INTRODUCTION
Diffraction analyses have been used for decades to analyze solid state formulations in both powder and tablet form. The ability to perform a nondestructive analysis is often combined with the capability of Search/Match algorithms to successively identify phases and reanalyze the residual pattern until multiple phases have been identified. One of the strengths of the diffraction technique has been to routinely identify multicomponent materials from a single analysis, without pretreatment to physically or chemically separate out the components, thus minimizing the possibility that the specimen has been altered by the preparation method. However this method depends on having a database that can correctly analyze each successive phase in the multicomponent analysis.
EXPERIMENTAL
Samples were chosen from contributions by the authors and co-workers. The samples were all commercially formulated products. The great majority were purchased from convenience stores near the author’s work locations. Three sets of samples were analyzed, one each for each year from 2003 to 2005. The sets were deliberately chosen to test the ability to analyze formulations in each of three different fields: Off the shelf pharmaceuticals—12 formulations; Vitamins and herbal supplements—10 vitamins, 6 natural products; Fertilizers—8 formulations. Post analysis we decided to separate out the vitamins and herbal supplements because of their logical grouping based on phase analysis.
In addition to the 36 formulations listed in Table I, several vitamins were analyzed multiple times over a period of 2 years, to look at lot-to-lot variability. These samples were chosen since they did exhibit some variability on initial testing. The selection was haphazard, with the exception that all samples received were analyzed and the study focused on as-received tablets taken directly from commercial packaging. In general, the formulated products are high sales volume items commonly found in the stores in North America.
Specimens were analyzed on five different powder diffractometers. The authors are grateful to Rigaku-MSC, Inc., PANalytical, and Bruker-AXS, each of whom donated diffractometer time to support this study during ICDD’s X-ray Diffraction Clinics. Samples were run on a Rigaku MiniFlex desktop X-ray Diffractometer, a PANalytical Alpha One with an X’Celerator Detector, and a Bruker D4 equipped with a Vantec detector. In addition, some specimens were analyzed on a PANalytical X’Pert Pro MPD with an X’Celerator Detector during an evaluation trial at Oak Ridge National Laboratory. Others were analyzed on the synchrotron at the Argonne Advanced Photon Source on beamline ID-32. In all cases, the authors had unique and temporary access to the equipment; therefore each sample was analyzed a single time.
Tablets were ground in a mortar and pestle and then one of three sample preparation methods was used, depending on the instrument available for analysis. At the ICDD, during clinics, specimens were typically run as thick powders using cavity mounts supplied by the vendors. In this method, cavity mounts were backfilled with powdered tablets. The samples were then run using Cu radiation typically between 5° and 70° 2θ. Total data collection time was approximately 2 h using a scintillation counter and Vantec detector. The data collection time was extended to 6–10 h when using PANalytical’s Alpha One configuration. At Oak Ridge National Laboratory, a thin film sample preparation method was used. Samples from powdered tablets were lightly dusted onto a Vaseline® coated zero background holder. Ni filtered Cu radiation was used with a 15 min total data collection time. The area from 5° to 80° 2θ was scanned using a 0.01° step size. At Argonne National Laboratory, a capillary sample preparation was used. Powdered tablets were placed into 1 mm glass capillaries. A wavelength of 0.495 Å was selected and data collection covered approximately 30° 2θ.
TABLE I. Product formulations analyzed by X-ray diffraction. Pharmaceuticals, vitamins, and health supplements were studied as ground tablets, and fertilizers as loose powders.
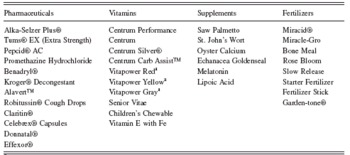
1 Vitapower comes in multiple colored tablets each with a different formulation.
The large variation in sample preparation methods, incident power, and instrumental optics resulted in a body of data with a wide variability in signal to noise and peak resolution. This could be the topic of another paper. In general, predictable results were obtained if one considered data collection times, detector responses, step sizes, and counting statistics represented by capillaries, thin films, and deep cavity mounts. We were able to perform phase identification and formulation analysis on all samples despite several orders of magnitude differences in detector count rates. The vitamin samples are particularly challenging test cases since they are typically composed of combinations of many ingredients at relatively low concentrations. Some multivitamins list over 30 bulk ingredients, combining minerals, herbal supplements, and vitamins in a single formulation.
DISCUSSION
By the end of the year 2005, the PDF will have ∼497,000 published, edited, and standardized reference material data sets. The data sets are collated into databases that are based on inorganic (PDF-4+, PDF-2) and organic chemistries (PDF-4/Organics). A detailed description of the contents of each database is available at http://www.icdd.com. However, an important feature, as we shall see, is that the databases contain common materials of the other’s chemistry to aid researchers in material identification and formulation analysis. Thus, today’s sophisticated yet common formulations with mixed inorganic, polymer, and organic chemistries can be identified and solved. Another important development is the transition of reference data from the historic d,I list pairs and “stick” figures into digitized patterns. The on-the-fly calculation of digitized patterns enables users to explore the effects of instrumental and optical configurations, variable wavelength, and slit geometries. In a recent development, PDF-4+ contains the ability to calculate X-ray, neutron, and electron diffraction patterns based on the appropriate scattering functions. With digital pattern capabilities users can explore the realm of semicrystalline, nano-crystalline, and amorphous content, thus expanding the analysis capability and taking advantage of the ability of powder diffraction to analyze materials that consist of imperfect single crystal domains.
Since more than 40 specimens were examined, details of each analysis will not be given; rather results from the view of capability and capability enhancements will be discussed.
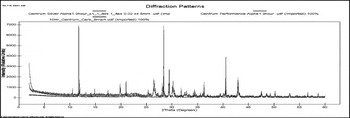
Figure 1. Data from powdered tablets of Centrum® vitamins: Carb Assist™, Silver®, and Performance. Typically, seven or more phases are identified in these multicomponent vitamin tablets.
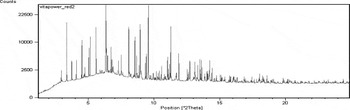
Figure 2. Data taken at the Argonne Advanced Photon Source (APS) on the powdered vitamin formulation of Vitapower “red” tablets. Vitapower tablets come in many colors each representing different formulations.
The analysis of off-the-shelf pharmaceuticals has been described in further detail in another paper (Fawcett et al., Reference Fawcett, Faber and Hubbard2004).
The strongest conclusion from this study was that the bulk formulations of >95% of all specimens from three different areas of commerce were analyzed and identified. The only specimens that were difficult to identify were materials of very low crystallinity or noncrystalline. To solve this wide range of problems, we not only used the diffraction data contained in the Powder Diffraction File, but also liberally used chemistry based searches and a knowledge of nanoparticle effects on diffraction patterns. Embedded searches are contained in the classification systems, structural prototypes, and pattern calculation algorithms in the PDF (Kabekkodu et al., Reference Kabekkodu, Faber and Fawcett2002; Faber, Reference Faber2004). Automated analyses performed well, but automated analyses complimented by chemistry and material knowledge performed even better. The study continuously demonstrated the power of a comprehensive reference collection where best-fit analyses were routinely solved using data from more than one database source contributor.
Within the PDF, the data source contributor was relatively easy to determine because of a new numbering system implemented in the PDF in 2003. Data sources are identified by a two-digit number prefix to the PDF file number. These are:
00: Derived from powder diffraction data, historically compiled by the ICDD
01: Data derived from ICSD single crystal data, calculated and standardized by ICDD
02: Data derived from CSD single crystal data, calculated and standardized by ICDD
03: Data derived from NIST references, calculated and standardized by ICDD
04: Data derived from LPF single crystal data, calculated and standardized by ICDD
ICSD=Inorganic Crystal Structure Database, CSD=Cambridge Structural Database, NIST=National Institute of Standards and Technology, LPF=Linus Pauling File
To see the value of multiple database contributions, one only needs to look at the PDF number prefix from the final Search/Match solutions.
The standardization process is critical because it allows users to compare data from each of the database contributors in a standard format. Editorial teams from each of these database organizations may in turn be standardizing data from hundreds of journal and author sources. This is an enormous effort when considering all editorial contributions from all collaborating database organizations. This is a major reason why proprietary or author-generated databases fail or even worse. Worse being that the user does not solve problems
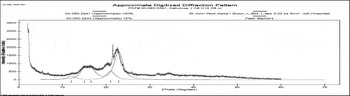
Figure 3. Diffraction pattern from a tablet of St. John’s Wort. Shown below the pattern is simulation of cellulose I and cellulose III, broadened to approximately 80 A. This sample is somewhat unusual with the clear mixture of cellulose forms from the ground plants components of St. John’s Wort.
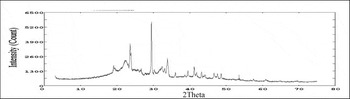
Figure 4. Commercial fertilizer stick containing a blend of crystalline and microcrystalline phases. Urea is of small crystallite size. Highly crystalline phases include potassium nitrate, calcite, and sodium hydrogen phosphate.
that can readily be solved using standardized data or forces an incorrect answer based on the limited information.
Data shown in Figure 1 are from three separate Centrum® vitamin formulations, Carb Assist™, Silver®, and Performance. In this analysis, the identification of many of the basic inorganic salts matched reference materials derived from ICSD single crystal data. The experimental Powder Diffraction File frequently matches the patterns for Vitamin C, calcite, and the calcium hydrogen phosphate hydrate salts. Contributions calculated from the CSD were used to identify most of the Vitamin B in the Vitapower (Figure 2) formulations and many active ingredients such as Loratidine, Ventafaxine hydrochloride, and Celecoxib in our study of pharmaceutical tablets.
Small crystallite size materials such as cellulose and starch were typically identified by simulating small crystallite domains in the digital diffraction pattern, as shown for a specimen of St. John’s Wort in Figure 3. The crystalline pattern in this specimen is readily identified as stearic acid. The active antioxidant was claimed to be present by the manufacturer at 0.3 wt %, however, it was not observed. Fertilizers often contain microcrystalline ingredients to control dissolution rate. Similarly, microcrystalline cellulose was found in many pharmaceutical formulations. In addition, we identified microcrystalline urea, corn starch, and alpha lactose monohydrate in other samples. Micronized ingredients are becoming more commonplace as a means of controlling dissolution in many formulations. We found microcrystalline ingredients in ∼61% of the formulations listed in Table I. In the analysis of microcrystalline materials, background selection and peak identification can be difficult because of the breadth and shape of the diffraction peaks. The authors had to frequently override default criteria used in automated X-ray analysis programs so that broad peaks can be correctly located and integrated.
A useful tool for analyzing similarities and differences among groups of materials in common material classes is cluster analysis. Cluster analysis modules have been incorporated
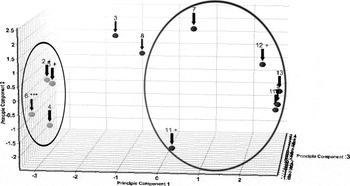
Figure 5. Principal component analysis (PCA) of a group of vitamins and health supplement formulations. Results obtained from the cluster analysis module of X’Pert HighScore Plus.
into several commercial software packages. The basic principles of cluster analysis have been described in a series of papers by Gilmore et al. (Reference Gilmore, Barr and Dong2004a, Reference Gilmore, Barr and Dongb). A short description is that cluster analysis correlates individual scans within a group. The digital diffraction data are statistically analyzed and examined for similarities and differences in the patterns. This is a very powerful tool when combined with phase identification, since the phase identification provides an intelligent interpretation of the cluster results, while the complimentary cluster analysis rapidly points out which scans are significantly different.
In the example above, 12 diffraction patterns from vitamins and supplements listed in Table I were analyzed by cluster analysis. As shown in Fig. 5, the analysis identified two clusters (circled) and two patterns that were not clustered. Phase identification shows that the cluster, circle on the left, is composed of natural materials with cellulose as a major phase (roots, bark, leaf). The cluster, circled on the right, is composed of a series of multivitamins that had common components such as calcite, sylvite, and periclase as sources of Ca, K, Cl, and Mg, respectively. The two outlier samples in red included a children’s vitamin that contained a very large amount of sucrose and the sample of saw palmetto that contained significant amounts of silica. The combination of phase identification and cluster analysis provided results that were fast to analyze and made common sense.
Our results also confirmed several other conclusions frequently reached in international studies. In high quality data sets inorganic crystalline materials above 1 wt % composition were identified. In several cases, more than seven phases could be identified in complex multivitamin formulas. When analyzing noncrystalline materials and very light element phases, detection suffers in direct proportion to the decrease in signal to noise.
CONCLUSIONS
The bulk formulations of 97% of all specimens from three different classes of materials were analyzed and identified. The only specimen that was difficult to identify was a material of very low crystallinity and variable chemistry. Saw palmetto was the only sample listed in Table I that could not be cleanly identified, in all other formulations a range from two to ten phases were found in each specimen. To solve this wide range of problems, we not only used the diffraction data contained in the Powder Diffraction File but also liberally used the chemical searches available with the database software and knowledge of nanoparticle effects on diffraction patterns (Figures 4 and 5). Automated analyses performed well, but automated analyses complimented by chemistry and material knowledge performed even better. The majority of the analysis results used multiple database sources in the phase identification solution. For formulation analysis, this is critical since the determination of components of a formulation depends on successive identification and analysis of residual patterns. Digital pattern analyses enabled the authors to identify and analyze semicrystalline and small crystallite size phase that were commonly present in many formulations.
ACKNOWLEDGMENTS
The authors would like to acknowledge the valuable assistance of Richard L. Harlow and Peter L. Lee in collecting data at Argonne National Laboratory, and Bernie Squires for sample preparation and data collection at the 2003 ICDD clinic.
APPENDIX: PHASE IDENTIFICATION OF FORMULATIONS
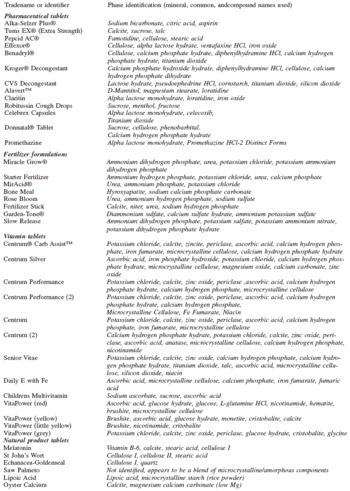
Authors’ note: Many of the above formulations contain ingredients in concentrations below 5 wt %. Detection of these phases is dependent upon sample preparation, experimental conditions, and search/match parameters. Experimental conditions were not optimized to identify minor and trace phases. The phase identification can be expected to change if conditions are changed.