The Journal of Dairy Research does not just aim to succeed, rather, it has success as an objective. If this sounds contradictory, it is because an objective is a tangible and definable outcome, whereas an aim is variously an action (to aim) or an object (the thing you are aiming at). As with much of the English language, there is muddle. Furthermore, aiming and missing does not mean that you did not aim! Has the Journal achieved success? For the second consecutive year our Impact Factor has increased, our community continues to grow and we have a very high rate of returning authors. Importantly, our submission rate has never been higher, whilst our Peer Review time has never been shorter. So yes, I would say that we have achieved our objectives and are succeeding. Are there things we could do better? Undoubtedly, but then that is the nature of success, it requires continuing improvement. Our next set of objectives include making Open Access publication more available without compromising our free-to-publish credentials, improving the services we offer to students and non-native English speaking researchers and increasing our collaboration with relevant international bodies such as the International Dairy Federation. Our overall objective is to combine quality (of research) and choice (of publication route). As part of that, I will include persuading our authors to have objectives rather than simply to aim and, if they insist on aiming, require that they aim high. By this, I mean that the objectives should preferably include the testing of a hypothesis through the design and implementation of an experiment or series of experiments. Recognizing that this is not always possible (for financial or ethical reasons, for instance), objectives that are restricted to the acquisition of data also find a place in the Journal, provided we judge that the data are novel and capable of having impact. In the last few months an inordinate amount of data have been collected on one topic, the SARS-CoV-2 virus or COVID19 disease. There is no real evidence yet of that data providing a workable solution to the pandemic, and there is growing evidence of poor-quality data leading to erroneous conclusions (Humer and Taylor, Reference Humer and Taylor2020). The approach taken by different countries has varied enormously, with decision making variously residing in the hands of politicians, health professionals or, arguably, the media. Regrettably, international transmission of the virus ran far ahead of international coordination of the response, and since a huge diversity of contradictory scientific opinion has been promulgated there is a serious risk that the public perception of science and scientists will suffer. The one fairly consistent feature has been to initially apply restrictions at population level rather than targeted to identifiable higher risk groups, a strategy that is now changing as the worse of the pandemic fades and preparations are made for a ‘second wave’. In short, we have ‘muddled-through’, and whilst the tragic impact of the virus on human deaths has been less than some predicted (and less than 10% of the total global communicable disease deaths, if official data are to be believed), its societal effects (collateral health consequences, economic impact, fear) are likely to be felt for many years to come. I am not suggesting that alternatives were available, deliberate infection to test hypothetical cures clearly being totally out of the question. Muddling-through is something that does have to be done in some circumstances and is regarded by some as a science in itself, especially when applied to issues such as economic and political theory (Lindblom, Reference Lindblom1959; Bendor, Reference Bendor1995). Others, however, have suggested that the approach has serious flaws, in relation to issues as diverse as disaster response (Gould, Reference Gould2020) and embryo protection (Fox and McGuinness, Reference Fox, McGuinness, Stanton, Devaney, Farrell and Mullock2015). I would suggest that collecting data for its own sake or in the hope that it will eventually lead to the resolution of a problem is akin to muddling-through, but in a knowledge-rich era it is increasingly the way that research is done. A new Journal dedicated to dairy science launched this year and, in an opening Editorial, Ametaj (Reference Ametaj2020) simultaneously espouses systems biology and the whole gamut of ‘omics’ as the future of lactation research, replacing the reductionist approach. Reductionism attempts to explain phenomena in terms of smaller and smaller entities, and I struggle with the concept that measuring every single miniscule entity in a biological sample in some way provides a non-reductionist approach. Our knowledge society enables us to generate massive amounts of data, but it is up to us to use that data in a way that provides actual benefit. We run the risk of allowing data to dictate the course of our research (Fig. 1, left) rather than regarding it as a component of the triumvirate (problem, data, idea) that should form the basis of a hypothesis to be tested (Fig. 1, right). For a specific example of the need for research that does actually test a hypothesis and to understand the inspiration for this Editorial, see Eric Hillerton's introduction to the mastitis cluster of research papers in this issue (Hillerton, Reference Hillerton2020). Meanwhile, send us your high quality objective-oriented research and we will publish it without charge to you, or if you prefer either you or your organization (through a Read and Publish arrangement) can pay for it to be available Gold Open Access. We offer quality and choice.
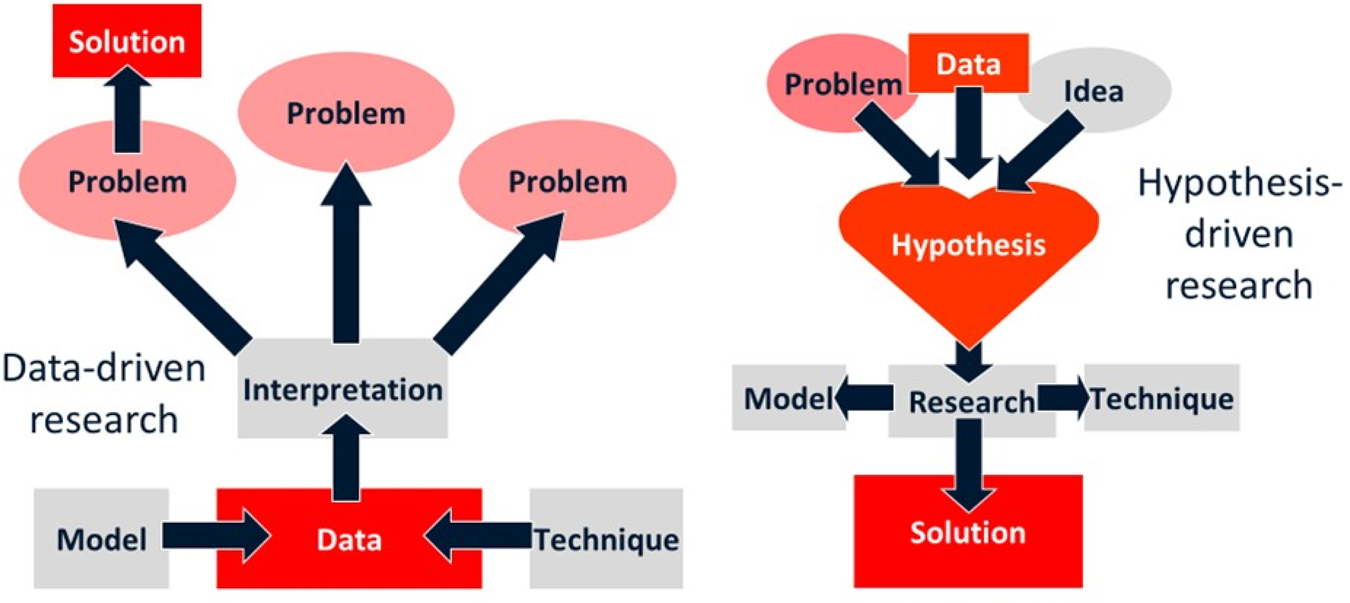
Fig. 1. Schematic of data-driven (left) and hypothesis-driven (right) approaches to research. The former focuses on our growing ability to measure, in the hope that solutions will arise by virtue of the knowledge acquired. The latter more classic approach recognizes a problem and uses a combination of data and thought to set a hypothesis that can be tested to arrive at a solution.