Despite its efficacy (Santoft et al., Reference Santoft, Axelsson, Öst, Hedman-Lagerlöf, Fust and Hedman-Lagerlöf2019), the accessibility of cognitive behavioral therapy for depression (CBT-D) is often limited by high cost and cultural barriers (Corrigan, Druss, & Perlick, Reference Corrigan, Druss and Perlick2014; Shi, Shen, Wang, & Hall, Reference Shi, Shen, Wang and Hall2020). The preference for self-reliance and stigma in some communities may hamper help-seeking behavior from mental health professionals (e.g. Corrigan et al., Reference Corrigan, Druss and Perlick2014; Shi et al., Reference Shi, Shen, Wang and Hall2020).
In addition to being a common symptom of major depressive disorder (MDD) (McClintock et al., Reference McClintock, Husain, Wisniewski, Nierenberg, Stewart, Trivedi and Rush2011; Tsuno, Besset, & Ritchie, Reference Tsuno, Besset and Ritchie2005), sleep disturbance, in particular insomnia, also predicts its onset, maintenance, and reoccurrence (Fang, Tu, Sheng, & Shao, Reference Fang, Tu, Sheng and Shao2019; Franzen & Buysse, Reference Franzen and Buysse2008; Vargas & Perlis, Reference Vargas and Perlis2020), as well as suicidal ideations and attempts (Harris, Huang, Linthicum, Bryen, & Ribeiro, Reference Harris, Huang, Linthicum, Bryen and Ribeiro2020; Liu et al., Reference Liu, Steele, Hamilton, Do, Furbish, Burke and Gerlus2020). Because of the high comorbidity and the bidirectionality of sleep disturbances and depression, sleep-focused interventions are recommended to be included in the treatment of MDD (Harvey, Murray, Chandler, & Soehner, Reference Harvey, Murray, Chandler and Soehner2011).
Sleep treatments, in particular CBT for insomnia (CBT-I), are efficacious in alleviating non-sleep symptoms in MDD (Ballesio et al., Reference Ballesio, Aquino, Feige, Johann, Kyle, Spiegelhalder and Baglioni2018; Feng, Han, Li, Geng, & Miao, Reference Feng, Han, Li, Geng and Miao2020; Ho, Chan, Lo, & Leung, Reference Ho, Chan, Lo and Leung2020). Among those with comorbid depression and insomnia, improvement in sleep in treatment predicts improvements in depression (Ballesio et al., Reference Ballesio, Aquino, Feige, Johann, Kyle, Spiegelhalder and Baglioni2018; Bei et al., Reference Bei, Asarnow, Krystal, Edinger, Buysse and Manber2018).
Sleep-focused treatments are well received (Ho, Chung, Yeung, Ng, & Cheng, Reference Ho, Chung, Yeung, Ng and Cheng2014), in part because they are perceived by some to be more ‘physiological’ than ‘psychological’ (Parker, Cheah, & Roy, Reference Parker, Cheah and Roy2001). CBT-I can be a preparatory and first-step prior to other more intensive treatments (Baddeley & Gros, Reference Baddeley and Gros2013; Ho, Yeung, Ng, & Chan, Reference Ho, Yeung, Ng and Chan2016). This study examined the efficacy of a non-assisted smartphone-based CBT-I for those with both depression and insomnia.
Internet-based and smartphone-based CBT-I
There is evidence to suggest that some help-seekers prefer self-help treatments (Hanson, Webb, Sheeran, & Turpin, Reference Hanson, Webb, Sheeran and Turpin2016; Segal, Hodges, & Hardiman, Reference Segal, Hodges and Hardiman2002). Self-help treatments can be a particularly viable alternative for those who are reluctant to seek professional help for mental health issues (Chin, Chan, Lam, Lam, & Wan, Reference Chin, Chan, Lam, Lam and Wan2015). Numerous studies have demonstrated the efficacy of self-help CBT-I in treating insomnia (Blom et al., Reference Blom, Tillgren, Wiklund, Danlycke, Forssén, Söderström and Kaldo2015; Horsch et al., Reference Horsch, Lancee, Griffioen-Both, Spruit, Fitrianie, Neerincx and Brinkman2017; Krieger et al., Reference Krieger, Urech, Duss, Blättler, Schmitt, Gast and Berger2019; Vedaa et al., Reference Vedaa, Kallestad, Scott, Smith, Pallesen, Morken and Sivertsen2020). Internet-based CBT-I (iCBT-I), in particular, is efficacious in alleviating not just insomnia but also depressive mood in insomnia patients (Ho et al., Reference Ho, Chan, Lo and Leung2020; Ye et al., Reference Ye, Zhang, Chen, Liu, Li, Liu and Jiang2015). Therapist-guided iCBT-I has been demonstrated to have comparable efficacy for insomnia and depression as therapist-guided iCBT-D in patients suffering from both (Blom, Jernelöv, Rück, Lindefors, & Kaldo, Reference Blom, Jernelöv, Rück, Lindefors and Kaldo2017).
The current study further extends this line of work to test the efficacy of iCBT-I in a pure self-help format, without any input from a therapist, for those with both depression and insomnia. Previous trials have demonstrated the efficacy of unguided iCBT on symptoms of depression and insomnia (Christensen et al., Reference Christensen, Batterham, Gosling, Ritterband, Griffiths, Thorndike and Mackinnon2016; Glozier et al., Reference Glozier, Christensen, Griffiths, Hickie, Naismith, Biddle and Ritterband2019). The current trial tested the efficacy of a smartphone-based intervention. Given the high accessibility of smartphones, mobile application is a promising platform to deliver timely treatment and professional help for common mental health problems (Economides et al., Reference Economides, Ranta, Nazander, Hilgert, Goldin, Raevuori and Forman-Hoffman2019; Linardon, Cuijpers, Carlbring, Messer, & Fuller-Tyszkiewicz, Reference Linardon, Cuijpers, Carlbring, Messer and Fuller-Tyszkiewicz2019; Mantani et al., Reference Mantani, Kato, Furukawa, Horikoshi, Imai, Hiroe and Kawanishi2017). Considering the increasing smartphone penetration, smartphone self-help application as a flexible and scalable modality of intervention can potentially help lessen the pressing needs for treatments of common mental disorders, including depression and insomnia.
Well-received, low-intensity treatments for depression and insomnia via smartphones might be a convenient and cost-effective adjunct to professional mental health services to overcome the economic, cultural, and personal barriers. This might be particularly pertinent in the context of the unprecedented COVID-19 pandemic. The detrimental impacts of the pandemic on mental health (Salari et al., Reference Salari, Hosseinian-Far, Jalali, Vaisi-Raygani, Rasoulpoor, Mohammadi and Khaledi-Paveh2020), especially for those with pre-existing mental disorders (Campion, Javed, Sartorius, & Marmot, Reference Campion, Javed, Sartorius and Marmot2020), further overwhelm the already overstretched public healthcare system and leave those in need without proper and timely care. Social distancing has made it difficult for those in need of professional help from seeking them. Smartphone-based services might help alleviate such burdens on both patients and their care providers.
Current study
The present study was a two-arm parallel waitlist controlled randomized controlled trial (RCT) investigating the efficacy of proACT-S, a smartphone-based, non-assisted self-help CBT-I in patients with comorbid depression and insomnia. The trial was conducted in Hong Kong, where the high smartphone penetration is high (85.8%; Census and Statistics Department, 2017). We hypothesized a greater reduction in both depression and insomnia severity, and improvement in sleep quality in the treatment group compared to waitlist control at the post-treatment follow-up. The waitlist control group was anticipated to have a significant decrease in the outcome measures after receiving the same treatment.
Anxiety symptomology was included as a secondary outcome because of the high comorbidity of anxiety disorders and depressive disorders (Kaufman & Charney, Reference Kaufman and Charney2000) and the overlap in their symptoms and risk factors, including sleep disturbances. Many have recognized the potentials of sleep treatments, such as CBT-I, and advocate for its use as a transdiagnostic approach to common mental disorders (Harvey et al., Reference Harvey, Murray, Chandler and Soehner2011). Subjective health was also included as a secondary outcome because of the potential health implications of sleep disturbances (Lekander et al., Reference Lekander, Andreasson, Kecklund, Ekman, Ingre, Akerstedt and Axelsson2013; Tan et al., Reference Tan, Storm, Reinwand, Wienert, de Vries and Lippke2018).
Treatment expectancy refers to the prognostic expectations about the treatment outcomes, both positive and negative (Goldstein, Reference Goldstein1962). Because treatment expectancy of post-treatment outcomes has been found to influence CBT treatment outcomes (Alaoui et al., Reference Alaoui, Ljótsson, Hedman, Svanborg, Kaldo and Lindefors2016), we included it as a covariate. We also measured user's acceptability of the treatment, given its relative novelty. COVID-19 has brought about an unprecedented crisis to global health. Our trial started before, and closed during, the pandemic. We included a comparison between those who completed the trial before and those during the pandemic.
Methods
Participants
The study was conducted in Hong Kong. Participants were recruited from university mass emails, community posters, and online advertisements on various platforms. Eligible participants who completed the study received HKD$100 as a token of appreciation. Interested participants went through two stages of screening to confirm their eligibility (reported below and in the published protocol; Hui, Wong, Ma, Ho, & Chan, Reference Hui, Wong, Ma, Ho and Chan2020). All participants provided written informed consent before proceeding to the screening.
A total of 2224 individuals completed the first stage of screening, which was a brief online self-report assessment. Eligible respondents were Hong Kong residents aged 18 years or above who were able to comprehend Chinese and type in Chinese or English, bothered by their sleep complaints for at least 3 months and for at least three nights per week, scored 8 or above on the Insomnia Severity Index (ISI) and 10 or above on the Patient Health Questionnaire (PHQ-9), able to access the Internet with smart devices using iOS or Android operational system, have a regularly used email address, and were able to provide informed consent.
The 685 respondents who met the above criteria were invited to proceed to the second stage of screening, which was a telephone diagnostic interview using the modified Chinese version of the Revised Clinical Schedule (CIS-R) (Chan et al., Reference Chan, Wong, Chen, Ng, Hung, Cheung and Lam2017). The interview identified the diagnosis of MDD, insomnia, as well as other comorbidities based on the International Statistical Classification of Diseases and Related Health Problems – Tenth Revision (ICD-10). The exclusion criteria included the diagnosis of psychosis or schizophrenia, past participation in the pilot trial of proACT-S, current and regular intake of prescribed psychiatric drugs for depression or insomnia, scored 2 or above on the suicidal ideation item of the Beck Depression Inventory-II (BDI-II; Beck et al., Reference Beck, Steer, Ball and Ranieri1996), report of current or past suicidal plans or acts in last 12 months, received psychological intervention on at least a monthly basis, or concurrent participation of any clinical trials targeting depression and/or insomnia. The prospective respondents (n = 188) who did not attend the diagnostic interview were excluded from the study.
For the diagnosis of insomnia, prospective participants were asked about their subjective experience of sleep disturbances and the additional criteria to quantify their sleep complaints as noted in DSM-5, such as the duration, severity, and frequency of their sleep problems (American Psychiatric Association, 2013; Chung et al., Reference Chung, Yeung, Ho, Ho, Yung, Yu and Kwok2014; Sivertsen et al., Reference Sivertsen, Pallesen, Friborg, Nilsen, Bakke, Goll and Hopstock2021). The average total sleep time of the participants was <6.5 h. A CONSORT flow diagram is displayed in Fig. 1.

Fig. 1. A CONSORT flow diagram.
Trial design
In this two-arm parallel RCT, after the baseline assessment described above, participants were randomized to the treatment or the waitlist control group based on a 1:1 allocation ratio algorithm in the back-end of the smartphone application. Eligible participants completed the two scheduled assessments and 6-week self-help CBT-I treatment using proACT-S, which was developed by the principal investigator.
Eligible participants in the treatment group started the treatment immediately after randomization. They were invited to complete the second assessment, i.e. the week 6 follow-up, after the 6-week treatment. The third assessment, the week 12 follow-up, was administrated 6 weeks after the second assessment. Six weeks after the randomization, the waitlist control group was invited to complete the week 6 follow-up. They were then invited to begin the treatment and to complete the week 12 follow-up after the treatment.
This clinical trial was registered retrospectively at ClinicalTrials.gov (Identifier: NCT04228146), and the corresponding RCT protocol was published (Hui et al., Reference Hui, Wong, Ma, Ho and Chan2020). The authors assert that all procedures contributing to this work comply with the ethical standards of the relevant national and institutional committees on human experimentation and with the Helsinki Declaration of 1975, as revised in 2008. All procedures involving human subjects were approved by The University of Hong Kong prior to the data collection (EA1810026).
Blinding
The participants were told that the treatment start date would be randomly assigned to them by proACT-S, after they completed the baseline assessment. This was done to minimize participants' knowledge of their group assignment in the study. The principal investigator was blind to both the outcome assessments and group assignment. The research team was blind to the outcome assessments as they were all completed by the participants via proACT-S. Two research members were not blind to the group assignment as they had to send out reminder emails and messages to the participants to enhance treatment compliance. The data analyst was not blinded to group allocation. However, all the codes are included on OSF (https://osf.io/psk8h/?view_only=4b6e04f61ed24a7a93dfb28d5dbe9056).
CBT-I intervention
The treatment content was constructed based on the Chinese translated version (Yang, Huang, & Lin, Reference Yang, Huang and Lin2008) of a CBT-I treatment manual (Morin & Espie, Reference Morin and Espie2003). The details of the treatment content are reported elsewhere (Hui et al., Reference Hui, Wong, Ma, Ho and Chan2020) and are included in the Supplementary material.
Withdrawal conditions
Participants were regarded as withdrawn from the study if they reported in any of the follow-up assessments: (1) having concurrent psychological treatment at least once per month; (2) taking prescribed psychiatric drugs such as antidepressants, tranquilizers, sleeping pills regularly; or (3) participating in any other academic studies or clinical trials related to insomnia and/or depression. These participants were allowed to continue to use proACT-S within the study period, but their study participation was regarded as withdrawn from the day they reported any of the abovementioned withdrawal conditions. As these participants could potentially produce bias in the treatment evaluation, we treated them as withdrawn. That is, their data available prior to withdrawal were analyzed, but the data since withdrawal were treated as missing. No participants were excluded from analyses in the post-randomization period.
Sample size calculation
A sample size of 199 was required to detect a small-sized effect of 0.2 (Ho et al., Reference Ho, Chan, Lo and Leung2020) using mixed ANCOVA with 12 covariates, assuming a power of 80% and an α value of 5% (Hui et al., Reference Hui, Wong, Ma, Ho and Chan2020). The study set an expected attrition rate of 30%, referencing the mean attrition rates reported in a meta-analysis of self-help CBT-I RCTs (Ho et al., Reference Ho, Chan, Lo and Leung2020); we increased the target sample size to 285 to detect a small effect size (Cohen's d = 0.2) for the score differences in each of the primary outcomes from baseline to post-intervention.
Outcomes
Primary outcomes
Depression symptoms
The Chinese version of Center for Epidemiologic Studies Depression Scale (CES-D; Radloff, Reference Radloff1977) was used to measure the severity of depressive symptoms during the past week. Cronbach's α in this study across the assessments ranged from 0.83 to 0.92.
Insomnia severity
The Chinese version of ISI (Bastien, Vallières, & Morin, Reference Bastien, Vallières and Morin2001) was used to assess the severity of insomnia symptoms and the associated daytime impairment over the past 2 weeks. Cronbach's α in this study ranged from 0.77 to 0.87.
Sleep quality
The Chinese version of Pittsburgh Sleep Quality Index (PSQI; Buysse, Reynolds, Monk, Berman, & Kupfer, Reference Buysse, Reynolds, Monk, Berman and Kupfer1989) was used to measure sleep quality and disturbances during the past month. Cronbach's α in this study ranged from 0.46 to 0.68, which is relatively low but consistent with previous studies (α = 0.55, Chung & Tang, Reference Chung and Tang2006; α = 0.66, Guo, Sun, Liu, & Wu, Reference Guo, Sun, Liu and Wu2016; α = 0.71–0.72 for insomniacs, Tsai et al., Reference Tsai, Wang, Wang, Su, Yang, Huang and Fang2005). There are debates regarding the dimensionality of PSQI, which potentially explains the variations in the scale's internal consistency (Manzar et al., Reference Manzar, BaHammam, Hameed, Spence, Pandi-Perumal, Moscovitch and Streiner2018).
Secondary outcomes
Subjective health
The Chinese version of 12-item Short Form Survey (SF-12) Version 1 (Ware, Kosinski, & Keller, Reference Ware, Kosinski and Keller1995) was used to measure subjective physical and mental health status.
Anxiety
The Chinese version of Hospital Anxiety and Depression Scale – Anxiety subscale (HADS; Zigmond & Snaith, Reference Zigmond and Snaith1983) was used to measure the severity of anxiety symptoms during the past week. Cronbach's α in this study ranged from 0.70 to 0.84.
Other measures
Treatment expectancy
The 6-item Credibility/Expectancy Questionnaire (Devilly & Borkovec, Reference Devilly and Borkovec2000) was modified to measure expectancy toward the treatment. It has been validated in CBT for people with depression or insomnia. Cronbach's α in this study ranged from 0.90 to 0.93.
Acceptability of treatment
The 26-item modified Participant Acceptability/Usability Rating Scale (Ben-Zeev et al., Reference Ben-Zeev, Brenner, Begale, Duffecy, Mohr and Mueser2014) was used to evaluate the self-help treatment delivered via proACT-S.
Demographic information
Participants' age, education level, marital status, gender, and occupation were obtained in the first stage of screening process.
Clinical comorbidities
Participants' clinical comorbidities on generalized anxiety disorder, panic disorder, phobias, and obsessive-compulsive disorder as defined in the ICD-10 were assessed in the second stage of screening by telephone interview.
Adverse events
Questions checking on any potential adverse events in the follow-up questionnaires. Participants were given the contact information (phone and SMS) of the trial team as well as referral information in case additional support was needed.
Statistical analyses
An intention to treat analysis was conducted. Multilevel modeling was employed to evaluate the within-group and between-group effects, and to assess randomized conditions by time interaction. No imputation was performed as previous literature showed that multilevel modeling can handle high attrition without substantially reducing statistical power (Chakraborty & Gu, Reference Chakraborty and Gu2009). All analyses were conducted in R. The lme4 package was used to run the multilevel models. Time points were nested within participants and a random participant-level intercept was included in the model. Randomized group, time, and their two-way interaction effect were included as fixed effects. Models were adjusted for baseline differences, if any, in demographic variables and comorbidity. A p value of <0.05 and 95% confidence interval were employed for main effects and interactions. Model fit was evaluated by the likelihood ratio test (LRT). Post-hoc t tests were performed to examine the between and within-group score differences. Sensitivity analyses were performed, including the adjusted models with baseline differences in treatment expectancy, per-protocol analyses on those who accomplished a satisfactory number of modules, and a selection of subgroup on participants who were recruited during the COVID-19 as secondary analyses to check the robustness of the results. Post-hoc subgroup analyses were also performed on whether having a comorbid anxiety disorder would modify the treatment effect.
Results
Demographic information
Participant recruitment started in March 2019 and closed in March 2020. The trial began in May 2019 and ended in August 2020, after the expiration date for the last participant to complete the week 12 assessment. A total of 320 eligible participants (73% female; M age = 27.3, s.d.age = 7.2) passed the two stages of screening, completed the baseline assessment, and enrolled in the clinical trial. All participants completed high school, and 90% had attained some university education (Table 1). There were significant differences between the two groups in marital status (χ2 = 6.33, p = 0.012) and gender distribution (χ2 = 9.93, p = 0.002); both variables were included in all analyses as covariates. There were no significant differences between the groups in education background, occupation, clinical comorbidities, and age.
Table 1. Demographic background and individual characteristics at baseline (N = 322)
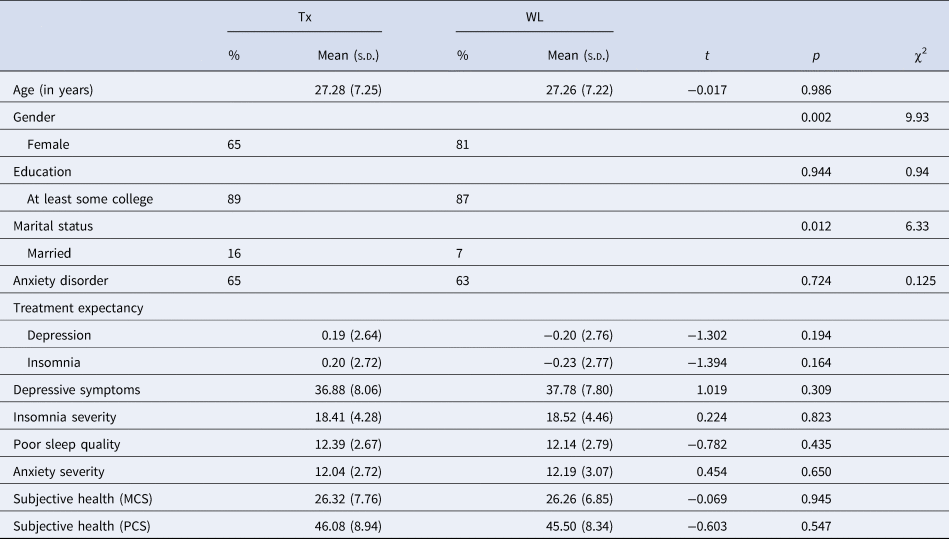
Note. Tx, treatment group; WL, waitlist control group. Depression severity was measured by the Center for Epidemiologic Studies Depression Scale. Insomnia severity was measured by the Insomnia Severity Index. Sleep quality was measured by the Pittsburgh Sleep Quality Index. Anxiety severity was measured by the Hospital Anxiety and Depression Scale – Anxiety subscale. Subjective health was measured by the SF-12 Version 1 (PCS, physical component score; MCS, mental component score).
Among the eligible participants, 52% (n = 167, 65% female; M age = 27.3, s.d.age = 7.3) were randomized to the treatment group and 48% (n = 153, 81% female; M age = 27.3, s.d.age = 7.2) to the waitlist control group.
Preliminary analyses
No significant baseline differences were found between the groups on any outcome measures (Table 1). The intra-class correlations in the unconditional models ranged from 0.26 to 0.58, suggesting it was appropriate to conduct multilevel models for the outcome measures. Both the adjusted and unadjusted models gave comparable results on the treatment effects, the results of the former are presented. Multilevel modeling results and the corresponding LRTs for both primary and secondary outcomes are presented in Tables 2 and 3.
Table 2. Fixed effects and random effects estimates for multilevel models of predictors of outcome variables

Note. Male was the reference group for gender. Marital status was a binary variable, i.e. married v. not; single/no longer in marriage was the reference group. Treatment condition referred to the randomized group the participants were assigned to; waitlist control group was the reference group.
Table 3. Likelihood ratio tests between multilevel models for outcome variables

Note. AIC, Akaike information criterion; BIC, Bayesian information criterion.
Primary outcomes
Depressive symptoms
In the multilevel model predicting depression symptoms, fixed effects for gender, marital status, and treatment condition were not significant. The week 6 follow-up time point [B = −2.39, s.e. = 0.72, p < 0.001, 95% CI (−3.81 to −0.99)] and the week 12 follow-up time point [B = −9.28, s.e. = 0.79, p < 0.001, 95% CI (−10.83 to −7.73)] showed significant negative associations with depression symptoms. Consistent with our hypothesis, the group by week 6 follow-up interaction showed significant association with depression symptoms B = −6.72, s.e. = 1.07, p < 0.001, 95% CI (−8.82 to −4.62) (Fig. 2). Follow-up simple effect analysis indicated that the treatment effect was significant at week 6 follow-up, B = −7.73, s.e. = 1.11, p < 0.001, 95% Cl (−9.91 to −5.55).

Fig. 2. Marginal effects of treatment effect between treatment and control groups across time points. Note. Figure 2 displays the marginal effects in five main models. The plots accounted for the random effects in the multilevel models. The lower the score in depressive symptoms, insomnia severity, sleep quality, and anxiety severity, the better the condition of the participants. The higher the score in subjective health, the better the health condition of the participants. BL, baseline; 6 W FU, 6-week follow-up; 12 W FU, 12-week follow-up.
Between-group comparison at week 6 follow-up was significant, in favor of the treatment group, t(188) = −6.44, p < 0.001, Cohen's d = 0.86, 95% Cl (−10.11 to −5.37). In the within-group score comparison, the treatment group corresponded to a large effect before v. immediately after the treatment, t(107) = 9.66, p < 0.001, Cohen's d = 0.93, 95% Cl (7.18–10.89). The control group corresponded to a small effect before v. after the 6-week wait time, t(137) = 4.64, p < 0.001, Cohen's d = 0.40, 95% Cl (1.36–3.38) (Table 4).
Table 4. Means, standard deviations, and post-hoc t tests across treatment group and control group and time

Note. Tx, treatment group; WL, waitlist control group. The treatment group received self-help CBT-I treatment after baseline assessment and completed week 6 follow-up assessment after being given access to the CBT-I treatment content. The waitlist control group had to wait 6 weeks upon the completion of baseline assessment and received self-help CBT-I treatment after the completion of week 6 follow-up assessment. By week 12 follow-up assessment, both groups had been given access to the self-help CBT-I treatment.
Insomnia
In the multilevel model predicting insomnia severity, fixed effects for gender, marital status, and treatment condition were not significant. Both the week 6 follow-up time point [B = −1.58, s.e. = 0.44, p < 0.001, 95% CI (−2.44 to −0.71)] and week 12 follow-up time point [B = −6.01, s.e. = 0.49, p < 0.001, 95% CI (−6.96 to −5.06)] showed significant associations with insomnia severity. Consistent with our hypothesis, the treatment condition by week 6 follow-up interaction showed a significant association with insomnia severity, B = −4.28, s.e. = 0.66, p < 0.001, 95% CI (−5.58 to −2.99) (Fig. 2). Follow-up simple effect analysis indicated that the treatment effect was significant at week 6 follow-up, B = −4.52, s.e. = 0.62, p < 0.001, 95% Cl (−5.74 to −3.30).
Between-group comparison at week 6 follow-up was significant, in favor of the treatment group, t(227) = −7.75, p < 0.001, Cohen's d = 1.00, 95% Cl (−5.93 to −3.53). Both the treatment [t(101) = 10.41, p < 0.001, Cohen's d = 1.03, 95% Cl (4.40–6.47)] and control [t(137) = 4.49, p < 0.001, Cohen's d = 0.38, 95% Cl (0.91–2.35)] groups showed a significant drop in the insomnia severity at the week 6 follow-up; the control group corresponded to a small effect size in the change, while the treatment group yielded a large effect size in the reduction of insomnia (Table 4).
Sleep quality
In the multilevel model predicting sleep quality, fixed effects for gender, marital status, and treatment condition were not significant; while the week 6 follow-up [B = −0.74, s.e. = 0.29, p = 0.011, 95% CI (−1.31 to −0.17)] and the week 12 follow-up [B = −2.80, s.e. = 0.32, p < 0.001, 95% CI (−3.42 to −2.17)] showed significant associations with sleep quality. The treatment condition by week 6 follow-up interaction yielded a significant association on sleep quality, B = −2.71, s.e. = 0.43, p < 0.001, 95% CI (−3.56 to −1.87). Follow-up simple effect analysis showed that the treatment effect was significant at week 6 follow-up, B = −2.42, s.e. = 0.40, p < 0.001, 95% Cl (−3.21 to −1.63) (Fig. 2).
Between-group comparison at week 6 follow-up was significant, in favor of the treatment group, t(206) = −6.74, p < 0.001, Cohen's d = 0.91, 95% Cl (−3.34 to −1.83). In the within-group comparison, the treatment group showed a large difference before and after the treatment, t(87) = 8.68, p < 0.001, Cohen's d = 0.93, 95% Cl (2.42–3.85). The change in the control group corresponded to a small effect size, t(116) = 2.41, p = 0.018, Cohen's d = 0.22, 95% Cl (0.10–1.04) (Table 4).
Secondary outcomes
Anxiety symptoms
In the multilevel model predicting anxiety severity, fixed effects for gender, marital status, treatment condition, and the week 6 follow-up time point were not significant. The week 12 follow-up time point showed a significant association with anxiety symptoms, B = −2.25, s.e. = 0.32, p < 0.001, 95% CI (−2.88 to −1.63). Treatment condition by week 6 follow-up interaction yielded a significant association with anxiety symptoms, B = −2.77, s.e. = 0.43, p < 0.001, 95% CI (−3.62 to −1.92). Follow-up simple effect analysis found that the treatment effect was significant at week 6 follow-up, B = −2.94, s.e. = 0.41, p < 0.001, 95% Cl (−3.74 to −2.13) (Fig. 2).
Between-group comparison at week 6 follow-up was significant, in favor of the treatment group, t(209) = −6.30, p < 0.001, Cohen's d = 0.83, 95% Cl (−3.75 to −1.96). In the within-group comparison, the treatment group showed medium-to-large difference before and after the treatment, t(107) = 6.69, p < 0.001, Cohen's d = 0.64, 95% Cl (1.71–3.16), while the control group showed no significant change (Table 4).
Subjective health
In the multilevel model predicting subjective physical health, fixed effects for gender, marital status, treatment condition, and the time points were not significant. Neither treatment group-by-time interaction terms were significant (all ps > 0.05; Fig. 2). Post-hoc t tests found no between and within-group differences across the follow-up time points on subjective physical health. Results on subjective mental health are consistent with the findings on other mental health measures reported above (see Supplementary material).
Clinical significance
Depression
Adopting a cut-off score at 20 or above (Vilagut, Forero, Barbaglia, & Alonso, Reference Vilagut, Forero, Barbaglia and Alonso2016), the percentage of participants in the treatment group scoring above the clinical threshold following the intervention decreased from 98% at baseline to 81% at the week 6 follow-up. The control group had a 1% decrease, from 99% at baseline to 98% at the week 6 follow-up. At the week 12 follow-up, the percentage in the treatment group scoring above the threshold sustained at 81% and the percentage in the control group reduced to 79% (Table 5).
Table 5. Percentages of participants with outcome scores above the clinical threshold across conditions and time

***p < 0.001.
Note. Tx, treatment group; WL, waitlist control group. The treatment group received the treatment after baseline assessment and completed week 6 follow-up assessment after being given access to the treatment content. The waitlist control group had to wait 6 weeks upon the completion of baseline assessment and received the treatment after the completion of week 6 follow-up assessment. By week 12 follow-up assessment, both groups had been given access to the treatment. Center for Epidemiologic Studies Depression Scale used cut-off score of ⩾20. Insomnia Severity Index used cut-off score of ⩾11. Pittsburgh Sleep Quality Index used cut-off score of >5. Hospital Anxiety and Depression Scale – Anxiety subscale used cut-off score of >10. For the anxiety severity, participants who endorse moderate and severe anxiety symptoms were grouped as ‘beyond clinical threshold’.
Insomnia
With a cut-off score at 11 or above (Morin, Belleville, Bélanger, & Ivers, Reference Morin, Belleville, Bélanger and Ivers2011), the percentage of participants in the treatment group meeting the clinical threshold decreased from 96% to 61% at the week 6 follow-up, while the control group found a decrease from 96% to 88%. At the week 12 follow-up, participants scoring above the threshold dropped from 61% to 52%. The control group saw a drop to 60% at the week 12 follow-up, after the given treatment (Table 5). We reported the results using 8 (i.e. in remission) as the cut-off score in online Supplementary Table S3.
Sleep quality
Using the cut-off score at above five (Buysse et al., Reference Buysse, Reynolds, Monk, Berman and Kupfer1989), the percentage of participants in the treatment group scoring above the poor sleep quality threshold decreased from 100% to 87%. The waitlist control group had a 1% decrease, from 100% at baseline to 99% at the week 6 follow-up. At the week 12 follow-up, the treatment group showed the maintenance of treatment effect with 87% scoring above the threshold. Among the control group, 84% scored above the threshold (Table 5).
Anxiety
Using the cut-off scores of 11–15 and 16–21 for moderate and severe anxiety symptoms, respectively (Snaith & Zigmond, Reference Snaith and Zigmond1994), the percentages of participants in the treatment group scoring above the clinical thresholds dropped from 61% at baseline to 32% for moderate anxiety symptoms, and from 11% at baseline to 5% for severe anxiety symptoms, at the week 6 follow up. The control group saw no improvement in the percentages of participants scoring above the threshold; those with moderate anxiety symptoms increased from 55% at baseline to 57% at the week 6 follow-up, and those with severe anxiety symptoms remained at 15% in both assessments. At the week 12 follow-up, the clinical threshold percentages in the treatment group showed the maintenance of the treatment effect, where 31% had moderate anxiety symptoms and 5% had severe anxiety symptoms. The waitlist control group showed a reduction in anxiety severity after the given treatment, where the percentages of participants with moderate and severe anxiety symptoms dropped from 57% at the week 6 follow-up to 37% at the week 12 follow-up and from 15% at the week 6 follow-up to 4% at the week 12 follow-up, respectively (Table 5).
Dropout
Dropout varied from 1.2% to 37.7% at different time points (Fig. 1). The total dropout for the treatment and waitlist control groups was 37.7% and 26.8%, respectively.
Acceptability and usability
Over half of the participants found proACT-S easy to use. Nearly 90% of the participants found proACT-S clear and easy to understand. Over 60% of the participants were satisfied with the experience using proACT-S and nearly 70% would like to continue to use proACT-S if it was available. Half of the participants found it helpful in managing their symptoms. Taking a more conservative view, if we included all dropout participants as perceiving a negative view toward proACT-S, the perception of ‘ease of use’ was 50% or above. By-item ratings are reported in online Supplementary Table S4.
Sensitivity analyses
First, to test whether treatment expectancy affected the primary outcomes of the study, adjusted models with the inclusion of treatment expectancy as a covariate were conducted. The results were similar to those reported above (online Supplementary Table S5). Second, the per-protocol analyses were performed, in which the reported multilevel models were conducted with a subsample that excluded those who did not complete at least five of the six treatment modules. The per-protocol analyses gave identical significant results (online Supplementary Table S6). Third, a subgroup of participants who started the study during the COVID-19 (52%), i.e. from February 2020 onwards, were included in another set of multilevel model analyses to check the intervention effects. Similar results were found (online Supplementary Table S7). Subgroup analyses on participants having comorbid anxiety disorder found a similar significant interaction effect between treatment conditions and time. In the model of anxiety severity, having an anxiety disorder was not a factor impacting the treatment (online Supplementary Table S8). Baseline severity of depression and insomnia were included along with other baseline characteristics in simple regression analyses to observe the impacts on the maintenance of treatment effects. The initial treatment expectation on depression alleviation, gender, or having comorbid anxiety disorder could likely be a factor impacting the maintenance of the treatment effects (online Supplementary Table S9).
Harms
No adverse events, increased suicidal risk, nor significant deterioration in primary outcome measures was reported throughout the study.
Discussion
This RCT demonstrated the efficacy of a 6-week, non-assisted, self-help smartphone-based CBT-I in adults with both depression and insomnia. The effect was sustained at 6 weeks post-intervention. The treatment group demonstrated greater reductions in depressive, insomnia, and anxiety symptoms, as well as greater improvements in sleep quality at follow-up compared to the waitlist control group. A higher proportion of participants in the treatment group no longer met clinical cut-offs for depression, insomnia, and anxiety at post-treatment compared to the control group. Sensitivity analysis revealed that participants with and without anxiety reported similar treatment effects at week 6 and week 12 follow-ups. Similar improvements were seen in the control group after they were given the treatment.
This study added to the literature to show that smartphone-based self-help CBT-I can be efficacious in treating depression and that the treatment effect can be sustained to at least 6 weeks after the end of treatment. The effect size in improvement in depression was large and comparable to the previous results of CBT-I (Feng et al., Reference Feng, Han, Li, Geng and Miao2020). While several studies have examined the efficacy of fully automated iCBT-I on depressive symptoms (Christensen et al., Reference Christensen, Batterham, Gosling, Ritterband, Griffiths, Thorndike and Mackinnon2016; Glozier et al., Reference Glozier, Christensen, Griffiths, Hickie, Naismith, Biddle and Ritterband2019), ours is one of the first to test a fully automated smartphone app CBT-I on those with comorbid depression and insomnia.
The results should be interpreted in the context of the strengths and limitations of unguided self-help treatments, which, according to a network meta-analysis of the effect of CBT-D, is more efficacious than waitlist but less so than treatment modalities that have some input from a therapist (Cuijpers, Noma, Karyotaki, Cipriani, & Furukawa, Reference Cuijpers, Noma, Karyotaki, Cipriani and Furukawa2019). The low-intensity nature of the treatment allows unguided self-help treatments to be readily disseminated, but, as our remission rate and adherence results suggest, it is far from being universally effective or helpful. As those suffering from depression would likely benefit from at least some forms of interactions with professionals (Newman, Szkodny, Llera, & Przeworski, Reference Newman, Szkodny, Llera and Przeworski2011), we speculate that, if resources permit, augmenting the current treatment with therapist support would further improve outcomes.
Not surprisingly, post-treatment improvements in sleep symptoms were observed. The effect sizes of the improvement in insomnia and sleep quality are similar to those reported in previous studies (Feng et al., Reference Feng, Han, Li, Geng and Miao2020; Horsch et al., Reference Horsch, Lancee, Griffioen-Both, Spruit, Fitrianie, Neerincx and Brinkman2017). In our treatment, sleep restriction was introduced at week 4, which is late than other iCBT-Is that reported the sequence of treatment components (Krieger et al., Reference Krieger, Urech, Duss, Blättler, Schmitt, Gast and Berger2019; van der Zweerde, van Straten, Effting, Kyle, & Lancee, Reference van der Zweerde, van Straten, Effting, Kyle and Lancee2019). The effects of sleep restriction on sleep parameters would likely be more pronounced with time, especially if the recommendations are followed through. The adjusted sleep time would be reduced during sleep restriction, which explains the observed improvement in sleep efficiency at the follow-up assessments (reported in online Supplementary Table S10).
The findings of our secondary outcomes were largely consistent with previous studies of iCBT-I for anxiety (Ye et al., Reference Ye, Zhang, Chen, Liu, Li, Liu and Jiang2015). Our results suggest CBT-I can also help alleviate anxiety symptoms among those with depression and insomnia. Half of the participants' anxiety symptoms had reduced to mild to normal levels after the treatment and this improvement was sustained at the week 12 follow-up. The cognitive restructuring elements in our self-help treatment might have acted as an aid to alleviate anxiety symptoms, and hence helped to improve sleep. Further studies are needed to elucidate the relationship between anxiety symptoms and sleep improvement in self-help treatments.
Our participants saw improvement in subjective mental health but not physical health at the end of the 6-week intervention. The low-intensity treatment might not be sufficiently robust to bring changes in subjective physical health. This is consistent with previous RCTs reporting that CBT-I failed to demonstrate significant between-group improvement in physical health during the study period (Chakravorty et al., Reference Chakravorty, Morales, Arnedt, Perlis, Oslin, Findley and Kranzler2019; Espie et al., Reference Espie, Emsley, Kyle, Gordon, Drake, Siriwardena and Luik2019; Siebmanns et al., Reference Siebmanns, Johansson, Ulander, Johansson, Andersson and Broström2021; Vedaa et al., Reference Vedaa, Kallestad, Scott, Smith, Pallesen, Morken and Sivertsen2020). However, better sleep is generally associated with better daytime functioning and subjective well-being (Chan, Poon, Leung, Lau, & Lau, Reference Chan, Poon, Leung, Lau and Lau2018; Weinberg, Noble, & Hammond, Reference Weinberg, Noble and Hammond2016). It is plausible that if the participants continued to see improvements in their sleep and mental health, their subjective physical health may enhance.
The improvement in anxiety and subjective mental health is particularly noteworthy at the time of the COVID-19 pandemic. The overall acceptability and receptiveness of proACT-S were positive. These figures are similar to previous smartphone-based studies (Rost et al., Reference Rost, Stein, Löbner, Kersting, Luck-Sikorski and Riedel-Heller2017).
Taken together, proACT-S demonstrated efficacy in reducing depressive and insomnia symptoms without any human input. Balancing the need to reduce cost and to improve retention as well as to boost the treatment effect, future advancement of similar self-help treatments might consider including simple and automated means of providing users with reminders, feedback, and encouragement, such as using in-app notifications.
Implications
In many countries, the mental health burden overwhelms existing healthcare services. COVID-19 further widened the gap between the demand and supply of mental health services (Campion et al., Reference Campion, Javed, Sartorius and Marmot2020). Efficacious psychological treatments are available, but their accessibility is restricted by their resource-demanding nature, unaffordability, preference for self-reliance and alternative forms of support, and stigma toward mental health issues (Chung, Tse, Lee, Wong, & Chan, Reference Chung, Tse, Lee, Wong and Chan2019; Corrigan et al., Reference Corrigan, Druss and Perlick2014; Hospital Authority, 2019; Shi et al., Reference Shi, Shen, Wang and Hall2020). Furthermore, Kazlauskas, Eimontas, Olff, Zelviene, and Andersson (Reference Kazlauskas, Eimontas, Olff, Zelviene and Andersson2020) indicated that populations with relatively high stigma toward mental health tend to have higher adherence to self-help intervention and receive greater benefits from low-intensity and highly accessible treatments, such as Internet-based ones. Considering the relative imbalance between the demand and supply of mental health services, the relatively high degree of mental health stigma in Chinese communities, and the preference for self-reliant (Shi et al., Reference Shi, Shen, Wang and Hall2020), proACT-S and other app-based self-help treatments might be able to help overcome some of the cultural barriers to access mental healthcare among Chinese-speakers. The study adds to the literature to demonstrate the efficacy of an app in Chinese, which can potentially provide support to a large group of patients.
proACT-S, like other CBT-I treatments, focuses on sleep but its efficacy was observable in depressive and anxiety symptoms. The bidirectionality of impact between sleep and mental health, especially the fact that sleep disturbance predisposes individuals to common mental disorders (Franzen & Buysse, Reference Franzen and Buysse2008; Riemann, Reference Riemann2007; Vargas & Perlis, Reference Vargas and Perlis2020), suggests that sleep-related intervention may be one of the means to promote psychological well-being. The sequence of improvement in depressive symptoms in response to transdiagnostic approaches such as sleep intervention is under ongoing investigation. There is evidence to suggest that sleep improvement established through CBT-I has a positive effect on the overall severity of depression (Cunningham & Shapiro, Reference Cunningham and Shapiro2018). CBT is also believed to facilitate changes in information processing to ameliorate vegetative and cognitive symptoms of depression that, in turn, would contribute to the improvement of other depressive symptoms (Bhar et al., Reference Bhar, Gelfand, Schmid, Gallop, DeRubeis, Hollon and Beck2008). An RCT examining the efficacy of guided online CBT-I on depression reported promising effects on reducing preservative thinking (van der Zweerde et al., Reference van der Zweerde, van Straten, Effting, Kyle and Lancee2019), further suggesting the underlying mechanism of the influence of improved sleep. The specific contributions of different sleep outcomes impacting daytime functioning and particular depressive symptoms warrant further investigation (Wong et al., Reference Wong, Lau, Wan, Cheung, Hui and Mok2013).
Our study adds to the growing call for using sleep interventions, such as CBT-I, as a first-line treatment for common mental disorders (Blom et al., Reference Blom, Jernelöv, Rück, Lindefors and Kaldo2017; Hagatun et al., Reference Hagatun, Vedaa, Harvey, Nordgreen, Smith, Pallesen and Sivertsen2018; Ho et al., Reference Ho, Chung, Yeung, Ng and Cheng2014; Thorndike et al., Reference Thorndike, Ritterband, Gonder-Frederick, Lord, Ingersoll and Morin2013; van der Zweerde et al., Reference van der Zweerde, van Straten, Effting, Kyle and Lancee2019). Smartphone-based treatments have lower barriers even compared with Internet-based treatments (Wilhelm et al., Reference Wilhelm, Weingarden, Ladis, Braddick, Shin and Jacobson2020). Our results suggest that proACT-S is not only efficacious but also well-received by some of its users. Rather than replacing the professional mental health services, proACT-S can be considered in conjunction with intensive treatment delivery by mental health professionals. It can, for example, be incorporated into a stepped care service delivery model as an early step in the treatment of common mental health problems (Ho et al., Reference Ho, Yeung, Ng and Chan2016).
Limitations
The current study has several limitations. First, the study participants were mostly young and well-educated. The findings have limited generalizability to the older and less educated population. To increasing the usability of proACT-S to those who are older or less-educated, further refinement of the app can be done, for example, by simplifying the contents and consider user feedback to further enhance the user experience.
Second, the study did not include a sleep diary as an outcome measure. Sleep diary could provide more precise and less memory-dependent sleep data than self-reported questionnaires (Ibáñez, Silva, & Cauli, Reference Ibáñez, Silva and Cauli2018).
Third, beyond the baseline assessment stage, our study relied on self-report measures. Future research could include actigraphy or polysomnography for observing the changes in objective sleep parameters (Ibáñez et al., Reference Ibáñez, Silva and Cauli2018) and conduct a follow-up clinical interview.
Fourth, the efficacy of the treatment was compared against a waitlist control group, as opposed to an active control group. Treatment studies with active control groups tend to show more modest effect sizes (e.g. Firth et al., Reference Firth, Torous, Nicholas, Carney, Pratap, Rosenbaum and Sarris2017a, Reference Firth, Torous, Nicholas, Carney, Rosenbaum and Sarrisb). The use of a waitlist or no treatment condition as the control group is common in RCTs of self-help CBT-I (Ho et al., Reference Ho, Chan, Lo and Leung2020). Future studies may include an inactive treatment, such as the monitoring of sleep using a sleep diary, in the control condition to enhance the validity of the evidence.
Fifth, while the treatment gains remained apparent at 6 weeks post-treatment, the lack of a longer-term follow-up limited our confidence in whether the treatment effect would sustain over time. van der Zweerde et al. (Reference van der Zweerde, van Straten, Effting, Kyle and Lancee2019) reported that the positive effect of guided online CBT-I on depressive symptoms was maintained at a 6-month follow-up. Similarly, a study reported that participants continued to see treatment gain from iCBT-I on reduced depressive symptoms at 6-month, 1-year, and 3-year follow-up (Blom et al., Reference Blom, Jernelöv, Rück, Lindefors and Kaldo2017). Further work is needed to see proACT-S can also stand the test of time.
Last, this study had a high attrition rate; the figures are not incomparable with those reported in previous RCTs of smartphone-based intervention (mean attrition of 24.1% at short-term follow-up, Linardon & Fuller-Tyszkiewicz, Reference Linardon and Fuller-Tyszkiewicz2020; mean attrition = 47.8% adjusted for publication bias, Torous, Lipschitz, Ng, & Firth, Reference Torous, Lipschitz, Ng and Firth2020). Several factors were identified to associate with a lower dropout rate in clinical trials of smartphone-based intervention, including integrating mood monitoring, providing monetary incentives, use of online enrollment, and provide human feedback/practice reminders (Torous et al., Reference Torous, Lipschitz, Ng and Firth2020). In the current study, we included a monetary incentive to attract and retain participants; this may hinder the generalizability of the findings as the incentive may have had an impact on adherence to the treatment and completion of outcome assessment.
Conclusion
This two-arm RCT study demonstrates the efficacy of proACT-S, an unguided smartphone-based CBT-I for depression. The transdiagnostic approach of sleep intervention for depression can help tackle the existing structural and cultural barriers of mental health needs. Because of its low cost, high accessibility, and minimal therapist involvement, we recommend considering a smartphone-based CBT-I such as proACT-S as a first-step intervention that can be disseminated at the community level to enhance the coverage of mental health services.
Supplementary material
The supplementary material for this article can be found at https://doi.org/10.1017/S0033291721003421.
Data
The data that support the findings of this study are available on request from the corresponding author, CSC.
Author contributions
CSC and FH formulated the research questions; CSC designed the study; CW and VH collected the data; CW analyzed the data, and CSC, CW, BY, VH, FH, and PC wrote the article.
Financial support
This work was supported by the Azalea (1972) Endowment Fund and the Seed Fund for Translational and Applied Research, The University of Hong Kong. The funders had no role in the study.
Conflict of interest
None.