Introduction
The measurement of clinical outcomes in cognitive behavioural therapy (CBT) has become routine practice in the UK’s Improving Access to Psychological Therapy (IAPT) programme. IAPT services offer evidence-based interventions for patients who present with common mental health problems such as anxiety disorders and depression. Patients may either self-refer themselves to an IAPT service or be referred by their General Practitioner or another primary health care clinician. The service uses a stepped care model whereby patients are triaged by the service and allocated to either a step 2, low-intensity intervention (mild level of severity) or a step 3, high-intensity intervention (moderate to severe presentation). Step 2 interventions are provided by psychological wellbeing practitioners and step 3 interventions are provided by more highly qualified high-intensity CBT therapists. This research focuses only on cognitive behavioural treatments delivered at step 3.
In the reporting year 2016/17, 567,106 patients had completed treatment within IAPT, with recovery rates reported by individual IAPT services ranging between 18.8 and 69.4%, with an average recovery rate of 49.3% (NHS Digital, 2017). The IAPT programme has significantly improved the reporting of clinical outcome data. Access to clinical outcome data enables services, commissioners and researchers to analyse the effectiveness of the IAPT programme. Gyani et al. (Reference Gyani, Shafran, Layard and Clark2013) analysed the clinical outcome data of 19,395 patients who received at least two treatment sessions within IAPT services. They report on the predictors of clinical recovery and improvement in relation to therapist variables and methods of interventions. The authors conclude that better outcomes are achieved when services use experienced therapists who adhere to the National Institute of Health and Care Excellence (NICE) guidelines for the treatment of depression and anxiety disorders. However, Gyani et al. acknowledge that despite the large volume of data captured within IAPT, little is known about the skills and qualities of effective therapists.
Whilst IAPT services reported that therapists were adhering to NICE-approved treatment protocols, live material was not available to validate this. Shafran et al. (Reference Shafran, Clark, Fairburn, Arntz, Barlow and Ehlers2009) argue that whilst IAPT therapists are trained to deliver disorder-specific treatment protocols, they may fail to follow the evidence base due to a gap in their knowledge, a belief that the protocol is not helpful or a preference for particular parts of a protocol. Therefore, whilst the major randomised control trials (RCTs) in CBT demonstrate that adherence to disorder-specific protocols relates to good clinical outcome, the IAPT dataset cannot demonstrate whether therapists are doing this in routine clinical practice. Very little is known about whether therapists in IAPT are adhering to an evidence-based protocol or whether therapists are competent at delivering CBT with fidelity to the model. This is of particular importance as there is some evidence that both adherence and competence (i.e. delivering CBT with fidelity to the model) are positively correlated with outcome (see Branson et al., Reference Branson, Shafran and Myles2015; Ginzburg et al., Reference Ginzburg, Bohn, Hofling, Weck, Clark and Stangier2012; Liness et al., Reference Liness, Beale, Lea, Byrne, Hirsch and Clark2019).
Ginzburg et al. (Reference Ginzburg, Bohn, Hofling, Weck, Clark and Stangier2012) reported that fidelity to an evidence-based protocol for social anxiety disorder strongly correlated with good clinical outcomes. Similar studies undertaken with patients presenting with a diagnosis of depression have also suggested that adherence to a disorder-specific protocol increases the likelihood of recovery (Kuyken and Tsivrikos Reference Kuyken and Tsivrikos2009; Shaw et al., Reference Shaw, Elkin, Yamaguchi, Olmstead, Vallis, Dobson, Lowery, Sotsky, Watkins and Imber1999; Webb et al., Reference Webb, DeRubeis and Barber2010). Each of these studies examined adherence in the context of a trial investigating the efficacy of a particular disorder-specific treatment protocol. Adherence to the protocols was undertaken by raters reviewing recorded therapy sessions and rating whether the therapist was delivering the protocol in the sessions that were reviewed (Kuyken and Tsivrikos, Reference Kuyken and Tsivrikos2009; Shaw et al., Reference Shaw, Elkin, Yamaguchi, Olmstead, Vallis, Dobson, Lowery, Sotsky, Watkins and Imber1999; Webb et al., Reference Webb, DeRubeis and Barber2010). The issue of adherence to a protocol and fidelity to the CBT model has been discussed by Waller and Turner (Reference Waller and Turner2016). The authors use the phrase ‘therapist drift’ to describe when therapists drift away from fidelity to the CBT model and fail to adhere to a protocol. Other studies have sought alternative explanations in relation to which therapist variables correlate with outcome. Huppert et al. (Reference Huppert, Bufka, DeRubeis, Gorman, Shear and Woods2001) reported a positive correlation between therapist experience (the number of years a therapist had practised for) and clinical outcome. However, Huppert et al. raise a number of questions including the need to differentiate the factors that make a therapist effective or ineffective. The authors argue that understanding these factors will enhance clinical practice, enabling supervisors to support therapists to become more effective. This is particularly important given that it has been reported that around 5.8% of variance in outcomes in IAPT is due to therapist effects (Saxon et al., Reference Saxon, Firth and Barkham2017) but very little is known about which therapist variables might account for differences in clinical outcome (Lutz et al., Reference Lutz, Rubel, Schiefele, Zimmermann, Bohnke and Wittmann2015). Many of the current studies in this field are RCTs undertaken in research centres. To date, with the exception of Ewbank et al. (Reference Ewbank, Cummins, Tablan, Bateup, Catarino, Martin and Blackwell2019), there have been very few large-scale studies exploring the associations between therapist behaviour and clinical outcome in routine clinical practice. Of those that do (see Branson et al., Reference Branson, Shafran and Myles2015; Liness et al., Reference Liness, Beale, Lea, Byrne, Hirsch and Clark2019), most researchers have not had access to recordings or transcripts of all the patients that the therapist had treated. This is due to the fact that collection of live therapy data is not only costly if appended to a standard service, but actually unfeasible at scale.
The present paper aims to shed light on whether, and if so how, therapist-related variables are associated with patient outcomes by employing an advanced statistical method (hierarchical log-linear analysis with backwards elimination). This multivariate technique adjusts for patient characteristics and their presenting severity scores in depression and anxiety, and has the potential to yield revealing interdependencies among all variables. The method, although less commonly encountered in mental health applications, is neither novel nor non-standard, requires use of categorical or ordinal data, is well-suited to exploratory analyses for generating hypotheses and can yield elegant and useful pictorial representations of complex multivariate data. It does not distinguish between dependent and independent data, nor does it automatically provide inferences about the direction of influence between variables. It does, however, lead to greater in-depth understanding of inter-relationships among a set of variables (than say odds ratios, whether adjusted for baseline data through logistic regression or not).
The final model can demonstrate visually intrinsic checks on its own coherency and plausibility by noting whether expected relationships are present (e.g. clinical recovery and longer-term improvement would be expected to be associated) and unexpected ones are absent (e.g. patient’s gender should be irrelevant). Without the overall model it would not be possible to ascertain the interdependent roles of patient or therapist variables of interest. The model produced, or more exactly its simplifying diagrammatic representation, demonstrates which variables are linked to which, without quantifying the size, or assessing the directional causality of, the effect. In common with exploratory analyses it is best seen as hypothesis-generating rather than hypothesis-proving, for which an entirely different dataset would be required. However, by utilising the order of magnitude of p-value as a surrogate measure of effect size, the analysis provides an indication of whether the relationship between variables is absent, weak, moderate or strong, as well as whether direct or indirect.
Internet enabled CBT
Internet enabled CBT (IECBT) is one method of providing CBT currently deployed within the UK’s National Health Service, under the IAPT programme. This method of CBT is delivered by the company Ieso Digital Health (see www.iesohealth.com). In 2019, 1.9% of patients who completed treatment in IAPT were treated using IECBT. Lack of publicly available data meant that it was not possible to identify if there were any significant differences in age, gender, presenting problem or severity between patients who were treated using IECBT and patients who had face-to-face CBT. In IECBT, a patient communicates with a qualified CBT therapist using a real-time text-based message system, in a secure virtual therapy room. An example of a CBT session conducted using this method can be seen in Fig. 1.

Figure 1. A (fictitious) example of an internet enabled CBT session (Bateup, Reference Bateup2018).
One aspect of IECBT that is novel and unique is the availability of therapy transcripts for every therapy appointment attended. This is the first time that it has been possible to examine live therapy material, at volume, in a clinical setting. The transcript of each therapy session, conducted in this way, is encrypted and held on a secure site for both therapist and patient to access at any time. In addition to a weekly CBT appointment, therapist and patient can also communicate with each other, in between therapy appointments. This asynchronous communication can be used to amplify the effect of CBT by encouraging the patient to focus on out-of-session tasks, goals and consolidating learning that has taken place during a therapy session. The clinical efficacy of IECBT has been demonstrated by Kessler et al. (Reference Kessler, Lewis, Kaur, Wiles, King, Weich, Sharp, Araya, Hollinghurst and Peters2009). In their study, Kessler and colleagues compared the delivery of IECBT with treatment as usual in 297 participants diagnosed with major depressive disorder, demonstrating the effectiveness of IECBT, with benefits maintained over 8 months. In addition, the recovery rate in the treatment arm of the study was similar to the published recovery rates of depressed patients in previous studies using face-to-face CBT (Kessler et al., Reference Kessler, Lewis, Kaur, Wiles, King, Weich, Sharp, Araya, Hollinghurst and Peters2009). Over 25,000 patients have now completed treatment within IAPT using IECBT. The effectiveness of IECBT has since been validated in a real-world clinical setting (Catarino et al., Reference Catarino, Bateup, Tablan, Innes, Freer and Richards2018).
Method
In this study, IECBT was delivered using a commercial package provided by Ieso Digital Health (https://www.iesohealth.com/), following internationally recognised standards for information security (ISO 27001; https://www.iesohealth.com/en-gb/legal/iso-certificates). Ieso Digital Health was the setting for this study. Patients treated by Ieso Digital Health either self-referred or were referred to the service by a primary care clinician. Upon registration, patients were assigned to a qualified CBT therapist accredited by the British Association for Behavioural and Cognitive Psychotherapies (BABCP). The BABCP is the lead organization for CBT in the UK and is responsible for accrediting cognitive behavioural therapists. Using the IECBT method, therapists conducted initial assessments in an online therapy room via one-to-one, synchronous, written conversation, after which the therapist identified the primary presenting problem (see Clark, Reference Clark2018) CBT treatment protocol. Therapists delivered treatment via weekly sessions in accordance with the Roth and Pilling CBT competency framework. This framework establishes the specific CBT competencies required to deliver effective interventions for people who present with depression and anxiety disorders (Roth and Pilling, Reference Roth and Pilling2008).
A total of 474 high-intensity CBT therapists accredited by BABCP were eligble to participate in this study. These represented the therapists who were treating patients using IECBT, at the company Ieso Digital Health, and had delivered a course of treatment to at least 10 patients, using IECBT, between April 2017 and April 2018. Of the 474 eligible therapists, n = 200 (42.2%) consented to participate. The therapists had all received training in delivering CBT using the IECBT method. The sample of therapists represents 5.3% of BABCP-accredited therapists working within IAPT, as calculated using the last IAPT workforce census (NHS England, 2016). Figure 2 illustrates how the study sample is sited in the population of IAPT therapists.

Figure 2. The study sample in comparison with the population of BABCP-accredited therapists.
The n = 200 therapists who consented to participate in this study were asked to provide demographic information about themselves. The therapists were asked to provide information regarding age, gender, years of experience as an accredited cognitive behavioural therapist, core profession and type of CBT training. Whilst not mandatory, many therapists working in the IAPT programme will have undertaken CBT training on an IAPT training programme. The IAPT training programme is delivered following a national curriculum focusing on the assessment and treatment of patients who present with depression and anxiety disorders (Clark, Reference Clark2011). Table 1 shows the demographic information for the sample. Therapist variables, together with patient starting score on the PHQ-9 and GAD-7 and patient outcomes, aggregated for each therapist (recovery and improvement rates), were used as input variables to the model. Of the patients included in the model, 53.3% were equal to or younger than the median age of 33 years (mean 35.08, SD 11.92) and 46.7% were older than the median age; 73.8% were female and 26.2% were male; 54.0% scored equal to or above the median of 13.0 (mean 13.41, SD 6.18) for severity at the start of treatment on the PHQ-9, and 55.9% scored equal to or above the median of 13.0 (mean 12.82, SD 5.14) for severity, at the start of treatment on the GAD-7; 75.9% reliably improved and 54.9% recovered. Table 2 shows the frequencies for each variable in the model.
Table 1. Social and demographic characteristics of the sample
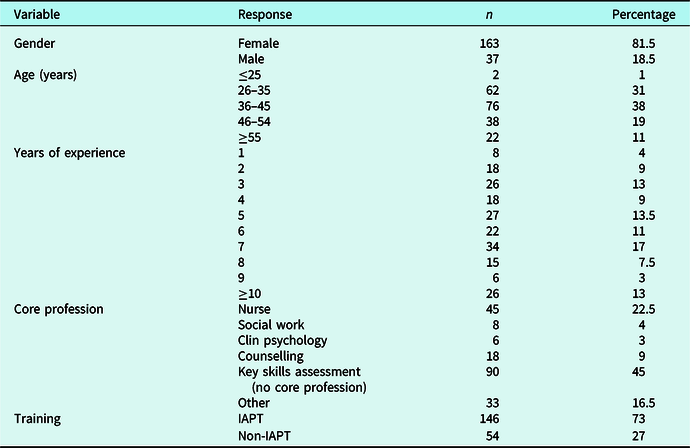
Table 2. Frequency table showing patient variables included in the model

Therapy transcripts were reviewed by six clinicians (including the first author) with the aim of assessing therapist competence using the revised version of the Cognitive Therapy Scale-Revised (CTS-R; Blackburn et al., Reference Blackburn, James, Milne, Baker, Standart, Garland and Reichelt2001) and adherence to evidence-based protocols using the Roth and Pilling (Reference Roth and Pilling2008) framework. All of the raters were BABCP accredited and had at least 12 months experience of delivering IECBT. Additionally, all the raters had previously worked as a tutor or supervisor on an IAPT training programme for at least 2 years. Inter-rater reliability training was delivered to all raters until an intraclass correlation coefficient (ICC) of ≥0.80 was achieved (95% confidence interval of an ICC estimate of 0.983 was 0.946–0.997) for rating both therapist fidelity to the CBT model and adherence to an evidence-based protocol. The 3-day inter-rater reliability training provided to the raters consisted of independent rating of 21 transcripts using the CTS-R and the review of 21 whole cases in order to rate adherence to a protocol. Raters were provided with training on the use and interpretation of the CTS-R manual and Roth and Pilling (Reference Roth and Pilling2008) framework. Raters shared their rationale for awarding scores and raters provided feedback to their peers. Following the training, raters were randomly allocated therapists to assess (three assessors were allocated 28 therapists and four were allocated 29 therapists). All raters were allocated therapists who were not known to them and were kept blind to the therapists’ clinical outcomes and demographic data. The raters were instructed to randomly select three completed cases from each of the therapist’s caseload using the following criteria:
Each case selected must consist of a complete episode of therapy, where the patient and therapist completed a piece of work together and a planned ending for therapy was agreed.
The case must have been treated at step 3 only.
The patient must have been at caseness at the beginning of therapy.
The case must have completed treatment during the time period April 2017 to April 2018.
The raters were then asked to randomly select a therapy session, from each of the three completed cases, in order to rate the therapist’s ability to deliver CBT with fidelity to the model, using the CTS-R. The CTS-R is a tool that is widely used to measure therapist fidelity to the CBT model. The tool consists of 12 items and raters assess the therapist’s adherence to each item on a 0–6 scale, where a score of 0 indicates that the item was not evident in the session being rated (Blackburn et al., Reference Blackburn, James, Milne, Baker, Standart, Garland and Reichelt2001).
The raters were asked to randomly select a session from the second, third, fourth, fifth, sixth or later treatment sessions, but not where the sixth (or later) session was the final treatment session. First and final treatment sessions tend to differ from other treatment sessions as they often contain fewer cognitive behavioural interventions and are therefore not suitable for CTS-R assessment (Blackburn et al., Reference Blackburn, James, Milne, Baker, Standart, Garland and Reichelt2001). Having completed the CTS-R on the selected session, the raters were then asked to look at the whole case, examining every transcript, all of the patient’s questionnaires and assessment notes. The raters were asked to decide whether the therapist had used an evidence-based treatment protocol that was appropriate to the patient’s primary presenting problem. The raters were asked to assess the therapist’s adherence to the protocol with reference to the Roth and Pilling (Reference Roth and Pilling2008) competency framework for disorder-specific protocols, NICE guidelines and the evidence base for third-wave cognitive behavioural interventions, such as acceptance and commitment therapy (ACT) and mindfulness-based cognitive therapy (MBCT). The raters were asked to record whether or not they found sufficient evidence that the therapist was using an evidence-based protocol by recording a mark of 0 where there was insufficient evidence, and a mark of 1 where there was evidence that a protocol had been adhered to. The raters repeated this process for the three completed cases, initially selected for each therapist, such that three entire cases had been assessed for fidelity to the model using the CTS-R and adherence to an evidence-based protocol. The resulting scores were then recorded as follows:
Fidelity to the CBT model was recorded as an ‘F’-score and was entered as the mean of the three CTS-R scores.
Adherence to an evidence-based protocol was recorded as an ‘A’-score where 4 indicated that all three cases contained evidence that the therapist delivered an evidence-based protocol, a score of 3 indicated that two out of three of the cases contained evidence of protocol, a score of 2 indicated that only one of the cases contained a protocol, and a score of 1 indicated that there was no evidence of a protocol in any of the three cases assessed.
Mean recovery rate and improvement rate were recorded for each therapist’s cohort of patients discharged between April 2017 and April 2018. Details of patient demographics (age, gender and severity at the start of treatment) were also compiled for each therapist. Therapists’ mean F-score was 40.5% (SD 11.7%, range 6–75%). The data were normally distributed with a median of 40% and mode of 40%. Table 3 shows the frequency of A-scores (adherence to an evidence-based protocol) across the sample.
Table 3. Frequency table for A-scores

Clinical outcomes
Analyses in this study explored associations between therapist variables and patients’ clinical outcomes. Clinical outcomes were calculated based on changes for PHQ-9 and GAD-7 scores, following IAPT definitions of clinical recovery and reliable improvement. The PHQ-9 and GAD-7 form part of the IAPT mandatory minimum data set and are routinely used at every appointment within all IAPT services.
GAD-7
The GAD-7 (Spitzer et al., Reference Spitzer, Kroenke, Williams and Löwe2006) is a 7-item measure for anxiety using a 4-point Likert scale (0–3, where 0 indicates the absence of a symptom and 3 indicates greater severity). A cut-off point of ≥8 indicates that the patient is a clinical case in a primary care population. A cut-off point of ≥15 indicates severe symptoms. The GAD-7 has been demonstrated to be a reliable and valid instrument with a sensitivity of 89%, a specificity of 82% and good internal consistency (Cronbach’s α = 0.92).
PHQ-9
The PHQ-9 (Kroenke et al., Reference Kroenke, Spitzer and Williams2001) is a 9-item measure for depression using a 4-point Likert scale (0–3, where 0 indicates the absence of a symptom and 3 indicates greater severity). A cut-off point of ≥10 indicates that the patient is a clinical case in a primary care population. The PHQ-9 has been demonstrated to be a valid and reliable tool with a sensitivity of 88% and specificity of 88%, when compared with mental health professional validation interviews (Kroenke et al., Reference Kroenke, Spitzer and Williams2001).
Definition of recovery
A patient is described as being recovered if they were a clinical case at the start of treatment (≥10 on PHQ-9 and/or ≥8 on GAD-7) but fell below the threshold to be considered a clinical case at the end of treatment. The calculation for recovery is based on the pre- and post-intervention scores using the PHQ-9 and GAD-7 (NHS Digital, 2018).
Definition of reliable improvement
A patient is described as being reliably improved if they were a clinical case (≥10 on PHQ-9 and/or ≥8 on GAD-7) at the start of therapy and their post-intervention scores fell ≥ the measurement error of the questionnaire, i.e. 6 on the PHQ-9 and/or 4 on the GAD-7 (NHS Digital, 2018).
Patients
Data from patients discharged between April 2017 and April 2018, having received a course of CBT at step 3, were included in this study. The following IAPT inclusion and exclusion criteria were applied for patients treated at step 3.
IAPT inclusion criteria
All patients aged 18+ who present with a common mental health disorder such as (but not exclusive to): anxiety, depression, post-traumatic stress disorder, obsessive compulsive disorder, generalised anxiety disorder, agoraphobia, panic disorder, specific phobias, health anxiety, unexplained medical symptoms and long-term medical conditions.
IAPT exclusion criteria
Patients who are or who become actively suicidal or present as a risk to others.
Patients who are experiencing symptoms of psychosis, hypermania, severe cognitive impairment, severe personality disorder or severe learning disability.
Patients who drop out of treatment.
In addition to the routine IAPT exclusion criteria, Ieso Digital Health operate the following exclusion criteria:
Patients who do not have access to an internet enabled device or an internet connection.
Patients who have a low level of literacy, i.e. patients who cannot write or read emails or texts.
Patients who are visually impaired and are unable to write on or read from a computer and do not have access to appropriate assistive technology for the visually impaired.
Patients who do not speak English.
Patients who become unsuitable for treatment within an IAPT service, for example a patient who becomes actively suicidal during treatment.
Patients meeting the exclusion criteria were referred on to more specialised or secondary care services.
Treatment provided by the therapists
The therapists provided CBT following the disorder-specific protocols used as standard within an IAPT CBT service. All IAPT services are required to deliver evidence-based disorder-specific interventions for depression and anxiety disorders. These follow NICE guidelines and the Roth and Pilling (Reference Roth and Pilling2008) framework, and form part of the clinical training for the IAPT training programme for cognitive behavioural therapists. Individual therapists may select any appropriate treatment protocol when working with a patient. This study did not dictate which treatment protocol the therapists selected, as this would interfere with normal service delivery and is beyond the scope of this trial. The number of treatment sessions offered to each patient was at the discretion of the therapist and generally depended upon the level of severity of the patient’s presenting problem. On average, patients treated at step 3 within IAPT complete treatment within 4 months. The mean number of sessions, for patients who completed a course of treatment and did not drop out, in the reporting year 2017–2018 was 6.8 (NHS Digital, 2018). The mean number of sessions for patients who completed treatment in this study was 6.76 (SD 3.13). The mean recovery rate was 51% (SD 19.02) and median was 50%. The clinical recovery data, for all therapists included in this study, were normally distributed.
Data analyses
A hierarchical log-linear analysis was performed to determine a statistical model for the associations among categorical variables including therapist (fidelity score and adherence score), patient (age, gender and initial anxiety and depression severity scores) and clinical outcomes (reliable improvement, recovery). Log-linear models offer a valuable systematic approach when analysing complex multi-dimensional contingency tables, allowing comparative analyses of differing effects between variables to be undertaken (Everitt, Reference Everitt1992). Log-linear models are a novel application in psychological therapy literature but are a more standard method in medical disciplines (see Helmy et al., Reference Helmy, Timofeev, Palmer, Gore, Menon and Hutchinson2010; Maimaris et al., Reference Maimaris, Summers, Browning and Palmer1994; Milewska et al., Reference Milewska, Citko, Jankowska, Milewski, Kononczuk, Wiesak, Morgan and Milewski2018). The model does not distinguish between dependent and independent variables and treats all variables as equal, thus making it possible to see which variables are associated with each other and which are not (Everitt, Reference Everitt1992). Unlike other statistical analyses (such as logistic regression) that distinguish between dependent and independent variables, the approach adopted has the advantages of considering all variables on an equal footing for data exploration purposes; the ability to consider more than one outcome variable simultaneously; a graphical output to enhance understanding of the associations between the variables; and thereby an inherent check on the plausibility of said associations, such as an anticipated link between outcome variables Improvement and Recovery. All the associations arising from the hierarchical log-linear model are illustrated in a diagrammatic format (see Fig. 3) which may aid visual interpretation. The hierarchical model is produced by successively eliminating, one at a time, the least statistically significant interaction effect among pairs of ordinal (or binary) variables. In general, from a pool of n such variables, the initial number of two-way interactions is n×(n–1)/2, so in the case as here of n = 8, this evaluates to 28 possible two-way interactions.

Figure 3. Graphical model of log-linear analysis findings.
In this analysis, 612 patients were included in the model, derived by a backwards elimination procedure beginning with an unsaturated model for all eight main effects and their 28 possible two-way interactions. The model required that all variables were transformed into categorical variables. Table S2 in the Supplementary material shows how this was done using a median split of each variable. Model selection was derived using SPSS (hierarchical log-linear model selection, IBM SPSS, version 24). The final model was checked to have no outliers and approximately normally distributed residuals. A graphical representation of this model, indicating the relative strengths of the various interactions, is given in Fig. 3 with the generating class shown in Table S1 in the Supplementary material.
Results
Figure 3 shows the final model derived from the log-linear analysis findings. Straight lines between variables indicate statistically significant interactions between variables, where three lines represent a p-value of <0.001. To denote the relative strengths of associations within the log-linear model, p-values are taken as surrogates for their corresponding test statistics as effect size measures and depicted in three categories. The model included gender as no effect only and the two-way interactions between A-score×F-score, age×GAD-7 at start of treatment, age×recovery, F-score×recovery, GAD-7×improvement, GAD-7×PHQ-9, GAD-7×recovery, PHQ-9×improvement and improvement×recovery, having excluded all other interactions as non-statistically significant. In therapist variables, A-scores and F-scores significantly interacted (p < 0.001), and F-scores interacted with recovery (p < 0.05). Recovery, in turn, was significantly related to the GAD-7 score (p < 0.001). The GAD-7 score was the pre-intervention score and was used as an indicator of severity at the start of treatment. The GAD-7 and PHQ-9 scores were also significantly related (p < 0.001), suggesting that patients were likely to be severe/not severe on both outcome measures at the start of treatment. The other patient variable related to recovery was patient age (p < 0.05). Additionally, age was related to GAD-7 scores (p < 0.01), suggesting that older patients, in this sample, were more likely to present with anxiety disorders and were also more likely to recover. The absence of any significant interaction with gender confirms that whether a patient is male or female makes no difference to other variables in the model. In Fig. 3, the absence of a line between any pair of variables is indicative of an absence of a statistically significant association. For example, in this model the patient’s gender has no impact on any of the other variables. By contrast, the presence of a single, double or triple line between variables, respectively, indicates increasingly strong statistically significant links and can be interpreted, respectively, as weak, moderate or strong associations. Table S1 (in the Supplementary material) indicates more precisely the relative strength of these inter-relationships or so-called ‘interaction effects’ (the simultaneous effect of one variable on another). The table entries can be used to determine the relative order of importance of the dependencies, although as a note of caution this may risk over-interpretation. The main message from the illustrative model shown in Fig. 3 is to distinguish among categories of none, weak, moderate or strong statistical interaction effects, not to make precise assertions about degree of strength within any one of these categories. Non-adjacent variables are also linked, but only indirectly, for example PHQ-9 appears related to Recovery through its association with GAD; or Adherence to a protocol to Recovery through its association with Fidelity to the CBT model. Therefore, Fig. 3 shows the commonsense associations produced by the model. For instance (and as mentioned in Introduction), the strength of the link between ‘Recovery’ and ‘Reliable Improvement’ serves to give confidence that the model overall is coherent and plausible. Thus when considering what is the role of age, or for that matter any other variable, in the context of the entire dataset the method applied means one can literally see where it fits into the big (and highly complex) picture.
It should be emphasised that just as a correlation analysis, between a pair of variables (e.g. with correlation coefficient numerically close to 1) does not imply causation, so too is this model-building and model-displaying tool best seen as an exploratory analysis, not one for establishing causal links. Further research is required to confirm the links that have been produced and illustrated in Fig. 3, but the exercise as a whole generates, rather than tests, hypotheses.
Discussion and implications for practice
This study sought to understand the associations between therapist variables and clinical outcome. Current research suggests that approximately 5–8% of the variability in clinical outcomes is due to therapist effects (Lutz et al., Reference Lutz, Rubel, Schiefele, Zimmermann, Bohnke and Wittmann2015). Whilst significant, therapist effects reveal very little about what the most, or least, effective therapists are doing with their patients. Therefore, this study focused on understanding whether fidelity to the CBT model and/or adherence to an evidence-based protocol, were related to clinical outcome. This was only possible because of the availability of therapy transcripts for every therapy session delivered by each therapist who participated in the study. It is widely accepted that therapists vary in their ability to effectively deliver interventions to patients, with some being far better than others (Baldwin and Imel, Reference Baldwin, Imel and Lambert2013; Lutz et al., Reference Lutz, Rubel, Schiefele, Zimmermann, Bohnke and Wittmann2015; Saxon and Barkham, Reference Saxon and Barkham2012). Understanding what the most effective therapists are doing with their patients has the potential to significantly impact on therapist training and supervision and may result in more patients reaching clinical recovery (Castonguay et al., Reference Castonguay, Eubanks, Goldfried, Muran and Lutz2015).
The findings from this study appear to support the assertions of Gyani et al. (Reference Gyani, Shafran, Layard and Clark2013), Ginzburg et al. (Reference Ginzburg, Bohn, Hofling, Weck, Clark and Stangier2012) and Shafran et al. (Reference Shafran, Clark, Fairburn, Arntz, Barlow and Ehlers2009) that fidelity to the CBT model and adherence to an evidence-based protocol are significantly related to achieving good clinical outcomes. Not all studies have reported a significant relationship between fidelity to the CBT model or adherence (see Kazantis et al., Reference Kazantis, Clayton, Cronin, Farchione, Limburg and Dobson2018; Webb et al., Reference Webb, DeRubeis and Barber2010). Previous studies have used a range of methods to rate fidelity and adherence within a range of treatment settings. This means it is difficult to compare the results from this study with previous studies. However, most studies have tended not to review large quantities of randomly selected therapy sessions. Where sessions have been reviewed, these have often been self-selected (see Brosan et al., Reference Brosan, Reynolds and Moore2006; Huppert et al., Reference Huppert, Bufka, DeRubeis, Gorman, Shear and Woods2001; Strunk et al., Reference Strunk, Brotman, DeRubeis and Hollon2010) or the research has been a randomised controlled study where therapists receive specific training in protocol adherence (see Ginzburg et al., Reference Ginzburg, Bohn, Hofling, Weck, Clark and Stangier2012; Haug et al., Reference Haug, Nordgreen, Ost, Tangen, Kvale, Hovland, Heiervang and Havik2016; Saxon et al., Reference Saxon, Firth and Barkham2017). Therefore, it is unremarkable when researchers report high levels of protocol adherence in RCTs. In this study, not only were the therapists treating patients in a real-world clinical setting, but therapists were not permitted to self-select therapy sessions for review. Therapy sessions were selected randomly by the raters. This is likely to increase the probability that the sessions reviewed were representative of the therapists’ work. In addition, all the sessions of three patients, who had completed treatment, were reviewed by the raters in order to assess adherence to a disorder-specific protocol. Most other research reported reviewing either single sessions or a small selection of sessions, not all the session for the entire treatment. In addition, there is an argument that suggests that it is problematic to assess adherence, from a small selection of sessions selected from an entire course of treatment, because adherence varies from session to session (Minonne, Reference Minonne2008). Thus, this study has not only built upon existing research, but it has extended the work of others in this important field.
In their meta-analysis Zarafonitis-Muller et al. (Reference Zarafonitis-Muller, Kuhr and Bechtdolf2014) report a positive correlation between clinical outcomes and therapist competence (r = 0.24) and adherence (r = 0.06). More recently, Kazantis et al. (Reference Kazantis, Clayton, Cronin, Farchione, Limburg and Dobson2018) also reported similar findings. This study also finds that competence (as assessed by the CTS-R) is associated with clinical recovery (p < 0.05). An interesting finding is that adherence (A-score) is related to fidelity to the CBT model (F-score), which in turn is related to recovery. This might suggest that, first and foremost, delivering therapy that contains the core elements of CBT, i.e. agenda setting, giving and eliciting feedback, guided discovery, conceptual integration, etc., is highly important, but when therapists achieve this whilst adhering to a protocol, clinical outcomes improve. Metaphorically, fidelity to the CBT model is the vehicle that is required to effectively deliver a disorder specific protocol and that one without the other is less effective. Indeed, the continuing research of the first author supports this conjecture.
A further noteworthy finding of this study is the distribution of the F-scores (fidelity to the CBT model) and A-scores (adherence to a protocol). Little is known about therapist behaviour in real-world settings (Brosan et al., Reference Brosan, Reynolds and Moore2006). This is the second study to have access to the therapy transcripts of every patient treated by the therapists working in a clinical setting. The first study (see Ewbank et al., Reference Ewbank, Cummins, Tablan, Bateup, Catarino, Martin and Blackwell2019) used a deep learning model on 90,934 internet enabled cognitive behavioral therapy transcripts to categorise therapist utterances. The authors demonstrated an association between certain utterances and clinical outcome. Given that there is extensive literature that supports the idea that there is significant variance in therapist competence (Johns et al., Reference Johns, Barkham, Kellett and Saxon2019), it is not surprising that the findings from this study also suggest this. Even studies that have not been conducted in real-world settings report this variance. Strunk et al. (Reference Strunk, Brotman, DeRubeis and Hollon2010) using data drawn from a randomised trial report a variance in fidelity to the model with a range of 17.8–56.6% and mean score of 39.7%. Similarly, the variance in therapist adherence to a protocol is not uncommon. There are many possible reasons why therapists drift away from a protocol. These include: negative beliefs about aspects of delivering treatment such as exposure (Deacon et al., Reference Deacon, Farrell, Kemp, Dixon, Sy and Zhang2013) which lead to avoidance of these aspects of therapy, therapist judgement in deciding treatment methods which leads to a dilution of protocols (Grove et al., Reference Grove, Zald, Lebow, Snitz and Nelson2000) and inflated self-beliefs about competence (Parker and Waller, Reference Parker and Waller2015) which may negatively bias therapists from effective continuing professional development. What is perhaps more important is this further evidence that therapist drift is a phenomenon. If fidelity to the CBT model and adherence to a protocol are correlated with clinical outcome, then further work is required to support therapists to amplify their work beyond what is currently available.
A next step would be to undertake a clinically driven thematic analysis of the transcripts of the most effective therapists to draw out key themes and strengths and then embed this learning into the continuing professional development of all therapists. Huppert et al. (Reference Huppert, Bufka, DeRubeis, Gorman, Shear and Woods2001) raise the question that if it were possible to understand the difference between effective and ineffective therapists then the next step is to understand whether or not it is possible to make ineffective therapists more effective. Furthermore, this learning may be delivered in a more immediate way, whilst the therapist is delivering a treatment session. Given that IECBT is delivered online, using written communication, it is possible to develop digitally enabled clinical decision support tools to provide feedback and guidance on good clinical practice, thus enhancing, or even amplifying, the effect of evidence-based psychological therapies. This may build upon the research of Lutz et al. (Reference Lutz, Rubel, Schiefele, Zimmermann, Bohnke and Wittmann2015) who argue that the application of feedback tools in routine care delivery can be used to support therapists to make sound clinical decisions.
Several possible limitations of this study should be highlighted. Firstly, this study analyses therapists who delivered CBT online using written communication. It is not yet possible to understand whether a therapist’s behaviour online differs from their behaviour when delivering treatment face-to-face. It should be noted that further research is required to understand any qualitative differences between the two methods. Secondly, this study examines CBT delivered in the context of the UK’s IAPT programme; it is therefore unknown whether the findings are generalisable across other populations. Thirdly, the study has used the CTS-R to rate therapist fidelity to the CBT model and whilst the CTS-R is commonly used tool, it was not developed to use on IECBT transcripts and therefore further research is required to understand whether it is the most appropriate instrument to use to assess therapist fidelity to the CBT model. Furthermore, this research has used the Roth and Pilling (Reference Roth and Pilling2008) framework to assess therapist adherence to a disorder-specific protocol. It is important to note that this method of reviewing every session in order to assess therapist adherence has (to the best of the authors’ knowledge) never been undertaken before. Further research is required to understand if this is a valid and reliable method. A fourth limitation of this study is that patients who dropped out of treatment were excluded from the analysis, thus reducing the patient sample size. Further research is needed to explore whether therapist fidelity or adherence were associated with patient drop-out. Finally, despite the fact this study used six raters and inter-rater reliability training was conducted, rating therapist treatment sessions, as in all research in this area, is undoubtedly prone to some subjectivity. These include rater bias and the risk that raters may select sessions that were simpler or easier to rate. Therefore, some caution should be assumed when interpreting the results.
Despite these limitations, the findings from this study add to the body of work already conducted in this area especially because many of the limitations cited in previous studies have been addressed. The delivery of evidence-based psychological interventions using technology has opened the door to learning more about what drives good clinical outcomes.
Supplementary material
To view supplementary material for this article, please visit https://doi.org/10.1017/S1754470X20000252
Acknowledgements
None.
Conflicts of interest
S.E.B. and A.C. are employees of Ieso Digital Health.
Financial support
The authors received no funding from an external source.
Ethical statement
This study was granted ethical approval by the Departmental Research Ethics Panel in the faculty of Health, Social Care and Medicine at Anglia Ruskin University in January 2018 (reference no. FHSE-DREP-17-069).
Key practice points
(1) Therapist drift away from the CBT model in IAPT is commonplace and is likely to affect clinical outcomes.
(2) Fidelity to the CBT model is associated with clinical outcomes.
(3) IECBT offers clinicians and researchers new ways of studying therapist behaviour.
(4) An under-used statistical method may increase understanding of complex multivariate data in psychotherapy.
Comments
No Comments have been published for this article.