Poor dietary intakes, such as low intakes of fruits, vegetables, nuts, seeds and seafood, was a leading risk factor for ill health in the 2015 Global Burden of Disease study( Reference Forouzanfar, Afshin and Alexander 1 ). Dietary patterns, as indicated by the assessment of intakes of key food groups and nutrients, can provide useful information on disease risk. For instance, indices for measuring dietary patterns such as the Alternative Healthy Eating Index-2010 (AHEI-2010)( Reference Chiuve, Fung and Rimm 2 ), the alternate Mediterranean Diet (aMed)( Reference Fung, Hu and McCullough 3 ) and the Dietary Approaches to Stop Hypertension (DASH) diet( Reference Fung, Chiuve and McCullough 4 ) have been associated with a lower risk of obesity( Reference Maskarinec, Novotny and Tasaki 5 , Reference Soltani, Shirani and Chitsazi 6 ), hypertension( Reference Appel, Moore and Obarzanek 7 ), diabetes( Reference Chiuve, Fung and Rimm 2 , Reference Nettleton, Steffen and Ni 8 , Reference Odegaard, Koh and Butler 9 ), CVD( Reference Fung, Chiuve and McCullough 4 , Reference Heidemann, Schulze and Franco 10 , Reference Estruch, Ros and Salas-Salvadó 11 ) and cancer( Reference Fung, Hu and McCullough 3 ). Although most studies have been conducted in Western populations, the few studies in Asia have reported similar findings. For example, in Japan and South Korea, traditional dietary patterns characterized by intakes of fruits, vegetables, potatoes, legumes and seaweed were inversely associated with hypertension( Reference Sadakane, Tsutsumi and Gotoh 12 , Reference Shin, Cho and Lee 13 ). In China, higher AHEI-2010 and DASH scores predicted lower mortality from CVD and diabetes( Reference Yu, Zhang and Xiang 14 ). In Singapore residents, higher AHEI-2010 scores have been associated with lower risk of myocardial infarction( Reference Neelakantan, Naidoo and Koh 15 ) and hip fracture( Reference Dai, Butler and van Dam 16 ), and a vegetable–fruit–soya dietary pattern has been associated with lower diabetes risk( Reference Odegaard, Koh and Butler 9 ) and all-cause mortality( Reference Odegaard, Koh and Yuan 17 ). Dietary patterns and quality scores are usually derived in studies comprising comprehensive dietary assessment methods( Reference Chiuve, Fung and Rimm 2 , Reference Fung, Chiuve and McCullough 4 , Reference Maskarinec, Novotny and Tasaki 5 , Reference Nettleton, Steffen and Ni 8 – Reference Heidemann, Schulze and Franco 10 , Reference Zeng, Xue and Cao 18 ) despite the need for information on intakes of only a limited number of food groups. In the absence of short, valid, population-specific screening tools, opportunities may be missed for assessing dietary quality in non-dietary studies and clinical settings. Quick and easy-to-use tools for assessing dietary quality have been developed and successfully validated against comprehensive dietary assessment methods in Europe( Reference Cleghorn, Harrison and Ransley 19 – Reference Schröder, Benitez Arciniega and Soler 24 ) and the USA( Reference Rifas-Shiman, Willett and Lobb 25 , Reference Mochari, Gao and Mosca 26 ), for use in clinical settings and in dietary and non-dietary studies. Such tools are lacking in Asian populations, despite the rapid rise in prevalence of diet-related chronic diseases( 27 , 28 ). The variety of traditional ethnic and international cuisines, along with availability of affordable out-of-home food, can make assessment of habitual dietary intakes in Asia challenging, particularly in urban populations. Singapore is a multi-ethnic urban Asian country (74 % Chinese, 13 % Malay, 9 % Indian, 3 % others)( 29 ) with rising chronic disease prevalence( 30 ). Dietary assessment in Singapore surveys and cohort studies has typically centred around extended FFQ aiming to comprehensively assess total diet( 31 – Reference Nang, Van Dam and Tan 33 ). Recently, we developed a short thirty-seven-item diet screener (DS)( Reference Neelakantan, Whitton and Seah 34 ).
The present study evaluates the reproducibility and validity of the short DS in assessing a priori dietary quality scores and intakes of selected foods. Results on the relative validity of the DS compared with a longer FFQ and dietary biomarkers are presented.
Methods
Diet screener development
Food groups included in international healthy eating indices (AHEI-2010, aMed and DASH) were considered for relevance to the local context( Reference Chiuve, Fung and Rimm 2 – Reference Fung, Chiuve and McCullough 4 ), as were items appearing in healthier dietary patterns derived in the Singapore population( Reference Butler, Wu and Wang 35 ), such as soya milk and soya products, coconut gravies, and wholegrain rice and noodles. However, it was noted that nutrient components of healthy eating indices were unlikely to be comprehensively assessed by the short DS. Local nutrition experts, epidemiologists, intervention programme planners and survey operation managers were also consulted. A list of thirty-seven food/beverage items was compiled, with a frequency scale including ten categories ranging from ‘never/rarely’ to ‘6 or more times per day’ and the reporting time frame of the past one year (see online supplementary material, Fig. S1). Standard serving sizes were indicated for each food/beverage item. Cognitive interviews were conducted in thirty participants of the three major ethnic groups in Singapore (ten Chinese, ten Malays, ten Indians), aged 25–75 years, recruited through newspaper advertisement or word of mouth. During these interviews participants were asked to complete the DS unassisted, which took 5–7 min. Researchers then followed a verbal probing protocol to ascertain any issues related to wording and layout.
As some healthy eating indices include a nutrient component, a nutrient database was constructed using nationally representative 24 h dietary recall data, to inform the nutrient profile of each DS food/beverage item and to inform the standard serving size in grams. The same 24 h dietary recall data were used to develop a nutrient database for the FFQ.
Validation study
The present study was part of a larger validation study that also assessed the relative validity of a longer FFQ, as previously reported( Reference Whitton, Ho and Tay 36 ). Briefly, 192 adult Singapore residents (aged 18–79 years) were recruited to take part in four study visits at their homes or a place of their convenience, over a period of approximately six months. The DS was administered twice by trained interviewers, approximately four months apart (median 3·75 months; interquartile range 3·1–4·7 months). Each time, a fasted blood sample was drawn, and filled overnight urine bottles were collected. A 163-item FFQ( Reference Neelakantan, Whitton and Seah 34 ) was administered approximately one month (median 36 d; interquartile range 33–41 d) after the second DS administration. The study methodologies, protocols and procedures were approved by National University of Singapore Institutional Review Board (reference code B-14–082).
Dietary data analysis
DS and FFQ response data were entered into in-house data entry software. Following data extraction and cleaning, intake frequencies were standardized to the number of servings per day and multiplied by standard portion sizes (in grams). Grams per day of each questionnaire item were multiplied by nutrient database values, which reflected the amount of nutrient per gram of food/beverage. Macronutrients for DS and FFQ were expressed as a percentage of total energy. AHEI-2010( Reference Chiuve, Fung and Rimm 2 ), aMed( Reference Fung, Hu and McCullough 3 ) and DASH( Reference Fung, Chiuve and McCullough 4 ) scores were calculated for both DS measurements and the mean of the two. For AHEI-2010, participants are scored proportionately between 0 and 10 corresponding to intake of each score component, with predefined cut-offs for maximum or minimum scores. For aMed, participants receive a score of 0 or 1 for each component depending on whether their intake is above or below the median intake. For DASH, for each component participants receive a score between 1 and 5 corresponding to which quintile of intake they fall in. The three scores all account for intakes of fruits, vegetables, nuts, legumes, whole grains and red meat. Additionally, AHEI-2010 and aMed account for intakes of seafood and alcohol, and fatty acid ratios; AHEI-2010 and DASH account for intake of sugar-sweetened beverages; and DASH accounts for intake of dairy. Trans-fat was not included in the AHEI-2010 score calculation as intake is very low in Singapore and not available in food composition tables. Sodium intake was not included in AHEI-2010 or DASH score calculation as it was considered that this would not be reliably assessed by either the DS or FFQ. Dietary quality scores were also calculated from the FFQ.
Biological samples
Blood and urine samples were stored at –4°C for a maximum of 24 h before processing, aliquoting into 1 ml tubes and freezing at –80°C. Blood was centrifuged to separate the plasma and ascorbic acid preservative (2 % v/v) was added to urine samples before freezing. To minimize the effect of inter-batch variation on biomarker measurements, participants’ samples were analysed pairwise in the same batch.
HPLC( Reference Lee and Ong 37 ) was used to measure plasma lipophilic antioxidants: lutein, zeaxanthin, α- and β-cryptoxanthin, α- and β-carotene, and lycopene. Plasma concentrations were quantified using photodiode array detection. The concentration of nineteen plasma fatty acids was measured using GC–MS/MS conducted on an Agilent 7890 GC system equipped with a 7001B triple quadrupole mass detector (Agilent, Santa Clara, CA, USA)( Reference Xu, Ho and Xu 38 ). Both free and esterified (TAG, phospholipids, cholesterol esters) fractions were measured. Concentration of the urinary metabolites daidzein, glycitein, genistein, equol and enterolactone were measured using HPLC (Dionex UltiMate 3000 LC system; Thermo Fisher Scientific, Waltham, MA, USA) from an established LC–MS/MS method( Reference Parker, Rybak and Pfeiffer 39 ) using phenyl C6 chromatography coupled with photodiode array and fluorometric detections. Urinary creatinine was measured using the Jaffe method (reaction with alkaline picrate using an auto-analyser). Urinary metabolite concentrations were expressed as nmol/mg creatinine. The within-day and between-day CV for all biosample measurements are detailed elsewhere( Reference Whitton, Ho and Tay 36 ).
To evaluate the relative validity of the DS in assessing PUFA intake, dairy fat intake( Reference Wolk, Vessby and Ljung 40 ) and fish intake( Reference Chung, Nettleton and Lemaitre 41 ), plasma PUFA, odd-chain SFA and EPA+DHA as a percentage of total plasma fatty acids were calculated. Mean concentrations of analytes from the two time points were used in subsequent analyses unless otherwise stated.
Statistical analysis
Continuous descriptive statistics were compared by ANOVA and categorical descriptive statistics were compared using the χ 2 test. Bonferroni post hoc tests and adjusted standardized residuals from χ 2 tests were used to ascertain between which ethnic groups differences were detected. To examine reproducibility, intraclass correlation coefficients (ICC) between the two DS measures were calculated by ANOVA. ICC>0·4 was considered to indicate fair to good reproducibility, while ICC≥0·75 was considered to indicate excellent reproducibility( Reference Rosner 42 ). Spearman’s rank-correlation coefficients (ρ) were calculated to examine the associations between the DS and FFQ dietary quality scores, with ρ>0·4 considered to indicate an acceptable association and ρ=0·5–0·7 considered to indicate a reasonably good association( Reference Willett 43 ). Proportions assigned to the same score quintile and to the same and or adjacent score quintile were calculated to evaluate agreement between the two methods. To further evaluate agreement and account for agreement occurring by chance, Cohen’s weighted kappa (κ w) with weights based on the squared distance between quintile categories was used, with κ w>0·4 indicating moderate, κ w>0·6 indicating good and κ w>0·8 indicating excellent agreement( Reference Landis and Koch 44 ). In addition, ρ were calculated to examine the associations between DS/FFQ scores and dietary biomarkers. Similarly, ρ were calculated between selected DS measures and dietary biomarkers. Carotenoid-containing supplement users (17 % of the study population) were excluded from analyses involving serum carotenoids and isoflavone-containing supplement users (also 17 %) were excluded from analyses involving urinary isoflavones, as the supplement contents could not be quantified. Partial correlations were calculated, adjusted for ethnicity, age, sex, energy intake, and additionally total fat (as a percentage of energy intake) for correlations with carotenoid biomarkers only. ICC between the two biomarker measurements were calculated in order to deattenuate all partial correlations for intra-individual variation( Reference Rosner and Willett 45 ). Analyses were conducted separately for the two DS and for the mean of the two. The statistical software package IBM SPSS Statistics version 23 was used for statistical analyses with the level of significance set at 5 %.
Results
Of the 192 participants enrolled, thirty-one (16 %) dropped out (six Chinese, eight Indians and seventeen Malays) and 161 completed the study. Table 1 shows the sociodemographic and dietary characteristics of the study participants according to ethnicity. The mean age was 44 years and equal proportions of males and females were recruited. Compared with the general population, the study population had higher levels of educational attainment but similar monthly household incomes, with a slightly larger proportion in the lower income categories (data not shown)( 46 ). Among ethnic groups, whole grain intakes and enterolactone concentrations were lowest in Malays, while oily fish intakes and plasma EPA+DHA concentrations were lowest in Indians.
Table 1 Sociodemographic characteristics, selected dietary intakes and dietary biomarkers, according to ethnicity, among Singapore residents (n 161) aged 18–79 years, in 2015/2016

$S, Singapore dollars; AHEI-2010, Alternative Healthy Eating Index-2010( Reference Chiuve, Fung and Rimm 2 ); aMed, alternate Mediterranean Diet( Reference Fung, Hu and McCullough 3 ); DASH, Dietary Approaches to Stop Hypertension( Reference Fung, Chiuve and McCullough 4 ); UCr, urinary creatinine; FA, fatty acid; ALA, α-linolenic acid.
† P value for the difference between ethnic groups (ANOVA for continuous variables and χ 2 test for categorical variables).
‡ Food and nutrient intakes, dietary pattern scores and dietary biomarker values are the mean of the two measurements.
§ Includes 100 % fruit juice.
║ Total carotenoids excluding lycopene.
¶ Significantly more Malays in the higher education category, and significantly fewer Malays in the university category, as compared with other ethnic groups (adjusted χ 2 post hoc test).
†† Statistically significant difference between Chinese and Malay groups.
‡‡ Statistically significant difference between Malay and Indian groups.
§§ Statistically significant difference between Chinese and Indian groups.
We evaluated the reproducibility of dietary quality scores and food groups assessed with the DS over 4 months. The median ICC was 0·71 for dietary quality score and 0·65 for food groups. ICC for dietary quality score ranged from 0·51 for AHEI-2010 in Malay participants to 0·85 for DASH in Indian participants (Table 2). For Chinese and Indian participants, better reproducibility was observed for dietary quality scores than for individual food groups.
Table 2 Reproducibility for repeat diet screener administrations within a 4-month interval, in the total group and according to ethnicity, among Singapore residents (n 161) aged 18–79 years, in 2015/2016

ICC, intraclass correlation coefficient; AHEI-2010, Alternative Healthy Eating Index-2010( Reference Chiuve, Fung and Rimm 2 ); aMed, alternate Mediterranean Diet( Reference Fung, Hu and McCullough 3 ); DASH, Dietary Approaches to Stop Hypertension( Reference Fung, Chiuve and McCullough 4 ).
† Food and nutrient variables were transformed using natural logarithms.
‡ Includes 100 % fruit juice.
§ No data shown for the Malay subgroup because n 1 for consumers of alcoholic beverages.
The a priori dietary quality scores derived from the DS were all significantly associated with the scores derived from the longer FFQ, with the strongest correlations observed with the DASH score (for mean DS: AHEI-2010, ρ=0·51; aMed, ρ=0·50; DASH, ρ=0·61; all P<0·05; Table 3). There was little difference in results obtained from the first and second administration of the DS.
Table 3 Correlation and agreement between a priori dietary quality scores derived from the diet screener (DS) and a 163-item FFQ among Singapore residents (n 161) aged 18–79 years, in 2015/2016
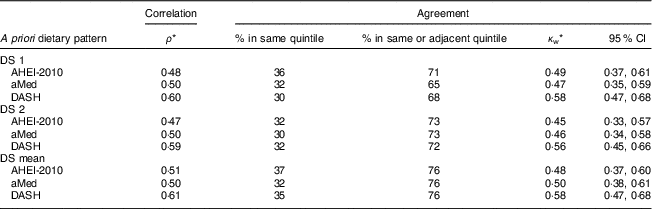
ρ, Spearman’s rank-correlation coefficient; κ w, Cohen’s weighted kappa; AHEI-2010, Alternative Healthy Eating Index-2010( Reference Chiuve, Fung and Rimm 2 ); aMed, alternate Mediterranean Diet( Reference Fung, Hu and McCullough 3 ); DASH, Dietary Approaches to Stop Hypertension( Reference Fung, Chiuve and McCullough 4 ).
* All values are statistically significant, P<0·05.
For all three of the dietary quality scores (based on the mean of two DS), 76 % of participants were accurately categorized into the same or adjacent quintile. Cohen’s κ w indicated moderate agreement between the two measures (κ w=0·48–0·58). Across ethnic groups, more Malay participants (78–87 %) were categorized into the same or adjacent quintile, as compared with Indian (66–73 %) and Chinese (71–78 %) participants (see online supplementary material, Table S1).
All dietary quality scores were significantly positively correlated with biomarker concentrations of enterolactone, total carotenoids, PUFA and α-linolenic acid (ρ=0·17–0·30), with correlation coefficients of a similar magnitude across different dietary quality scores (Table 4). We also compared the strength of the association of the DS and the long FFQ with dietary biomarkers. Dietary quality scores based on the DS were significantly correlated with plasma carotenoids (for all scores) and plasma odd-chain SFA concentrations (for aMed and DASH), whereas these associations were not observed for dietary quality scores based on the FFQ. Associations between dietary quality scores and other biomarkers were of a similar magnitude for the DS and FFQ.
Table 4 Correlation of a priori dietary quality scores derived from the diet screener (DS) and the long FFQ with biomarker concentrations among Singapore residents (n 161) aged 18–79 years, in 2015/2016

AHEI-2010, Alternative Healthy Eating Index-2010( Reference Chiuve, Fung and Rimm 2 ); aMed, alternate Mediterranean Diet( Reference Fung, Hu and McCullough 3 ); DASH, Dietary Approaches to Stop Hypertension( Reference Fung, Chiuve and McCullough 4 ); UCr, urinary creatinine; FA, fatty acid; ALA, α-linolenic acid.
* Correlation is statistically significant, P<0·05.
† Adjusted for energy intake, ethnicity, age and sex, and corrected for intra-individual variation between two biomarker measurements.
‡ For associations with isoflavones, isoflavone supplement users were excluded (DS mean, n 28; FFQ, n 17).
§ For associations with carotenoids, carotenoid supplement users were excluded (DS mean, n 27; FFQ, n 17); additionally adjusted for fat intake (% of energy).
║ Total carotenoids excluding lycopene.
We also examined the associations between intakes of key food groups (or nutrients reflecting food groups) assessed with the DS and dietary biomarkers. Significant associations were observed between soya protein intake and urinary isoflavone concentration (mean DS, ρ=0·48), whole grain intake and urinary enterolactone concentration (ρ = 0·23), fruit intake and serum β-cryptoxanthin concentration (ρ=0·23), fruit and vegetable intake and serum carotenoid concentration (ρ=0·27), oily fish intake and plasma long-chain n-3 PUFA concentration (ρ=0·31), and dairy fat intake and plasma odd-chain SFA concentration (ρ=0·30; Table 5). Apart from soya protein intake, correction for within-person variation in biomarkers did not change the observed associations substantially.
Table 5 Correlation of food and nutrient intakes from the diet screener (DS) measurements with biomarkers among Singapore residents (n 161) aged 18–79 years, in 2015/2016

Adj., adjusted; Deatt., deattenuated; UCr, urinary creatinine; FA, fatty acid.
* Correlation is statistically significant, P<0·05.
† Adjusted for energy intake, ethnicity, age and sex. Carotenoid associations additionally adjusted for total fat intake (% of energy).
‡ Adjusted for energy intake, ethnicity, age and sex, and corrected for intra-individual variation between two biomarker measurements. Carotenoid associations additionally adjusted for total fat intake (% of energy).
§ For associations with isoflavones, isoflavone supplement users were excluded (DS mean, n 28; DS1, n 20; DS2, n 19).
║ For associations with carotenoids, carotenoid supplement users were excluded (DS mean, n 27; DS1, n 20; DS2, n 20).
¶ Includes 100 % fruit juice.
†† Total carotenoids excluding lycopene.
Discussion
The thirty-seven-item DS was designed to assess intakes of selected food groups that could represent overall dietary patterns. The present study examined the relative validity of the DS by comparing estimated intakes and derived dietary quality scores with dietary biomarkers and dietary quality scores from a 163-item FFQ. Our results indicate reasonably good validity of the DS in assessing intakes of key food groups and dietary quality relative to a longer FFQ. Our results also demonstrate good reproducibility of the DS.
The relative validity of the assessment of dietary quality scores with a DS and 163-item FFQ in our study is comparable to findings in other studies( Reference van Lee, Feskens and Meijboom 22 , Reference Schröder, Benitez Arciniega and Soler 24 ). For instance, the Dutch Healthy Diet Index score calculated from a thirty-four-item screener was associated with the same score calculated from a 180-item FFQ in 1235 Dutch adults (ρ=0·56; 95 % CI 0·53, 0·60), but limits of agreement, an indicator of individual-level agreement between the two methods, were wide( Reference van Lee, Feskens and Meijboom 22 ). In a Spanish cohort study, a diet quality score calculated from an eighteen-item screener and a Mediterranean diet score calculated from a fifteen-item screener were associated with the same scores calculated from ten days of 24 h dietary recall (diet quality score, r=0·61; Mediterranean diet score, r=0·40), while agreement was considered reasonable( Reference Schröder, Benitez Arciniega and Soler 24 ). Correlation coefficients reflect the ability of the DS to rank individuals according to diet quality, which is often the primary objective in research measuring dietary exposures. However, it should be noted that correlation coefficients do not assess the accuracy of the DS in measuring absolute levels of dietary intake. Cohen’s κ w assessed the agreement in the classification in quantile categories. As a result, it also reflects the accuracy in ranking individuals based on their dietary intakes and does not evaluate the accuracy of absolute intake assessment.
In our study the strongest associations between DS dietary quality scores and FFQ dietary quality scores were observed for the DASH score. This may be related to the DASH score’s inclusion of dairy intake, which was well assessed by the DS, and exclusion of fatty acid intakes, which were poorly assessed by the DS. In fact, the DS was able to assess dairy fat intake as well as the longer FFQ in our study and longer FFQ in other studies( Reference Brevik, Veierød and Drevon 47 , Reference Sun, Ma and Campos 48 ).
Associations between dietary quality scores and biomarker concentrations in our study were slightly lower than those observed in male US health professionals (Diet Quality Index Revised score and plasma α-carotene, r=0·43, and plasma β-carotene, r=0·35)( Reference Newby, Hu and Rimm 49 ). Associations between dietary questionnaire measures and biomarker concentrations such as carotenoids and isoflavones reported in the literature tend to be lower than 0·4( Reference Willett 43 ). Associations between DS fruit and vegetable intake and carotenoid concentrations in our study were slightly lower than those observed in other studies. For example, fruit and vegetable intake assessed with a short questionnaire was more strongly correlated (ρ = 0·37) with total plasma carotenoid concentrations in a Dutch population( Reference Bogers, Van Assema and Kester 50 ). This finding may be related to the weaker association between DS vegetable intake and carotenoid concentrations in our study. In Asian settings, it may be particularly challenging for participants to estimate vegetable intakes, because vegetables are often not served discretely in meals but are typically mixed with other ingredients. Mixed dishes with small amounts of a variety of vegetables make the amounts more difficult to estimate for participants. In addition, other sources of carotenoids may be more relevant, such as spices in curries, as carotenoids are not a specific marker for fruit and vegetables. Furthermore, greater variation in cooking methods of vegetables, which can impact carotenoid bioavailability, may affect the association between vegetables and carotenoids, as may co-ingestion of bioavailability enhancers or inhibitors( Reference Willett 43 ). Finally, although the healthy eating indices used have been associated with health outcomes in Asian settings( Reference Odegaard, Koh and Butler 9 , Reference Yu, Zhang and Xiang 14 – Reference Odegaard, Koh and Yuan 17 ), suggesting they capture relevant aspects of diet quality in Asia, they were developed in Western populations and may not be the optimal measure of diet quality in Asia. DS associations with biomarkers for soya, whole grain and oily fish intakes were comparable to those observed for the longer FFQ and with other studies( Reference Chung, Nettleton and Lemaitre 41 , Reference Lee, Wen and Xiang 51 – Reference Lin, Wolk and Håkansson 54 ) that used longer questionnaires to assess intake.
The reproducibility of the DS over a 4-month interval was also assessed. Our results suggest reasonable reproducibility of food intakes, and good reproducibility of dietary patterns, in comparison with other studies. For instance, the ICC for foods in our study were slightly lower than those reported in a French study with a fourteen-item FFQ administered twice with a 15 d interval (ICC=0·77 for fruit and vegetables)( Reference Laviolle, Froger-Bompas and Guillo 20 ), possibly because the interval between administrations was longer in our study. Slightly better reproducibility was observed for assessment of dietary patterns than in other studies, such as a study in China in which two a posteriori dietary patterns were derived from a seventy-six-item FFQ administered one year apart (ICC=0·55–0·57)( Reference Liu, Wang and Lin 55 ). This suggests that a single administration of the DS is likely to be sufficient to assess dietary patterns. Poorer reproducibility was observed in Indians for oily fish, and in Malays for dairy fat. It is unclear why this dairy fat would be affected, and other items not, but may be related to observation of the fasting month during the study period since ethnic Malays are generally Muslims and changes in dietary intakes during fasting have been observed in other studies( Reference Yeoh, Zainudin and Loh 56 ).
A strength of the present study is that it is the first short dietary screening tool assessing dietary quality to be developed and validated in an Asian population. Although several studies have assessed the relative validity of short instruments in capturing dietary patterns( Reference Cleghorn, Harrison and Ransley 19 – Reference Mochari, Gao and Mosca 26 ), the authors are not aware of any such studies in Asian populations. A further strength was the inclusion of biomarkers in the study, which enabled comparison with a method free from biases common to dietary questionnaires and 24 h dietary recalls such as food composition data inaccuracies, use of standard serving sizes and participant biases related to misreporting( Reference Willett 43 ). A limitation to comparing dietary intakes and biomarkers in validation studies in the Singapore setting is the lack of comprehensive food composition data, for example on carotenoids and isoflavones. We used food groups (e.g. fruit/vegetables) or nutrients (e.g. protein from soya) as proxies for actual carotenoid or isoflavone contents of foods; this may have attenuated associations, and to a varying degree across population subgroups, because of preferences for different types of fruit for instance. A further limitation is that concentration biomarkers allow ranking only, rather than those indicating absolute intake amount such nitrogen in 24 h urine samples. Another potential limitation was that slightly more participants with higher education were included in our study as compared with the general Singapore population. However, cognitive interviews conducted as part of screener development did not indicate any differential in ability to answer the questions based on education level( Reference Neelakantan, Whitton and Seah 34 ). It should also be noted that the sample was self-selected and so may have had different, unmeasured characteristics as compared with the general population. Further, our sample size was modest, therefore analyses of validity according to ethnic group may have been underpowered.
Conclusions
The present study evaluated the relative validity and reproducibility of a thirty-seven-item DS designed to assess intakes of selected food groups that could represent overall dietary patterns in a multi-ethnic Asian population. The results showed that the dietary quality scores derived from the screener could be reasonably reproduced within a 4-month interval, and that intakes of whole grains, soya protein, fruit, vegetables, oily fish and dairy fat, but not PUFA, could be adequately assessed relative to more accepted longer FFQ. For the accurate assessment of the intake of dietary components such as nutrients, more detailed instruments such as comprehensive FFQ or repeated 24 h recalls or diet records remain the method of choice. However, with administration time of just 5–7 min, the DS may be incorporated in future epidemiological studies and health intervention programmes focused on major dietary patterns with minimal participant burden.
Acknowledgments
Financial support: This study was supported by funding from the Ministry of Education Academic Research Fund Tier 1 FRC, Singapore. The funding sponsors had no role in the design of the study; in the collection, analyses or interpretation of the data; in the writing of the manuscript; or in the decision to publish the results. Conflict of interest: The authors declare no conflict of interest. Authorship: R.M.v.D., S.A.R. and C.W. conceived of and designed the study; C.W. coordinated the fieldwork; J.C.Y.H. conducted the fieldwork and data entry; C.W. analysed the data and wrote the manuscript. All authors reviewed and approved the final manuscript. Ethics of human subject participation: This study was conducted according to the guidelines laid down in the Declaration of Helsinki and all procedures involving human subjects were approved by the National University of Singapore Institutional Review Board (reference code B-14–082). Written informed consent was obtained from all subjects.
Supplementary material
To view supplementary material for this article, please visit https://doi.org/10.1017/S1368980018001830