1. Introduction
There is a growing consensus that the rapid depletion and collapse of many shared common pool resources (CPRs) has ushered in the sixth mass extinction event. Peer monitoring and punishment has been highlighted as an important means to mitigate a ‘tragedy of the commons’, given the evidence that participants in both laboratory and field settings tend to punish free riders (Ostrom et al., Reference Ostrom, Gardner and Walker1994; Fehr and Gächter, Reference Fehr and Gächter2000). This tendency is often called ‘prosocial punishment’ to reflect individuals' willingness to incur personal costs to discipline free riders even in one-shot interactions (Herrmann et al., Reference Herrmann, Thöni and Gächter2008). This runs counter to predictions based on standard assumptions of payoff maximizing self-interested agents, which entail that a second-order dilemma will arise from a failure to engage in such a form of self-governance. A closer look at the experimental evidence, however, shows that past experimental studies have focused primarily on perfect peer monitoring, where everyone can monitor and punish everyone else appropriating from the shared CPR. The extant data do not reflect the incredible diversity of self-governance arrangements documented so far, of which imperfect monitoring and punishment institutions are an important part. Appropriators' opportunities to monitor and punish are frequently restricted due to biophysical and/or socio-political barriers. Some examples include imperfect monitoring and punishment arrangements around downstream irrigation systems, recreational fishing grounds and hunting wildlife, state boundaries, dykes and other walled structures, and exclusionary social categories like gender (Leader-Williams and Milner-Gulland, Reference Leader‐Williams and Milner‐Gulland1993; Ostrom et al., Reference Ostrom, Gardner and Walker1994; Agarwal, Reference Agarwal2001; Henrich et al., Reference Henrich, McElreath, Barr, Ensminger, Barrett, Bolyanatz, Cardenas, Gurven, Gwako, Henrich, Lesorogol, Marlowe, Tracer and Ziker2006; Ostrom, Reference Ostrom2010).
Our main contribution is to examine experimentally how differences in structural features of imperfect peer monitoring and punishment institutions impact cooperation in a nonlinear CPR dilemma. To this end, we systematically varied the following two features: the number of peers that an individual can monitor and punish in a given group, and whether the relationship between two individuals is symmetric (so that they can simultaneously monitor and punish one another). Specifically, we performed a lab experiment in which we assigned individuals to one of three types of networks which exogenously varied their ability to monitor and punish their neighbors in a one-shot nonlinear CPR dilemma: the complete network (CN), and the imperfect undirected and directed circle networks (UCN and DCN respectively). This allowed us to examine the causal role of two key structural properties: network density and directedness. Network density specifies the number of agents that an individual can monitor and punish in relation to the total number of individuals in the group (the number of existing ties divided by the number of possible ties). Directedness instead specifies whether the monitoring and punishment relationship between two agents is asymmetric (a unidirectional relationship between individuals such that each tie points from the monitor to the appropriator being monitored and, possibly, punished). The choice of UCN and DCN was also supported by the field observation that, while some agents may be temporarily or permanently isolated from others due to for instance geographical, political or socio-cultural constraints, they generally cannot be excluded from the benefits of the CPR.
The CN has the highest network density because all potential relationships between group members are actually realized: everyone can punish all other players since each individual is connected to everyone else. In such dense networks, it is plausible that agents will be able to deter over-appropriation more effectively provided that individuals in this treatment take advantage of the larger combined punishment capacity. However, this need not be the case; in fact, the opposite may happen if punishers struggle to coordinate. That is, denser networks may be less effective at deterring non-cooperative behavior if the threat of being punished diminishes as the number of potential targets increases and the coordination of prosocial punishment becomes more difficult. In addition, the increasing number of potential targets to sanction may reduce the severity of assigned sanctions. Conversely, incomplete and less dense networks like UCN may present a relatively clearer picture of the roles of individuals located at different nodes (and of the ensuing responsibility for deterring freeriding). Such monitoring and punishment roles are arguably clearer in the DCN, because the network structure specifies a single individual who can punish one target; by removing other potential punishers from the decision setting, this eliminates any ambiguity about the individual's responsibility.Footnote 1
Importantly, network density and directedness delineate the ‘burden of responsibility’ in different ways across appropriators in the CN, UCN and DCN. Research from both social psychology and experimental economics suggests that this element of responsibility is key, especially in the context of one-shot interactions. In social psychology, the ‘bystander effect’ consistently documents how the increased availability of other potential helpers – who are strangers – decreases the likelihood of helping across different settings (Fischer et al., Reference Fischer, Krueger, Greitemeyer, Vogrincic, Kastenmüller, Frey, Heene, Wicher and Kainbacher2011). To illustrate, Cryder and Loewenstein (Reference Cryder and Loewenstein2012) found that this burden of responsibility impacts prosocial choices in experimental social dilemmas: participants were three times more likely to donate money to others when they were solely responsible for a recipient's outcome than when this responsibility was shared with others.
Similarly, experimental economics research on ‘moral wiggle room’ also points to the importance of clearly defined rules between the giver, recipient, and payouts in influencing cooperation, primarily in dictator games (Dana et al., Reference Dana, Weber and Kuang2007). Dictators have no ‘wiggle room’ to interpret selfish behavior favorably when selfish dictators are easily recognized by recipients. But generous behavior decreases when responsibility is clouded, for instance when recipients do not know the range of payout or dictators can choose to randomly determine selfish versus fair payouts (Dana et al., Reference Dana, Weber and Kuang2007). While this finding has been replicated several times in the dictator game context, mixed results from other strategic settings question how generalizable these findings are (Lind et al., Reference Lind, Nyborg and Pauls2019). But taken together, contributions within this strand of literature suggest that when the burden of responsibility of acting prosocially is specified clearly to individuals, then cooperative actions are more likely.
Another strand of the experimental economics literature speaks to this question by varying the responsibility for punishment through exogenous changes to the monitoring and punishment network in public goods (PG) games and shows that outcomes are contingent on the network structure (Carpenter et al., Reference Carpenter, Kariv and Schotter2012; Leibbrandt et al., Reference Leibbrandt, Ramalingam, Sääksvuori and Walker2015; Boosey and Isaac, Reference Boosey and Isaac2016). For example, Carpenter et al. (Reference Carpenter, Kariv and Schotter2012), the closest study to ours, varied the network structure by restricting both monitoring and punishment opportunities in a linear PG contribution game with a strangers-matching protocol. They found that contributions are similar across CN and UCN networks, but that the punishment amount is highest in DCN, followed by UCN and lastly by CN. They argued that the network property of directedness clearly specified the responsibility of assigning punishment from a single agent to another: ‘more punishment is used to maintain or increase contributions in directed networks as the asymmetry in the relations between any pair of subjects gives different monitoring roles to different subjects, which, in turn, increases punishment expenditures’ (Carpenter et al., Reference Carpenter, Kariv and Schotter2012: 95). Leibbrandt et al. (Reference Leibbrandt, Ramalingam, Sääksvuori and Walker2015) held monitoring constant (by giving all subjects perfect feedback about other group member's choices) but varied punishment opportunities and found that the network topology significantly affected contributions and punishment decisions in PG games, but not overall efficiency levels. They argued that this is because the greater the number of people who can punish and be punished, the greater the contributions to the public good and the greater the amount of punishment used in the group. Extant studies, however, provide no direct evidence on how imperfect monitoring and punishment networks and punishment capacity impact CPR appropriation, punishment behavior and efficiency.
Finally, our study adds to a third strand of literature; that is, the literature on CPR dilemmas, which has largely focused on the benefits of perfect peer monitoring and punishment institutions.Footnote 2 In order to capture the complex dynamics of CPRs that appropriators typically face in the field, we modelled the CPR dilemma as a nonlinear dilemma, thus following the literature on nonlinear CPR experiments pioneered by Ostrom et al. (Reference Ostrom, Walker and Gardner1992). Table 1 summarizes experimental variables in related investigations.Footnote 3 Nearly all nonlinear CPR dilemma experiments without a punishment stage find that appropriation moves towards the Nash allocation following a pulsing pattern (see Ostrom, Reference Ostrom2006; Saijo et al., Reference Saijo, Feng and Kobayashi2017). Ostrom et al. (Reference Ostrom, Walker and Gardner1992) were the first to experimentally explore how adding perfect peer monitoring and sanctioning opportunities affected appropriation. They found that monitoring and sanctioning marginally mitigated over-appropriation relative to a baseline without any punishment, but that the punishment given was variable and the cost of sanctioning reduced payoffs to levels below what was the socially-optimal level when there was no opportunity to communicate with others in the group. Cason and Gangadharan (Reference Cason and Gangadharan2015) revisited Ostrom et al.'s (Reference Ostrom, Walker and Gardner1992) appropriation dilemma to confirm findings that peer sanctioning has a positive but limited impact on appropriation, highlighting that net earnings were not significantly different from the no punishment baseline. They noted that subjects may find it more difficult to distinguish prosocial from antisocial behavior since nonlinear payoffs push the social optimum and Nash equilibrium closer together. They also suggested that curtailing freeriding can be more challenging because the gains from defection are larger in the nonlinear CPR environment (relative to linear PG games). When replicating Cason and Gangadharan (Reference Cason and Gangadharan2015), Kingsley (Reference Kingsley2015) confirmed that punishment alone does not improve welfare in the CPR game despite increasing cooperation.
Table 1. Nonlinear CPR experiments with and without monitoring and punishment
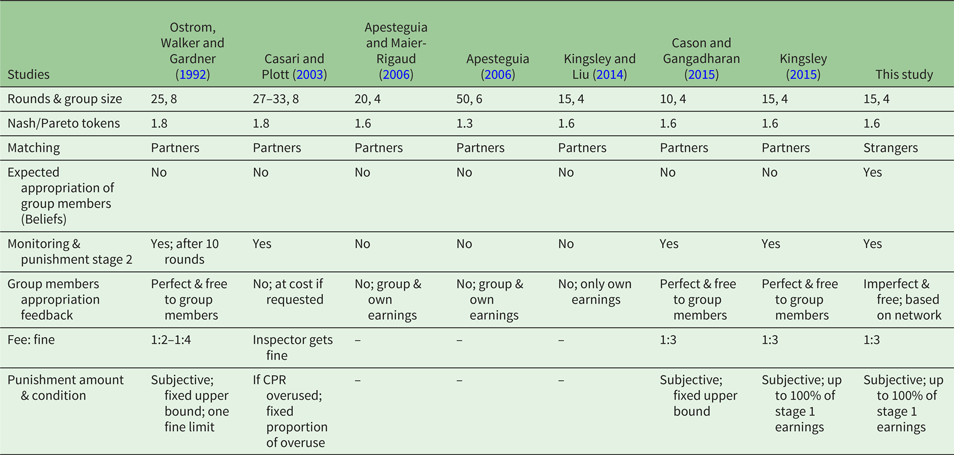
Notes: All studies reviewed here use lab experiments with students. The ratio of Nash/Pareto tokens determines the size of the externality (Apesteguia and Maier-Rigaud, Reference Apesteguia and Maier-Rigaud2006; Cason and Gangadharan, Reference Cason and Gangadharan2015).
Our experiment featured a nonlinear CPR dilemma similar to that of Ostrom et al. (Reference Ostrom, Walker and Gardner1992), Cason and Gangadharan (Reference Cason and Gangadharan2015) and Kingsley (Reference Kingsley2015). As discussed above, a consistent finding in this literature is that perfect institutions may improve cooperation but not necessarily efficiency. Based on this premise, and given that our main aim was to test the effect of ‘imperfect’ institutions that systematically reduce the ability to observe and punish peers (and thereby the burden and cost of acting prosocially), we chose the case of perfect peer monitoring and punishment as the comparison group. This design choice is similar to Boosey and Isaac (Reference Boosey and Isaac2016) and Carpenter et al. (Reference Carpenter, Kariv and Schotter2012) who also keep perfect monitoring and punishment as their baseline. However, since experiments with no monitoring and punishment represent another important baseline for comparison, we will also discuss our findings in relation to earlier CPR experiments without punishment (in the results section 3), while recognizing the many design differences (as noted in table 1). For instance, compared to prior CPR experiments that largely use a partner-matching protocol, we used stranger-matching to address potential reputation effects (discussed in section 2).
Another important design feature is the type of punishment used in this experiment. Compressing monitoring and punishment opportunities into the network structure is similar in spirit to institutions studied in Casari and Plott (Reference Casari and Plott2003) and De Geest et al. (Reference De Geest, Stranlund and Spraggon2017). In Casari and Plott's (Reference Casari and Plott2003) CPR experiment, an agent's choice to inspect her peers simultaneously revealed both the target's appropriation choice and sanctioning exacted, with an important distinction being that sanctioning is automatically assigned under certain conditions. De Geest et al. (Reference De Geest, Stranlund and Spraggon2017) studied the impact of imperfect monitoring and punishment between two groups of CPR users instead, where ‘insiders’ could simultaneously observe and sanction none, all or a subset of the ‘outsiders’ or ‘poachers’. While they observed little variation in appropriation, they found that insiders were unable to coordinate their sanctions to fully deter the outsiders in the partial and perfect monitoring and punishment treatments as their sanctions were too low and they were unwilling to punish low levels of poaching. We focused on how imperfect opportunities to monitor and punish fellow appropriators affect the management of the CPR.
Lastly, we also elicited information on beliefs that players hold about others' expected appropriations. Eliciting beliefs may induce subjects to think through their decisions more carefully and is thereby one means to address the previously voiced concern about the considerable confusion that may arise from the complexity of nonlinear environments noted by Cason and Gangadharan (Reference Cason and Gangadharan2015). Extant experiments that elicit beliefs over others' contributions in PG games (Croson, Reference Croson2000; Neugebauer et al., Reference Neugebauer, Perote, Schmidt and Loos2009; Fischbacher and Gächter, Reference Fischbacher and Gächter2010; Gächter and Renner, Reference Gächter and Renner2010; Smith, Reference Smith2013) or expected appropriation in CPR games (Velez et al., Reference Velez, Stranlund and Murphy2009), consistently finds that people's stated beliefs are ‘optimistic’ as they underestimate others' appropriations (or overestimate contributions). We examine whether beliefs vary due to the monitoring and punishment network structure, a question which is previously unexplored in the literature to the best of our knowledge.Footnote 4
We find that while appropriations are similar across types of networks, differences in punishment choices arise from the network structure, which in turn impact efficiency. More precisely, we found that free riders were punished in all treatments, in line with past studies. However, we also found that the network impacted the type of punishment realized: UCN attracted more severe punishment and more frequent prosocial punishment compared to CN and DCN. Overall this suggests that the effect of making the ‘burden of responsibility’ clearer by reducing the network density or number of potential punishers can depend on whether the network is directed or if pairs have asymmetric monitoring and punishment relations. In addition, imperfect monitoring and punishment networks are more efficient because the larger punishment capacity available in CN elicited more punishment overall. Finally, while individuals underestimated others' appropriation from the commons in all treatments, they were more optimistic in the imperfect networks.
The paper proceeds as follows. Section 2 details the experimental game, design, procedures and hypotheses. Section 3 presents the results, and section 4 discusses their implications.
2. Experimental design and hypotheses
2.1 Procedures and overview
The experiment was held at the London School of Economics and Political Science's Behavioural Research Lab from 20–26 November 2015 and was advertised as a study on economic decision-making. Participation was open to registered students; subjects had no previous experience in CPR or network experiments. The experimental game was executed using z-Tree, and subjects interacted anonymously with each other by taking decisions in private (Fischbacher, Reference Fischbacher2007).
Our design consisted of a between-subjects CPR game with three monitoring and punishment network treatment groups: CN, UCN and DCN. The network treatment and the subject's node type within the network (A, B, C, or D) were held constant throughout each experimental session. An experimental session consisted of 15 decision-rounds, and this was common knowledge. A stranger-matching procedure was used, where each round started with the computer randomly forming four-person network groups by selecting one subject of each type. Thus, while the node type and the network treatment were fixed in each session, the composition of the network group in each round depended solely upon chance and was independent of group formation in any of the other rounds. We conducted seven sessions with either 16 or 20 subjects, for a total of 172 subjects, where each subject participated only in one session. Since group membership was randomized at the beginning of each round, the independent observations are at the session-level (the number of sessions was three for CN, and two for both UCN and DCN). Table 2 summarizes the experimental design and the number of observations in each network treatment.Footnote 5
Table 2. Experimental design
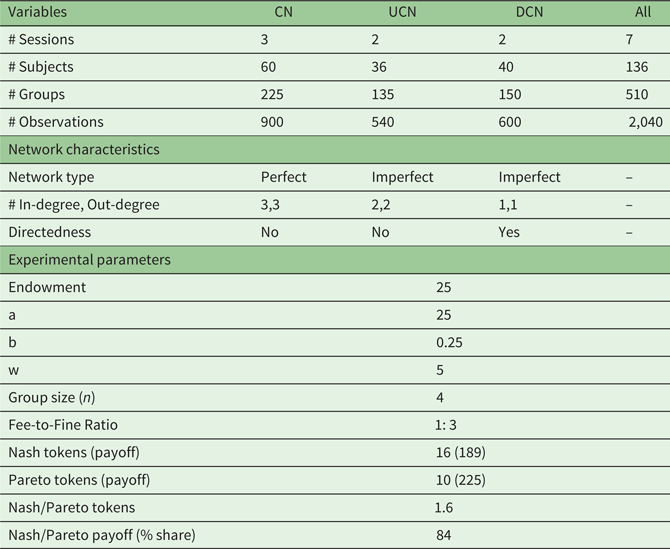
Each round in each session consisted of two stages. In stage one, subjects received an endowment of 25 tokens and decided how to divide it between a common account and a private account. As explained in more detail in the following section, a subject's decision of how much of her endowment to allocate to the common account can be interpreted as a decision about how much to appropriate from the CPR. In stage two, eligible subjects could monitor and assign punishment points to those in their network group. Alongside making an appropriation decision, subjects could also input their beliefs about the expected token placement of the other three group members in the common account in stage one in each round to estimate what their payoffs would be.
The network structure and payoff function, parameter values, punishment rules and number of rounds were common knowledge. Experimental tokens were converted to British pounds (GBP) at the end of the experiment at the rate of 25 experimental tokens = GBP 1, and average earnings were around GBP 7.5.
2.2 The common pool game with networks
Let $e$ be the total amount of tokens available to each subject (i.e., a subject's initial endowment). As previously mentioned, in the first stage subjects decide how many tokens from their initial endowment to allocate between a common account and a private account, where allocation to the private account is assumed to yield a constant marginal return $w$
. We denote by ${x_i} \in [{0,\; e}]$
the amount that individual $i$
allocates to the common account. Consistent with the CPR literature, we interpret ${x_i}$
as the appropriation choice of subject $i$
; the total appropriation of all subjects within the network group is given by $X = \sum\nolimits_i {{x_i}}$
.
The group return from appropriation is $F (X) = aX-b{X^2}$. This nonlinear payoff structure is commonly used in the literature and aims to approximate the complexity arising from socio-ecological dynamics that agents typically face in the field (Ostrom et al., Reference Ostrom, Walker and Gardner1992). The return from appropriation to an individual agent is proportional to the ratio between her appropriation effort $({x_i})$
and the total effort of all appropriators $(X)$
. The ratio ${x_i}/X$
is referred to as the ‘individual distribution factor’ (Apesteguia and Maier-Rigaud, Reference Apesteguia and Maier-Rigaud2006), and captures the negative externality accruing to the group from an individual's appropriation decision: the higher ${x_i}$
is in relation to $X$
, the higher is $i$
's appropriation from the CPR, allowing an appropriator to capture a larger share of returns from the CPR $(aX - \textrm{\;} b{X^2})$
. The first-stage payoff of each agent $i$
is given by:

As per equation (1), subjects made their CPR appropriation decision by dividing tokens between the private and common accounts in stage one, and also stated their expectation about each of the other group members' appropriation decision (instructions and interface snapshots are provided in online appendix A and B respectively). At the end of stage one, each subject was informed only of his/her earnings from the common and private account, and of the total earnings from stage one.
In stage two, subjects simultaneously monitor and make punishment decisions regarding their neighbors, depending on the types of networks they are embedded in, as illustrated in figure 1. A tie between two subjects within a network group indicates that they are connected to each other in a monitoring and punishment relationship, and the arrowhead points to the agent whose appropriation can be monitored and punished. For each subject $i$, ${N_i}$
denotes her monitoring and punishment neighborhood, i.e., the set of subjects $j \ne i$
who can be monitored by $i$
. Subject i can thus observe how many tokens subjects in ${N_i}$
placed into their private and group accounts in stage one, and can furthermore assign deduction points to target individuals in the neighborhood. An undirected tie between any two nodes implies that the pair of subjects can monitor and punish each other as in the CN and UCN (panels 1a and 1b). Conversely, a directed tie denotes an asymmetric relationship between two agents, so that only the appropriator towards whom the arrowhead points can be observed and punished but not vice versa, as in the DCN (panel 1c). Thus, if a tie runs from $i$
to $j$
, then $i$
can monitor $j\; ({j\; \in \; {N_i}})$
but $j$
cannot monitor$\; i\; (i\; \notin \; {N_j})$
. To summarize, in the CN all subjects can monitor and punish one another; in the UCN subjects monitor and punish those to whom they are directly connected (e.g., A can monitor and punish B and D, and A is in turn monitored by B and D, but not C); and asymmetric pairwise relationships exist in the DCN because A can only monitor and punish B, B can only monitor and punish C, and so on. In terms of network properties, the only difference between CN and UCN is that the latter has a lower network density (66 per cent for UCN versus 100 per cent for CN) and is incomplete; while the only difference between UCN and DCN is that the latter has directed rather than undirected ties.
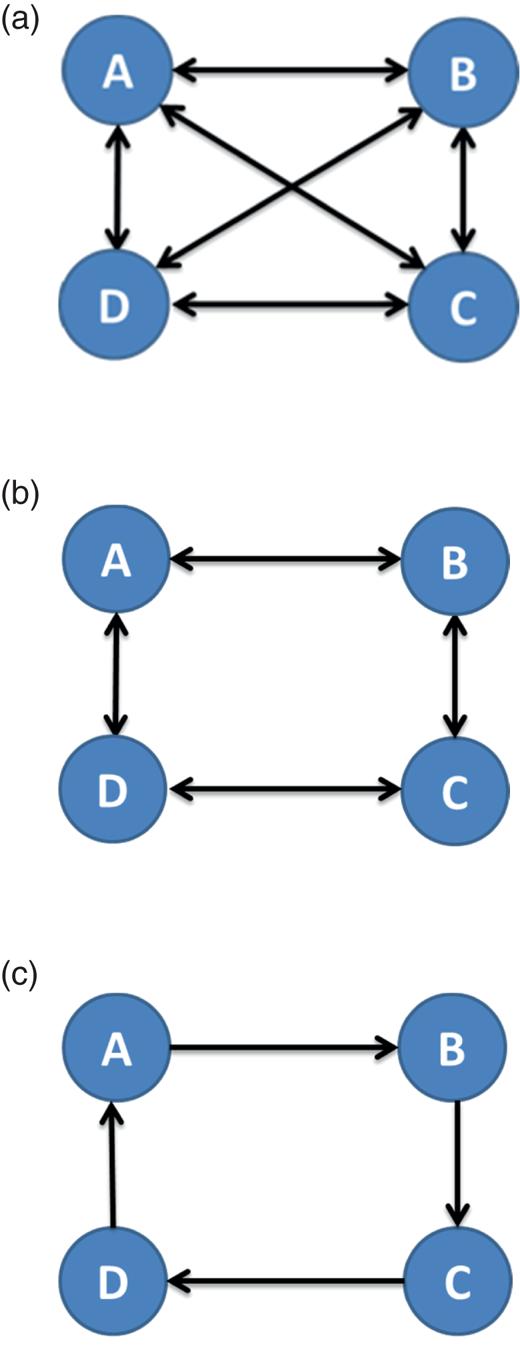
Figure 1. Network treatment groups: (a) Complete Network [0] (CN), (b) Undirected Circle Network [1] (UCN), (c) Directed Circle Network [2] (DCN).
Punishing others entails a personal cost which depends on the number of deduction points assigned. Each agent $i$ can punish $j$
in her monitoring and punishment neighborhood $(j \in \; {N_i})$
to reduce $j$
's payoff from the first stage by $p_j^i$
at a personal unit cost of ${c_a} = 1$
per deduction point assigned. Each punishment point received reduces $j$
's payoff by ${c_r}$
= 3. Following recent CPR experiments (table 1), the fee-to-fine ratio – which represents the cost to the punisher from levying one deduction point and the cost to the target – is 1:3, and it is held constant across all network treatments. The maximum amount of punishment points that could be assigned was bounded by the player's earnings in the first stage, thus allowing for a system of graduated sanctions for appropriators determined by the subjective choice of the agent assigning punishment (Fehr and Gächter, Reference Fehr and Gächter2000). Before proceeding to the next stage, each individual could calculate the total cost of deduction points assigned to others. Equation (2) presents the earnings to each agent from stage two, which could range between 0 and the net earnings from stage one after the cost of assigning and receiving deduction points:

At the end of stage two, each subject was informed only of his/her total earnings from stage one, the total cost of punishment points given to and received by others, and net earnings from stage two.
Let us consider the standard prediction for this CPR game. If we assume that subjects are rational self-interested agents who choose to maximize their earnings in each round and apply the logic of backward induction, each individual will not issue punishment points in stage two since it is costly to do so ($p_i^j = 0$, for all $i$
and $j \in \; {N_i}$
). Consequently, subjects will recognize that nobody will punish in this second stage and choose to appropriate at the socially-inefficient level in stage one in all types of network groups. Thus the standard prediction is that the availability of peer monitoring and punishment in stage two cannot deter free riding in stage one.
Unlike standard treatment comparisons that change only one characteristic at a time, the comparisons of this study necessitate changes in both information and punishment to reflect the underlying change in the type of network architectures studied here. However, as noted in table 1, all institutional features were kept fixed across all treatments, including the use of n = 4 individuals per network group, 15 periods of play, and a strategy space of {0, 1, …, 24, 25} tokens to be allocated to the common and/or the private account. Parameter values were chosen to ensure a good spread in values between the Nash and Pareto equilibrium allocations – 16 and 10 tokens which will yield a payoff of 189 and 225 tokens respectively – while keeping the ratio of Nash to Pareto equilibrium strategies (which determines the size of the negative externality from appropriation) comparable to recent CPR experiments in the literature (table C1 in the online appendix compares parameters to past studies).
The experimental data may show that repeated interactions can influence play across time, which can arise from subjects learning about the rules of the game, or about the preferences or ‘types' of subjects in each session, as well as from strategic play during the early rounds in the game. Since the analysis is for one-shot games, we used a stranger-matching design to balance the need for repeated experience by subjects while attempting to reduce reputation effects (e.g., as in Kreps et al., Reference Kreps, Milgrom, Roberts and Wilson1982). We followed Andreoni and Croson's (Reference Andreoni, Croson, Plott and Smitt2008) suggestion that it is more prudent to use a stranger-matching design when studying single-shot games.Footnote 6 While perfect stranger-matching could mitigate investments in reputations, only five rounds could be played with this protocol due to constraints like lab capacity, and this is arguably too short a period to allow for learning of the rules of the game. Moreover, past studies have not clearly established large differences in outcomes between stranger and perfect-stranger-matching (Fehr and Gächter, Reference Fehr and Gächter2000, Reference Fehr and Gächter2002). We tried to address these concerns, however, by first anonymizing an individual's identity while displaying their decisions in stage two (barring their node type) on the computer screen. Secondly, the probability of being in the same group in the following period was small (about 0.25 (0.5) per cent for 20 (16) subjects per session), also helping to mitigate strategic play in earlier periods of the session.
Finally, we paid participants for one randomly selected round of the 15 rounds played at the end of the game to mitigate strategic play in the early rounds and potential wealth effects. The round was selected at random by drawing one of 15 numbered ping pong balls at the end of the experiment. After this, subjects were paid their earnings from the CPR game, and the potential earnings from their stated expectation about the appropriation of others in the group for that round (if it matched the actual choices that the others made).Footnote 7 Since it was possible to receive a negative payoff in a given period, we instituted a rule that if players received negative payoffs in the round selected for payment, they would obtain no payment (we did not invoke this rule in any instance of payment).
Much care was taken to ensure that subjects understood the rules of the experiment and the incentive structure. Printed instruction booklets, with detailed explanations of the network structure and the CPR game, were placed at the computer terminals. The framing of instructions was maintained consistent across all treatments, similar to that in the literature in trying to avoid value-laden language. For example, the common pool appropriation decision was framed in terms of allocating tokens to a common account. The punishment decision was framed as the assignment of deduction points to the other people in the network group.Footnote 8 Apart from numerical examples to illustrate payoffs within the instruction text, an earnings table (see online appendix A) was also displayed showing potential payoffs in tokens corresponding to different token placements in the common account by the subject and total tokens placed by the three others in the group to aid comprehension, similar to the earnings table used in Apesteguia and Maier-Rigaud (Reference Apesteguia and Maier-Rigaud2006). To further familiarize subjects with the incentive structure and rules, subjects first read the instructions by themselves at the start of the session, and the instructions were then read aloud by the experimenter. After this, subjects answered trial questions at the end of the instruction booklet, which was checked by the experimenter before proceeding with the experiment.Footnote 9 Experimental sessions typically lasted for an hour and 15 min, with 15 min of instructional time.
2.3 Hypotheses
Building upon insights from the existing literature, we formulate hypotheses about whether and how appropriation and punishment outcomes vary across network structures. Specifically, our first hypothesis is
Hypothesis 1 There is no difference in appropriation across CN, UCN and DCN.
This hypothesis builds upon results from the role of imperfect monitoring and punishment networks in the PG game context, which suggest that average contributions tend to be similar across networks where all players are connected to one another, as is the case with the CN, UCN and DCN (Carpenter et al., Reference Carpenter, Kariv and Schotter2012; Boosey and Isaac, Reference Boosey and Isaac2016).
To better understand how the network structure impacts punishment, we consider the ‘punishment amount', which refers to the total number of punishment points received by an individual in stage two of the CPR game in a given round. In addition, we estimate the ‘punishment severity’ by weighting the average punishment received by the number of potential punishers targeting each subject (denoted by the in-degree). That is, we assign weights of 3, 2 and 1 in the CN, UCN and DCN, respectively. Lastly, we also consider the ‘punishment incidence’, which is whether the subject received any punishment in a given round. In denser networks, like the CN, each individual faces a higher number of potential punishers than in less dense networks; this, in turn, may result in a higher punishment amount being received by each individual, as well as a higher incidence of punishment. On the other hand, less dense networks like UCN, which features fewer punishment opportunities, may increase the severity of punishment allocated per opportunity. How this potential increase in punishment severity affects the amount and the willingness to punish is an open empirical question. Turning to the available experimental evidence, Carpenter et al. (Reference Carpenter, Kariv and Schotter2012) found that punishment amounts are similar between CN and UCN networks, but that the punishment amount used in the DCN is higher, possibly because the attribute of directedness specifies clearer monitoring and punishment responsibilities for each subject. Thus, we hypothesize that:
Hypothesis 2a The amount of punishment and incidence elicited in UCN is at least as large as in CN, but punishment severity is higher in UCN.
Hypothesis 2b The punishment amount, severity and incidence elicited by DCN is at least as high as in CN and UCN.
Understanding individual-level punishment behavior allows us to examine the types of punishment given in relation to the appropriation behavior of the target agent. Past studies have highlighted three broad non-standard motivations for punishing peers, namely: (i) prosocial inclinations to punish free riders, (ii) antisocial punishment to penalize cooperators, and (iii) punishment due to errors (Ostrom et al., Reference Ostrom, Walker and Gardner1992; Herrmann et al., Reference Herrmann, Thöni and Gächter2008; Casari and Luini, Reference Casari and Luini2012). When considering prosocial punishment, past studies indicate that punishing free riding is generally triggered by negative emotions that arise from a violation of either reciprocity or fairness norms, as well as from feeling exploited (being the ‘sucker’) (Ostrom et al., Reference Ostrom, Gardner and Walker1994; Fehr and Schmidt, Reference Fehr and Schmidt1999; Frey and Meier, Reference Frey and Meier2004; Fehr et al., Reference Fehr, Fischbacher and Kosfeld2005). Ostrom et al. (Reference Ostrom, Walker and Gardner1992) further differentiated between one-round prosocial punishment received because the person fined was the highest (or one of the highest) appropriator in the current round, and lagged punishment where the punished person was one of the highest appropriators in the prior round. Regarding antisocial punishment, they noted the occurrence of blind revenge, which is targeted at low appropriators by those who received punishment in a previous round. Other studies also remark on the possibility of targeted revenge aimed at an identifiable punisher when counter-punishment opportunities are available, as well as spiteful or expressive punishment by those who punish because they have a ‘hard-wired’ taste for it (Nikiforakis, Reference Nikiforakis2008; Jensen, Reference Jensen2010; Casari and Luini, Reference Casari and Luini2012). While our experiment was not designed to differentiate between these motivations, it can nonetheless shed some light on this issue.
To measure whether different network treatments affect prosocial punishment and antisocial punishment, we define them as follows. ‘Prosocial punishment’ is the punishment given to a target agent who allocated more tokens to the common account than the theoretical Pareto optimal benchmark of 10 tokens. ‘Antisocial punishment’, on the other hand, is measured as the punishment given to a target agent who appropriates at or below this Pareto optimal level. Building on Ostrom et al. (Reference Ostrom, Walker and Gardner1992), antisocial punishment is likely to stem from three sources: blind revenge by someone who was a low CPR appropriator in the previous round; free rider revenge if the punisher was a free rider who appropriated above the Pareto optimal level and was punished in the last round; or other types of antisocial punishment arising from error or a hard-wired taste for punishment. However, unlike in Ostrom et al. (Reference Ostrom, Walker and Gardner1992), we can eliminate the possibility of lagged prosocial punishment (targeting an agent who over-appropriated in the previous round) and targeted antisocial counter-punishment (as a response to being punished by the target in the previous round), since we use a stranger-matching design without a counter-punishment stage where individual players cannot be identified in the subsequent round as their identities are anonymized. Assuming that those types with a hard-wired and vengeful predisposition to punish were evenly distributed across network treatments, ex-ante, we reason as follows.
The past experimental literature on the bystander effect shows that a reduction in the number of possible ‘helpers’ increases prosocial behavior (Cryder and Loewenstein, Reference Cryder and Loewenstein2012). This implies that the burden of responsibility is more diffused in the CN due to a larger number of potential punishers who can act prosocially by sanctioning free riders in stage two. The UCN, thus, will offer less ambiguity about an individual's responsibility to punish free riders since they are less densely connected and, arguably, the DCN can offer even more clarity because it is directed. Free riders may be more likely to be punished – especially in the DCN – as it offers unambiguous responsibility to monitor and punish a single agent. Indeed, Carpenter et al. (Reference Carpenter, Kariv and Schotter2012) found that free-riders – defined as those who contribute nothing to the public good – are more likely to be punished in DCN relative to the UCN (but that the difference was not statistically significant).
By this logic, it is also possible that the clearer delineation of responsibility afforded by UCN and DCN in this CPR dilemma may also attenuate the extent of antisocial punishment. We remain agnostic about whether the pattern of types of antisocial punishment – namely blind revenge, free rider revenge and residual cases such as punishment by error – differs across network groups.
Hypothesis 3 There is more prosocial punishment and less antisocial punishment in UCN and DCN than in CN.
3. Results
We report the results in four subsections corresponding to each outcome, i.e., appropriation, punishment, efficiency (as measured by net payoffs) and beliefs. In each, we consider if outcomes vary across networks by using random-effects panel regression models with robust standard errors, instead of standard statistical tests, due to our experimental design: network treatments assigned at the session level, stranger-matching for each of the 15 rounds to form network groups of four and a payout from one randomly selected round. This model allows us to control for potential session-fixed effects using session dummies and learning using round dummies. We also include individual-level controls for gender, experience in lab sessions, and whether student's main discipline of study was economics.Footnote 10Table 3 reports the summary statistics for the key outcomes by each network treatment for each session pooled across all observations and rounds.
Table 3. Descriptive statistics by network
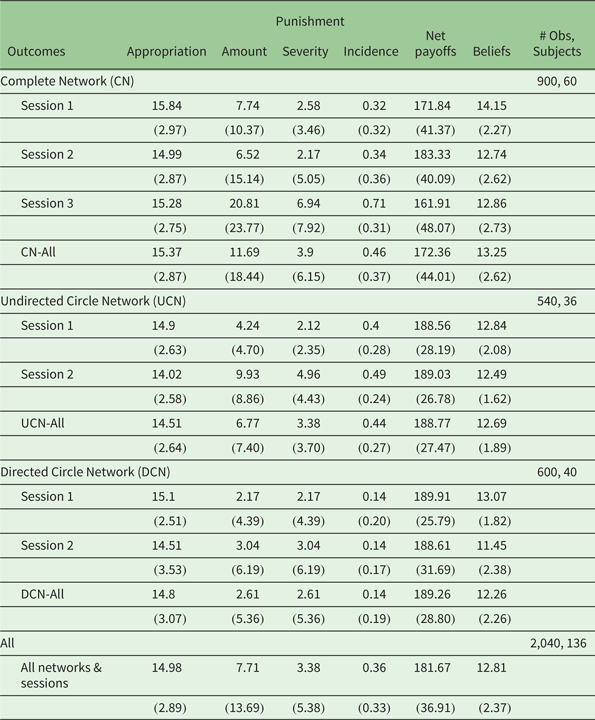
Notes: Appropriation is the average number of tokens allocated to the common account by an individual in each session. Punishment amount is the average of the total punishment received by an individual from other group members in stage two of the CPR game. Punishment severity is this punishment amount received divided by the number of potential punishers targeting each subject, which is determined by the in-degree in each network, i.e., by 3, 2 and 1 in the CN, UCN and DCN respectively. Punishment incidence is whether the subject received any punishment in a given round. Net payoffs are the returns to the subject after the cost of giving and receiving punishment have been deduced from their stage one earnings. Beliefs are the average of the number of tokens that subjects expect other group members to place in the common account in a given round.
3.1 Appropriation
When we look at appropriation levels in the CN (in table 2), the average amount of tokens allocated to the common account pooled across all rounds was 61.5 per cent of the endowment or 15.37 tokens. This figure is close to recent findings from previous studies with perfect monitoring and punishment: for example, 59 per cent and 66.4 per cent respectively in Kingsley (Reference Kingsley2015) and 56.6 per cent and 62 per cent respectively in Cason and Gangadharan (Reference Cason and Gangadharan2015). It is also comparable to (albeit marginally lower than) recent CPR experiments without any punishment, e.g., 74.8 per cent in Apesteguia and Maier-Rigaud (Reference Apesteguia and Maier-Rigaud2006) and 67 per cent in Kingsley and Liu (Reference Kingsley and Liu2014). Turning to the UCN and DCN, we found that appropriation was similar to CN at a little under 15 tokens.
Figure 2 illustrates that the average appropriation for each round begins close to the mid-point of the choice interval in all treatments near the Pareto optimal level of 10 tokens and trends towards the Nash equilibrium across all networks. In line with Ostrom et al. (Reference Ostrom, Walker and Gardner1992) and other recent works, appropriations follow a mild pulsing pattern since allocations to the common account increased and then decreased, with a tendency for the variance to decrease over time. The increase in average appropriation in rounds 8–15 compared to rounds 1–7 is statistically significant only in the imperfect UCN and DCN networks (at 5 per cent and 1 per cent respectively in a random-effects regression with session dummies; results in online appendix table C4).
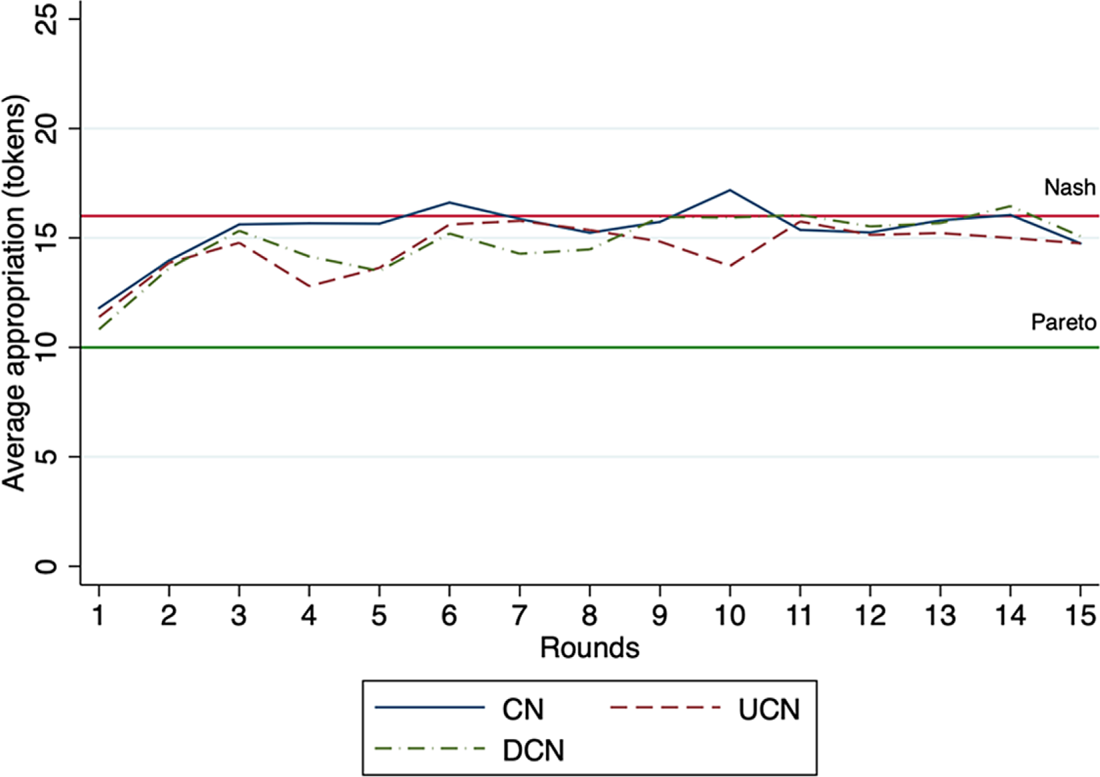
Figure 2. Appropriation and beliefs about others' appropriation.
Table 3 reports the results from the random-effects regression models (with session and round fixed effects and controls). Individual-level appropriation is regressed on network treatment dummies (with CN as the omitted category) in model (1), and while the coefficients UCN and DCN are positive, they are not statistically significant. This result confirms that appropriation from the CPR is similar across the perfect (CN) and imperfect (UCN and DCN) networks. In addition, the differences between UCN and DCN are also not statistically significant (Wald test p-value = 0.524 in model 1). Thus, we cannot reject hypothesis 1.Footnote 11
Result 1 On average, appropriation is not different across CN, UCN and DCN.
3.2 Punishment
Table 2 shows that the average punishment amount received was the highest in CN (11.7 points), compared to UCN and DCN (6.7 and 2.6 points respectively). This suggests that the higher punishment capacity due to the greater number of opportunities offered by denser networks elicited higher levels of punishment points received on average. When we turn to punishment severity to control for the different punishment capacity offered by each network, the figure shows that CN and UCN seem to elicit marginally higher punishment severity at 3.9 and 3.4 respectively, compared to the DCN, which elicited 2.6. From figure 3a, the average share of individuals receiving any punishment in a given round is again similar in the CN and UCN at 46 per cent and 44 per cent respectively, and lower in the DCN at 14 per cent. Figures 3b and c display the average punishment amount received and severity by subjects across the three network groups in each round. We see that punishment patterns are erratic in the CN, consistent with previous results from Cason and Gangadharan (Reference Cason and Gangadharan2015). Average punishment amount and severity also show uneven patterns in both imperfect networks. The differences in average punishment amount and in severity between rounds 8–15 and rounds 1–7 are not statistically significant in any of the networks, but the likelihood of an individual receiving punishment is marginally lower in all networks in this second period (online appendix table C5).
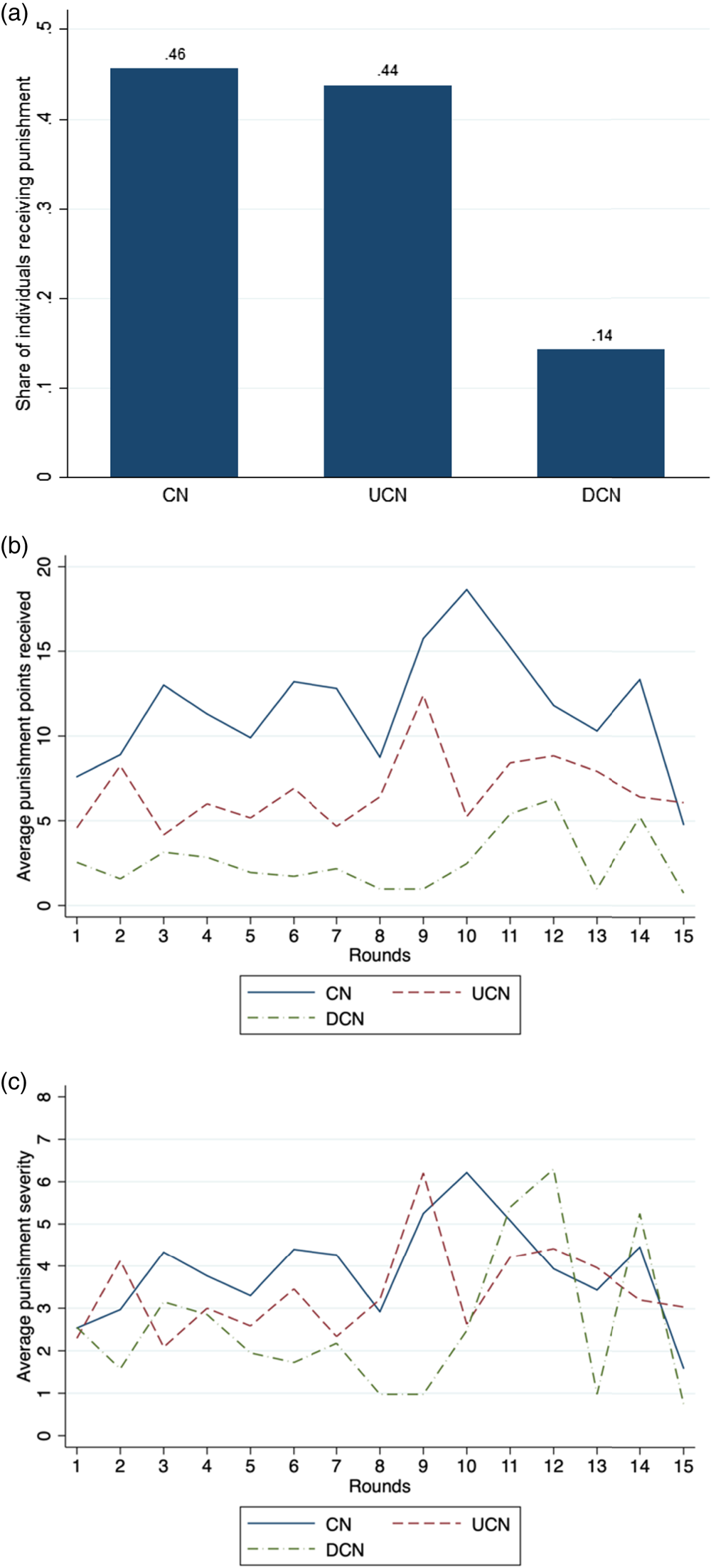
Figure 3. Punishment received.
Table 4 checks whether the average amount of punishment points received by individuals, and their severity and incidence, differs across networks. The results show that CN elicits higher punishment amounts than DCN (the difference is significant at 1 per cent) but that the difference with the UCN is not statistically significant. On the other hand, the UCN elicited more severe punishment than the CN (the difference is significant at 5 per cent) and that punishment severity is not different between CN and DCN. The likelihood of receiving punishment is also higher in the UCN relative to the CN (the odds are around 2.3 times higher with the difference significant at 1 per cent) and lower in the DCN (probability is 0.3 times lower, with the difference significant at 1 per cent). Differences between UCN and DCN coefficients show that punishment amount and incidence is higher in UCN (Wald test p-value < 0.01 from models 2 and 4), but that the difference in punishment severity is not statistically significant (Wald test p-value = 0.12 in model 3).
Table 4. Average outcomes across networks
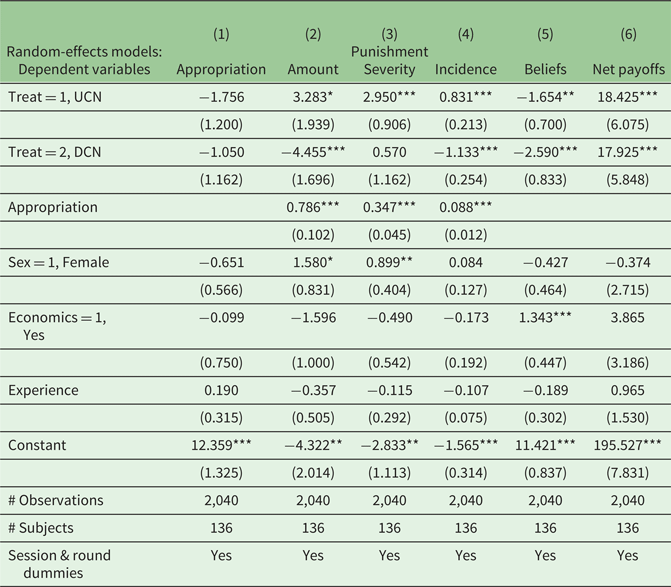
Notes: Robust standard errors in parentheses; ***p < 0.01, **p < 0.05, *p < 0.1. Omitted category = CN. Random-effects logistic regression models are used for punishment incidence since the outcome is binary (Punishment incidence = 1 if yes, 0 if no). The UCN and DCN coefficients imply that the odds of being punished are 2.3 (higher than CN) and 0.3 (lower than CN) respectively.
When we restricted attention to only those who appropriated over the Pareto optimal level of 10 tokens, we found that the results were qualitatively similar, the main difference being that punishment amount, severity and incidence were all higher (online appendix table C6). These results can be summarized as follows:
Result 2a The punishment amount elicited by UCN is similar to that in CN, but punishment severity and incidence is higher in UCN than in CN.
Result 2b The punishment amount and incidence elicited by DCN is lower than in both CN and UCN, but punishment severity is similar to both CN and UCN.
The above results imply that we cannot reject hypothesis 2a but cannot fully support hypothesis 2b.
Next, we proceed to analyze individual-level punishment behavior. We first examine if the punishment amount received was influenced by whether the target of the punishment was a free rider, i.e., an agent who allocated a token amount greater than the Pareto optimal level of 10 tokens to the common account. We estimated the difference between the appropriation of a subject in a given round and the Pareto equilibrium allocation of 10 tokens, such that a positive deviation denotes appropriation above the socially-optimal level or free riding, and zero and negative values denote allocations at or below the socially-optimal level. From figure 4, it is clear that individuals engage in prosocial punishment in all networks since those who appropriated more than the socially-optimal appropriation level are punished more severely than lower appropriators. In addition to confirming this established finding from the literature (Ostrom et al., Reference Ostrom, Walker and Gardner1992; Fehr and Gächter, Reference Fehr and Gächter2000; Cason and Gangadharan, Reference Cason and Gangadharan2015), we also see some antisocial punishment, i.e., punishment targeting those who extract at or less than the Pareto equilibrium, albeit at lower levels (Herrmann et al., Reference Herrmann, Thöni and Gächter2008).
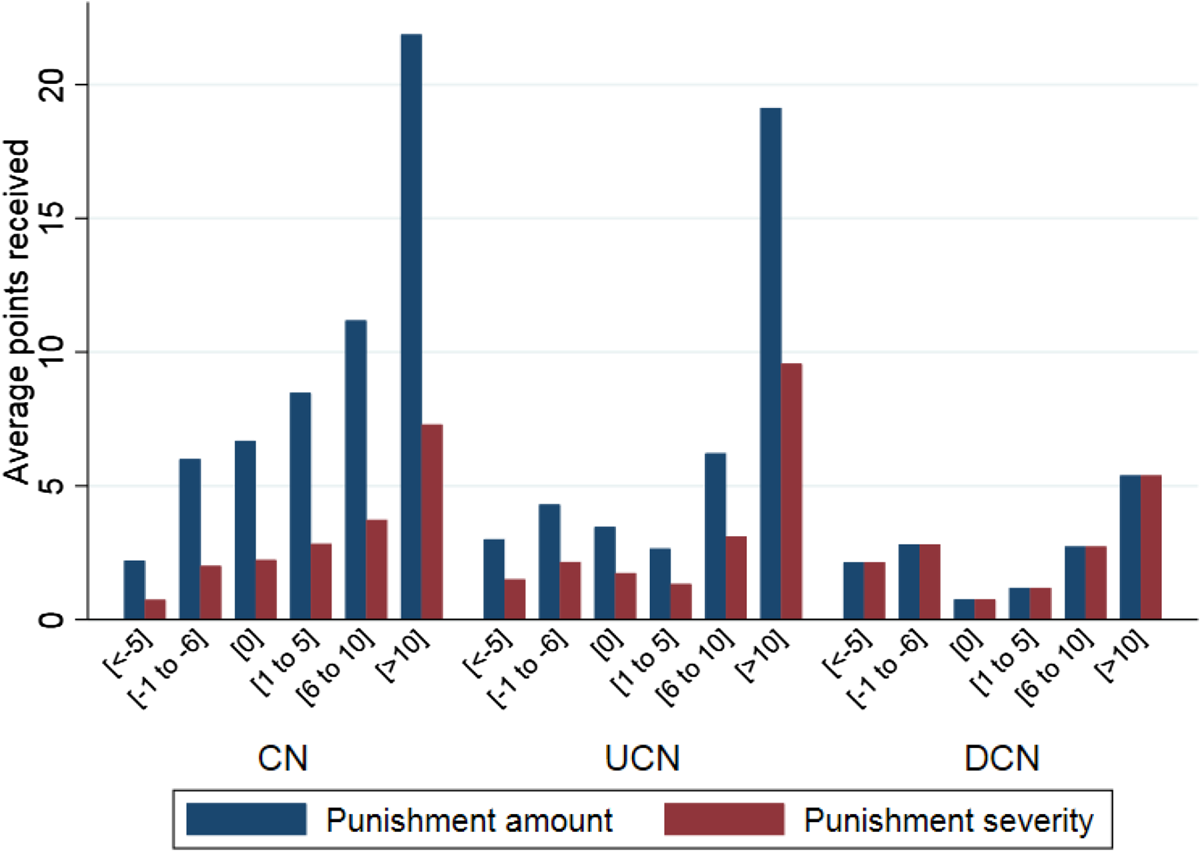
Figure 4. Punishment by deviation from Pareto optimal appropriation.
In order to better understand individual-level punishment behavior, we examined individual punishment flows between pairs of players to factor in whether the target of punishment was a free rider and the motivations of the punisher. We mapped the frequency of different types of pairwise punishment flows, i.e., the number of times a type of punishment was given between two subjects. Building on Ostrom et al. (Reference Ostrom, Walker and Gardner1992),Footnote 12 we first categorized pairwise punishment flows according to no punishment (standard prediction) and prosocial punishment (aimed at those who appropriated above the Pareto optimal level). The remaining instances of punishment were categorized as antisocial punishment since they are aimed at those appropriating at or below the Pareto optimal level. Of these antisocial punishment pairwise flows, we further subdivided punishment flows into three categories: ‘blind revenge’ if the person giving punishment was a low CPR investor who was fined by a person fined in a previous round; ‘free rider revenge’ if the person giving punishment was a free rider who appropriated above the Pareto equilibrium level round in the last round and was punished; and lastly ‘other’ types of antisocial punishment that do not fit these two categories and offer no obvious explanation as they may stem from either error or a hard-wired taste for punishment or some combination of both. To account for the differences in monitoring and punishment capacity across networks, we considered the shares of each type of punishment given on the basis of the opportunities to give punishment in each network (i.e., three, two and one in CN, UCN and DCN respectively).
When we look to the descriptive statistics presented in figure 5, it is clear that those in the DCN and CN chose to levy no punishment 85.8 per cent and 80 per cent of times respectively compared to those in the UCN at 72.8 per cent. This is consistent with the previous result that the average punishment incidence was higher in UCN and lower in DCN relative to CN, which was based on the aggregated outcomes in table 2. Interestingly, it appeared that prosocial punishment was used more often in the UCN – it constituted 22.7 per cent of all pairwise punishment flows compared to 16.8 per cent and 11 per cent of the time in CN and DCN respectively. There is also a marginally higher share of free rider revenge in this treatment – 2.6 per cent versus 1.9 per cent and 1.5 per cent in CN and DCN respectively – possibly reflecting the likelihood that free riders were more likely to be punished more frequently in UCN. Parallel to this, given the higher incidence of punishment overall, blind revenge is also a bit higher in UCN at 0.93 per cent compared to 0.41 per cent in CN and 0.33 per cent in DCN. Overall, assuming that the total frequency of antisocial punishment is the sum of how often blind revenge, free rider revenge and other types of punishment were levied between pairs, UCN seems to elicit a marginally higher incidence of antisocial punishment (at around 4.9 per cent, mostly driven by higher free rider revenge) relative to CN and DCN (both at around 3.2 per cent).
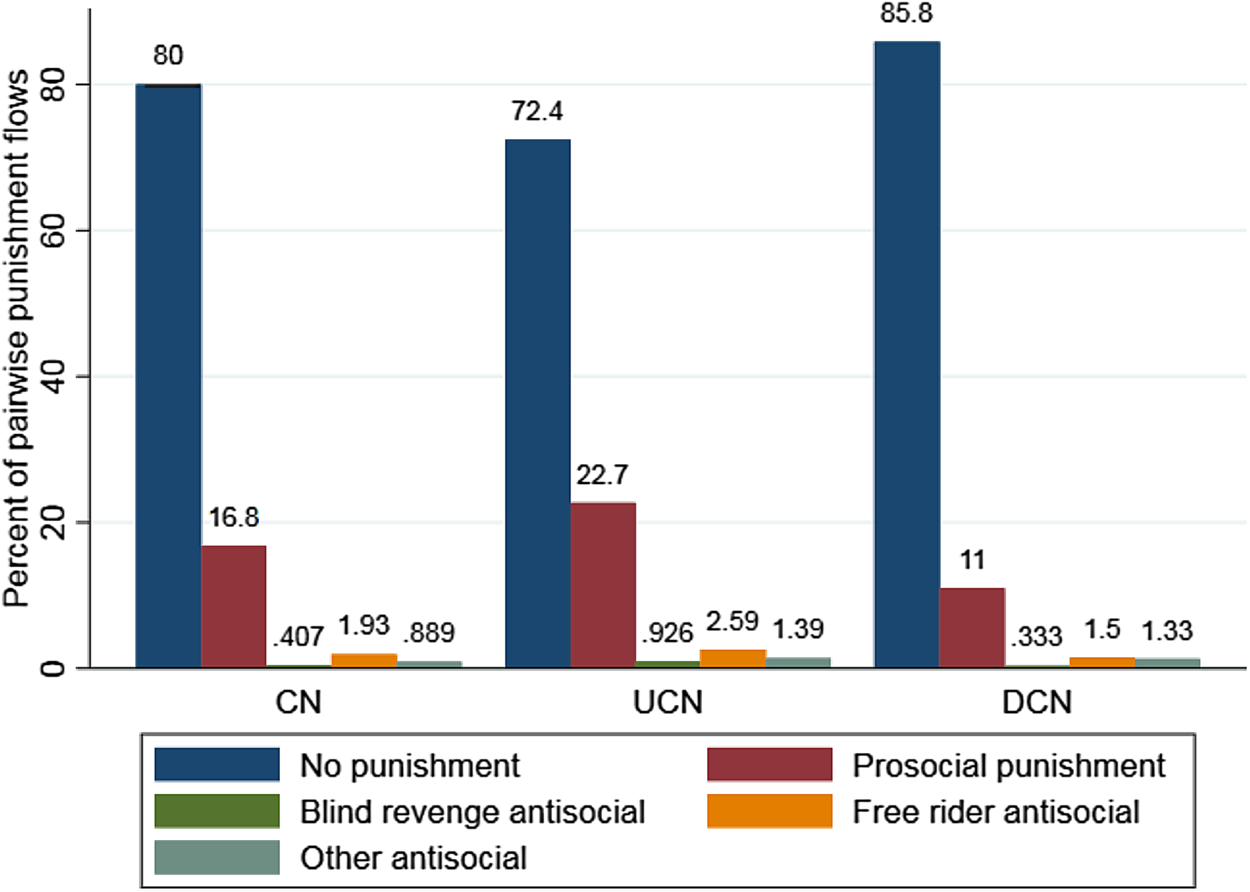
Figure 5. Types of pairwise punishment flows.
To assess whether prosocial and antisocial punishment flows are statistically different across networks, we regressed the share of the instances of each type of pairwise punishment on the network dummies in random-effects regressions. The omitted category was CN and we controlled for stage one earnings (since the maximum amount of punishment that could be assigned was bound by stage one earnings), subject attributes, and added round and session dummies. Comparing UCN to CN gives us the effect of lower network density on punishment, and comparing UCN and DCN gives us the effect of directedness or asymmetric monitoring relationships between pairs of agents. The results presented in table 5 show that there is more prosocial punishment in UCN relative to CN (statistically significant at 1 per cent in model 1). The coefficients on the imperfect networks are also different from each other (Wald test p-value < 0.01 in model 1), confirming that UCN elicits more frequent prosocial punishment than DCN. On the other hand, as seen in model 2, the levels of antisocial punishment are not statistically different between CN and UCN. Differences in antisocial punishment between UCN and DCN are also not statistically significant (Wald test p-value = 0.91 in model 2).Footnote 13 Overall, this suggests that lower network density increases prosocial punishment in undirected networks but not necessarily in directed networks.
Result 3 Prosocial punishment was used more frequently in UCN than in CN and DCN. There are no differences in the frequency of antisocial punishment across networks.
Table 5. Types of punishment given across networks
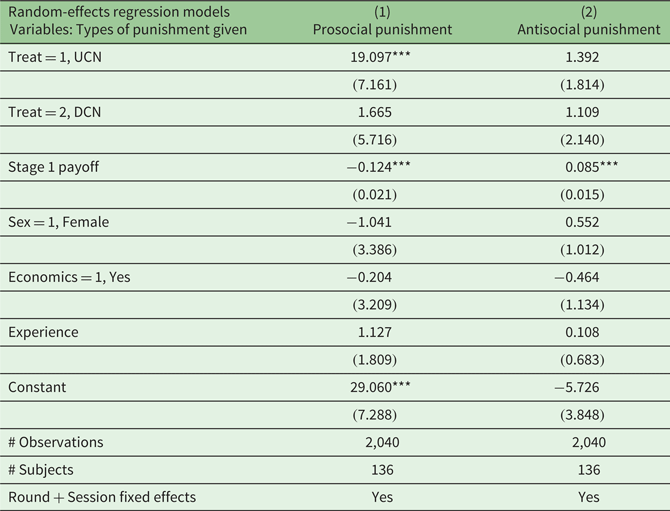
Notes: Robust standard errors in parentheses; ***p < 0.01. Omitted category = CN.
The above results lend partial support for hypothesis 3.
3.3 Efficiency
The average payoffs accruing to individuals pooled across all 15 rounds are lower than the socially-optimal level in all networks, but are marginally higher in UCN and DCN compared to CN. Table 3 also formally supports this result, as the coefficients of DCN and UCN are both positive and statistically significant at 1 per cent, but the coefficients on the imperfect networks are not different from each other (Wald test p-value = 0.918 in model 5). Figure 6 illustrates that payoffs start above the Nash equilibrium level, but trend downwards in all networks. The erratic punishment patterns in all treatments – but especially in the CN – are the major reason for the variation in efficiency, a result similar to Cason and Gangadharan (Reference Cason and Gangadharan2015). Average payoffs decline in all treatments and the decline is larger in the imperfect networks (online appendix table C7).
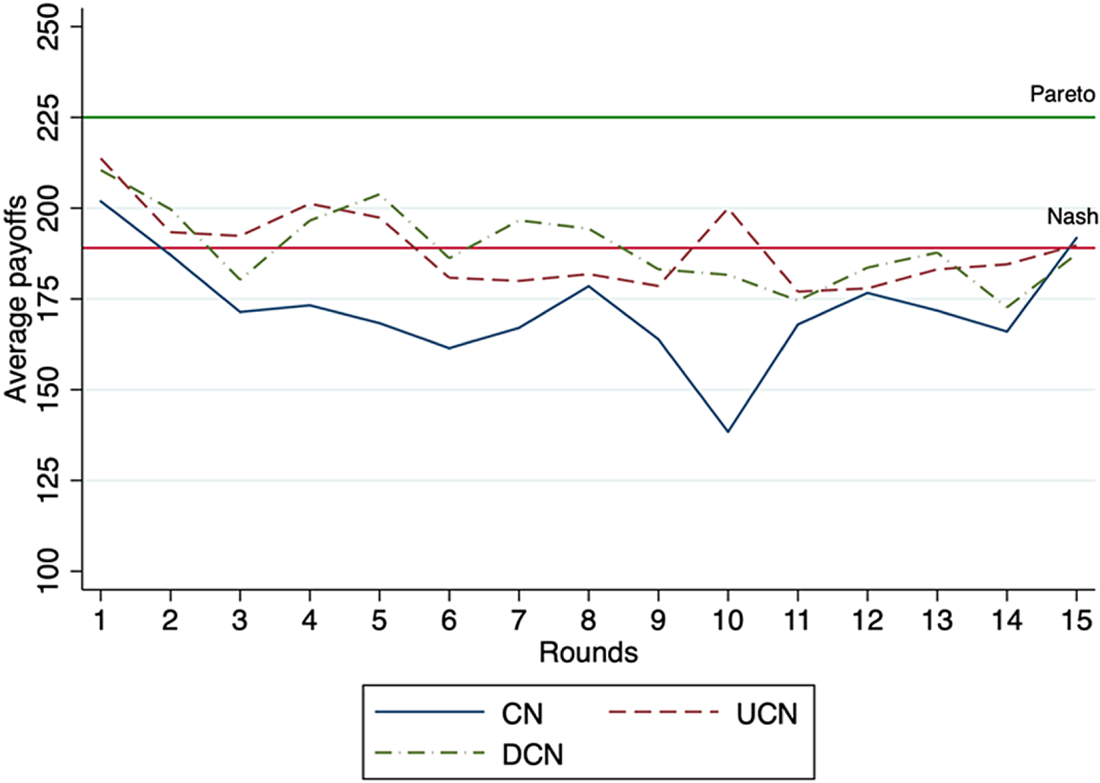
Figure 6. Average payoffs.
3.4 Beliefs about the appropriation of others in the group
The average beliefs are higher in CN compared to UCN and DCN. Table 3 provides formal support for this as stated beliefs are around 2–3 tokens lower in all imperfect networks relative to the CN (significant at 1 per cent), but the UCN and DCN coefficients are not different from each other (Wald test p-value = 0.247 in model 6). Notably, economics students state higher beliefs which were closer to appropriation levels (by a magnitude of 1–0.66, significant at 1 per cent) relative to non-economics students in table 3. This is in line with findings that economics training leads people to expect others to defect more in social dilemmas (Frank et al., Reference Frank, Gilovich and Regan1993). Figure 7 shows that stated beliefs over others' appropriation start at the Pareto appropriation and trend towards the Nash equilibrium in all networks. Expectations that others appropriate less than the Nash equilibrium tend to decline in all treatments (online appendix table C8). Overall, this suggests that individuals retain more optimistic beliefs in imperfect networks.
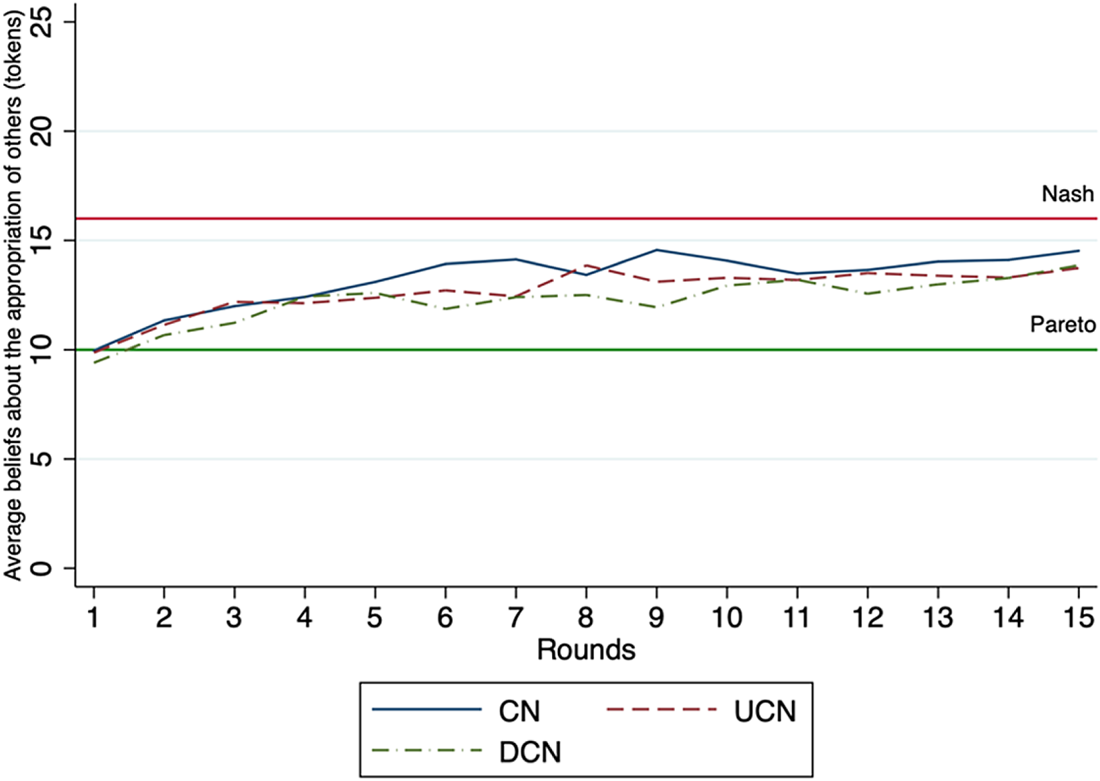
Figure 7. Average beliefs about the appropriation of others.
4. Discussion
This paper has explored the effectiveness of imperfect peer monitoring and punishment networks in promoting cooperation in an experimental CPR dilemma. We considered three types of networks – CN, UCN and DCN – which systematically reduced the number of potential punishers in a group by varying the structural attributes of network density and directedness. By doing so, it changed the perceived ‘burden of responsibility’ to personally undertake costly peer monitoring and punishment aimed at mitigating free riding, an important feature according to studies that suggest that the availability of other agents who can engage in peer sanctioning reduces one's tendency to act prosocially (by shouldering the costly punishment). By focusing on a nonlinear CPR appropriation dilemma – a neglected class of social dilemma games that are said to be quite relevant to understanding more complex socio-ecological dynamics in the field – our experiment extended the emerging literature that studies whether imperfect monitoring and punishment networks affect cooperation levels relative to perfect institutions, which has so far focused on linear public good games. In this way, our paper confirms some key conclusions drawn from these different strands of literature, but also contributes to the external validity of prior experimental results in a new context with different design choices. For example, average appropriation levels in our experiment were comparable to previous nonlinear CPR experiments with perfect peer monitoring and punishment (Cason and Gangadharan, Reference Cason and Gangadharan2015; Kingsley, Reference Kingsley2015) and lower than those without sanctioning institutions (Apesteguia and Maier-Rigaud, Reference Apesteguia and Maier-Rigaud2006; Kingsley and Liu, Reference Kingsley and Liu2014; Cason and Gangadharan, Reference Cason and Gangadharan2015; Kingsley, Reference Kingsley2015).
We found that relative to the complete network, the imperfect UCN and DCN networks do not affect appropriation in systematically different ways. This confirms recent findings from comparable public goods games employing these network structures that contributions are similar across these three networks (Carpenter et al., Reference Carpenter, Kariv and Schotter2012; Boosey and Isaac, Reference Boosey and Isaac2016). It also falls in line with findings from De Geest et al. (Reference De Geest, Stranlund and Spraggon2017) that there is not much systematic variation in the average appropriation of group members across perfect and imperfect monitoring and punishment treatments (keeping in mind that their experiment varies the ability of insiders to monitor and punish outsiders). Before discussing why this may be the case, we first turn to our results on how imperfect monitoring and punishment network impact punishment choices.
Our result that there is a greater amount of punishment points received in networks with higher punishment capacity (e.g., the CN and UCN) corresponds to findings in Leibbrandt et al. (Reference Leibbrandt, Ramalingam, Sääksvuori and Walker2015) that sanctioning is higher when more structural opportunities to sanction are available. We also found that free riders are less likely to be sanctioned in UCN (which also sees the lowest frequency of any type of punishment) and the prosocial punishment flows are highest in the UCN. This result suggests that lowering the number of potential punishers (or ‘helpers’) increases prosocial behavior and is in line with the studies on the bystander effect (Cryder and Loewenstein, Reference Cryder and Loewenstein2012). However, when monitoring and sanctioning roles are made even clearer – as in the DCN – we do not see even greater prosocial punishment relative to the DCN. These results differ from those predicted by the bystander effect studies and Carpenter et al. (Reference Carpenter, Kariv and Schotter2012), who find that punishment levels are amongst the lowest in the CN and that more prosocial punishment is used in DCN (to maintain or raise contributions), compared to CN and UCN. A possible reason is that people may prefer coordinated punishment and may thus be willing to punish someone only when another individual is willing to do so as well (Ramalingam et al., Reference Ramalingam, Godoy, Morales and Walker2016; Molleman et al., Reference Molleman, Kölle, Starmer and Gächter2019). Another related reason may be that individuals may act as ‘rational bystanders’ if they believe that helping is only effective when more than one help‐giver pitches in (Greitemeyer and Mügge, Reference Greitemeyer and Mügge2013) or that their personal contribution will be superfluous (Krueger and Massey, Reference Krueger and Massey2009); this is not unlikely in more complex environments like the nonlinear CPR appropriation dilemma. To unpack this further, a replication using a larger number of treatments that vary the potential number of punishers across both directed and undirected networks of varying sizes and types would be most useful.
Finally, differences in punishment flows across network treatments can result in a variation in efficiency between perfect and imperfect networks – the heavier punishment amount used in the CN reduces average payoffs to levels below the Nash equilibrium strategy payoff. The significant loss of efficiency from punishment expenditures echoes findings originally highlighted in Ostrom et al. (Reference Ostrom, Walker and Gardner1992) and replicated in Cason and Gangadharan (Reference Cason and Gangadharan2015), that the costs of perfect peer monitoring and punishment can significantly erode payoffs in the CPR dilemma. In the UCN networks, however, lower aggregate levels of more severe punishment – which tends to be more prosocial – elicits marginally higher payoffs. Lower punishment expenditures also elicit marginally higher payoffs in the DCN. Overall, these findings suggest that neither removing ties nor changing the network property of directedness necessarily increases efficiency due to the same underlying dynamics. They also confirm findings from previous studies that perfect peer monitoring and punishment institutions need not be socially efficient. In addition, we show that even the imperfect peer monitoring and punishment institutions considered here need not be socially efficient.
Why do peer punishment and monitoring not have a more positive impact on cooperation? One reason may be that since free riding takes longer to be identified in these nonlinear environments, a longer period of interactions is necessary for individuals to realize the long-run benefits of punishment (Gächter et al., Reference Gächter, Renner and Sefton2008). There may well be differences across networks if learning is faster in the CN because networks are denser; we found that beliefs were closer to the actual appropriation levels in the CN compared to UCN and DCN networks. De Geest et al. (Reference De Geest, Stranlund and Spraggon2017) also have some evidence that full observability of the outsiders allowed the insiders to better coordinate their harvests, despite their inability to fully deter the outsiders. However, another possibility may be that peer monitoring and sanctioning are effective only when they are combined with other institutional features that allow individuals to make the burden of responsibility of acting prosocial clearer with each other by communicating to coordinate their harvesting and punishment strategies (Ostrom et al., Reference Ostrom, Walker and Gardner1992; Boyd et al., Reference Boyd, Gintis and Bowles2010; Cason and Gangadharan, Reference Cason and Gangadharan2016; Molleman et al., Reference Molleman, Kölle, Starmer and Gächter2019).
Our study is not without some limitations. Firstly, our results emerge from the interaction of imperfect monitoring and punishment opportunities, i.e., we cannot unpack if such patterns are due to different monitoring networks that determine the information feedback available to players, or punishment opportunities that determine the possibility of sanctioning peers. Given this, it could be that the effect of the different networks on appropriation could be washed away when introducing punishment. Past studies, however, offer mixed evidence on the separate impact of feedback alone. For instance, Carpenter et al. (Reference Carpenter, Kariv and Schotter2012) and Boosey and Isaac (Reference Boosey and Isaac2016) consider the CN and UCN. The former vary both monitoring and punishment opportunities (similar to our paper) and the latter manipulate only punishment networks and hold perfect feedback fixed across both treatments, but both papers find qualitatively similar results in terms of appropriation, aggregate and individual-level punishment flows and efficiency. Cox and Stoddard (Reference Cox and Stoddard2015) also found that varying the individual and aggregate-level feedback does not impact cooperation in a linear PG dilemma with a stranger-matching design (also see Apesteguia and Maier-Rigaud (Reference Apesteguia and Maier-Rigaud2006) and Cason and Khan (Reference Cason and Khan1999)). Nevertheless, we cannot rule out that perfect monitoring will have distinct effects when we vary only punishment networks, and we believe that this is an important empirical question for future investigation. Similarly, whether eliciting beliefs affects cooperation in this nonlinear CPR setting is also an open question. Past work has found mixed evidence ranging from either having no effect (Wilcox and Feltovich, Reference Wilcox and Feltovich2000), a negative effect through inducing more self-interested behavior (Croson, Reference Croson2000), or a positive effect through inducing behavior closer to the socially-optimal level (Gächter and Renner, Reference Gächter and Renner2010).
To take this work forward, some natural extensions are to first separate the effects of perfect and imperfect monitoring (and the observability of others' choices) from the sanctioning opportunities available to each agent, by manipulating the punishment network structures relative to cases without any monitoring and sanctioning. To ensure that the differences are not driven simply by network density or directedness, a larger set of networks could be considered as in Carpenter et al. (Reference Carpenter, Kariv and Schotter2012).Footnote 14 In addition, to check how sensitive our results are to the design choices used in this experiment, future studies can examine how behavior evolves over a different set of features, including using a benchmark without punishment and a larger number of rounds, relying on partner as opposed to stranger-matching, and using different types of punishment technologies.
Supplementary material
The supplementary material for this article can be found at https://doi.org/10.1017/S1355770X19000457
Acknowledgements
We thank the Grantham Research Institute on Climate Change and the Environment for funding this research. We are grateful for comments received at WCERE 2018, EAERE 2016, Bioecon 18, ICES-ASC 2016, Grantham Research Institute on Climate Change and the Environment Empirical Seminar and the Environment cluster seminar at the Department of Geography and Environment. We extend special thanks to John Stranlund and Timo Goeschl, as well as the guest editor and referees in this special issue for their very helpful suggestions, and to Timothy Cason for the z-tree code for the experiment (which we modified for this experiment). All remaining errors are ours.