Around 10% of British children and adolescents have psychiatric disorders that result in substantial distress or social impairment (Reference Meltzer, Gatward and GoodmanMeltzer et al, 2000). Although there are evidence-based treatments for many child mental health problems (Reference Goodman and ScottGoodman & Scott, 1997), only about 20% of children with psychiatric disorders are in contact with specialist mental health services (Reference Offord, Boyle and SzatmariOfford et al, 1987; Reference Burns, Costello and AngoldBurns et al, 1995; Reference Leaf, Alegria and CohenLeaf et al, 1996; Reference Meltzer, Gatward and GoodmanMeltzer et al, 2000). There would seem to be substantial scope, therefore, for the development and implementation of routine screening measures to detect children at high risk of mental health problems with a view to further assessment and treatment if necessary. In psychiatric clinic samples, diagnostic predictions based on the Strengths and Difficulties Questionnaire (SDQ) agree well with clinical diagnoses (Reference Goodman, Renfrew and MullickGoodman et al, 2000b ). This study examines how well the SDQ can predict child psychiatric disorders in a large British community sample.
METHOD
Sample
In 1999, the Office for National Statistics carried out a survey of the mental health of British 5- to 15-year-olds. The total sample of 10 438 children was recruited through child benefit records. Child benefits are available without means-testing and are claimed on behalf of around 98% of British children. Details of ascertainment and representativeness have been presented elsewhere (Reference Meltzer, Gatward and GoodmanMeltzer et al, 2000). Parents provided questionnaire and interview information on 99% of the sample (with the remaining 1% largely being composed of parents who could not speak English well). Ninety-seven per cent of families gave permission to send teachers a postal questionnaire, with a completed questionnaire being returned by 80% of teachers. Questionnaires and interviews were completed by 95% of the eligible 11- to 15-year-olds. For the purpose of the analyses reported in this paper, children were only included if full information was available, that is parent and teacher SDQs on all children, plus self-report SDQs on subjects aged 11 or over. By these criteria, a total of 7984 children (76%) had full data while 2454 children (24%) had incomplete data. Exclusion of all children with incomplete data made subsequent analyses much easier to interpret since it was possible to compare screening efficiency using complete or partial information on exactly the same subjects. Since the children with full data were at lower psychiatric risk than the children with incomplete data (Reference Meltzer, Gatward and GoodmanMeltzer et al, 2000), the rates of disorder reported here are lower than the published rates based on the full sample (Reference Meltzer, Gatward and GoodmanMeltzer et al, 2000). Studying the SDQ on a slightly ‘super-normal’ community sample should not have exaggerated its screening efficiency — indeed, it is more likely to have attenuated it. Of the 7984 subjects included in the present study, 49.7% were male and 50.3% were female; the mean age (s.d.) was 10.2 years (3.1).
Questionnaire measures
The SDQ is a brief questionnaire that can be administered to the parents and teachers of 4- to 16-year-olds and to 11- to 16-year-olds themselves (Goodman, Reference Goodman1997, Reference Goodman1999; Reference Goodman, Meltzer and BaileyGoodman et al, 1998). Besides covering common areas of emotional and behavioural difficulties, it also enquires whether the informant thinks that the child has a problem in these areas and, if so, asks about resultant distress and social impairment. Further information on the SDQ and copies of the questionnaire in over 40 languages can be obtained free from http://www.sdqinfo.com. Computerised algorithms exist for predicting psychiatric disorder by bringing together information on symptoms and impact from SDQs completed by multiple informants (Reference Goodman, Renfrew and MullickGoodman et al, 2000b ). The algorithm makes separate predictions for three groups of disorders, namely conduct—oppositional disorders, hyperactivity—inattention disorders, and anxiety—depressive disorders. Each is predicted to be unlikely, possible or probable. Predictions of these three groups of disorders are combined to generate an overall prediction about the presence or absence of any psychiatric disorder.
Psychiatric diagnosis
The children were assigned psychiatric diagnoses on the basis of the Development and Well-Being Assessment (DAWBA; Reference Goodman, Ford and RichardsGoodman et al, 2000a ), an integrated package of questionnaires, interviews and rating techniques designed to generate psychiatric diagnoses on 5- to 16-year-olds. Non-clinical interviewers administer a structured interview to parents and older children, supplementing the structured questions with open-ended questions to get respondents to describe the problems in their own words. Experienced clinical raters assign ICD—10 (World Health Organization, 1994) and DSM—IV (American Psychiatric Association, 1994) diagnoses after reviewing the interview records and teacher questionnaires. In the validation study of the DAWBA (Reference Goodman, Ford and RichardsGoodman et al, 2000a ), there was excellent discrimination between community and clinic samples in rates of diagnosed disorder. Within the community sample, subjects with and without diagnosed disorders differed markedly in external characteristics and prognosis. In the clinic sample, there was substantial agreement between DAWBA and case-note diagnoses.
In the study reported here, DAWBA diagnoses were generated blind to the SDQ scores. For the present paper, the diagnoses are nearly all based on the research diagnostic criteria of ICD—10. Choosing ICD—10 rather than DSM—IV makes little difference as far as emotional and conduct—oppositional disorders are concerned, where group membership is very similar whichever classification is used. It is only for the hyperactivity disorders that there are marked discrepancies between the two classifications — hence screening efficiency is reported separately for ICD—10 hyperkinetic disorders and DSM—IV attention-deficit/hyperactivity disorders (ADHD).
RESULTS
Overall screening efficiency
The SDQ algorithm predicted that a psychiatric disorder was ‘unlikely’ in 70.1% of the sample, was ‘possible’ in 19.4% of the sample and was ‘probable’ in 10.5% of the sample. The proportion of ‘probables’ was 13.4% for boys and 7.7% for girls. Table 1 shows the match between prediction and ICD—10 psychiatric diagnosis: less than 2% of ‘unlikely’ children had a psychiatric diagnosis, compared with 11% for ‘possible’ children and 53% for ‘probable’ children (χ2 for trend=2059, 1 d.f., P < 0.001).
Table 1 Overall agreement between Strengths and Difficulties Quesionnaire (SDQ) prediction and psychiatric diagnosis
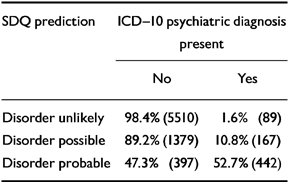
SDQ prediction | ICD-10 psychiatric diagnosis present | |
---|---|---|
No | Yes | |
Disorder unlikely | 98.4% (5510) | 1.6% (89) |
Disorder possible | 89.2% (1379) | 10.8% (167) |
Disorder probable | 47.3% (397) | 52.7% (442) |
The SDQ predictions were dichotomised into ‘positive’ and ‘negative’ in order to make it possible to describe the screening efficiency of the SDQ in the conventional manner in terms of specificity, sensitivity, positive predictive value and negative predictive value. ‘Probable’ predictions were counted as positive, whereas ‘unlikely’ and ‘possible’ predictions were both counted as negative. For nearly all predictions, though, it is worth noting that the majority of ‘false negatives’ (i.e. children with a particular diagnosis who were not rated ‘probable’ by the SDQ) were rated ‘possible’ rather than ‘unlikely’. In other words, most of the false negatives were partial rather than complete. For example, 256 children with an ICD—10 diagnosis of psychiatric disorder were not rated as ‘probable’ by the SDQ algorithm; 167 (65%) of these ‘false negatives’ were rated as ‘possible’ rather than ‘unlikely’ (Table 1). With this reservation, the screening efficiency of multi-informant SDQs for the entire group of 5- to 15-year-olds is as follows: sensitivity 63.3% (95% CI 59.7-66.9%), specificity 94.6% (94.1-95.1%), positive predictive value 52.7% (49.3-56.1%), negative predictive value 96.4% (96.0-96.8%).
The likelihood of the algorithm detecting psychiatric disorder varied with the severity of the disorder. Children with ICD—10 psychiatric disorders were dichotomised into milder and more severe cases on the basis of the level of associated distress and social impairment. The proportion of these children predicted to have a ‘probable’ disorder by the SDQ algorithm was 45% (153/342) for the milder cases compared with 81% for the more severe cases (289/356) (continuity-adjusted χ2=98.2, 1 d.f., P < 0.001).
Sensitivity to different diagnoses
These findings on screening efficiency apply to all diagnoses combined. How did this vary by type of psychiatric disorder? The following analyses focus just on sensitivity since this value is likely to be of particular importance in deciding whether the screening efficiency is adequate to warrant a formal trial of screening. As shown in Table 2, the sensitivity varies according to the diagnosis, identifying over 70% of individuals with conduct, hyperactivity, depressive and some anxiety disorders, but under 50% of individuals with specific phobias, separation anxiety, eating disorders and panic disorder/agoraphobia. In general, sensitivity was slightly lower for females than for males — a difference that was statistically significant for all disorders combined (continuity-adjusted χ2=13.5, 1 d.f., P < 0.001) but not for any diagnostic group or individual diagnosis.
Table 2 Sensitivity of the Strengths and Difficulties Questionnaire (SDQ) prediction by diagnostic groups

Detecting | Sensitivity, % |
---|---|
Any psychiatric disorder | 63.3 (442/698) |
Any conduct-oppositional disorder | 76.2 (292/383) |
Any hyperkinetic disorder (ICD-10) | 86.1 (99/115) |
Any ADHD disorder (DSM-IV) | 75.4 (147/195) |
Any depressive disorder | 74.6 (50/67) |
Any anxiety disorder | 50.5 (142/281) |
Separation anxiety disorder | 45.5 (25/55) |
Specific phobia | 30.9 (25/81) |
Social phobia | 72.7 (16/22) |
Panic disorder/agoraphobia | 40.0 (4/10) |
Post-traumatic stress disorder | 72.7 (8/11) |
Obsessive-compulsive disorder | 75.0 (12/16) |
Generalised anxiety disorder | 64.4 (29/45) |
Other anxiety disorder | 69.8 (60/86) |
Less common diagnoses | 67.5 (27/40) |
Pervasive developmental disorder | 77.3 (17/22) |
Tic disorder | 60.0 (3/5) |
Eating disorder | 44.4 (4/9) |
Other less common disorder | 80.0 (4/5) |
Predictive efficiency by age and informant
The analyses presented so far have been for all ages from 5 to 15, and for predictions based on full information on each child (i.e. parent and teacher SDQs for all children, plus self-report SDQ for 11- to 15-year-olds). Further analyses were carried out splitting the sample into those who had and had not reached their 11th birthday. These further analyses examined how the sensitivity changed when predictions were based on incomplete data, for example, looking at predictions when just parent SDQs were entered into the predictive algorithm. Table 3 presents data on children aged under 11, showing the sensitivity of SDQ predictions for various broad-band diagnoses. These predictions are based on the combination of parent and teacher SDQs (PT), or just parent SDQs (P) or just teacher SDQs (T). For all diagnoses, PT has a greater sensitivity than either P or T. Comparing the sensitivities of P and T, then T is better than P at predicting externalising disorders, although this is only significant for conduct disorder (McNemar χ2=4.7, 1 d.f., P < 0.05). Conversely, P is better than T at detecting internalising disorders, although this is only significant for anxiety disorders (McNemar χ2=10.8, 1 d.f., P < 0.01).
Table 3 Sensitivity of the Strengths and Difficulties Questionnaire (SDQ) prediction for children aged 5 to 10 (n=4776)

Detecting | Sensitivity | ||
---|---|---|---|
PT | P | T | |
Any psychiatric disorder (n=383) | 62.1% (238) | 29.8% (114) | 34.5% (132) |
Any conduct-oppositional disorder (n=211) | 73.5% (155) | 36.0% (76)† | 47.9% (101) |
Any hyperkinetic disorder (ICD-10) (n=75) | 86.7% (65) | 33.3% (25) | 49.3% (37) |
Any ADHD disorder (DSM-IV) (n=117) | 75.2% (88) | 29.9% (35) | 41.9% (49) |
Any anxiety disorder (n=145) | 45.5% (66) | 33.8% (49) | 15.9% (23)* |
Any depressive disorder (n=13) | 69.2% (9) | 53.9% (7) | 30.8% (4) |
Less common diagnoses (n=25) | 76.0% (19) | 40.0% (10) | 20.0% (5) |
Table 4 presents comparable data for children aged 11 or over. There are more columns in Table 4 than in Table 3 because children aged 11 or over can complete the self-report SDQ. Consequently, the full multi-informant prediction is based on parent, teacher and self-report SDQs (PTS). There are three sets of predictions based on just two of these three informants (P, T, S). For all diagnoses, PTS has the greatest sensitivity. If one rater has to be dropped, PT is generally better than PS or TS. The main cost of dropping the self-ratings is missing some emotional disorders. If one adult informant has to be dropped (i.e. comparing PS with TS, or comparing P with T), then retaining the teacher rating detects more externalising disorders, while retaining the parent rating detects more internalising disorders. S is the single least useful screening strategy, being less sensitive than P for all disorders, and being less sensitive than T for all disorders other than depression. (Significant differences between P, T and S are shown in Table 4.)
Table 4 Sensitivity of the Strengths and Difficulties Questionnaire (SDQ) prediction for children aged 11 to 15 (n=3208)

Detecting | Sensitivity | ||||||
---|---|---|---|---|---|---|---|
PTS | PT | PS | TS | P | T | S | |
Any psychiatric disorder (n=315) | 64.8% (204) | 59.4% (187) | 41.3% (130) | 47.9% (151) | 33.7% (106) | 38.7% (122) | 15.9% (50)* † |
Any conduct-oppositional disorder (n=172) | 79.7% (137) | 77.3% (133) | 44.8% (77) | 61.6% (106) | 40.1% (69)† | 55.8% (96) | 14.5% (25)* † |
Any hyperkinetic disorer (ICD-10) (n=40) | 85.0% (34) | 85.0% (34) | 47.5% (19) | 65.0% (26) | 45.0% (18) | 65.0% (26) | 10.0% (4)* † |
Any ADHD disorder (DSM-IV) (n=78) | 75.6% (59) | 75.6% (59) | 46.2% (36) | 59.0% (46) | 41.0% (32)† | 59.0% (46) | 12.8% (10)* † |
Any anxiety disorder (n=136) | 55.9% (76) | 47.1% (64) | 44.9% (61) | 41.2% (56) | 33.1% (45) | 27.9% (38) | 22.1% (30)* |
Any depressive disorder (n=54) | 75.9% (41) | 61.1% (33) | 63.0% (34) | 55.6% (30) | 44.4% (24) | 31.5% (17) | 33.3% (18) |
Less common diagnoses (n=15) | 53.3% (8) | 53.3% (8) | 20.0% (3) | 40.0% (6) | 20.0% (3) | 40.0% (6) | 6.7% (1) |
SDQ predictions for type of disorder
The SDQ algorithm generates specific predictions for ‘conduct disorders’, ‘hyperactivity disorders’ and ‘emotional disorders’ as well as an overall prediction for ‘any disorder’. Table 5 shows the proportion of children with particular clinical diagnoses who received ‘probable’ SDQ predictions for each of these specific categories. For each psychiatric disorder, substantially more children obtained the SDQ ‘any disorder’ rating than the more specific ratings. Detecting children with emotional and hyperactivity disorders was particularly dependent on the presence of comorbidity. For example, although the SDQ algorithm detected three-quarters of children with a clinical diagnosis of depression as having ‘any disorder’, the specific prediction was more often a conduct than an emotional disorder.
Table 5 Detecting broad diagnostic groupings through different Strengths and Difficulties Questionnaire (SDQ) predictions

Clinical diagnosis | Proportion rated as ‘probable’ by SDQ for | |||
---|---|---|---|---|
Conduct disorder | Hyperactivity disorder | Emotional disorder | Any disorder | |
Any psychiatric disorder (n=698) | 44.4% (310) | 21.5% (150) | 15.0% (105) | 63.3% (442) |
Any conduct-oppositional disorder (n=383) | 68.2% (261) | 26.6% (102) | 7.3% (28) | 76.2% (292) |
Any hyperkinetic disorder (ICD-10) (n=115) | 62.6% (72) | 63.5% (73) | 3.5% (4) | 86.1% (99) |
Any ADHD disorder (DSM-IV) (n=195) | 55.9% (109) | 50.8% (99) | 7.2% (14) | 75.4% (147) |
Any anxiety disorder (n=281) | 25.3% (71) | 9.3% (26) | 28.1% (79) | 50.5% (142) |
Any depressive disorder (n=67) | 47.8% (32) | 16.4% (11) | 34.3% (23) | 74.6% (50) |
Less common diagnoses (n=40) | 22.5% (9) | 35.0% (14) | 22.5% (9) | 67.5% (27) |
Characteristics of ‘false positives’
As shown in Table 1, there were 397 children who were predicted by the SDQ algorithm to have a ‘probable’ disorder, but who did not have an ICD—10 psychiatric diagnosis. Who were these ‘false positives’? The SDQ algorithm is designed so that it will not predict a ‘probable’ disorder unless at least one informant has reported the combination of a high symptom score and resultant impact. The perceived level of these reported problems can be gauged from an SDQ question that asks informants to rate whether the child's difficulties are absent, minor, definite or severe. All 397 of the false positives were reported as having some difficulties by at least one informant, with 273 (69%) being reported as having definite or severe difficulties by at least one informant. Of the false positives, 235 (59%) had a hyperactivity score in the ‘abnormal’ range according to at least one informant; the corresponding numbers scoring in the abnormal range for the emotional symptoms score and the conduct problems score were 246 (62%) and 235 (59%). All children scored in the abnormal range on at least one symptom score, while 251 (63%) scored in the abnormal range on at least two of the symptom scores. Compared with the rest of the sample, the false positives were more likely to be male (60% v. 49%, continuity-adjusted χ2=18.1, 1 d.f., P < 0.001), but did not differ in age (10.4 years v. 10.2 years, t=1.3, 7982 d.f., NS).
DISCUSSION
Predicting the presence of psychiatric disorder
The present study of a large epidemiological sample shows that a predictive algorithm based on multi-informant SDQs is able to detect children with psychiatric disorders in the community with reasonable efficiency. The algorithm identifies about two-thirds of the children with psychiatric disorders (including four-fifths of those with severe psychiatric disorders), generating only slightly more false positives than negatives. This level of prediction is potentially useful for researchers who want to ascertain ‘high-risk’ samples for further study, and also for clinicians who want to embark upon a community screening programme.
The screening efficiency of the algorithm depends on the diagnosis. Identification is good (with a sensitivity of 70-90%) for conduct—oppositional disorders, hyperactivity disorders, depression, pervasive developmental disorders, and some anxiety disorders. By contrast, identification is poor (with a sensitivity of 30-50%) for specific phobias, panic disorder/agoraphobia, eating disorders and separation anxiety. Not surprisingly, the algorithm seems most likely to miss children with relatively encapsulated symptoms that are not well covered by the SDQ. Thus, the SDQ contains no questions about dieting or panic attacks and only one question each on fears and separation anxiety. Children may have severe and disabling symptoms in these areas and yet have low SDQ symptom scores — and without a high score in at least one domain (conduct, emotion or hyperactivity), the algorithm will not predict that a disorder is ‘probable’. If the algorithm is not good at detecting ‘islets’ of severe symptoms, it is much better at detecting children with more generalised symptomatology. In effect, the algorithm capitalises on the high level of comorbidity that is a well-recognised feature of child psychopathology (Reference Angold, Costello and ErkanliAngold et al, 1999). For example, the algorithm detects three-quarters of children with depressive or obsessive—compulsive disorders despite the fact that the SDQ has only one question on misery and no questions at all on obsessions or compulsions. This is because depressive and obsessive—compulsive disorders are commonly associated by a broad range of anxiety and conduct symptoms. Similarly, three-quarters of children with pervasive developmental disorders are recognised as a result of associated conduct, emotional and hyperactivity problems even though the SDQ does not cover ‘core’ autistic symptoms.
Predicting the type of disorder
In child mental health clinics, the algorithms can predict the broad type of disorder — conduct, emotional or hyperactivity — with relatively few false negatives (Reference Goodman, Renfrew and MullickGoodman et al, 2000b ). Prediction of type of disorder in a community sample is more prone to false negatives. In the milder cases that predominate in community as opposed to clinic samples, emotional disorders are particularly likely to be missed. For example, a child from a clinic sample with a severe depressive conduct disorder may correctly be predicted by the SDQ algorithm to have both a conduct and an emotional disorder, whereas a child from a community sample with a milder depressive conduct disorder may be predicted to have a conduct disorder but not an emotional disorder. To a lesser extent, children in the community with mild hyperkinetic conduct disorder may be predicted to have a conduct disorder but no hyperactivity disorder. Consequently, if researchers or clinicians want to detect as many emotional or hyperactivity disorders as possible, they would be well advised to use the SDQ prediction for ‘any disorder’ rather than for ‘emotional disorder’ or ‘hyperactivity disorder’. A second-stage screening procedure can then be used to detect which SDQ ‘positive’ children have the disorder of particular interest.
Choice of informant
The SDQ prediction works best when SDQs have been completed by all possible informants, namely parents and teachers in all instances, and young people themselves from the age of 11 onwards. If it is impossible or uneconomical to collect SDQs from all possible informants, who are the most useful informants? Overall, parents and teachers provide information of roughly equal predictive value, although their relative value depends on the type of disorder. Thus information from parents is slightly more useful for detecting emotional disorders while information from teachers is slightly more useful for detecting conduct and hyperactivity disorders. For young people aged 11 or over, self-report SDQs provide an additional source of possible information. For conduct and hyperactivity disorders, self-report data are of less predictive value than data from either parents or teachers. For emotional disorders, self-report data are about as useful as teacher data, but less useful than parent data.
False negatives and positives
While the SDQ predictions identified both false negatives and false positives, some of these misclassifications were simply questions of degree. Most of the false negatives were children who were predicted to have ‘possible’ disorders by the SDQ algorithm. In order to generate the Yes—No predictions that are needed to describe screening efficiency in conventional terms, predictions of ‘unlikely’ and ‘possible’ were combined for most of the analyses reported in this paper. In the real world, the three categories of ‘unlikely,’ ‘possible’ and ‘probable’ could elicit a graded response. In a screening programme, for example, children predicted by the SDQ algorithm to have a ‘probable’ disorder could subsequently be assessed in more detail, while children predicted to have a ‘possible’ disorder could have the SDQ screening repeated some 6 months later to see whether symptoms have resolved or progressed. As regards false positives, it is important to note that these children were all regarded as having problems by at least one informant. This makes it less likely that the offer of further assessment would come as a complete surprise to the child or family. Furthermore, a more detailed assessment may help allay existing concerns, or may facilitate access to help for problems that are real even if they do not necessarily warrant a clinical diagnosis.
Potential value in screening
The findings of this study suggest that the SDQ could potentially be considered for a community-wide screening programme to improve the detection and treatment of child mental health problems. At present, only a minority of children with psychiatric disorders reach specialist mental health services — around 20% or less according to many studies (Reference Offord, Boyle and SzatmariOfford et al, 1987; Reference Burns, Costello and AngoldBurns et al, 1995; Reference Leaf, Alegria and CohenLeaf et al, 1996; Reference Meltzer, Gatward and GoodmanMeltzer et al, 2000). Community-wide deployment of SDQ-based screening could potentially double or treble this proportion (although other screening measures would be needed for disorders such as anorexia nervosa that are not well detected by the SDQ). Whether improving detection would be useful depends on many factors. First, although there is good evidence from clinical trials for the efficacy of a range of treatments for child psychiatric disorders, it is far less clear that the sorts of treatments commonly deployed in child mental health services are effective in practice (Reference Weisz, Donenberg and HanWeisz et al, 1995). There would obviously be no point in identifying a greater proportion of children with psychiatric disorders in the community if the only consequence were greater access to ineffective treatments. Second, even if treatments are effective, there is no point identifying more children in need of treatment if existing services are already overstretched and no resources are available to see the extra cases identified by screening. Third, it is important to ensure that the screening process does not do serious harm, for example by causing anguish to false positives or by labelling children who would have been better off unlabelled. Finally, community-wide screening would consume considerable resources, not only in the administration and scoring of the questionnaires, but also in subsequent assessment of screen-positive children to see if they really have problems that warrant specialist attention. These resources might have been employed more profitably in other ways, such as on primary prevention programmes or on improving specialist services. Given all these uncertainties, it would be imprudent to implement SDQ-based screening programmes without extensive prior evaluation at pilot sites.
Clinical Implications and Limitations
CLINICAL IMPLICATIONS
-
▪ Strengths and Difficulties Questionnaires (SDQs) administered to multiple informants can identify around two-thirds of children and adolescents with psychiatric disorders in the community.
-
▪ SDQs are good at detecting conduct, hyperactivity, depressive and some anxiety disorders, but are poor at detecting specific phobias, separation anxiety and eating disorders.
-
▪ SDQs completed by parents and teachers are generally better predictors than SDQs completed by adolescents about themselves.
LIMITATIONS
-
▪ Improving the detection of child psychiatric disorders is not an end in itself — further studies need to determine if improved or earlier detection leads to better outcome.
-
▪ This study did not assess whether offering further assessments to the ‘false positives’ generated by SDQ screening would have caused distress to the children or their families.
-
▪ There was no economic evaluation.
eLetters
No eLetters have been published for this article.