The awfulness of 2020 has been described with eloquence and detail by many authors around the world. Put simply, at nearly every turn, events went in the wrong direction. So, it is nice to report a 2020 project that went well – in fact, for a change, something that went wonderfully better than expected.
Early in the COVID-19 pandemic, early career researchers were hit hard – particularly those having to work from home with young children. The leadership of the Psychiatric Genomics Consortium (PGC) partnered with the editors of Psychological Medicine to commission a series of invited reviews. This effort had multiple intentions.
Above all else, we believed we needed a set of high-quality reviews to capture the state-of-the-science in psychiatric genetics. This field is moving quickly and important papers are appearing with such regularity that it can be difficult even for cognoscenti to keep up. We wanted these reviews to have similar structures to enable cross-paper comparisons. We are unaware of any systematic attempt to review all major psychiatric disorders in a similar way and appearing in the same place at the same time. In addition, the reviews were commissioned from sets of early career researchers. This served several purposes above and beyond a review paper for a CV. Writing a field review requires mastery of the literature which pays dividends in writing subsequent grants. Moreover, some of the teams that formed for these reviews will continue to collaborate in the future.
We, therefore, commissioned 13 reviews (Table 1) from a total of 52 early career researchers. The topics correspond to the disorder groups around which the PGC is organized (typical Diagnostic and Statistical Manual of Mental Disorders/International Classification of Diseases groupings) and these reviews follow that pattern. Considerable detail about the background, epidemiology, genetic epidemiology, current genomic findings, controversies and future plans are in the reviews. We wish to add to these reviews a number of higher-level conclusions that have emerged from hundreds of psychiatric genetics studies in the ‘genomic era’ that began in 2007 (Sullivan & Geschwind, Reference Sullivan and Geschwind2019).
Table 1. Topics for commissioned reviews
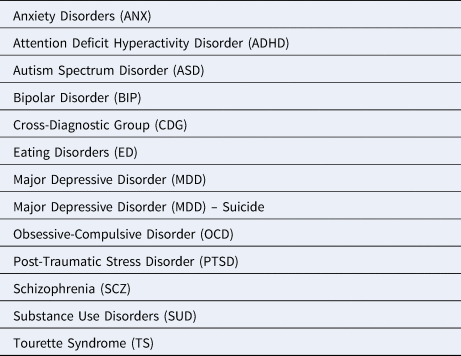
Open science. The genomic era in psychiatry has become distinctive in its openness (Sullivan et al., Reference Sullivan, Agrawal, Bulik, Andreassen, Borglum and Breen2018). The field used to be characterized by research groups set up as independent and occasionally collaborating or warring city-states. We learned early that no single group had sufficiently large sample sizes and that cooperation was essential to progress. Ready collaboration has become the norm. Investigators almost always make summary statistics freely available; coupled with clever ways to use these aggregated data, psychiatric disorders often appear in genomic papers about other biomedical diseases, functional genomic studies, and methods development. Individual data can often be obtained from controlled-access repositories (e.g. the Database of Genotypes and Phenotypes, dbGaP, or the European Genome-phenome Archive), and the PGC has a streamlined process for secondary data analyses.
Complexity. Twenty years ago, many authors believed that the genetic architectures would be simple with a few major genes underlying these disorders. Increasingly large genomic studies – common variation, rare copy number variants, ultra-rare coding variation, and even whole-genome sequencing in case-control, trio, and dense pedigree designs – have found no evidence to support these beliefs, and the studies were large enough to make detection power nearly 100%. Along the way, we found that early methodological approaches like linkage analyses and candidate gene association studies (i.e. genotyping one or a few genetic markers in COMT, DISC1, or the serotonin transporter in a few hundred cases and controls) had essentially no yield due to being severely under-powered (Border et al., Reference Border, Johnson, Evans, Smolen, Berley, Sullivan and Keller2019; Farrell et al., Reference Farrell, Werge, Sklar, Owen, Ophoff, O'Donovan and Sullivan2015; Sullivan, Reference Sullivan2007; Sullivan, Reference Sullivan2013). Instead, we find complexity. All psychiatry disorders have contributions from many genes. The preponderance of risk is from common genetic variants of individually small effects, typically in regulatory (not coding) regions. Far smaller subsets of patients with earlier onset/more severe forms of disorders carry a rarer variant of stronger effect. To date, over all disorders, the PGC has studied over 485 000 cases with one of the disorders in Table 1 and discovered genome-wide significant findings in 445 loci (about 10% are significant for more than one disorder). The PGC group leaders project that over 2 million cases will be in analyses by 2025.
New epidemiology. Adding genetic data to typical clinical and epidemiological study designs has proven to be more informative and important than many might have suspected – particularly in psychiatry where a lack of anatomic, biochemical, and pathophysiological defining features is a crucial limit to progress (these are psychiatric disorders and not diseases). For example, genetic data can be used to understand the relation between a risk factor and a disorder: careful evaluation of genetic data using ‘Mendelian randomization’ led to the conclusion that the association of neonatal vitamin D levels and subsequent risk of schizophrenia appears to be non-causal (perhaps due to lesser sunlight exposure during pregnancy in women with or at risk for schizophrenia) (Revez et al., Reference Revez, Lin, Qiao, Xue, Holtz, Zhu and McGrath2020). A comprehensive evaluation of 106 risk factors for major depression (MD) identified a subset that was plausibly causal and potentially modifiable (Choi et al., Reference Choi, Stein, Nishimi, Ge, Coleman, Chen and Smoller2020).
Other methods use genetic data from large samples to conduct analyses that were previously only possible in twin and pedigree studies. It is straightforward to evaluate the single-nucleotide polymorphism (SNP)-heritability of a disorder or of a putatively more homogeneous subtype of a disorder (Bulik-Sullivan et al., Reference Bulik-Sullivan, Loh, Finucane, Ripke, Yang and Neale2015b). For instance, we recently found that the SNP-heritability of severe major depressive disorder (MDD) (i.e. people receiving electro-convulsive therapy) was far higher than for mild or moderate MDD (Clements et al., Reference Clements, Karlsson, Lu, Juréus, Rück, Andersson and LandénIn Press). In addition, we can compute the genetic correlation between disorders to quantify the extent to which the genetic basis of two disorders overlap (Bulik-Sullivan et al., Reference Bulik-Sullivan, Finucane, Anttila, Gusev, Day and Neale2015a). Psychiatric disorders are notable for their strong genetic overlaps (unlike neurological disorders) (Anttila et al., Reference Anttila, Bulik-Sullivan, Finucane, Bras, Duncan, Escott-Price and Neale2018). Based on its surprising genetic correlations with body mass and a set of metabolic traits, anorexia nervosa may be best understood as a metabo-psychiatric disorder (Watson et al., Reference Watson, Yilmaz, Thornton, Hübel, Coleman, Bryois and Bulik2019).
The perils of going large? A persistent tension in the field is the tradeoff between smaller samples with detailed phenotypes and larger samples with light phenotyping. However, a better way to consider the problem is whether the ascertainment and phenotyping used in a study yield a high probability of moderate or severe caseness in putative cases and a low probability in putative controls. If misclassification rates are low (a few percent at most), then power will be minimally impacted. There are standard albeit time-consuming ways to obtain deep phenotyping (personal interviews with medical record review) but also inexpensive/rapid ways that can approximate these ends with larger samples for detection of small genetic effects. For instance, the epidemiology, genetic epidemiology, and genetics of schizophrenia defined by being hospitalized twice in Sweden are indistinguishable from conventional samples (Lichtenstein et al., Reference Lichtenstein, Bjork, Hultman, Scolnick, Sklar and Sullivan2006; Lichtenstein et al., Reference Lichtenstein, Yip, Bjork, Pawitan, Cannon, Sullivan and Hultman2009; Ripke et al., Reference Ripke, O'Dushlaine, Chambert, Moran, Kähler, Akterin and Sullivan2013; Schizophrenia Working Group of the Psychiatric Genomics Consortium, 2014). The Cardiff group has obtained a large number of schizophrenia cases via clozapine clinics (Pardinas et al., Reference Pardinas, Holmans, Pocklington, Escott-Price, Ripke, Carrera and Walters2018). Brief self-reports for lifetime MD and bipolar disorder, or self-report current symptom scales, may be particularly problematic and might bias results away from those obtained in samples of greater severity (Cai et al., Reference Cai, Revez, Adams, Andlauer, Breen, Byrne and Flint2020). As we have argued elsewhere, all ascertainment and phenotyping strategies need to be evaluated empirically (Wray et al., Reference Wray, Ripke, Mattheisen, Trzaskowski, Byrne and Sullivan2018), and papers now typically contain information that allows evaluation of these issues.
Getting global. Finally, as noted in many of the reviews as well as in the PGC review in Cell (Peterson et al., Reference Peterson, Kuchenbaecker, Walters, Chen, Popejoy, Periyasamy and Duncan2019), we urgently require larger samples from individuals of non-European ancestry. This carries surmountable technical challenges but also many benefits including the possibility of extending the potential advantages of genomically-driven precision psychiatry to anyone.
Acknowledgements
We thank NaEshia Ancalade and Jill Opalesky for assistance in organizing the reviews. PFS was supported by the Swedish Research Council [Vetenskapsrådet, award D0886501 and US NIMH (U01 MH109528 and R01 MH077139)]. PFS reports the following potentially competing financial interests: Lundbeck (advisory committee, grant recipient) and RBNC Therapeutics (advisory committee, shareholder). KSK has no potential conflicts of interest.