their well-diversified underwriting portfolios. Research works on optimal (re)insurance design with counterparty’s risk burgeoned from Cummins and Mahul (Reference Cummins and Mahul2003), Mahul and Wright (Reference Mahul and Wright2004), and Mahul and Wright (Reference Mahul and Wright2007), to name a few. In recent actuarial literature, the use of an independent Bernoulli variable to denote the default event is not rare; see, for example, Bernard and Ludkovski (Reference Bernard and Ludkovski2012) addressing that the assumption is sufficiently rich to be of theoretical interest, and Chi et al. (Reference Chi, Hu and Huang2023) pinpointing such assumption can be met when the reinsurer’s default is mainly induced by investment risks while its underwriting risk is well-managed and fully diversified globally. The present study also implemented the independent assumption between default event and underlying loss.
Upon the reinsurer’s default, the insurer may still have the chance to obtain some part of the promised compensation, that is, partial recovery from the reinsurer. Optimal reinsurance design under the reinsurer’s partial recovery has attracted much attention in recent years. For example, Bernard and Ludkovski (Reference Bernard and Ludkovski2012) investigated the impact of counterparty risk on contract design under a multiplicative default risk model with partial recovery. In their studies, the reinsurer is assumed to be risk-neutral, while the insurer is risk-averse and uses either expected utility (EU) or a CTE risk criterion. Asimit et al. (Reference Asimit, Badescu and Cheung2013) studied optimal reinsurance design within the framework of VaR or convex distortion risk measures and modeled the default risk allowing the possibility that the reinsurer fails to pay the full agreed-upon indemnity whenever it exceeds the level of regulatory capital calculated by the VaR of the ceded loss. Besides, both parties may have different beliefs about the recovery rate, yet it is likely that the insurer is more pessimistic than the reinsurer. Slightly different from Asimit et al. (Reference Asimit, Badescu and Cheung2013), Cai et al. (Reference Cai, Lemieux and Liu2014) made the default assumption that the reinsurer may fail to pay the promised amount when the promised indemnity exceeds the total of the reinsurer’s initial capital regulated by VaR and the reinsurance premium, rather the initial capital itself. In the presence of the regulatory initial capital and the counterparty default risk, the authors investigated optimal reinsurance designs from the insurer’s point of view and derived optimal reinsurance strategies that maximize the expected utility of an insurer’s terminal wealth or minimize the VaR of an insurer’s total retained risk. Albrecher and Cani (Reference Albrecher and Cani2019) discussed the potential of randomizing stop-loss treaties for efficient risk management by introducing additional external randomness in the determination of the retention function for a given occurred loss, and explored the reason why and to what extent randomizing a treaty can be beneficial for the insurer who aims to maximize his expected net profit under regulatory solvency constraints and cost of capital considerations. While randomized contracts rarely exist in insurance practice, it is theoretically equivalent to the mechanism of optimal reinsurance design under the potential default risk of the reinsurer. Boonen and Jiang (Reference Boonen and Jiang2022) studied the optimal insurance design subject to an incentive compatibility condition from the perspective of an insured when there is a possibility for the insurer to default on its promised indemnity, where the premium is determined by the expected value coverage and the insured employs the mean-variance preference. Recently, Reichel et al. (Reference Reichel, Schmeiser and Schreiber2022) explored the ideal reinsurance coverage structure from the point of view of the insurer by incorporating counterparty risk, where a cost criterion is established to determine the optimal level of hedging reinsurers’ counterparty risk. Chi et al. (Reference Chi, Hu and Huang2023) revisited the study of an optimal risk management strategy for an insurer who wants to maximize the expected utility by purchasing reinsurance and managing reinsurer’s counterparty risk with a default-free hedging instrument. The ex-post moral hazard is excluded by imposing the no-sabotage condition and the optimal strategy is derived analytically.
However, to the best of the authors’ knowledge, there is little study on optimal reinsurance design subject to the reinsurer’s default risk when the insurer adopts a general distortion risk measure and the probability of default risk is modeled as a Bernoulli variable, let alone taking into consideration the reinsurer’s profit in the contract design. Our present work intends to fill this gap by studying the optimal reinsurance design in the presence of reinsurer’s default risk under distortion risk measures.
Compared with the existing studies, the contributions of our paper are as follows:
-
(i) The reinsurer’s default event/probability is modeled by a Bernoulli random variable independent of the underlying risk, which is very different from the default mechanism adopted by Asimit et al. (Reference Asimit, Badescu and Cheung2013) and Cai et al. (Reference Cai, Lemieux and Liu2014). Though this setting seems to be simpler compared with the dependence setting considered in both Bernard and Ludkovski (Reference Bernard and Ludkovski2012) and Boonen and Jiang (Reference Boonen and Jiang2022), it is assumed here that the insurer employs a general convex distortion risk measure. This setting is more general than the CTE measure studied in Bernard and Ludkovski (Reference Bernard and Ludkovski2012). Besides, the techniques used for the simplifications of distortion risk measures are based on the survival function of the insurer’s retained loss, which also differs by nature from the methods employed in Asimit et al. (Reference Asimit, Badescu and Cheung2013) and Cai et al. (Reference Cai, Lemieux and Liu2014).
-
(ii) Instead of directly utilizing the stop-loss treaty in designing the contract, we first provide a rigorous proof of the optimality of the stop-loss policies when the premium is charged according to the expected value premium principle. The studies in Bernard and Ludkovski (Reference Bernard and Ludkovski2012) and Albrecher and Cani (Reference Albrecher and Cani2019) assume the stop-loss compensation function a priori without mathematically justifying the setup. We then derive the explicit survival function of the reinsurer’s retained loss under which the distortion risk measure can be simplified into a nice expression. Three explicit and commonly used distortion functions, namely the TVaR, Gini, and Proportional Hazard (in short, PH) distortion, are considered in detail.
-
(iii) In contrast to the existing studies within the framework of default risk, we not only minimize the risk position of the insurer but also consider the reinsurer’s profit by deriving the optimal pricing coefficient by adopting the solution concept of Bowley solution. We present comprehensive numerical examples to demonstrate the sensitivity of the optimal deductible value with respect to default probability, safety loading, and recovery rate.
-
(iv) Under the default mechanism set by the event whether the compensated loss exceeds the sum of the initial capital and the premium charged from the contract, a recent follow-up working paper is Chen et al. (Reference Chen, Cheung and Zhang2024), which explores how the Bowley reinsurance contract is designed by a monopolistic reinsurer. The current paper differs substantially from theirs since the present work considers that the insurer employs any general convex distortion risk measure while their work assumes the insurer uses the VaR measure. Besides, the reinsurer is supposed to adopt the TVaR measure while their model considers a VaR-minimzation reinsurer.
The exposition of the paper is as follows. Section 2 first introduces necessary notation and then presents the problem formulations studied in the paper. Section 3 studies the optimal ceded loss function for the insurer by minimizing the distortion risk measures of the total retained loss. We first identify the optimality of the stop-loss treaty and then solve optimal deductible level for three explicit distortion functions employed by the insurer corresponding to the TVaR, Gini, and PH distortion measures. In Section 4, we search for the optimal safety loading by maximizing the reinsurer’s net profit. Section 5 presents numerical examples and sensitivity analysis to demonstrate our main findings. Section 6 concludes the paper. All proofs of the main results are delegated to the online supplementary file.
2. Problem formulation
Let
$\mathbb{R}=(\!-\infty, \infty)$
,
$\mathbb{R}_+=[0, \infty)$
,
$\mathbb{R}_{++}=\mathbb{R}_{+}\setminus\{0\}$
and
$\overline{\mathbb{R}}_+=\mathbb{R}_+\cup\{\infty\}$
. We denote the aggregate loss facing the insurer by the nonnegative random variable X, whose distribution function and survival function are given by
$F_X$
and
$\bar{F}_X$
, respectively. We assume that X is not degenerate at 0, and has a finite mean, that is,
$0< \mathbb{E}[X]< \infty$
. In general, X can have a point mass at zero and an absolutely continuous density on
$\mathbb{R}_{++}$
. Specifically, the event
$\{X=0\}$
may occur with a positive probability, that is,
$0< F_X(0)< 1$
. With a bit abuse of notation, the probability density function of X on the absolutely continuous part is denoted by
$f_X$
. Throughout, we denote the indicator function by
$\mathbf{1}_A(x)$
such that
$\mathbf{1}_A(x)=1$
if
$x\in A$
, otherwise 0.
Consider a two-point random variable Y, independent of X, such that
$\mathbb{P}(Y=1)=p$
and
$\mathbb{P}(Y=\gamma)=1-p$
for some fixed
$p \in(0,1]$
.Footnote 2 The parameter
$\gamma\in[0,1)$
denotes the recovery rate in the case of default.Footnote 3 The parameter p is interpreted as the performance probability. When
$0< p< 1$
, the reinsurer will default with probability
$1-p$
, resulting in a partial performance, which is in contrast to the full performance case when
$p=1$
. The terms “partial performance” and “full performance” are used in Doherty and Schlesinger (Reference Doherty and Schlesinger1990), Mahul and Wright (Reference Mahul and Wright2004), and Bernard and Ludkovski (Reference Bernard and Ludkovski2012). By paying reinsurance premium, the insurer can receive the following reinsurance payment from the reinsurer:

Once default, the reinsurer compensates a proportion of promised I(X), say
$\gamma I(X)$
, where
$\gamma$
is the (partial) recovery rate. As a result, the retained loss of the insurer after purchasing such a reinsurance contract is

Following the literature, admissible ceded loss functions
$I(\!\cdot\!)$
(or the so-called indemnity functions) come from the following collection:

The imposed conditions ensure that
$\mathcal{I}$
is 1-Lipschitz. The first condition satisfies the principle of indemnity, that is, the reinsurer compensates nothing if the loss is 0, and the ceded loss to the reinsurer should not exceed the realized loss. The second condition is known as the incentive-compatible (IC) constraint; it is also called the no-sabotage condition.
Throughout this paper, it is assumed that the reinsurer adopts the classical expected value premium principle,
$\pi_r(\!\cdot\!)=(1+\theta)\mathbb{E}[\cdot]$
,
$\theta\geq 0$
, to calibrate reinsurance premiums.Footnote 4 Consequently, the reinsurance premium for the policy in (2.1) equals

Definition 2.1. A distortion function
$g\;:\; [0, 1]\rightarrow [0, 1]$
is a continuous, increasing function such that
$g(0)=0$
and
$g(1)=1$
. A risk measure
$\rho_g$
of a nonnegative random variable Z with a distortion function g is defined as

The set of all distortion functions is denoted by
$\mathcal{G}_{d}$
, and the set of all concave distortion functions is denoted by
$\mathcal{G}_{cv}$
.
Two prominent examples of distortion risk measures are the value-at-risk (VaR) and the tail Value-at-Risk (TVaR). The VaR and TVaR of a nonnegative random variable Z at a confidence level
$1-\alpha$
where
$\alpha \in(0,1)$
are defined as

and

provided that both integrals exist. Thus, VaR and TVaR are two distortion risk measures, corresponding to the distortion functions
$g(t)=\mathbf{1}_{(\alpha, 1]}(t)$
and
$g(t)=\min\{1,t/\alpha\}$
, respectively.
We assume that the insurer aims to minimize the risk of his retained loss as measured by a distortion risk measure, while the reinsurer aims to maximize her expected profit less the distortion-risk-measure-based reserve and cost. The distortion functions adopted by them are denoted by
$g_i$
and
$g_r$
, respectively. We want to derive the Bowley solution by studying the following sequential game between the insurer and the reinsurer:
-
• (Problem faced by the insurer) Knowing the safety loading
$\theta$ in the premium principle, the insurer wants to minimize the convex distortion risk measureFootnote 5 of his aggregate retained loss by solving
(2.4)where\begin{equation}\min_{I\in\mathcal{I}}\rho_{g_i}\left(R(X,Y)+\pi_r(I(X,Y))\right)\!,\end{equation}
$g_{i}\in\mathcal{G}_{cv}$ represents the risk-averse preference of the insurer. The optimal ceded loss function that solves this problem is denoted by
$I^*(\cdot;\;\theta)$ , which depends on the given premium loading
$\theta$ .
-
• (Problem faced by the reinsurer) Based on the knowledge about
$I^*(\cdot;\;\theta)$ derived from the previous step, the reinsurer is profit-seeking and aims to maximize her net earnings by solving the following problem:
(2.5)where\begin{equation}\max_{\theta\in\mathbb{R}_+} \pi_r(YI^*(X;\;\theta))-C(YI^*(X;\;\theta))-\rho_{g_r}(YI^*(X;\;\theta)),\end{equation}
$g_r\in\mathcal{G}_{d}$ denotes the distortion function employed by the reinsurer, and
$C(\!\cdot\!)$ is a cost function. Usually, we take the form of
$C(\!\cdot\!)$ as
$C(\!\cdot\!)=c\mathbb{E}[\cdot]$ , where
$c\geq 0$ is a fixed constantFootnote 6; see Raviv (Reference Raviv1979) and Cheung et al. (Reference Cheung, Yam and Zhang2019) for similar assumptions. The optimal premium loading is denoted as
$\theta^*$ . Thus, the corresponding optimal ceded loss function is
$I^*(\cdot;\;\theta^*)$ . The pair
$(\theta^*,\ I^*(\cdot;\;\theta^*))$ of solutions among the two parties is called the Bowley solution of the problems described above.
Remark 2.2. The distortion risk measures are closely related to Yaari’s dual theory, which is often adopted in decision making on various research areas such as optimal (re)insurance and risk management. For example, useful classical references that link it to actuarial science can be found in Wang (Reference Wang1996) and Wang and Young (Reference Wang and Young1998). Our work assumed that the reinsurer adopts a distortion function denoted by
$g_r$
. The corresponding dual distortion function is denoted by
$\tilde{g}_r(t)=1-g_r(1-t)$
for
$t\in[0,1]$
. The terminal earnings, which are evaluated by the distortion risk measure for the reinsurer, can be expressed by

where
$D_{\tilde{g}_r}$
is the Yaari’s dual utility and

By applying the translation invariance property of Yaari’s dual utility, the optimization problem becomes

Hence, the reinsurer’s problem is equivalent to maximizing Yaari’s dual utility of her terminal wealth. When
$g_r$
is concave, we have
$\tilde{g}_r$
is convex, and the reinsurer is endowed with a risk-averse attitude. Hence, it becomes natural to comprehend Problem (2.5) if we look at it from the utility side. The transformation of Problem (2.4) for the insurer can be implemented in a similar manner.
3. Optimal ceded loss function for the insurer
In this section, we solve Problem (2.4). The solution relies on first proving the optimality of stop-loss treaties by using the convex ordering.
Definition 3.1 (Denuit et al., Reference Denuit, Dhaene, Goovaerts and Kaas2006). Given two random variables X and Y such that
$\mathbb{E}[X]=\mathbb{E}[Y]$
, X is said to be smaller than Y in the convex order, written as
$X\leq_\textrm{cx}Y$
, if
$\mathbb{E}[(X-d)_+]\leq \mathbb{E}[(Y-d)_+]$
for all
$d\in\mathbb{R}$
; or equivalently,
$\mathbb{E}[\phi(X)]\leq \mathbb{E}[\phi(Y)]$
for all convex functions
$\phi\;:\; \mathbb{R}\rightarrow \mathbb{R}$
.
The following lemma plays a key role in our subsequent analysis.
Lemma 3.2 (Ohlin, Reference Ohlin1969). Let X be a random variable,
$h_1$
and
$h_2$
be increasing functions such that
$\mathbb{E}[h_1(X)] = \mathbb{E}[h_2(X)]$
. If there exists
$x_0\in\mathbb{R}\cup \{\infty\}$
such that
$h_1(x)\geq h_2(x)$
for all
$x< x_0$
and
$h_1(x)\leq h_2(x)$
for all
$x > x_0$
, then
$h_1(X)\leq_\textrm{cx} h_2(X)$
.
We first show that the stop-loss treaty is optimal compared with any given admissible ceded loss
$I\in \mathcal{I}$
in the sense of the convex order. All proofs of propositions and theorems in this paper are delegated in the supplement file.
Theorem 3.3. Any admissible ceded loss function I(X) in Problem (2.4) is suboptimal to the stop-loss contract
$I_d(X)\;:\!=\;(X-d)_+$
, where
$d\in \overline{\mathbb{R}}_+$
is such that
$\mathbb{E}[(X-d)_+]=\mathbb{E}[I(X)]$
.
Remark 3.4. While Theorem 3.3 is based on the actuarial premium principle, the result remains valid for any premium principle of the form
$h(\mathbb{E}(\!\cdot\!))$
, where h belongs to the class

Here, the expected value premium principle corresponds to
$h(x)=(1+\theta)x\ (\theta> 0)$
, which obviously belongs to
$\mathcal{H}$
.
In light of Theorem 3.3, Problem (2.4) boils down to solving the following simplified problem:

where we only need to determine the value of the optimal deductible level by minimizing the risk position of the insurer. The original problem is now reduced to a one-dimensional one.
According to (2.3), one can note that
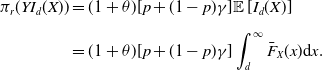
Let
$R_d(X, Y)\;:\!=\;X-I_d(X, Y)$
. The following result computes the exact expression of the survival function of
$R_d(X, Y)$
.
Proposition 3.5. For any given
$d\in \overline{\mathbb{R}}_+$
, the survival function of
$R_d(X,Y)$
is given by
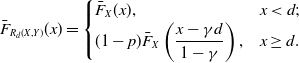
By combining (3.2) with (3.3), and utilizing the translation invariant property of distortion risk measures, the expression of the objective function in (3.1) can be simplified as
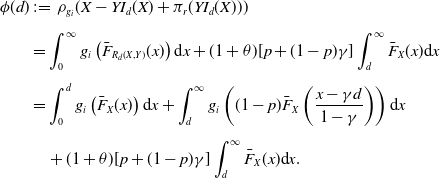
Taking the derivative of
$\phi(d)$
gives rise to

Minimizing
$\phi(d)$
over
$d\in\overline{\mathbb{R}}_+$
would be difficult if we do not know the explicit expression of
$g_i$
. In what follows, we discuss three examples of distortion risk measures:
-
• TVaR risk measure:
$g_{i}(t)=\min\{1,t/\alpha\}$ ,
$\alpha\in(0,1)$ ;
-
• Gini’s distortion principle:
$g_{i}(t)=(1+r)t-rt^2$ ,
$r\in(0,1)$ ;
-
• Proportional hazard (PH) transform distortion:
$g_{i}(t)=t^k$ ,
$k\in(0,1]$ .
All these three distortion functions are increasing and concave, and thus belong to the class
$\mathcal{G}_{cv}$
. Note, however, that the distortion of TVaR is not strictly concave, while the Gini’s distortion function and the PH transform distortion with
$k\in(0, 1)$
are strictly increasing and concave. The reasons we handpick these three representative distortion risk measures are based on the following rationales. Both VaR and TVaR are recognized measures within the banking and insurance sectors, notably in frameworks such as Basel III and Solvency II. The transition from VaR to TVaR for regulatory purposes has been advocated in 2012, as documented in the Basel Committee on Banking Supervision.Footnote 7 The observed limitations of VaR prompted the shift so that tail risks during periods of financial market distress can be better captured. Gini’s distortion principle originates from the Gini mean difference, initially proposed by Corrado Gini. Its connection to distortion risk measures is expounded in Denneberg (Reference Denneberg1990). Moreover, it maintains a close relationship with economic welfare theory and various other research domains, as elaborated in Yitzhaki and Schechtman (Reference Yitzhaki and Schechtman2013). The PH distortion risk measure features a non-flat tail distortion function in calculating the insurance premium, see Wang (Reference Wang1995).
Remark 3.6. It is worth mentioning that our model can be extended to incorporate budget constraints. Suppose the insurer has a premium budget, denoted by
$\tilde{\pi}> 0$
. Then the insurer’s problem is formulated as

It can be shown that the stop-loss contract is still optimal based on Lemma 3.2 and Theorem 3.3. Let
$\tilde{d}$
satisfy

Problem (3.4) can then be reformulated as

In this case, the optimal deductible determined in the next several sections on
$d\geq0$
can be naturally adapted to the range
$d\geq \tilde{d}$
.
Remark 3.7. It should be noted that the assumption on the constant partial recovery rate can be extended to an arbitrary random variable that is independent of
$X$
. To see this, suppose the recovery rate is described by a random variable
$\Gamma$
, which takes values in [0, 1)Footnote 8 and is independent of X. The retained loss function becomes
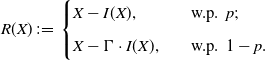
One can prove that stop-loss contracts remain optimal by using Lemma 3.2 and Theorem 3.3, together with the fact that the convex order is closed under independent mixtures; see Theorem 3.A.12 of Shaked and Shanthikumar (Reference Shaked and Shanthikumar2007).
3.1. TVaR distortion
In this subsection, we consider the case where the insurer chooses TVaR as the distortion risk measure with
$g_{i}(t)=\min\{1,t/\alpha\}$
,
$\alpha\in(0,1)$
. In this case,

By a change-of-variable, the above expression becomes

For fixed performance probability p, safety loading
$\theta$
, and confidence level
$\alpha$
, the optimal deductible can be derived explicitly and are summarized in the following theorem.
Theorem 3.8. Let
$ \kappa\;:\!=\;1/((1+\theta)(p+(1-p)\gamma)+(1-p)(1-\gamma)/\alpha)$
and
$\nu\;:\!=\;\gamma/((1+\theta)(p+$
$(1-p)\gamma))$
. The optimal deductible
$d^*$
is determined as follows.
Case 1.
$\bar{F}_X(0)\leq \alpha$
.
-
(a) If
$1/\alpha\geq (1+\theta)$ , then
$d^*=0$ (full reinsurance);
-
(b) otherwise if
$1/\alpha< (1+\theta)$ , then
$d^*=\infty$ (no business).
Case 2.
$\bar{F}_X(0)> \alpha$
.
-
(a) For
$p\geq 1-\alpha/\bar{F}_X(0)$ ,
-
(i) if
$\alpha< \kappa< \bar{F}_X(0)$ , then a finite
$d^*=\bar{F}^{-1}_X(\kappa)$ ;
-
(ii) if
$\bar{F}_X(0)\leq \kappa$ , then
$d^*=0$ ;
-
(iii) if
$\kappa=\alpha$ , then
$d^*\geq \bar{F}^{-1}_X(\alpha)$ ;
-
(iv) otherwise if
$\kappa< \alpha$ , then
$d^*=\infty$ .
-
(b) For
$0< p< 1-\alpha/\bar{F}_X(0)$ ,
-
(i) if
$\alpha< \kappa \leq \alpha/(1-p)$ , then
$d^*=\bar{F}^{-1}_X(\kappa)$ ;
-
(ii) if
$\kappa> \alpha/(1-p)$ , let
$\nu=\gamma/((1+\theta)(p+(1-p)\gamma))$ , if
$\nu< \bar{F}_X(0)$ , then
$d^*=\bar{F}^{-1}_X(\nu)$ ; if
$\nu\geq \bar{F}_X(0)$ , then
$d^*=0$ ;
-
(iii) if
$\kappa=\alpha$ , then
$d^*\geq \bar{F}^{-1}_X(\alpha)$ ;
-
(iv) otherwise if
$\kappa< \alpha$ , then
$d^*=\infty$ ;
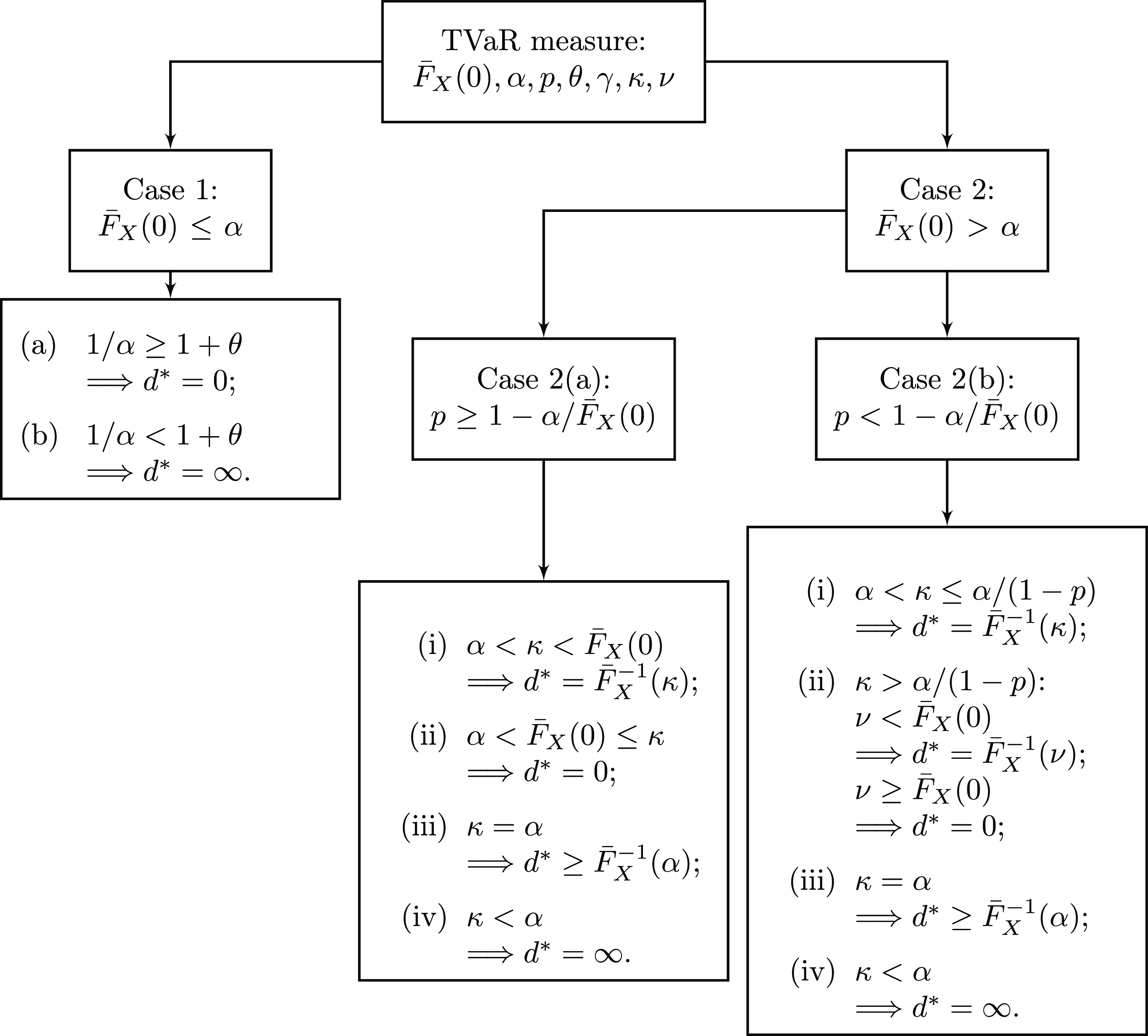
Figure 1. Flowchart illustration of Theorem 3.8.
The optimal deductibles
$d^*$
depicted in Theorem 3.8 are schematically represented by the flowchart in Figure 1. The findings of Theorem 3.8 can be understood as follows:
-
• When
$\bar{F}_X(0)\leq \alpha$ , the probability that the aggregate loss is zero is higher than
$1-\alpha$ . If the reinsurance premium is reasonable from the insurer’s perspective (i.e.,
$1/\alpha\geq 1+\theta$ ), he will buy reinsurance and indeed full reinsurance is optimal for him; otherwise, paying a high premium to cover the risk that is less likely to occur is irrational.
-
• When
$\bar{F}_X(0)> \alpha$ , the situations become more complicated, and multiple factors determine the optimal deductible level:
-
– (a) If
$p\geq 1-\alpha/\bar{F}_X(0)$ , the default is unlikely to occur. The relations between
$\kappa$ ,
$\alpha$ , and p affect the choice of optimal deductible level: if
$\alpha< \kappa< \bar{F}_X(0)$ , the loading
$\theta$ is subject to a range, indicating that the premium is charged within a range. It is optimal for the insurer to pay a premium in exchange for a stop-loss contract with deductible
$d^*=\bar{F}^{-1}_X(\kappa)$ ; when
$\bar{F}_X(0)\leq \kappa$ , the premium is charged at a lower loading level, so buying full reinsurance contract is optimal for the insurer; if
$\kappa=\alpha$ , we know that
$1/\alpha=1+\theta$ , displaying an equality relationship between confidence level and loading. From the insurer’s perspective, any deductible level equal to or greater than
$\bar{F}^{-1}_X(\alpha)$ is optimal; otherwise, when
$\kappa< \alpha$ , we have
$1/\alpha< 1+\theta$ . As for the insurer, it is optimal not to buy any reinsurance because
$1/\alpha< 1+\theta$ somehow means that the premium is too expensive.
-
– (b) If
$p< 1-\alpha/\bar{F}_X(0)$ , there is a higher chance that the default might happen. The relations between
$\alpha$ , p,
$\kappa$ , and
$\nu$ influence the choice of optimal deductible level: if
$\alpha< \kappa\leq \alpha/(1-p)$ , the premium is charged within a range, then it is optimal for the insurer to buy a stop-loss contract with deductible
$d^*=\bar{F}^{-1}_X(\kappa)$ ; if
$\alpha/(1-p)< \kappa$ , we further define
$\nu$ , satisfying
$\nu> \kappa$ , which affects the choice of optimal deductible level. If
$\nu< \bar{F}_X(0)$ , the premium is charged in a lower range, and it is still optimal for the insurer to buy a stop-loss contract with deductible
$d^*=\bar{F}^{-1}_X(\nu)$ . If
$\nu\geq \bar{F}_X(0)$ , the premium is charged at an even lower rate, and it is optimal to buy full reinsurance; if
$\kappa=\alpha$ , similar arguments apply that buying a stop-loss treaty with deductible equal to or greater than
$\bar{F}_X^{-1}(\alpha)$ is optimal to the insurer; otherwise if
$\kappa\leq \alpha$ , given the tendency of default and the exceedingly expensive premium, it is not optimal for an insurer to buy any reinsurance.
Remark 3.9. In Section 3.2 of Bernard and Ludkovski (Reference Bernard and Ludkovski2012), under the assumption that the aggregate loss random variable is continuously distributed and there is no point mass at zero, the authors investigate the optimal deductible using the CTE (which is the same as the TVaR in this case) when the default probability is loss-dependent. The indemnity function is assumed a priori to be a stop-loss treaty, lacking theoretical justification to which the current work contributes in Theorem 3.8. If the probability of a full recovery is a constant
$=p_0$
, corresponding to the loss-independent setup in this work, when
$1+\theta< 1/\alpha$
, the unique optimal deductible within
$\left(\bar{F}_X^{-1}(\alpha/(1-p)), \bar{F}_X^{-1}(\alpha)\right)$
is given by
$d^*=\bar{F}^{-1}_X(\kappa)$
. This case is covered in Case 2 of Theorem 3.8.
When
$\gamma=0$
, we arrive at a particular case where the reinsurer compensates nothing if the default occurs. In this case, Theorem 3.8 reduces to the following:
Corollary 3.10. Let
$\tilde{\kappa}\;:\!=\;1/((1+\theta)p+(1-p)/\alpha)$
. When
$\gamma=0$
, the optimal deductible
$d^*$
is determined as follows:
Case 1.
$\bar{F}_X(0)\leq \alpha$
.
-
(a) If
$1/\alpha\geq (1+\theta)$ , then
$d^*=0$ ;
-
(b) otherwise if
$1/\alpha< (1+\theta)$ , then
$d^*=\infty$ .
Case 2.
$\bar{F}_X(0)> \alpha$
.
-
(a) For
$p\geq 1-\alpha/\bar{F}_X(0)$ ,
-
(i) if
$\alpha< \tilde{\kappa}< \bar{F}_X(0)$ , then
$d^*=\bar{F}^{-1}_X(\tilde{\kappa})$ ;
-
(ii) if
$\alpha< \bar{F}_X(0)\leq \tilde{\kappa}$ , then
$d^*=0$ ;
-
(iii) if
$\tilde{\kappa}=\alpha$ , then
$d^*\geq\bar{F}^{-1}_X(\alpha)$ ;
-
(iv) otherwise if
$\tilde{\kappa}< \alpha$ , then
$d^*=\infty$ .
-
-
(b) For
$0< p< 1-\alpha/\bar{F}_X(0)$ ,
-
(i) if
$\alpha< \tilde{\kappa} \leq \alpha/(1-p)$ , then
$d^*=\bar{F}^{-1}_X(\tilde{\kappa})$ ;
-
(ii) if
$\tilde{\kappa}=\alpha$ , then
$d^*\geq\bar{F}^{-1}_X(\alpha)$ ;
-
(iii) if
$\tilde{\kappa}< \alpha$ , then
$d^*=\infty$ .
-
The case where
$p=1$
corresponds to the situation that the reinsurer will never default. Theorem 3.8 then reduces to the following:
Corollary 3.11. When
$p=1$
, the optimal deductible
$d^*$
is determined as follows:
Case 1.
$\bar{F}_X(0)\leq \alpha$
.
-
(a) If
$1/\alpha\geq (1+\theta)$ , then
$d^*=0$ ;
-
(b) otherwise if
$1/\alpha< (1+\theta)$ , then
$d^*=\infty$ .
Case 2.
$\bar{F}_X(0)> \alpha$
.
-
(a) if
$\alpha< 1/(1+\theta)< \bar{F}_X(0)$ , then
$d^*> 0$ and it is given by
$d^*=\bar{F}^{-1}_X(1/(1+\theta))$ ;
-
(b) if
$\alpha< \bar{F}_X(0)\leq 1/(1+\theta)$ , then
$d^*=0$ ;
-
(c) if
$\alpha=1/(1+\theta)$ , then
$d^*\geq\bar{F}^{-1}_X(1/(1+\theta))$ ;
-
(d) otherwise if
$1/(1+\theta)< \alpha$ , then
$d^*=\infty$ .
Case 2(a) and 2(c) of Corollary 3.11 echo the classical findings in Theorem 3.1 of Cai and Tan (Reference Cai and Tan2007). Our results enumerate all possible situations that might occur and articulate the optimal strategies the insurer should adopt.
3.2. Gini’s distortion
As for the Gini’s distortion principle,
$g'_{i}(t)=(1+r)-2rt$
, for
$r\in(0,1)$
, which leads to
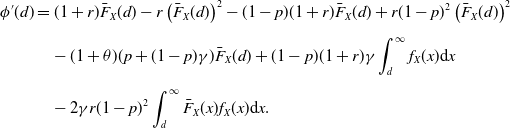
We can calculate the two integrals above as

As a result,

For fixed performance probability p, safety loading
$\theta$
, and Gini coefficient r, the optimal deductible can then be found, and the result is summarized in the following theorem.
Theorem 3.12. Let
$\zeta\;:\!=\;(r-\theta)(p+(1-p)\gamma)/(r(1-(1-p)^2(1-\gamma))$
. The optimal deductible
$d^*$
is given as follows:
-
(a) If
$r \leq \theta$ , then
$d^*=\infty$ .
-
(b) Assume
$r> \theta$ . If
$0< \zeta< \bar{F}_X(0)$ , then
$d^*=\bar{F}^{-1}_X(\zeta)$ ; if
$\zeta\geq \bar{F}_X(0)$ , then
$d^*=0$ .
Proof. We know that

If
$r\leq \theta$
, it is easy to see
$\phi'(d)\leq 0$
. As
$\phi(d)$
is decreasing on
$\mathbb{R}_+$
, we have
$d^*=\infty$
. Otherwise, if
$r> \theta$
, we have

Letting
$\phi'(d)=0$
,
$d=\bar{F}^{-1}_X(\zeta)$
is a potential optimal deductible level. If
$0< \zeta< \bar{F}_X(0)$
, it is clear that
$d^*=\bar{F}^{-1}_X(\zeta)$
. However, if
$\zeta\geq \bar{F}_X(0)$
,
$\phi'(d)\geq 0$
on
$\overline{\mathbb{R}}_+$
, so
$\phi(d)$
is increasing, and hence
$d^*=0$
.
Corollary 3.13. When
$p=1$
so that there is no default risk, the optimal deductible
$d^*$
is given as follows:
-
(a) If
$r\leq \theta$ , then
$d^*=\infty$ .
-
(b) Assume
$r> \theta$ . If
$0< 1-\theta/r< \bar{F}_X(0)$ , then
$d^*=\bar{F}^{-1}_X(1-\theta/r)$ ; if
$1-\theta/r\geq \bar{F}_X(0)$ , then
$d^*=0$ .
3.3. PH transform distortion
For the PH transform, we have
$g_i(t)=t^k$
,
$k\in (0, 1]$
and
$g_{i}'(t)=kt^{k-1}$
. In this case,
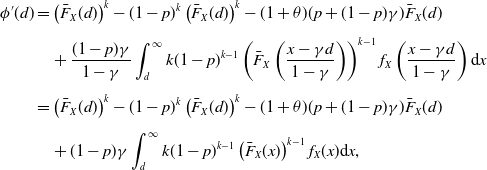
in which the integral in the last line can be calculated as
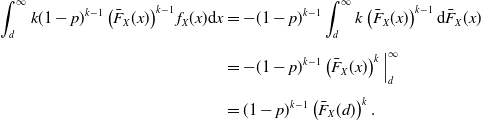
Consequently, we obtain a more concise expression of
$\phi'(d)$
:

For fixed performance level p, safety loading
$\theta$
, and PH index k, the optimal deductible is derived in the next theorem.
Theorem 3.14. Let
$\eta\;:\!=\;(1+\theta)(p+(1-p)\gamma)/(1-(1-\gamma)(1-p)^k)$
. The optimal deductible
$d^*$
is determined as follows:
-
(a) If
$\eta> (\bar{F}_X(0))^{k-1}$ , then
$d^*=\bar{F}^{-1}_X(\eta^{1/(k-1)})$ ;
-
(b) otherwise if
$\eta \leq (\bar{F}_X(0))^{k-1}$ , then
$d^*=0$ .
Proof. We have

If
$\eta> (\bar{F}_X(0))^{k-1}$
, when
$0\leq d< \bar{F}^{-1}_X(\eta^{1/(k-1)})$
,
$\phi'(d)< 0$
, and
$\phi(d)$
is decreasing; when
$d> \bar{F}^{-1}_X(\eta^{1/(k-1)})$
,
$\phi'(d)\geq 0$
, so
$\phi(d)$
is increasing. Therefore, the optimal deductible equals
$d^*=\bar{F}^{-1}_X(\eta^{1/(k-1)})$
. Otherwise, if
$\eta \leq (\bar{F}_X(0))^{k-1}$
, we have
$\phi'(d)\geq 0$
, so
$\phi(d)$
is increasing on
$\mathbb{R}_+$
. It then follows that
$d^*=0$
.
Corollary 3.15. When
$p=1$
so that there is no default risk, the optimal deductible
$d^*$
is given as follows:
-
(a) If
$1+\theta> (\bar{F}_X(0))^{k-1}$ , then
$d^*=\bar{F}^{-1}_X((1+\theta)^{1/(k-1)})$ ;
-
(b) otherwise if
$1+\theta \leq (\bar{F}_X(0))^{k-1}$ , then
$d^*=0$ .
As per Corollary 3.15, given a fixed loading
$\theta$
, the two parties always agree with a stop-loss treaty or full reinsurance treaty under the setting of PH transform distortion measure.
4. Optimal safety loading for the reinsurer
The objective of this section is to determine the optimal safety loading
$\theta^*$
when the reinsurer determines the reinsurance premium, where the reinsurer employs the TVaR measure with
$g_r(t)=\min\{1,t/\beta\}$
,
$1>\beta> 0$
, and the insurer adopts the three distortion measures studied in Section 3. To study this problem, we need the following result on the exact expression of
$I_d(X, Y)$
.
Proposition 4.1. For any given
$d\in \overline{\mathbb{R}}_+$
, the survival function of
$I_d(X,Y)$
is given by

The objective function in (2.5) can be re-expressed as

where
$d^*$
is an implicit function of
$\theta$
. From (4.1), we see that
$\bar{F}_{I_{d^*}(X, Y)}(x)$
is decreasing in x for a given
$d^*$
since it is a linear combination of two decreasing functions
$\bar{F}_X(x/\gamma+d^*)$
and
$\bar{F}_X(x+d^*)$
. Moreover, we find that

Suppose there exists an
$x_{\theta}\in\mathbb{R}_{++}$
such that
$\bar{F}_{I_{d^*}(X,Y)}(x_{\theta})=\beta$
. Then
$\psi(\theta)$
becomes
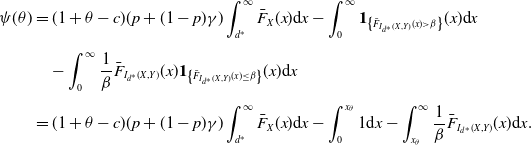
Otherwise, if such an
$x_{\theta}$
does not exist,
$\bar{F}_{I_{d^*}}(X,Y)\leq \beta$
, and hence
$\psi(\theta)$
becomes

Differentiating
$\psi(\theta)$
with respect to
$\theta$
yields
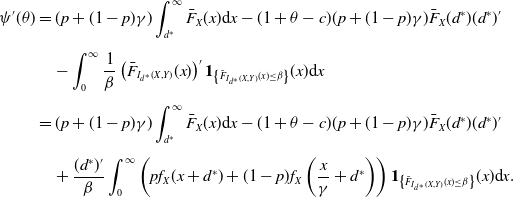
4.1. TVaR-TVaR case
This part considers the Bowley solution under the setting where both the insurer and the reinsurer employ the TVaR measures. As the insurer is usually more risk averse than the reinsurer, we assume that the respective confidence levels satisfy
$1>\beta>\alpha> 0$
.
As in Theorem 3.8, when
$\kappa=\alpha$
, that is,
$1/\alpha=1+\theta$
, the insurer is indifferent between
$d^*_1$
,
$d^*_2$
as long as both of them are greater than
$\bar{F}^{-1}_X(\alpha)$
. However, if the reinsurer increases the loading
$\theta$
, it becomes more expensive for the insurer to transfer the risk. In the limiting case as
$\theta\rightarrow \infty$
, we obviously have
$d^*\rightarrow \infty$
so that the insurer will not reinsure his risk. In the general framework, the reinsurer usually takes the lead and has the advantage over the insurer. The reinsurer will encourage insurers to buy contracts that would offer better coverage. Even though the insurer is indifferent between two deductibles as long as they yield the same minimum risk level, the reinsurer will choose to provide a contract with a lower deductible. In the following analysis of the reinsurer’s problem, we assume whenever
$\kappa=\alpha$
in Theorem 3.8, the optimal deductible
$d^*$
equals
$\bar{F}^{-1}_X(\alpha)$
.
In the sequel, we write
$\kappa_\theta$
,
$\nu_{\theta}$
instead of
$\kappa$
,
$\nu$
to indicate that they are functions of
$\theta$
. Regarding Theorem 3.8, we discuss solutions to the reinsurer’s problem (2.5) as follows:
Case 1:
$\bar{F}_X(0)\leq \alpha$
.
-
(a) If
$1/\alpha\geq 1+\theta$ , then
$d^*=0$ . In this case, by (4.2), we have
(4.3)which is an increasing function of\begin{equation}\begin{split} \psi(\theta) &= (1+\theta-c)(p+(1-p)\gamma)\int_0^{\infty}\bar{F}_X(x)\textrm{d} x\\[5pt] &\quad-\int_0^{\infty} \min\left\{1,\frac{1}{\beta}\left(p\bar{F}_X(x)+(1-p)\bar{F}_X(x/\gamma)\right)\right\}\textrm{d} x,\end{split}\end{equation}
$\theta$ .
-
(b) Otherwise if
$1/\alpha< 1+\theta$ , then
$d^*=\infty$ and
$\psi(\theta)=0$ .
Case 2:
$\bar{F}_X(0)> \alpha$
.
-
(a) For
$p\geq 1-\alpha/\bar{F}_X(0)$ , we find the optimal
$\theta^*$ by examining relationships between
$\kappa_{\theta}$ ,
$\alpha$ and p.
-
(i) If
$\alpha\leq \kappa_{\theta}< \bar{F}_X(0)$ , then
$d^*=\bar{F}^{-1}_X(\kappa_{\theta})$ . We now have
(4.4)\begin{equation}\begin{split} \psi'(\theta) &=(p+(1-p)\gamma)\int_{\bar{F}^{-1}_X(\kappa_{\theta})}^{\infty} \bar{F}_X(x)\textrm{d} x+(1+\theta-c){(p+(1-p)\gamma)}\frac{\kappa_{\theta} \kappa'_{\theta}}{f_X(\bar{F}^{-1}_X(\kappa_{\theta}))}\\[5pt] &\quad -\frac{p\kappa'_{\theta}}{\beta f_X(\bar{F}^{-1}_X(\kappa_{\theta}))}\int_0^{\infty} f_X(x+\bar{F}_X^{-1}(\kappa_{\theta}))\mathbf{1}_{\left\{\bar{F}_{I_{d^*}(X,Y)}(x)\leq \beta\right\}}(x)\textrm{d} x\\[5pt] &\quad -\frac{(1-p)\kappa'_{\theta}}{\beta f_X(\bar{F}^{-1}_X(\kappa_{\theta}))}\int_0^{\infty}f_X\left(\frac{x}{\gamma}+\bar{F}_X^{-1}(\kappa_{\theta})\right)\mathbf{1}_{\left\{\bar{F}_{I_{d^*}(X,Y)}(x)\leq \beta\right\}}(x)\textrm{d} x.\end{split}\end{equation}
Denote the solution set of
$\psi'(\theta)=0$
by
$\mathcal{A}^*\;:\!=\;\{\theta_1, \theta_2, \cdots\}$
. Define

-
• If
$\iota> 0$ , we let
$\mathcal{A}_{1,\iota}^*=\left\{\theta\in\mathcal{A}^*\;:\;\iota < \theta\leq 1/\alpha-1\right\}$ denote the admissible solution set;
-
• otherwise if
$\iota\leq 0$ , we define
$\mathcal{A}_{2,\iota}^*=\left\{\theta\in\mathcal{A^*}\;:\; 0\leq \theta\leq 1/\alpha-1\right\}$ .
-
(ii) If
$\bar{F}_X(0)\leq \kappa$ , then
$d^*=0$ . In this case,
$\psi(\theta)$ has the expression of (4.3).
-
(iii) Otherwise if
$\kappa_{\theta}< \alpha$ , we have
$d^*=\infty$ . In this case,
$\psi(\theta)=0$ .
-
(b) For
$0< p< 1-\alpha/\bar{F}_X(0)$ , we consider the following cases:
-
(i) if
$\alpha\leq \kappa_{\theta}\leq \alpha/(1-p)$ , then
$d^*=\bar{F}_X^{-1}(\kappa_\theta)$ . In this case,
$\psi'(\theta)$ is again given by (4.4). As in Case 2(a)(i) above, we denote the solution set of the equation
$\psi'(\theta)=0$ by
$\mathcal{A}^*\;:\!=\;\{\theta_1, \theta_2, \cdots\}$ . Define
\begin{equation*}\varphi=(1-p)\gamma/(\alpha(p+(1-p)\gamma))-1.\end{equation*}
-
• If
$\varphi> 0$ , we let
$\mathcal{A}_{1,\varphi}^*=\left\{\theta\in\mathcal{A^*}\;:\; \varphi \leq\theta\leq 1/\alpha-1\right\}$ denote the admissible solution set;
-
• otherwise if
$\varphi\leq 0$ , we define
$\mathcal{A}_{2,\varphi}^*=\left\{\theta\in\mathcal{A^*}\;:\; 0\leq \theta\leq 1/\alpha-1\right\}$ .
-
(ii) If
$\kappa_{\theta}> \alpha/(1-p)$ and
$\alpha/(1-p)< \nu_{\theta}< \bar{F}_X(0)$ , then
$d^*=\bar{F}_X^{-1}(\nu_{\theta})$ . The derivative function of
$\psi(\theta)$ now equals
(4.5)\begin{equation}\begin{split} \psi'(\theta) &=(p+(1-p)\gamma)\int_{\bar{F}^{-1}_X(\nu_{\theta})}^{\infty} \bar{F}_X(x)\textrm{d} x+(1+\theta-c){(p+(1-p)\gamma)}\frac{\nu_{\theta} \nu'_{\theta}}{f_X(\bar{F}^{-1}_X(\nu_{\theta}))}\\[5pt] &\quad -\frac{p\nu'_{\theta}}{\beta f_X(\bar{F}^{-1}_X(\nu_{\theta}))}\int_0^{\infty} f_X(x+\bar{F}_X^{-1}(\nu_{\theta}))\mathbf{1}_{\left\{\bar{F}_{I_{d^*}(X,Y)}(x)\leq \beta\right\}}(x)\textrm{d} x\\[5pt] &\quad -\frac{(1-p)\nu'_{\theta}}{\beta f_X(\bar{F}^{-1}_X(\nu_{\theta}))}\int_0^{\infty}f_X\left(\frac{x}{\gamma}+\bar{F}_X^{-1}(\nu_{\theta})\right) \mathbf{1}_{\left\{\bar{F}_{I_{d^*}(X,Y)}(x)\leq \beta\right\}}(x)\textrm{d} x.\end{split}\end{equation}
As before,
$\mathcal{A^*}=\{\theta_1, \theta_2, \cdots\}$
denotes the solution set of
$\psi'(\theta)=0$
. Further, we let

-
• If
$\xi> 0$ , we let
$\mathcal{A}_{1,\xi,\varphi}^*=\left\{\theta\in\mathcal{A^*}\;:\; \xi< \theta< \varphi\right\}$ denote the admissible solution set;
-
• if
$\xi\leq 0$ and
$\varphi> 0$ , we define
$\mathcal{A}_{2,\xi,\varphi}^*=\left\{\theta\in\mathcal{A^*}\;:\; 0\leq \theta< \varphi\right\}$ ;
-
• otherwise if
$\varphi\leq 0$ , we define
$\mathcal{A}_{3,\xi,\varphi}^*=\{0\}$ , which is a singleton.
Moreover, if
$\bar{F}_X(0)\leq \nu_{\theta}$
, we have
$d^*=0$
. The expression of
$\psi(\theta)$
is given by (4.3).
(iii) If
$\kappa_{\theta}< \alpha$
, we have
$d^*=\infty$
. In this case,
$\psi(\theta)=0$
.
Theorem 4.2. The optimal safety loading
$\theta^*$
of the reinsurer’s problem (2.5) and the Bowley solution
$(\theta^*, d^*(\theta^*))$
Footnote 9 is summarized as follows.
Case 1.
$\bar{F}_X(0)\leq \alpha$
.
-
(a) If
$0\leq \theta\leq 1/\alpha-1$ ,
$d^*=0$ . The Bowley solution is given by
$(1/\alpha-1, 0)$ .
-
(b) Otherwise, if
$1/\alpha-1< \theta$ ,
$d^*=\infty$ . The Bowley solution is given by
$(\theta^*, \infty)$ for all
$\theta^*> 1/\alpha-1$ .
Case 2.
$\bar{F}_X(0)> \alpha$
.
-
(a1)
$p\geq 1-\alpha/\bar{F}_X(0)$ and
$\kappa_{\theta}\geq \alpha$ . (i) if
$\kappa_{\theta}< \bar{F}_X(0)$ ,
$d^*=\bar{F}^{-1}_X(\kappa_{\theta})$ . If
$\iota> 0$ , the possible optimal loading is denoted by
$\theta^*_{1,\iota}=\textrm{arg max}_{\theta\in \mathcal{A}_{1,\iota}^*\cup\{1/\alpha-1\}} \psi(\theta)$ ; otherwise if
$\iota\leq 0$ , we denote
$\theta^*_{2,\iota}=\textrm{arg max}_{\theta\in \mathcal{A}_{2,\iota}^*\cup\{0\}\cup\{1/\alpha-1\}} \psi(\theta).$ (ii) Otherwise if
$\bar{F}_X(0)\leq \kappa_{\theta}$ ,
$d^*=0$ , then we denote
$\theta^*_{\iota}=\max(\iota,0)$ . Hence, the optimal safety loading is concluded by
\begin{equation*} \theta^*=\textrm{arg max}_{\theta\in\{\theta^*_{1,\iota},\theta^*_{2,\iota}, \theta^*_{\iota}\}} \psi(\theta), \end{equation*}
$(\theta^*, d^*(\theta^*))$ .
-
(a2)
$p\geq 1-\alpha/\bar{F}_X(0)$ and
$\kappa_{\theta}< \alpha$ . In this case,
$d^*=\infty$ . The Bowley solution is given by
$(\theta^*, \infty)$ for all
$\theta^*> 1/\alpha-1$ .
-
(b1)
$0< p< 1-\alpha/\bar{F}_X(0)$ and
$\kappa_{\theta}\geq\alpha$ . (i) If
$\kappa_{\theta} \leq \alpha/(1-p)$ , then
$d^*=\bar{F}^{-1}_X(\kappa_{\theta})$ . If
$\varphi> 0$ , we denote
$\theta^*_{1,\varphi}=\textrm{arg max}_{\theta\in \mathcal{A}_{1,\varphi}^*\cup\{1/\alpha-1\}} \psi(\theta);$ otherwise if
$\varphi\leq 0$ , we denote the possible optimal loading by
$\theta^*_{2,\varphi}=\textrm{arg max}_{\theta\in \mathcal{A}_{2,\varphi}^*\cup\{0\}\cup\{1/\alpha-1\}} \psi(\theta).$ (ii) If
$\kappa_{\theta}> \alpha/(1-p)$ , let
$\nu_{\theta}=\gamma/((1+\theta)(p+(1-p)\gamma))$ . If
$\nu_{\theta}< \bar{F}_X(0)$ , we know
$d^*=\bar{F}^{-1}_X(\nu_{\theta})$ . If
$\xi> 0$ , we denote
$\theta^*_{1,\xi}=\textrm{arg max}_{\theta\in \mathcal{A}^*_{1,\xi,\varphi}} \psi(\theta);$ if
$\xi\leq 0$ and
$\varphi> 0$ , we denote
$\theta^*_{2,\xi}=\textrm{arg max}_{\theta\in \mathcal{A}_{2,\xi,\varphi}^*\cup\{0\}} \psi(\theta);$ otherwise if
$\varphi\leq 0$ , then
$\theta^*_{3,\xi}=0$ . Furthermore, if
$\nu_{\theta}\geq \bar{F}_X(0)$ ,
$d^*=0$ and we denote
$\theta^*_{\xi}=\max(\xi,0)$ . Hence, the optimal safety loading is determined by
\begin{equation*} \theta^*=\textrm{arg max}_{\theta\in\{\theta^*_{1,\varphi},\theta^*_{2,\varphi}, \theta^*_{1,\xi},\theta^*_{2,\xi},\theta^*_{3,\xi}, \theta^*_{\xi}\}} \psi(\theta), \end{equation*}
$(\theta^*, d^*(\theta^*))$ .
-
(b2)
$0< p< 1-\alpha/\bar{F}_X(0)$ and
$\kappa_{\theta}< \alpha$ . In this case,
$d^*=\infty$ . The Bowley solution is given by
$(\theta^*, \infty)$ for all
$\theta^*> 1/\alpha-1$ .
The various cases described in Theorem 4.2 are schematically shown in Figure 2. The takeaways of Theorem 4.2 are as follows:
-
• When
$\bar{F}_X(0)\leq \alpha$ and the reinsurance premium is set within a reasonable range (i.e.,
$0\leq \theta\leq 1/\alpha-1$ ), the optimal contract is full reinsurance, corresponding to
$d^*=0$ . For the profit-seeking purpose, the reinsurer would charge the premium as much as possible. Thus, the optimal safety loading would be
$\theta^*=1/\alpha-1$ , and the complete Bowley solution is given by
$(\theta^*,d^*(\theta^*))=(1/\alpha-1, 0)$ .
-
• When
$\bar{F}_X(0)> \alpha$ , multiple parameters determine the Bowley solution. The comparisons regarding
$\kappa_{\theta}$ associated with a certain parameter select local optimal value for
$\theta$ . A comparison among local solutions that achieve maximum for the reinsurer’s objective determines the global maximum
$\theta^*$ . The pair
$(\theta^*, d^*(\theta^*))$ denotes the Bowley solution.
-
– (a1) If
$p\geq 1-\alpha/\bar{F}_X(0)$ , and
$\kappa_{\theta}\geq \alpha$ , we introduce a parameter
$\iota$ . By comparing
$\kappa_{\theta}$ and
$\bar{F}_X(0)$ , there would be three local solutions parameterized by
$\iota$ , that is,
$\theta^*_{1,\iota}$ ,
$\theta^*_{2,\iota}$ , and
$\theta^*_{\iota}$ . The final step is to select the global solution that yields the maximum objective value from the set
$\{\theta^*_{1,\iota}, \theta^*_{2,\iota}, \theta^*_{\iota}\}$ .
-
– (a2) If
$p\geq 1-\alpha/\bar{F}_X(0)$ , and
$\kappa_{\theta}\geq \alpha$ , it is not optimal for the insurer to enter the reinsurance contract, that is,
$d^*=\infty$ . However, the Bowley solution can still be characterized as
$(\theta^*, \infty)$ as long as
$\theta^*> 1/\alpha-1$ .
-
– (b1) If
$0< p< 1-\alpha/\bar{F}_X(0)$ and
$\kappa_{\theta}\geq \alpha$ , we introduce parameters
$\varphi$ and
$\xi$ to find six local solutions. The global solution is selected from local solutions. The discussion is similar to that in (a1), and we omit it here.
-
– (b2) If
$0< p< 1-\alpha/\bar{F}_X(0)$ and
$\kappa_{\theta}> \alpha$ , the Bowley solution is given by
$(\theta^*, \infty)$ as long as
$\theta^*> 1/\alpha-1$ . The discussion is omitted as it is similar to (a2).
-
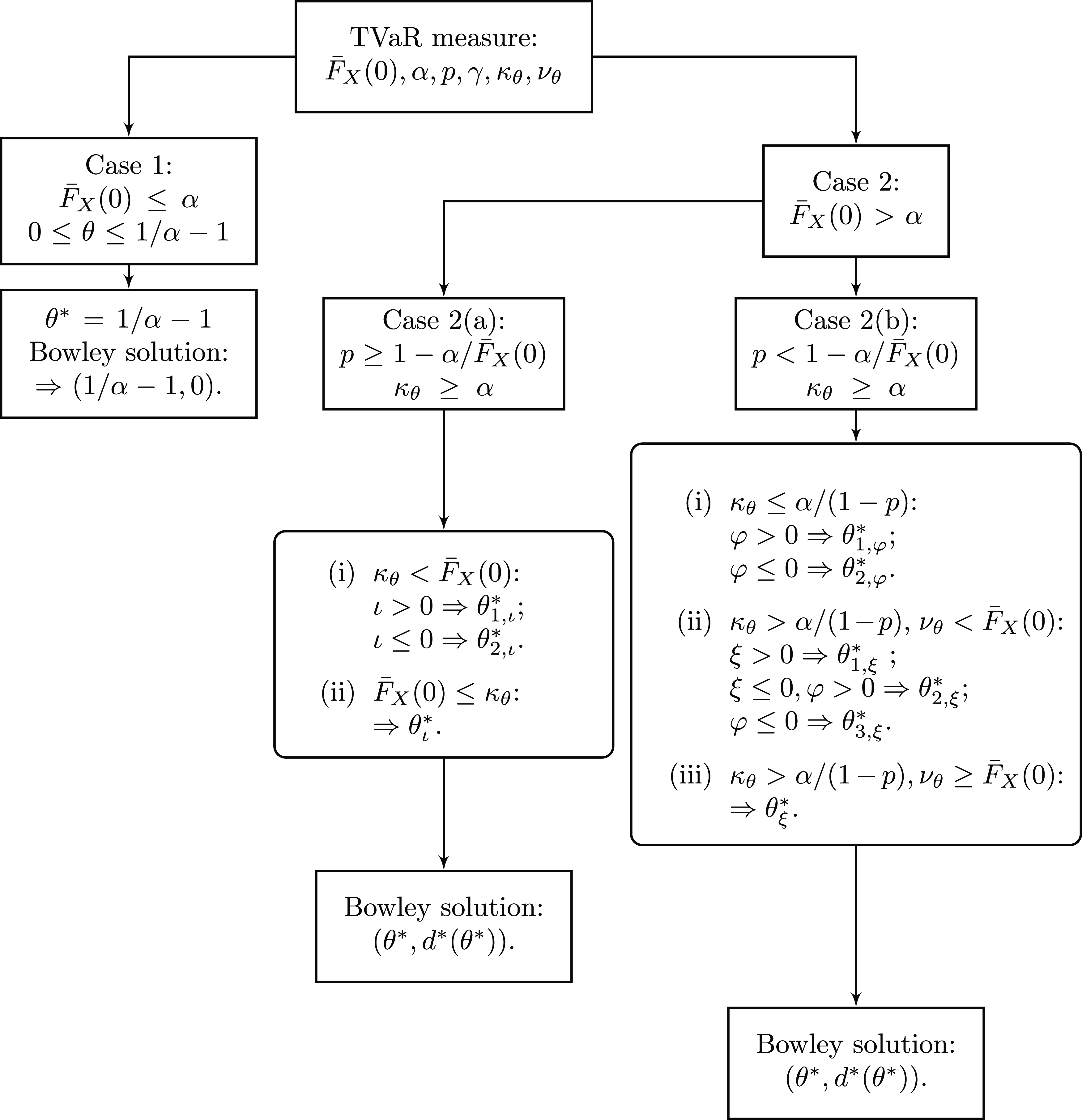
Figure 2. Flowchart illustration of Theorem 4.2 for finite
$d^*$
only.
4.2. Gini-TVaR case
When
$r\leq \theta$
, it is optimal for an insurer not to enter into any reinsurance arrangement (
$d^*=\infty$
). In this case, we discover that
$\psi(\theta)=0$
. Besides, the finite optimal deductible exists when
$r> \theta$
. We write
$\zeta_{\theta}$
instead of
$\zeta$
to emphasize the dependence on
$\theta$
. If
$0< \zeta_{\theta}< \bar{F}_X(0)$
, then
$d^*=\bar{F}_X^{-1}(\zeta_{\theta})$
. The derivative function
$\psi'(\theta)$
is given by

Let
$\mathcal{B^*}\;:\!=\;\{\theta_1, \theta_2,\cdots\}$
be the solution set of the equation
$\psi'(\theta)=0$
. Denote

-
• If
$\varrho> 0$ , we let
$\mathcal{B}_{1,\varrho}^*=\left\{\theta\in\mathcal{B^*}\;:\;\varrho < \theta< r\right\}$ denote the admissible solution set;
-
• otherwise if
$\varrho\leq 0$ , we define
$\mathcal{B}_{2,\varrho}^*=\left\{\theta\in\mathcal{B^*}\;:\; 0\leq \theta< r\right\}$ .
Moreover, if
$\zeta_{\theta}\geq \bar{F}_X(0)$
, then
$d^*=0$
. In this case,
$\psi(\theta)$
is given by (4.3), and it is increasing in
$\theta$
.
Theorem 4.3. The optimal safety loading
$\theta^*$
to Problem (2.5) is summarized as follows.
-
(a) If
$r\leq \theta$ ,
$d^*=\infty$ and the Bowley solution is given by
$(\theta^*, \infty)$ for all
$\theta^*\geq r$ .
-
(b1) If
$0< \zeta_{\theta}< \bar{F}_X(0)$ , we have
$d^*=\bar{F}_X^{-1}(\zeta_{\theta}).$ If
$\varrho> 0$ , we denote
$\theta^*_{1,\varrho}=\textrm{arg max}_{\theta\in \mathcal{B}_{1,\varrho}^*} \psi(\theta);$ otherwise if
$\varrho\leq 0$ , we denote
$\theta^*_{2,\varrho}=\textrm{arg max}_{\theta\in \mathcal{B}_{2,\varrho}^*\cup\{0\}} \psi(\theta).$ Hence, the optimal safety loading is given by
$\theta^*=\textrm{arg max}_{\theta\in \{\theta^*_{1,\varrho},\ \theta^*_{2,\varrho}\}} \psi(\theta)$ . The Bowley solution is characterized by
$(\theta^*, \bar{F}_X^{-1}(\zeta_{\theta^*}))$ .
-
(b2) Otherwise if
$\zeta_{\theta}\geq \bar{F}_X(0)$ , we have
$d^*=0$ . In this case, the optimal safety loading is
$\theta^*_{\varrho}=\max(\varrho, 0)$ . Hence, the Bowley solution is given by
$(\theta^*_{\varrho}, 0)$ .
4.3. PH-TVaR case
To emphasize the dependence on
$\theta$
, we write
$\eta_{\theta}$
in the sequel. If
$\eta_{\theta}> (\bar{F}_X(0))^{k-1}$
, then
$d^*=\bar{F}^{-1}_X(\eta_{\theta}^{1/(k-1)})$
and we have
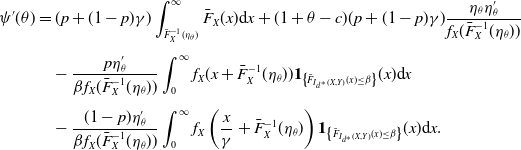
We denote by
$\mathcal{C}^*\;:\!=\;\{\theta_1, \theta_2,\cdots\}$
the solution set of
$\psi'(\theta)=0$
, and set

-
• If
$\varsigma> 0$ , we let
$\mathcal{C}_{1,\varsigma}^*=\left\{\theta\in\mathcal{C^*}\;:\;\varsigma < \theta< \infty\right\}$ denote the admissible solution set;
-
• otherwise if
$\varsigma\leq 0$ , we define
$\mathcal{C}_{2,\varsigma}^*=\left\{\theta\in\mathcal{C^*}\;:\; 0\leq \theta< \infty\right\}$ .
Moreover, if
$\eta_{\theta}\leq (\bar{F}_X(0))^{k-1}$
, then
$d^*=0$
. In this case,
$\psi(\theta)$
is expressed by (4.3) and increases in
$\theta$
.
Theorem 4.4. The optimal safety loading
$\theta^*$
to Problem (2.5) and the associated Bowley solution
$(\theta^*, d^*(\theta^*))$
are characterized as follows.
-
(a) If
$\eta_{\theta}> (\bar{F}_X(0))^{k-1}$ ,
$d^*=\bar{F}^{-1}_X(\eta_{\theta}^{1/(k-1)})$ . If
$\varsigma> 0$ , we denote
$\theta^*_{1,\varsigma}=\textrm{arg max}_{\theta\in \mathcal{C}_{1,\varsigma}^*} \psi(\theta);$ otherwise if
$\varsigma\leq 0$ , we denote
$\theta^*_{1,\varsigma}=\textrm{arg max}_{\theta\in \mathcal{C}_{2,\varsigma}^*\cup\{0\}} \psi(\theta).$ Hence, the optimal safety loading is determined by
$\theta^*=\textrm{arg max}_{\theta\in \{\theta^*_{1,\varsigma},\ \theta^*_{2,\varsigma}\}} \psi(\theta).$ The Bowley solution is characterized by
$(\theta^*, \bar{F}_X^{-1}(\eta_{\theta^*}))$ .
-
(b) Otherwise, if
$\eta_{\theta}\leq (\bar{F}_X(0))^{k-1}$ , we obtain
$d^*=0$ . In this case, the optimal
$\theta^*$ is decided by
$\theta^*_{\varsigma}=\max(\varsigma, 0)$ . Hence, the resulting Bowley solution is
$(\theta^*_{\varsigma}, 0)$ .
5. Numerical examples
In this section, several numerical examples are provided to illustrate the main results of this article. We assume that the distribution function of the aggregate loss X of the insurer is given by a shifted Pareto distributionFootnote 10; see Albrecher and Cani (Reference Albrecher and Cani2019) and Chi et al. (Reference Chi, Hu and Huang2023). The c.d.f. is parametrized as follows:
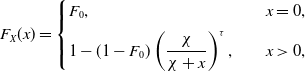
where
$F_0\;:\!=\;F_X(0)$
denotes the mass at
$x=0$
,
$\chi> 0$
is the scale parameter and
$\tau> 0$
is the shape parameter. For illustration purposes, we set
$\chi=1000$
,
$\tau=3$
, and
$F_0=0.3$
in the numerical examples.
5.1. Sensitivity analysis on the insurer’s optimal deductible level
In this subsection, we visually exhibit curves of optimal deductible
$d^*$
and investigate some quantitative properties of the resulting results for the insurer’s problem. By varying one parameter at a time, we analyze the effect of performance probability p, recovery rate
$\gamma$
, and safety loading
$\theta$
on optimal deductible
$d^*$
from the insurer’s side.
We start with an example where the TVaR measure with
$\alpha=0.05$
is adopted. Besides, we take
$\theta=0.1$
and
$\gamma=0.3$
. We plot
$d^*$
as a function of p in Figure 3. The two strategies, full insurance and the stop-loss contract, are denoted in red and blue color, respectively. In this example,
$\bar{F}_X(0)=0.7> \alpha$
, so only Case 2 of Theorem 3.8 is illustrated. The figure is separated into two regions by the rightmost red dashed vertical line located at
$p=1-\alpha/\bar{F}_X(0)\approx 0.929$
. For
$p\in(0, 0.929)$
, subcases are treated separately, see the left black dashed vertical line at
$p\approx 0.838$
corresponding to
$\kappa=\alpha/(1-p)$
. The highest point corresponds to
$p=0.838$
and
$d^*=315.1498$
, which means that the maximum optimal deductible value that the insurer would set is
$315.1498$
when the default probability is 0.162.

Figure 3. An illustration of
$d^*$
for the TVaR measure with
$\theta=0.1$
,
$\gamma=0.3$
and
$\alpha =0.05$
.

Figure 4. Effect of p on
$\kappa$
and
$\nu$
for the TVaR measure with
$\theta=0.1$
,
$\gamma=0.3$
and
$\alpha = 0.05$
.
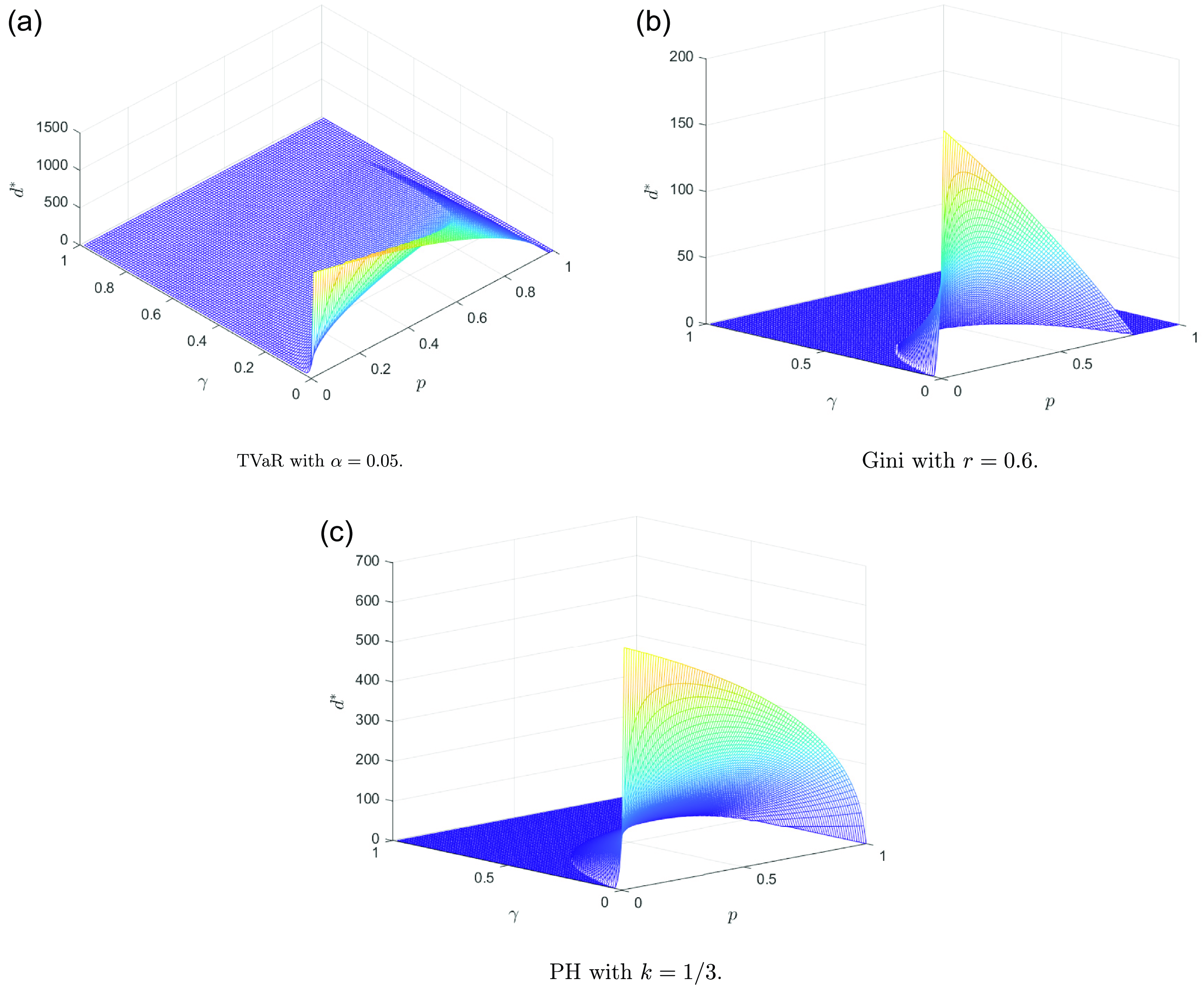
Figure 5. Effect of p and
$\gamma$
on
$d^*$
with
$\theta=0.1$
.

Figure 6. Effect of p and
$\theta$
on
$d^*$
with
$\gamma=0.3$
.
In Figure 3, the five labels
$\mathcal{R}_1,\ \mathcal{R}_2,\ \mathcal{R}_3,\ \mathcal{R}_4$
, and
$\mathcal{R}_5$
denote the following regions:
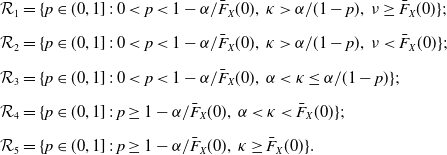
We further provide Figure 4 to address the relationship among strategies and shaded regions, which are determined by the effect of p on
$\kappa$
and
$\nu$
. Combining Theorem 3.8, we connect Figures 3 and 4 as follows:
-
• There are three subcases when
$p\in (0, 0.929)$ :
-
– for
$p\in \mathcal{R}_1$ , corresponding to the second part of Case (2b)(ii), reinsurance is cheap, and buying full insurance benefits the insurer most though the reinsurer may default;
-
– for
$p\in \mathcal{R}_2$ , corresponding to the first part of Case (2b)(ii), buying some reinsurance is desirable when the probability of full reimbursement is small;
-
– for
$p\in \mathcal{R}_3$ , corresponding to Case (2b)(i),
$d^*$ is contingent on the value of
$\kappa$ and decreasing in p.
-
• There are two subcases when
$p\in[0.929, 1]$ , representing an even higher possibility of full recovery:
-
– for
$p\in \mathcal{R}_4$ , corresponding to Case (2a)(i), the insurer continues to decrease his own retained loss;
-
– for
$p\in \mathcal{R}_5$ , corresponding to Case (2a)(ii), the insurer finds it optimal to buy full insurance even if the premium is expensive.
Figure 5 shows how the value of
$d^*$
is affected by the interaction between the performance probability p and the recovery rate
$\gamma$
for a fixed safety loading. When
$\gamma$
increases, the insurer pays more attention to the loading compared to the default risk. It agrees with the intuition that the optimal deductible decreases in general.

Figure 7. Effect of p and
$\alpha$
on
$d^*$
for the TVaR measure with
$\theta=0.1$
and
$\gamma=0.3$
.
In Figure 6, we examine the effects of the performance probability p and safety loading
$\theta$
on
$d^*$
for the three distortion functions, subject to a fixed recovery rate
$\gamma$
. As
$\theta$
increases, the optimal deductible increases. This makes sense because the more expensive the contract premium is, the more reluctant the insurer would be to purchase a reinsurance contract. The insurer would rather retain more risk by raising the deductible level. In the extreme case, if
$\theta\rightarrow \infty$
, we have
$d^*\rightarrow \infty$
. The insurer is discouraged from buying any reinsurance contract.
Moreover, for the TVaR measure, we show how the performance probability p and
$\alpha$
affect
$d^*$
with fixed
$\theta=0.1$
and
$\gamma=0.3$
in Figure 7. When default risk exists, a higher
$\alpha$
reflects a less prudent attitude of the insurer, so the value of
$d^*$
decreases. The insurer would be willing to transfer more risk to the reinsurer.
Two special scenarios are examined. When
$p=1$
, which corresponds to the no-default case, the values of the optimal level of deductibles for three distortion risk measures are plotted in Figure 8. As
$\theta$
increases, the optimal strategies switch from full insurance to a stop-loss treaty with increasing deductibles. It makes sense that when
$\theta$
is small, the premium is cheap and the insurer would transfer more risk. When
$\theta$
gets larger, the premium becomes more expensive, which discourages reinsurance. As we expect, even if full repayment is available,
$d^*\rightarrow \infty$
as
$\theta\rightarrow \infty$
, so the insurer will not reinsure his risk.
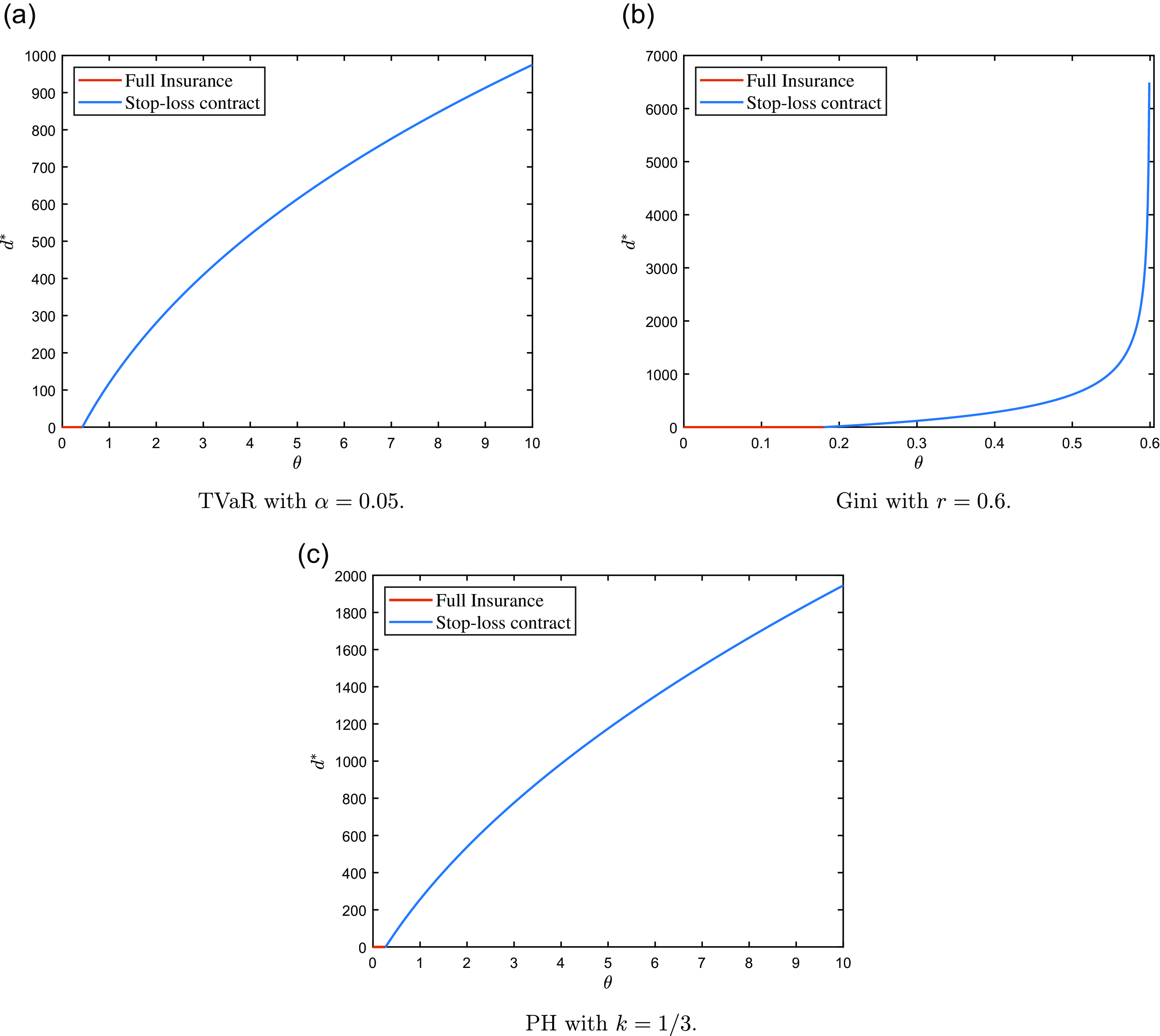
Figure 8. Effect of
$\theta$
on
$d^*$
when
$p=1$
.
When
$\gamma=0$
so that there is no compensation in case the reinsurer defaults, we pay attention to the effect of p and
$\theta$
on
$d^*$
for three distortion risk measures. The results are presented in Figure 9. When the loading is low and the full performance probability is high, the insurer opts for full insurance. Otherwise, a stop-loss reinsurance contract is optimal. As the loading
$\theta$
increases, the optimal deductible increases, reflecting that the insurer is discouraged from buying reinsurance.

Figure 9. Effect of
$\theta$
and p on
$d^*$
when
$\gamma=0$
.
5.2. Scenario analysis on the Bowley solution
In the following, we adopt a discretization method on
$\theta\in[0,\infty)$
, provide some examples to illustrate the reinsurer’s problem (2.5), and present the Bowley solutions.
Example 5.1
(TVaR Case). Suppose both the insurer and the reinsurer adopt the TVaR risk measure. The former sets a confidence level of
$1-\alpha$
, while the latter sets a confidence level
$1-\beta$
where
$\beta> \alpha$
. We take
$\alpha=0.05$
,
$\beta=0.1$
, and
$c=0.35$
.
-
(i) Suppose that
$\gamma=0.1$ and
$p=0.2< 0.929$ . For those
$\theta$ such that
$\kappa_{\theta}\leq \alpha/(1-p)=0.0625$ ,
$d^*> 0$ and
$\varphi=4.7143> 0$ . The optimal safety loading must belong to
$\mathcal{A}^*_{1,\varphi}$ . As
$\psi(\theta)$ is increasing,
$\theta^*_{1,\varphi}=1/\alpha-1=19$ . On the other hand, for those
$\theta$ such that
$\kappa_{\theta}> 0.0625$ , we have
$\nu_{\theta}< \bar{F}_X(0)$ , so
$d^*> 0$ . However, as
$\xi=-0.4898< 0$ , the optimal safety loading must belong to
$\mathcal{A}^*_{2, \xi, \varphi}$ . In this case,
$\theta^*$ does not exist. To sum up, the optimal safety loading is
$\theta^*=\theta^*_{1,\varphi}=19$ and the Bowley solution is
$(\theta^*,\ d^*(\theta^*))=(19,\ 1410.22)$ .
-
(ii) Suppose that
$\gamma=0.35$ and
$p =0.2< 0.929$ . For those
$\theta$ such that
$\kappa_{\theta}\leq 0.0625$ ,
$d^*> 0$ and
$\varphi=10.6667> 0$ . The optimal safety loading must belong to
$\mathcal{A}^*_{1,\varphi}$ . As
$\psi(\theta)$ is increasing, we obtain
$\theta^*_{1,\varphi}=1/\alpha-1=19$ . On the other hand, for those
$\theta$ such that
$\kappa_{\theta}> 0.0625$ and
$\nu_{\theta}< \bar{F}_X(0)$ ,
$d^*> 0$ . Since
$\xi=0.0417> 0$ , the optimal safety loading must belong to
$\mathcal{A}^*_{1, \xi, \varphi}$ . Furthermore, for those
$\theta$ such that
$\kappa_{\theta}> 0.0625$ and
$\nu_{\theta}\geq \bar{F}_X(0)$ ,
$d^*=0$ . In this case, the optimal safety loading is
$\theta^*_{\xi}=0.0417$ . To sum up, as
$\psi(\theta)$ is an increasing function, the optimal safety loading is
$\theta^*_{1,\varphi}=19$ , and the Bowley solution equals
$(\theta^*,\ d^*(\theta^*))=(19,\ 1410.22)$ .
-
(iii) Suppose that
$\gamma=0.35$ and
$p =0.95> 0.929$ ,
$\iota = -0.1953< 0$ . The optimal deductible is always positive, so the optimal safety loading must belong to admissible set
$\mathcal{A}^*_{2,\iota}$ . As
$\psi(\theta)$ is an increasing function,
$\theta^*=1/\alpha-1=19$ . The Bowley solution in this case is given by
$(\theta^*,\ d^*(\theta^*))=(19,\ 1410.22)$ .
-
(iv) Suppose that
$p=1$ . In this case, there is no default risk, and
$\iota=0.4286$ . For those
$d^*> 0$ , the optimal safety loading must belong to admissible set
$\mathcal{A}^*_{1,\iota}$ . In this case, we obtain
$\theta^*_{1,\iota}=1/\alpha-1=19$ . For those
$d^*=0$ , the maximum of
$\psi(\theta)$ is given by
$\theta_{\iota}=0.4286$ . Putting all these together,
$\theta^*=\theta^*_{1,\iota}$ , hence the Bowley solution is given by
$(\theta^*,\ d^*(\theta^*))=(19,\ 1410.22)$ .
It is noted that the Bowley solutions are given by the same strategy
$(\theta^*, d^*(\theta^*))=(19, 1410.22)$
under different parameter settings described above. This is due to the assumption in Section 4.1 on the insurer’s indifferent attitude when
$1+\theta=1/\alpha$
. In this case, the insurer would buy a stop-loss treaty with
$\bar{F}^{-1}_X(\alpha)$
being the deductible. As the reinsurer takes the lead and she is profit seeking, the reinsurer opts for selecting a loading which as large as possible.
Example 5.2
(TVaR Case). Suppose the insurer adopts Gini’s distortion principle while the reinsurer adopts the TVaR measure with a confidence level of
$1-\beta$
. We take
$r=0.6$
,
$c=0.35$
, and
$\beta=0.1$
.
-
(i) Suppose that
$\gamma=0.1$ and
$p=0.2$ ,
$\varrho=-0.036< 0$ . The optimal deductible is always positive, and the optimal safety loading must belong to admissible set
$\mathcal{B}^*_{2,\varrho}$ . However, the function (4.2) is increasing on [0,r), the
$\theta^*$ does not exist, nor does the Bowley solution.
-
(ii) Suppose that
$\gamma=0.1$ and
$p=0.6$ ,
$\varrho=0.0383> 0$ . For those
$d^*> 0$ , the optimal safety loading must belong to admissible set
$\mathcal{B}^*_{1,\varrho}$ . In this case, the objective function (4.2) is increasing on
$(\varrho,r)$ , and hence Bowley solution does not exist as the maximum is not attainable. However, for
$d^*=0$ ,
$\psi(\theta)$ attains it maximum at
$\theta=0.0383$ . The optimal safety loading
$\theta^*=0.0383$ and the Bowley solution exists, and it is given by
$(\theta^*,\ d^*(\theta^*))=(0.0383,\ 0)$ .
-
(iii) Suppose that
$\gamma=0.3$ and
$p=0.6$ ,
$\varrho=0.082> 0$ . For those
$d^*> 0$ , the optimal safety loading must belong to admissible set
$\mathcal{B}^*_{1,\varrho}$ . As in case (ii) above, the Bowley solution does not exist. However, for
$d^*=0$ ,
$\psi(\theta)$ attains it maximum at
$\theta=0.082$ . The optimal safety loading
$\theta^*=0.082$ , and the Bowley solution exists, and it is given by
$(\theta^*,\ d^*(\theta^*))=(0.082,\ 0)$ .
-
(iv) Suppose that
$p=1$ . When the default risk disappears,
$\varrho=0.18> 0$ . For those
$d^*> 0$ , the optimal safety loading must belong to admissible set
$\mathcal{B}^*_{1,\varrho}$ . Again, the Bowley solution does not exist. However, for
$d^*=0$ ,
$\psi(\theta)$ attains it maximum at
$\theta=0.18$ . The optimal safety loading
$\theta^*=0.18$ , and the Bowley solution exists, and it is given by
$(\theta^*,\ d^*(\theta^*))=(0.18,\ 0)$ .
Under the setting that the insurer adopts Gini’s distortion principle and
$\theta=r$
, the insurer will not enter into any reinsurance arrangement in the first place, which in turn gives rise to a jump point in the objective function
$\psi(\theta)$
. This results in the nonexistence of the Bowley solution in some cases.
Example 5.3
(TVaR Case). Suppose the insurer adopts PH transform distortion while the reinsurer adopts the TVaR measure with a confidence level of
$1-\beta$
. We take
$k=1/3$
,
$c=0.35$
, and
$\beta=0.1$
. In this example, we assume the shape parameter for the shifted Pareto distribution is 4 to ensure
$\alpha k> 1$
.
-
(i) Suppose that
$\gamma=0.1$ and
$p=0.2$ ,
$\varsigma=-0.2547< 0$ . The optimal deductible is always positive, so the optimal safety loading must belong to admissible set
$\mathcal{C}^*_{2,\varsigma}$ . The objective function (4.2) is maximized at
$\theta=92.15$ . In this case, the Bowley solution is solved to be
$(\theta^*,\ d^*(\theta^*))=(92.15,\ 5114.0116)$ .
-
(ii) Suppose that
$\gamma=0.3$ and
$p=0.2$ ,
$\varsigma=0.0095> 0$ . For those
$d^*> 0$ , the optimal safety loading must belong to admissible set
$\mathcal{C}^*_{1,\varsigma}$ . The maximum over
$\mathcal{C}^*_{1,\varsigma}$ is given by
$\theta^*_{1,\varsigma}=92.15$ . For
$d^*=0$ , the maximum of
$\psi(\theta)$ is
$\theta^*_{\varsigma}=0.0095$ . To sum up, the Bowley solution exists, and it is given by
$(\theta^*,\ d^*(\theta^*))=(92.15,\ 4456.3813)$ .
-
(iii) Suppose that
$\gamma=0.3$ and
$p=0.6$ ,
$\varrho=-0.1469< 0$ . The optimal deductible is always positive so the optimal safety loading must belong to admissible set
$\mathcal{C}^*_{2,\varsigma}$ . In this case, the Bowley solution is solved to be
$(\theta^*,\ d^*(\theta^*))=(92.15,\ 4811.9451)$ .
-
(iv) Suppose
$p=1$ ,
$\varsigma=0.2684> 0$ . For those
$d^*> 0$ , the optimal safety loading must belong to admissible set
$\mathcal{C}^*_{1,\varsigma}$ . In this case, the maximum is given by
$\theta^*_{1,\varsigma}=92.15$ . However, for those
$d^*=0$ ,
$\psi(\theta)$ attains it maximum at
$\theta=0.2684$ . The optimal safety loading
$\theta^*_{\varsigma}=0.2684$ . To sum up, the Bowley solution is given by
$(\theta^*,\ d^*(\theta^*))=(92.15,\ 4008.62)$ .
In all these four cases, one observes that the objective function
$\psi(\theta)$
increases first, then decreases to zero when
$\theta\rightarrow \infty$
. Given that p is fixed, a higher recovery rate encourages the insurer to select a lower deductible. On the other hand, if the recovery rate is fixed, a higher value of p leads to a more expensive premium, forcing the insurer to retain more risk and raising the deductible.
6. Concluding remarks
This article examines the optimal reinsurance design from the perspectives of both insurers and reinsurers while taking into account the potential default risk originating from the reinsurer’s end. The probability of default is characterized by an independent Bernoulli random variable consisting of two outcomes. The default risk affects the indemnity in a multiplicative way allowing for partial recovery while not covering the entire agreed-upon indemnity. In the scenario where the insurer employs convex distortion risk measures, we initially established the theoretical optimality of stop-loss treaties when the premium calculation is guided by the expected value premium principle. The insurer’s problem was then redirected to determining the optimal deductible. Explicit expressions for the optimal deductibles were derived for three specific risk measures: TVaR, Gini’s distortion principle, and PH transform distortion. Subsequently, we adhered to the concept of the Bowley solution and examined the optimal safety loading to maximize the reinsurer’s profit with TVaR being the risk measure regulating the reserve requirement. The examples demonstrated the main results of this paper in twofold. For the insurer’s scenario, comprehensive visual illustrations were presented to unveil the impacts of performance probability, recovery rate, and safety loading on the optimal deductible level. On the other hand, with risk preferences set by both the insurer and the reinsurer, numerical examples were also provided to delineate complete Bowley solutions.
Several possible developments or extensions are left for future research. For example, the insurer and the reinsurer might hold divergent beliefs on the default probability and the recovery rate. In this case, the objective functions can be properly modified to be linear ones, and our proposed procedures can still be applied. Another possible extension is to expand the range of the recovery rate levels so that more a general random variable Y can be accommodated, reflecting a more practical consideration. It is worth noting that our present work assumes that the insurer adopts a convex distortion risk measure, which excludes the case of the VaR measure. Therefore, it is interesting to separately investigate the optimal reinsurance contract under the VaR measure when there exists a default risk for the reinsurer.
Note that independence assumption of the default event from the loss is needed to facilitate these extensions. The most captivating direction is to relax such independence assumption by imposing certain dependence structures between the recovery rate and the loss. However, the reinsurance problem becomes difficult to solve when the default event is dependent on the insurer’s loss. For example, suppose that the dependence structure is introduced by replacing
$\gamma$
with
$\gamma(X)$
, the retained loss becomes
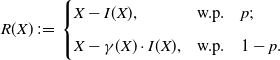
We may assume that
$0\leq \gamma(X)< 1$
and
$\gamma(x)$
is decreasing in x. While it is still possible that there exists a
$d\in\overline{\mathbb{R}}_+$
such that
$X-I(X)$
and
$X-(X-d)_+$
have the same premiums, we can no longer establish the convex order between
$X-\gamma(X)\cdot I(X)$
and
$X-\gamma(X)\cdot(X-d)_+$
. Such dependence setting impedes the facilitation of Lemma 3.2. We conjecture some notions of bivariate stochastic orderings might be helpful to simplify the problems.
Acknowledgements
The authors are grateful to Prof. Yichun Chi for helpful discussions on a preliminary version of this work. The authors sincerely acknowledge the Editor, Prof. Steven Vanduffel, and three anonymous reviewers for their insightful and constructive comments and suggestions, which have greatly improved the presentation of this manuscript. Ka Chun Cheung is supported by a grant from the Research Grants Council of the Hong Kong Special Administrative Region, China (Project No. 17303721). Yiying Zhang acknowledges the National Natural Science Foundation of China (No. 12101336, 72311530687), Guangdong Basic and Applied Basic Research Foundation (No. 2023A1515011806), and Shenzhen Science and Technology Program (No. JCYJ20230807093312026).
Competing interests
No potential competing or conflict interests were reported by the authors.
Supplementary material
To view supplementary material for this article, please visit https://doi.org/10.1017/asb.2024.24.