1. Introduction
The rise of the Internet has led to many changes in language use since it has both modified old texts varieties (such as advertisements and reviews) into completely new forms and led to the creation of completely new kinds of texts (such as discussion forums and Internet blogs). These text classes can be considered as registers, and they can be determined as situational uses of language (Biber, Reference Biber1988). The Internet is the largest source of linguistic data, mostly text (Johannessen and Guevara, Reference Johannessen and Guevara2011). The purpose of the study is to analyze the registers found on the entire Finnish Internet. To this end, we introduce the Finnish Corpus of Online Registers – FinCORE.
A Web corpus can be seen as a huge collection of texts collected mechanically from the Internet (Baroni and Bernardini, Reference Baroni and Bernardini2005; Baroni & Kilgarriff Reference Baroni and Kilgarriff2006; Kilgarriff Reference Kilgarriff2007). The different Web corpora are used in both language research and NLP (Natural Language Processing) (Kilgarriff & Grefenstette Reference Kilgarriff and Grefenstette2003). For instance, The COW (COrpora from the web) is a result of a project that has the goal of determining the value of linguistic material collected from the Internet (Schäfer Reference Schäfer2016) for fundamental linguistic research (Schäfer & Bildhauer Reference Schäfer and Bildhauer2012). OSCAR (Open Super-large Crawled ALMAnaCH Corpus), on the other hand, is a huge multilingual corpus obtained by language identification (Scheible et al., Reference Scheible, Thomczyk, Tippmann, Jaravine and Boeker2020; Suarez et al., Reference Suarez, Romary and Sagot2020), filtering of Common Crawl data without any metadata and intended to be used in the training of different language models for NLP (Suarez et al., Reference Suarez, Sagot and Romary2019).
Web corpora have many advantages: they are very extensive and contain a remarkable range of linguistic variation, which rarely ends up in manually collected material (Palander-Collin and Laippala, Reference Palander-Collin and Laippala2020). However, because of the computational collection method, Web corpora do not usually have information on the text registers. This substantially limits the use of the data, as register contextualizes the language use attested in the texts and provides a framework for understanding its linguistic characteristics (Biber, Reference Biber2012). This is also noted by Egbert et al. (Reference Egbert, Biber and Davies2015) who state that without systematic and clear register classification, Web corpora cannot be fully benefited from. From a technical perspective, register crucially affects the automatic processing of language (Mahajan et al., Reference Mahajan, Sharmistha and Roy2015; Van der Wees et al., Reference Van der Wees, Bisazza and Monz2018; Webber, Reference Webber2009). Thus, register information would greatly benefit from the use of Web corpora for the purposes of NLP, as well.
Automatic register identification would offer a solution for the lack of register information in Web corpora. The biggest challenge in this process has been the lack of annotated corpora representing the unrestricted range of registers found online. Because of this, we do not know what kinds of registers the Web would include, and it has not been possible to develop machine learning systems for automatic Web register identification on the entire, unrestricted Web. The same challenges concern the application of unsupervised machine learning methods such as clustering. Although clustering has been shown to provide linguistically motivated structure to large datasets (Biber and Egbert, Reference Biber and Egbert2018; Gries et al., Reference Gries, Newman and Shaoul2011), the composition of the resulting clusters would be very difficult to evaluate without register-annotated data as a point of comparison.
The Internet register or genre corpora used in many previous studies have usually consisted of a predetermined and restricted set of registers (e.g., Crowston et al., Reference Crowston, Kwasnik and Rubleske2011; Eissen and Stein, Reference Eissen and Stein2004; Pritsos and Stamatatos, Reference Pritsos and Stamatatos2018) that have been purposely selected to represent a particular, predetermined number of classes. Therefore, their results are not directly applicable to the unrestricted Web, which has a much wider range of linguistic variation than this kind of restricted data. The FinCORE corpus presented in this paper reflects the unrestricted Web. In addition to presenting the corpus and the registers in it, we demonstrate that an accurate register identification system can be trained on it.
In addition to the lack of corpora, another challenge associated with Web registers is caused by unclear register boundaries, agreement among annotators (see, e.g., Rosso and Haas, Reference Rosso and Haas2011; Crowston et al., Reference Crowston, Kwasnik and Rubleske2011) and hybrid text classes (see Rosso Reference Rosso2008, pp. 1062-1063, Biber and Egbert, Reference Biber and Egbert2018), which means that a text shares characteristics of several registers. We show in this paper that despite the wide range of variation, registers from the unrestricted Finnish Internet can be annotated reliably by well-trained annotators, and that the registers have sufficiently well-defined linguistic characteristics to enable their automatic identification, as well.
Registers on the unrestricted Web are already presented in English by the Corpus of Online Registers of English (CORE) which features the full range of registers found on the English-speaking, open Web (Egbert et al., Reference Egbert, Biber and Davies2015, Laippala et al., Reference Laippala, Rönnqvist, Oinonen, Kyröläinen, Salmela, Biber, Egbert and Pyysalo2022), and English Web registers have been studied in a number of other studies, as well (e.g., Asheghi et al., Reference Asheghi, Sharoff and Markert2016; Crowston et al., Reference Crowston, Kwasnik and Rubleske2011; Vidulin et al., Reference Vidulin, Lustrek and Gams2009). However, there is a lack of register-annotated Web register corpora in languages other than English. This is the case also for Finnish; we do not know what kind of registers the Finnish Internet contains and what characterizes them. This paper addresses these questions.
In summary, the three main objectives of this article are:
-
1. to introduce FinCORE and its collection principles. FinCORE is a 7.1 million word corpus of online registers of Finnish collected from the Internet and manually annotated for registers.
-
2. to present the FinCORE registers and their situational characteristics. FinCORE follows the register taxonomy established for the English CORE as closely as possible. This paper presents the decisions that have been made when adapting the scheme from the original English CORE to Finnish. Thereby, we examine to what extent the scheme is applicable to other languages and thus if registers are sufficiently similar across languages to allow for the use of a common scheme.
-
3. to show that the FinCORE registers are sufficiently well-defined linguistically to allow for their automatic identification. Sharoff et al. (Reference Sharoff, Wu and Markert2010) questioned whether online registers have sufficiently well-defined linguistic characteristics to allow for their reliable identification. Furthermore, Laippala et al. (Reference Laippala, Egbert, Biber and Kyröläinen2021) showed how online registers vary in terms of how well they can be identified.
Together with this article, we will also release the FinCORE annotations under a CC BY open license. The full FinCORE dataset is available for download at [github.com/TurkuNLP/FinCORE_full].
The rest of the article is organized as follows: we start by presenting previous studies on Web corpora and Web register identification in Section 2 and our research methods, experimental setup and data presentation in Section 3. Section 4 introduces the register classes of FinCORE, while in Section 5, we present the results of the register identification experiments as well as an error analysis. Section 6 concludes this paper.
2. Related work
In this section, we present previous research on Web corpora and automatic Web register identification. Studies on the identification of texts from the Internet have included both the terms genre and register. These terms, however, originate from different paradigms. The term genre is typically used in text identification studies (Santini et al., Reference Santini, Mehler and Sharoff2010; Petrenz and Webber, Reference Petrenz and Webber2011; Pritsos and Stamatatos, Reference Pritsos and Stamatatos2018; Sharoff, Reference Sharoff2018) and discourse analysis (e.g., Halliday, Reference Halliday1985; Miller, Reference Miller1984), while register is often used in text linguistic corpus studies (Biber et al., Reference Biber, Conrad and Reppen1998; Biber, Reference Biber2019). FinCORE follows the CORE register taxonomy, and this register approach to linguistic variation. Therefore, we apply also the term register, that, following Biber (Reference Biber1988) and Biber and Conrad (Reference Biber and Conrad2009), we define as text varieties that are associated with particular situations of use and communicative purposes.
2.1 Web corpora and registers
The three most recent large Web text collections with manual register or genre annotations for English are the Leeds Web Genre Corpus (LWGC) (Asheghi et al., Reference Asheghi, Sharoff and Markert2016), the 20-Genre Collection and the English CORE. The 20-Genre Collection (Vidulin et al., Reference Vidulin, Lustrek and Gams2009) corpus was compiled with the target of building a Web genre classifier that could annotate Web pages with genres within search engines. However, selected genres mostly form wide classes (e.g., informative) with addition of some specific genres that are of high interest for a user (e.g., FAQ) and genres that the user would like to filter out (e.g., error message). The LWGC identifies 15 genre classes collected via crowdsourcing (Asheghi et al., Reference Asheghi, Sharoff and Markert2016), but the genre classes feature only a selected set of genres frequently attested on the Web, and the texts have been manually selected to present these classes. Thus, the corpus does not cover the full range of language use attested on the unrestricted open Web, which restricts the application potential of the dataset for register identification. Egbert et al. (Reference Egbert, Biber and Davies2015) and the CORE corpus were the first to include all the texts and registers found on the unrestricted open Web. The English CORE corpus register taxonomy is hierarchical and developed in a data-driven manner. Specifically, the register scheme was created using first a decision-tree survey to annotate the situational characteristics of a sample of documents and then several rounds of pilot annotations to eventually form a comprehensive list of registers and subregisters to correspond to the sample and finally to the unrestricted Web (Egbert et al., Reference Egbert, Biber and Davies2015). Table 1 contrasts the CORE main registers and the classes used in LWGC and the 20-Genre Collection in order to give an overview of the corpus compositions. The detailed CORE register taxonomy is presented and compared to FinCORE in Section 3.
Table 1. Main registers in LWGC, CORE & 20-Genre collection
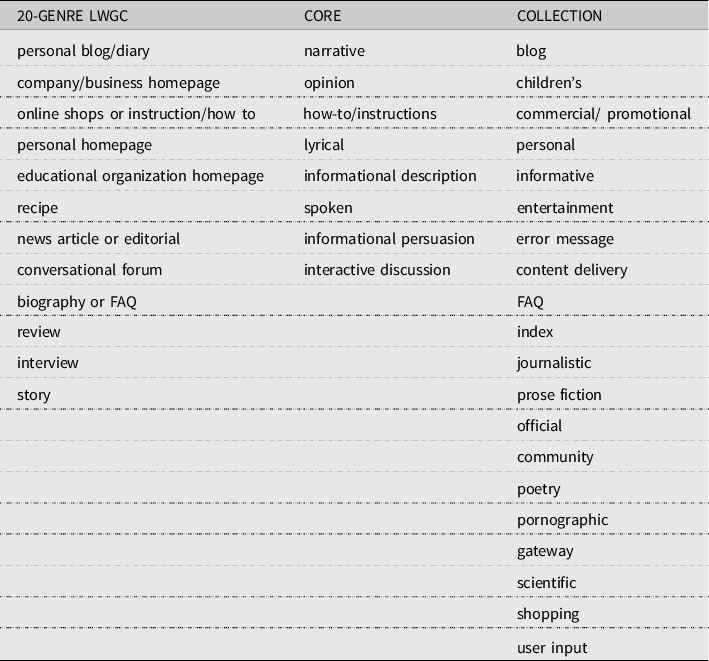
Operationalizing registers as discrete classes, where each text belongs to exactly one register class, does not necessarily suit Internet data very well, because many online texts can share multiple communicative purposes (Biber and Egbert, Reference Biber and Egbert2018; Biber et al., Reference Biber, Egbert and Keller2020). To solve this, the CORE corpus includes hybrid classes that combine characteristics of several registers (e.g., narrative + opinion) (see Biber and Egbert, Reference Biber and Egbert2018). Another solution is suggested by Sharoff (Reference Sharoff2018), who analyzes registers by describing texts based on proportions of dimensions, such as argumentative or hard news.
Prior to these most recent corpora, a number of other genre annotated corpora had been introduced. The Multilabeled Genre Collection (MGC) (Vidulin et al., Reference Vidulin, Lustrek and Gams2007) consists of Web pages classified into 20 genres collected by targeting Web pages in these genres, as well as using random Web pages and popular Web pages. Similar to the hybrid texts in CORE, MGC allows for texts to be categorized into several genre classes. KI-04 (Meyer zu Eissen and Stein, Reference Meyer zu Eissen and Stein2004) was annotated using a scheme developed based on a survey on useful genre classes. The KRYS I (Berninger et al., Reference Berninger, Nielsen, Abbott, Wijsman and Raskind2008) collection was annotated using 70 genres grouped into 10 sets, e.g., review and commentary. The SANTINIS (Santini, Reference Santini2007) corpus was annotated based on seven genres exclusive to the Web, e.g., blogs and FAQs. Finally, the Syracuse (Crowston et al., Reference Crowston, Kwasnik and Rubleske2011) collection consists of 3,027 Web pages annotated based on 292 genres.
A challenge with the existing genre-labeled collections is the reliability of the annotations. MGC, KRYS I, and I-EN-Sample have been double-annotated. However, agreement measures were under 60% (average percentage annotator agreement) for the part of the corpora that have been selected randomly from the Web, causing doubts about how well genres can be identified even by humans (Sharoff et al., Reference Sharoff, Wu and Markert2010). In the case of CORE, after the careful tuning of the annotation scheme, at least three of the four coders – recruited via MTurk – agreed on 69% of the documents (Egbert et al. (Reference Egbert, Biber and Davies2015). These results show that the development of the annotation scheme provides improvements to the agreement.
2.2 Automatic identification of Web registers using machine learning
Machine learning is a part of artificial intelligence, where algorithms can learn to execute tasks automatically by learning from data, without any specific rules or instructions. Automatic register identification is a typical text classification task, where the algorithm creates a classification model based on training data that consists of examples of texts, and their register classes that have been manually added. This model can then be used for identifying the register of new texts (Argamon, Reference Argamon2019).
Evaluation is an important aspect of machine learning, its goal being to develop methods that perform as well as possible. Importantly, the focus is on how well the methods generalize to new texts. Therefore, the model is evaluated on a set of texts that are not used in the training of the model. A validation set is used to optimize the hyperparameters. Typical evaluation metrics include precision, defined as the fraction of relevant instances among all the retrieved ones. For instance, precision could show how many of the documents identified as news articles actually belonged to this category. Recall is the fraction of retrieved instances among all the ones existing in the data. For instance, it could show the proportion of news articles the method was able to identify among all the news articles in the data. F1-score measures the balance of precision and recall. Its highest possible value is 1.0, and the lowest 0, if either the precision or the recall is zero. In a text classification task, F1-score is typically averaged from class-specific scores. Micro-average is counted by first calculating the sum of all true positives and false positives, over all the classes. Then the precision for the sums is computed. Macro-average is computed using the arithmetic, unweighted mean of all the per-class F1 scores. Accuracy is the fraction of predictions a classifier predicted correctly calculated by dividing the number of correct predictions by the total number of predictions.
Earlier studies on Web register identification focused on statistical machine learning methods such as support-vector machines (Boser et al., Reference Boser, Guyon and Vapnik1992). These methods are based on simple feature frequency data representations, such as bag-of-words or character n-grams. Using support-vector machines on the large LWGC, Asheghi et al. (Reference Asheghi, Markert and Sharoff2014) showed that online registers can be identified in a representative collection with an accuracy of 78.9% on 15 classes based on plain texts. However, as we mentioned above, LWGC represents only registers exclusive to the Web, and furthermore, the texts have been selected manually to represent the classes. This makes the task of register identification on LWGC easier than it would be on a corpus featuring the full range of texts found on the Web.
On the unrestricted Web, Biber and Egbert (Reference Biber and Egbert2016) applied stepwise discriminant analysis to classify CORE registers, achieving 34% precision and 40% recall. These results reflect the difficulty of register identification on the unrestricted Web; it is much more difficult than when applied to the restricted Web.
The best results on register/genre identification are currently obtained with neural networks (McCulloch and Pitts, Reference McCulloch and Pitts1943), as we will show below. One of the main advantages of neural networks is that they can benefit from information obtained from larger datasets than the training data used in the particular task. In a simple format, the information can be in word vectors produced with methods such as Word2vec (Mikolov et al., Reference Mikolov, Chen, Corrado and Dean2013) or FastText (Joulin et al., Reference Joulin, Grave, Bojanowski and Mikolov2017). These vectors are the result of a simple algorithm that takes as input running text from a very large corpus and generates vector representations for the corpus words. The task of the algorithm is to predict the linguistic context of the words, and, as semantically similar words share similar contexts (Firth, Reference Firth1957), semantically similar words get nearby vectors.
A convolutional neural network (CNN) is a type of a neural network that can benefit from word vectors. First, proposed for image processing (LeCun and Bengio, Reference Lecun and Bengio1995), they have been used in a wide range of NLP tasks, in particular in text classification (Severyn and Moschitti, Reference Severyn and Moschitti2015; Zhang et al., Reference Zhang, Zhang and Vo2015; Zhang and Wallace, Reference Zhang and Wallace2015). Laippala et al. (Reference Laippala, Kyllönen, Egbert, Biber and Pyysalo2019) showed that a CNN based on FastText vectors clearly outperforms the previous register identification results on CORE presented by Biber and Egbert (Reference Biber and Egbert2016).
The most recent, transformer-based language models such as the Bidirectional Encoder Representations from Transformers (BERT) (Devlin et al., Reference Devlin, Chang, Lee and Toutanova2018) have achieved great advances compared to architectures based on word vectors. One of the most important technical innovations (Vaswani et al., Reference Vaswani, Shazeer, Parmar, Uszkoreit, Jones, Gomez, Kaiser and Polosukhin2017) of BERT is bidirectional training, which allows the creation of context-sensitive representations. BERT is pretrained on a large corpus of unlabeled text, including Wikipedia and the Book Corpus consisting of 800 million words of free novel books. The original study introducing BERT (Devlin et al., Reference Devlin, Chang, Lee and Toutanova2018) addressed only English. A range of language-specific BERT models have since been created (De Vries et al., Reference De Vries, van Cranenburgh, Bisazza, Caselli, van Noord and Nissim2019, Martin et al., Reference Martin, Muller, Suárez, Dupont, Romary, de La Clergerie and Sagot2020, Virtanen et al., Reference Virtanen, Kanerva, Ilo, Luoma, Luotolahti, Salakoski, Ginter and Pyysalo2019, Kuratov & Arkhipov Reference Kuratov and Arkhipov2019). Using the English BERT, Laippala et al. (Reference Laippala, Rönnqvist, Oinonen, Kyröläinen, Salmela, Biber, Egbert and Pyysalo2022) achieved an F1-score of 68%, showing that register identification from the unrestricted Web can be done with a decent performance and highlighting the possibilities offered by BERT when compared to simpler methods.
The BERT architecture has also been applied to train multilingual models. The Multilingual BERT (Devlin et al., Reference Devlin, Chang, Lee and Toutanova2018) was trained using Wikipedia, while XLM-RoBERTa (XLM-R) (Conneau et al., Reference Conneau, Khandelwal, Goyal, Chaudhary, Wenzek, Guzman, Grave, Ott, Zettlemoyer and Stoyanov2020), trained using Web data, was claimed to be the first multilingual model to outperform monolingual ones (Conneau et al., Reference Conneau, Khandelwal, Goyal, Chaudhary, Wenzek, Guzman, Grave, Ott, Zettlemoyer and Stoyanov2020; Libovický et al., Reference Libovický, Rosa and Fraser2020; Tanase et al., Reference Tanase, Cercel and Chiru2020). Furthermore, Conneau et al. (Reference Conneau, Khandelwal, Goyal, Chaudhary, Wenzek, Guzman, Grave, Ott, Zettlemoyer and Stoyanov2020) showed that XLM-R provides strong performance improvements over earlier multilingual models such as mBERT. Similarly, Repo et al. (Reference Repo, Skantsi, Rönnqvist, Hellström, Oinonen, Salmela and Laippala2021) explored cross-lingual Web register identification using main registers from four register-annotated corpora and showed that the best results, 73.18 F1-score, were achieved with XLM-R compared to 58.04 for a CNN and 72.98 for FinBERT.
3. Research data and methods
In this section, we present first the annotation principles of FinCORE. Then, we discuss the experimental setup of register identification, especially the methods we apply to this end.
FinCORE consists of over 7 million words and 10,754 documents. Based on the learning curve presented by Laippala et al. (Reference Laippala, Rönnqvist, Oinonen, Kyröläinen, Salmela, Biber, Egbert and Pyysalo2022) for English, this size was estimated to be sufficient for the purposes of register identification. Similarly, many register studies operate on smaller datasets, suggesting that the size is sufficient for linguistic purposes as well.
FinCORE is based on a random sample of the Finnish Internet Parsebank, which is a mass-scale corpus of the Finnish Web developed and in detailed described by Luotolahti et al. (Reference Luotolahti, Kanerva, Laippala, Pyysalo and Ginter2015), and already used in a number of studies in both linguistics and NLP (Huumo et al., Reference T., A.-J., J., M. J., T., F., V., Milla, Penttilä and Viimaranta2017, Laippala et al., Reference Laippala, Kyröläinen, Kanerva and Ginter2018, Virtanen et al., Reference Virtanen, Kanerva, Ilo, Luoma, Luotolahti, Salakoski, Ginter and Pyysalo2019). The Parsebank has been compiled with two methods. First, a dedicated crawl was established to retrieve Finnish documents from the Web. To this end, seed URLs were selected from data with a language detection tool (https://github.com/CLD2Owners/cld2), and a Web crawl was performed by using these seeds. Second, Finnish documents were identified and retrieved from Common Crawl, an organization that crawls the Internet providing its archives and datasets for public use (https://commoncrawl.org/). Texts were cleaned from menus and listings with boilerplate removal, and deduplicated using Onion (https://corpus.tools/wiki/Onion).
3.1 Register taxonomy and annotation process of FinCORE
The FinCORE registers are annotated following the taxonomy of the English CORE (see Section 2.1). In order to better target the texts included in FinCORE, we made some minor modifications to the original CORE register scheme. Specifically, we did not use some subregister classes originally included in CORE, because they were extremely rare in Finnish, and we added some new classes in order to fully describe the registers found in the Finnish data (e.g., machine-translated/generated texts). In the English CORE, there is a subregister other under each main category that corresponds to texts that represent some other subregister than those listed in the taxonomy. Additionally, texts for which the CORE annotators could not agree on a subregister, were assigned only to the main register, such as narrative. In order to simplify the scheme and decrease the number of subregisters, we did not include the other classes in FinCORE, but annotated all texts in the main register if it clearly belonged to a certain main register, but not to any particular subregister.
These minor adjustments were made in an iterative manner based on the annotators’ remarks. If a text variety not denoted by any of the subregister labels was repeatedly found during the annotation, a new subregister label could be decided, and this label could be given to the documents retrospectively. On the other hand, if a register or subregister was found to be very infrequent after the first round of annotation, this register label could be deleted from the final labels. The final FinCORE scheme consists of nine main registers divided into 30 subregisters, as opposed to eight main and 39 subregisters in the original CORE.
The FinCORE and CORE register taxonomies are presented in Figure 1, with their differences highlighted. Overall, the fact that only minor changes to the original CORE scheme needed to be made indicate that the original CORE scheme can also be used for other languages and that the set of registers found on the English web corresponds relatively well to other languages, or at least to Finnish.

Figure 1. The left column shows the finCORE register taxonomy, while the right column shows the original CORE taxonomy. the differences between these two taxonomies are in bold.
In the narrative main register, one of the subregisters occurring in FinCORE did not have a dedicated class in the English CORE taxonomy, namely community blog. We introduce this in FinCORE to feature a blog written by, as the name implies, a community. In FinCORE, there is also a specific class job description under informational descriptions, while in the English CORE, there is no such separate class. In the English CORE, there are also two different FAQ classes, the other one under how-to/instructional texts and the other under informational descriptions. As FAQs are rare (See Table 5) in Finnish, it did not make sense to keep them separate, so they were combined into one subcategory in FinCORE, under the informational texts. The English CORE opinion main register contains subregisters that were not found in FinCORE, letter to the editor and advertisement. The English CORE also includes reader/viewer responses under interactive discussion main register, technical support under informational description, and persuasive essay under informational persuasion, but these registers are not included in FinCORE, as there were no such texts in the FinCORE sample. The English CORE lyrical main class includes song lyrics and prayer as subclasses, and the spoken main class transcript of video/audio and TV/movie script as subclasses. No such texts were, however, found in the Finnish data. Machine-translations does not exist at all in the English CORE, but it was added to FinCORE, because a large number of machine-translated texts were received during the annotation.
In the English CORE, each text was annotated by four coders using Mechanical Turk. In our study, the register annotation of the data was operated individually by annotators with a linguistics background. In addition to the document text resulting from the cleaning process described above, the annotators had access to the document url. If the document was still accessible, the annotators could visit the website in order to better interpret the register. We double-annotated the texts first, and when a sufficient level of agreement was found, we changed to single annotation. Nevertheless, difficult cases were always resolved in a group. The measured human inter-annotator agreement, counted prior to the discussions, was 79.66%. When annotators used only main registers, a 83.22% consensus was reached. Previous studies have found remarkably low inter-annotator agreement scores for register annotation (see Section 2.1). Therefore, the agreement scores achieved for FinCORE can be considered as satisfactory.
While the CORE hybrids were formed based on systematic disagreements between the annotators, the FinCORE hybrids were explicitly created by the individual annotators. This allowed the creation of hybrid texts even when a text was annotated by a single person. The annotation was done with a custom annotation tool, which provided annotators with a wide selection of flags to identify additional viewpoints of the texts, see Section 4.10.
3.2 Experimental setup in the classification task
The main goal of the register identification experiments is to show that the FinCORE registers are sufficiently clearly defined, and that the corpus provides a useful basis of register identification from the unrestricted Finnish Internet.
During these experiments, we use the train, development, and test set splits typically applied in machine learning (see Section 2.2). In other words, our data is randomly divided into train, test, and development sets using stratified sampling with a 70% (train)/10% (dev)/20% (test) split. Stratified random sampling is a method through which a sample group that best represents the entire data being studied, can be obtained, ensuring that each subgroup of interest – register classes in our case – is represented. We then train the models on the train set, make an investigation on the development data to find the best hyperparameters, and finally confirm the results on the test set.
We compare three state-of-the-art methods, all in a multi-label setting, where each document can have one or several independently assigned register labels. Following the propagated setting by Laippala et al. (Reference Laippala, Rönnqvist, Oinonen, Kyröläinen, Salmela, Biber, Egbert and Pyysalo2022), the main register labels are propagated, that is, repeated when a text has a subregister label. For instance, a text annotated as an opinion blog would receive both, the main register label opinion and the subregister label opinion blog.
As the first classifier, we modify the cross-lingual Convolutional Neural Network (CNN) used by Laippala et al. (Reference Laippala, Kyllönen, Egbert, Biber and Pyysalo2019) to a multi-label setting. These results are used as a baseline, as previous studies have already shown that more complex architectures, such as BERT, outperform CNN in register identification (Laippala et al., Reference Laippala, Rönnqvist, Oinonen, Kyröläinen, Salmela, Biber, Egbert and Pyysalo2022, Repo et al., Reference Repo, Skantsi, Rönnqvist, Hellström, Oinonen, Salmela and Laippala2021). Second, we use the Finnish monolingual FinBERT (Virtanen et al., Reference Virtanen, Kanerva, Ilo, Luoma, Luotolahti, Salakoski, Ginter and Pyysalo2019) available at https://huggingface.co/TurkuNLP/bert-base-finnish-cased-v1. Third, we apply XLM-R, which has outperformed both monolingual and multilingual models in previous studies (see Section 2.2).
We apply several hyperparameters in the training of these methods. Epoch indicates the number of passes of the entire training dataset the learning algorithm has completed. Increasing kernel size means increasing the total number of features. The model has a higher complexity to address a given problem, and it should perform better for a particular training set. Prediction threshold represents the probability of the classifier that the prediction is actually true. Grid-searching is the process of scanning the data to configure optimal parameters for a model. Learning rate controls the speed at which the model learns to adapt to a problem. Smaller learning rates require more training epochs, whereas higher learning rates require fewer and result in rapid changes. We perform a grid search on learning rates (9e–1e) and number of training epochs (3–7). For the CNN, we performed a search for the best parametres on the kernel size (1–2), learning rate (1e), and prediction threshold (0.4, 0.5, 0.6).
As evaluation metrics to measure the classifiers’ performances, we use F1, precision, and recall (see Section 2.2). During the training, we set as the learning target the micro-average of the F1-score. When optimizing the hyperparameters against the development set, we select the ones that provide the best micro-average F1-score. Instead of the macro-average that would focus on maximising the register class-specific performances, we select the micro-average as the main goal of the classification experiments in order to provide accurate register information for as many documents as possible in a large Web dataset.
4. Registers of fincore
In this section, we present the FinCORE registers, their frequencies, and the different additional tags that we applied during the annotation (see Section 3.1). Table 2 shows the frequencies of the main registers.
Table 2. Frequency of main register labels. note that due to the hybrid classes, some of the texts are classified into two different classes. finCORE consists of 10,754 texts total

As can be seen from the Table 2, narrative is by far the largest class. Informational description, machine-translated/generated text, opinion, informational persuasion are fairly evenly distributed in our data. How-to/instructions, interactive discussion, lyrical and spoken are clearly less frequent. Appendix 1 in the online supplementary material provides examples of all the registers. In this section, we present them in detail. In the English CORE, narrative is also the largest class, just as lyrical and spoken are clearly the two smallest classes (Biber and Egbert, Reference Biber and Egbert2016). The register distribution of CORE in terms of text count is otherwise very similar to FinCORE, with the difference that CORE lacks the machine-translated/generated class, as mentioned (see detailed description in Section 4.9).
The average text lengths for all main registers are presented in Table 3, which shows that, on average, interactive discussions, opinions and spoken texts tend to be longer than other texts, whereas how-to/instructions and informational persuasions tend to be numerically the shortest. Overall, the text lengths feature a wide range of variation, with the longest documents covering tens of thousands of words and the shortest only a couple of sentences.
Table 3. Average length (number of words) of finnish texts. the standard deviation total is 293

4.1 Narrative
The purpose of the main register narrative is to tell about events that have already happened, and they are typically aimed at an audience that does not have any prior special knowledge regarding the topics of the text.
Narrative texts are divided into eight subregisters presented below. Table 4 shows the frequency of these classes. The two clearly largest registers are news report/news blog and personal blog. Furthermore, community blog, magazine/online article, and sports report seem to be of roughly the same size. Community blogs were relatively frequent among the FinCORE texts, differing clearly from personal blog in terms of the author.
Table 4. Frequencies of categories in the narrative main register

Table 5. Frequencies of categories in the informational description main register

4.1.1 News report/news blog, Sports report, Magazine/online article
All these subregisters have the aim of narrating about events and being typically professionally edited and preplanned. Journalism is usually associated with four features: factuality, mass circulation, institutionalism, and topicality (the pursuit of timeliness) (Bruun et al., Reference Bruun, Koskimies and Tervonen1986). News only work as news at a certain point in a certain place: once it has lost its relevance, it no longer serves as journalism but only as an example of the journalistic product of its time (Bruun et al., Reference Bruun, Koskimies and Tervonen1986).
In addition to actual news reports, we have also annotated bulletins from, e.g., communities, and ministries under this register. As we discussed above, the publications are timebound and published as soon as possible after the described events. The texts are not assumed to be accompanied with opinionated expressions.
The writer of sports report is either a sports journalist or a sports expert, while the audience consists of readers interested in sports. The purpose of the text is to narrate sports events, describe athletes, or to do an analysis about a topic related to sports. Similar to news reports, it is assumed that these texts are not opinionated.
The goal of a magazine/online article is to entertain the reader (see Herkman, Reference Herkman2005; Suhola et al., Reference Suhola, Turunen and Varis2005). Magazine/online articles can cover, for example, some specific topic in detail, such as video game or aging. These texts can be distinguished from news report/news blog in terms of timeliness – whereas news reports are on recent events, magazine articles are less directly linked to the time of the writing. This difference can also traditionally be seen between printed newspapers and periodicals.
4.1.2 Personal blog, Community blog, Travel blog
All these blogs tell about events – either personal ones, events related to a community, or something about traveling. Typically, the bloggers both plan and edit the texts by themselves. For personal blogs, the posts can cover almost any topic, including parenthood, knitting or games. The audience is either interested in these topics or in the blogger. Personal blog is one of the registers that can be exclusively found online.
Community blog is annotated as a separate subregister only in FinCORE. The texts are very similar to personal blogs – the main difference being that they are typically written by several writers who represent communities, companies, or associations to whom the blog belongs. A text written by a politically active group or party is not a community blog but an opinion blog expressing political opinions.
The writers of travel blogs are travelers or experts of travel, while the audience of the blogs consists of individuals interested in either travel destinations or the travelers. The purpose of the text is to describe and write about travel destinations or to tell about the author’s journey in detail.
4.1.3 Historical article, Story
Historical articles narrate about historical and significant events, while all fictional texts fall under the story register. Stories are usually carefully preplanned but not necessarily professionally edited, and they can also be found on discussion forums, in which case they receive discussion forum as their first register (see Section 4.4) and story as their second register.
Texts that can be regarded as histories of societies, and associations are annotated as historical article. The writer can be, for example, a historian, or a blogger interested in history, and the audience are usually readers who are interested in historical topics.
4.2 Informational description (or explanation)
The purpose of the main register informational description is to describe, inform, or explain something in detail. Usually, the writer or writers are not indicated, and the production circumstances can vary from very carefully written (such as research articles) and technical (legal terms/conditions) to unedited and familiar (such as descriptions).
Table 5 shows the frequency of subregisters under the informational description main register. Description of a thing is clearly the most frequent, covering 32% of the texts. Job descriptions, research articles, course materials, and FAQs are rare, but the other subgregisters are approximately the same size.
4.2.1 Description of a thing, Description of a person
The purpose of these two subregisters is to describe – e.g., the contents of a course, a company, or a person. The scope of description of a thing is very wide, as it covers all the other descriptions except those describing a person. Descriptions of animals are annotated as description of a thing. Job applications, where the writer describes themself, are marked as description of a person.
4.2.2 Encyclopedia article, Research article, Course material
All encyclopedias, including Wikipedia, fall under the class of encyclopedia articles. The writers range from journalists to amateurs, while the writers of the texts in the research article register are researchers or students of a specific field of science. Encyclopedia articles describe and inform the reader about a topic. The difference between these and description of a thing (4.2.1) is in the more detailed level of description. Furthermore, the layout of Wikipedia articles is the same in all the languages, which makes them relatively easy to identify (Biber and Egbert, Reference Biber and Egbert2018).
Course material provides material to be studied, or produced on a course, while research article reports on a study. Importantly, research articles are not evaluated during the annotation; they are included in this category even if of low quality.
4.2.3 Information blog, Report
When a blog is informative and neutral, that is, not expressing the writer’s personal opinions, it is annotated as an information blog. Accordingly, the purpose is to inform the reader. The text can be based on scientific findings but also on the writer’s expertise on a specific field.
Report covers texts that describe events, such as meetings or public announcements, or conclude a series of events, such as the results of a research project. The writer is usually not mentioned, unless the text is a transcript written by a secretary.
4.2.4 FAQ, Legal terms/conditions, Job description
Frequently asked questions are nearly always directly followed by the answers. The texts discuss a product or a service sold on the website, and the answers are written by company employees.
Legal terms/conditions concern, e.g., sale transactions and terms of competitions. The writer is never mentioned. The texts are usually composed of lists and often include section signs () making the text structure easily identifiable.
Job descriptions are job advertisements on company websites or websites focused on job applications. The audience consists of job applicants or otherwise interested readers.
4.3 Opinion
The main register opinion expresses a writer’s or a group’s (such as a parliamentary group’s) opinion and, in some cases, gives background information on it. Religious texts are also annotated to this register. In some cases, the writer is mentioned by a pseudonym or by their actual name. According to Nieminen (Reference Nieminen2010), problems are encountered in describing the structure of opinion texts, as it can be difficult to find the elements of opinion in them (Nieminen, Reference Nieminen2010, pp. 213-214). Such factors can significantly complicate the annotation of texts in this class.
The opinion main register consists of five subregisters. Religious texts/sermons, reviews, and opinion blogs are the three largest ones, while advice and opinion are only represented a few times in the total, as shown in Table 6.
Table 6. Frequencies of categories in the opinion main register

4.3.1 Review, Opinion blog
Reviews can be written by one or multiple writers, and the topics range from video games and films to products and hotels. Websites containing only reviews of a product, but not directly selling it, are annotated as review, not as description with intent to sell (see 4.6); customer reviews on Amazon’s websites are good examples of this register. Opinion blogs are held by, e.g., political parties or politicians, who want to share their opinions publicly to attract potential voters. The texts express clear opinions.
4.3.2 Religious text/sermon, Advice
All confessional texts are annotated as religious text/sermon. Usually in FinCORE, the text is not skillfully edited, and it is targeted towards believers of a certain religious group. Neutral texts discussing religion are not annotated to this class.
Advice texts give advice to the reader, e.g., saving tips or advice on how to increase follower counts on social media, the focus being on the thoughts and feelings of the reader. Since advice belongs to the opinion main register, the texts must include the advice giver’s personal opinion or experience. The audience can be very varied. In FinCORE, horoscopes are also annotated under this class.
4.4 Interactive discussion; Discussion forum, Question-answer forum
Interactive discussion includes two subregisters, discussion forum, and question-answer forum. Table 7 shows that discussion forums cover more than two-thirds of all texts under this main class. Additionally, texts including interactive discussion but not belonging to a specific subregister are annotated under the main register only. These include, for example, nimenhuuto.com, a Web information channel designed for organizing the activities of sports teams, and texts consisting only of user-generated comments that would typically be found after blogs posts.
Table 7. Frequencies of categories in the interactive discussion the main register

The texts in discussion forums are interactive – the discussion starter participates in the discussion also after initializing it. In contrast, in question-answer forum, the person asking the question does not participate in the discussion afterwards. Writers in both subregisters are not professional, and they do not edit the text. Moreover, the texts are often very colloquial, and the writers go by a pseudonym. If they express their personal opinions in a clear manner, opinion (Section 4.3) is added as a secondary register.
4.5 How-to/instructions; Recipe
How-to/instructions contains only one subregister, recipe (4.5.1.). Texts annotated in the main register cover more than 90% of the texts (see Table 8). Among others, these include assembly instructions for furniture, driving instructions, and instructions for giving a presentation. Blogs receive the secondary register of how-to/instructions when they include clear instructions. Additionally, recipes occur frequently in personal blogs (Section 4.1.2) and magazine/online articles (Section 4.1.1). In both cases, the texts are annotated as recipe only.
Table 8. Frequencies of categories in the how-to/instructions main register

4.6 Informational persuasion; Description with intent to sell, News-opinion blog/editorial
Informational persuasion informs, and, at the same time, persuades the reader (see Biber and Egbert, Reference Biber and Egbert2018, p. 36). The persuasion can be related to marketing, selling, or even rationalizing one’s personal opinion to the reader. In FinCORE, the informational persuasion main register includes two subregisters: description with intent to sell and news-opinion blog/editorial. Descriptions with intent so sell form the large majority of texts within this class, as shown in Table 9.
Table 9. Frequencies of categories in the main register informational persuasion

In CORE, informational persuasion and its subregisters are very infrequent, forming only less than 2% of the texts. In FinCORE, however, this register is much more frequent, comprising 12% of the corpus. We assume that this difference is because of the different compilation methods of the corpora; Google searches, on which CORE is based, seem to retrieve fewer persuasive texts than what are retrieved by crawling.
Finally, according to Biber and Egbert (Reference Biber and Egbert2018: 38–39), texts within informational persuasion are not necessarily easy to identify. The distinction between purely persuasive texts and descriptive and persuasive texts is not evident in FinCORE either. We have annotated texts that have an underlying persuasive purpose but do not include any explicit elements of persuasion or marketing as informational persuasion, without any subregister label. Descriptive texts with explicit elements of persuasion are annotated as description with intent to sell.
Description with intent to sell targets simultaneously both description and persuasion. Unlike informational persuasion, these texts have explicit elements of persuasion. The texts are not usually professionally edited
Editorials and columns in professionally edited newspapers are annotated as news-opinion blog/editorial. Accordingly, columns published on a political party’s website do not belong to this class but to opinion blog (Section 4.3.1). The text must be supported by facts.
4.7 Lyrical; Poem
The main register lyrical contains only one subregister, poem. Poems or poetry websites can be easily identified due to their particular modes of expression. The writer’s name and identity often occur with the text. Poems can also occur as a part of personal blog (Section 4.1.2), in which case poem is marked as a secondary register.
4.8 Spoken; Interview, Formal speech
This main register covers all versions of originally spoken language (see Table 10). These are relatively rare in FinCORE (see Table 2).
Table 10. Frequencies of categories in the spoken main register

Interviews covers clearly speech-like texts with questions and answers – this makes them different, e.g., sports report (Section 4.1.1) even if both texts discussed sports. Similarly, formal speech is always written in a speech-like manner, covering, among others, parliamentary speeches.
4.9 Machine-translated/generated text
As these texts form a particular group that needs to be distinguished from texts written by humans, we wanted to annotate these as a class of their own despite the fact that they form a technical category rather than a register category. When the translation quality was high enough to enable the identification of the actual register, it was also annotated. While many machine-translated texts can nowadays be of high quality, the ones in FinCORE could be identified by lack of coherence and existence of grammatical errors.
4.10 Additional tags
Texts collected from the Web can present many kinds of features that can affect their processing. To include this information in the annotation, the annotators could describe the texts with eight additional tags.
Unsure is used if the annotator is not certain about the text register, indicating that it may be difficult to identify. Comments is added if the text, such as a personal blog or a news report/news blog, is followed by a large number of comments that cover the majority of the entire document. Missing text is used when a part of the text is missing. This can be due to the crawling process, but also, e.g., news websites front pages present only the beginnings of the texts. Foreign language is added if the text contains a significant part in some other language than Finnish. This is common in blog comment sections. Special characters is used to denote markings related to, e.g., coding. Generated text stands for a website’s metatext, such as instructions for the use of a website, for logging in, or for how to behave while visiting the website.
Untypical for register is added if a text presents, e.g., structurally or stylistically untypical characteristics – such as a text following the Wikipedia layout but containing personal opinions. Multiple texts is used when the text includes several separate pieces of text, for instance several beginnings of news articles and the hyperlink Continue reading.
Figure 2 shows the frequencies of the additional tags. Multiple text is the most frequent and comments the second most frequent – unsurprising considering the frequency of personal blogs in the corpus. Untypical for register and unsure have very similar frequencies, the remaining tags being less frequent.

Figure 2. Frequency of additional tags.
4.11 Hybrids
We established the following guidelines for annotating hybrids:
-
Encyclopedia articles are not annotated as hybrids unless the text follows a remarkably atypical format.
-
News-like texts simultaneously reporting future events, such as festivals and persuading readers to participate, are annotated as news report/news blog and informational persuasion.
-
Obituaries are annotated as description of a person and narrative.
-
Betting tips and gambling sites are annotated as sports report and description with intent to sell if the text purpose is clearly to sell a product. If the purpose is to give advice or to assess a certain game or match, the text is annotated as sports report and advice.
-
Role-playing games are annotated as discussion forum and story. Text-based role-playing games happen over text on discussion forums, and they are usually follow-up stories, in which the user continues to play based on the text of the previous user.
-
Reviews with clearly indicating product names and prices are annotated as review and description with intent to sell.
Table 11 presents the frequency of hybrids in FinCORE, and the examples of all the hybrids listed separately in this section can be found in Appendix 2 in the online supplementary material. The most frequent combination is formed by Machine-translated/generated texts and Review, the most likely because reviews are often machine translated. The second most frequent hybrid is News report/news blog and Description with intent to sell. Nowadays, advertisements may be added to news as part of an article. They may also contain surreptitious advertising. Similarly, News report/news blog and Informational persuasion often promote an event. Discussion forum and Story consist of forum discussions, that, in fact, are fictional texts, usually serial stories. Personal blog and Review and Personal blog and Recipe are results from reviews and recipes addressed to readers on personal blogs.
Table 11. Frequency of hybrids. hybrids that appeared less than five times in the data have been removed from the table. finCORE contains a total of 810 hybrids

5. Identification results and analysis
After having described the FinCORE registers in the previous section, we now focus on their automatic identification. First, we present the identification results achieved by the different classifiers, discuss the register-specific scores, and inspect the classification mistakes in order to gain insight into possible reasons behind the misclassifications. Registers may differ in terms of how well they are linguistically defined. This has an effect on how well they can be identified automatically, as shown in previous studies (Biber and Egbert, Reference Biber and Egbert2016; Reference Biber and Egbert2018; Laippala et al., Reference Laippala, Egbert, Biber and Kyröläinen2021). Thus, it is important to inspect the individual registers and how well each of them can be identified.
5.1 Classifier performances
Table 12 presents the results achieved by the three classifiers presented in Section 3.2. As can be seen, XLM-R achieved numerically the best performance, 79% micro F1-score, as opposed to the very similar 78% achieved by FinBERT. The CNN performance was clearly lower with a micro F1 of 60%. The same performance differences between the classifiers are shown in the macro F1-scores, although the scores are clearly lower. This indicates that in particular the smaller registers receive low identification rates.
Table 12. Identification results, averaged over three runs. data contains a total of 39 registers of which nine are main classes

The micro F1-score achieved by XLM-R indicates, that the FinCORE registers are well enough defined linguistically to allow for their automatic identification. Using a multilingual model trained on the English CORE and a smaller version of FinCORE, Repo et al. (Reference Repo, Skantsi, Rönnqvist, Hellström, Oinonen, Salmela and Laippala2021), achieved a micro F1-score of 73% on FinCORE. Furthermore, Laippala et al. (Reference Laippala, Rönnqvist, Oinonen, Kyröläinen, Salmela, Biber, Egbert and Pyysalo2022) reported a micro F1-score of 68% on the English CORE. These results are not directly comparable with ours as the applied register classes differ and as Repo et al., removed machine-translations, lyrical, spoken and hybrid classes from their data. However, they show that the full FinCORE allows for competitive register identification results.
5.2 Register-specific identification results
Table 13 shows the register-specific identification results with XLM-R. For the main registers, machine-translated/generated text received the highest identification scores. This is unsurprising considering that these texts are usually very different from those written by humans (see Appendix 1 in the online supplementary material for an example). Similarly, the identification scores were high for narrative. As narrative had the most examples in the training data, this was also expected. However, informational description is the second most frequent class, but its F1-score drops drastically compared to the previously mentioned. Interactive discussion, on the other hand, achieved high scores even with relatively few examples.
Table 13. 3. identification results on test data. main registers are in bold

For the subregisters, sports report received very high identification scores. Sports reports are often quite similar to each other in structure; they contain a lot of numbers (i.e., match results) and names (goal scorer, athlete, etc.) (see Appendix 1 in the online supplementary material). These characteristics make them simple for a human annotator to identify, and similarly, they seem to guarantee high identification scores with XLM-R.
Question-answer forums, research articles, and job descriptions received high F1-scores even if relatively infrequent in the training data. All these subregisters share characteristics that can contribute to their identification. Question-answer forums are usually composed of the question followed by an answer. Research articles are consistent with scientific writing, and job descriptions consist of the description of the position, salary, job title, the number of working hours, etc.
Similarly, reviews, discussion forums, and religious texts/sermons also received high identification scores. Religious text/sermon often contains a lot of repetitive quotations from the Bible (see Appendix 1 in the online supplementary material), while discussion forums are characterized by salient informal language. Review is consistently identified, but the reason behind this is more difficult to determine. Personal blogs, news reports, and descriptions with intent to sell were coherently identified – this can be explained by a high number of examples in the training data.
However, not all classes were coherently identified. Reports, FAQs, poems, course materials, advices, and informational blogs have only a few examples in the training data, which likely explains the low identification scores. However, the low number of examples in training data does not explain why magazine/online articles and community blogs received low identification scores. This variation can reflect the distinctiveness of the texts in these registers. Some registers have very well-defined characteristics that may lead to better identification scores, whereas some registers with wider inner variation may not be so well-defined (see Laippala et al., Reference Laippala, Egbert, Biber and Kyröläinen2021).
Finally, also news-opinion blogs/editorials received low identification scores. In addition to the low degree of distinctiveness, this may be due to their similarity with opinion blogs. This emerged already during the annotation. Editorials nearly always contain the author’s opinions, which sometimes makes the distinction between opinion blogs and news-opinion blogs/editorials blurry (see an example of misclassified text provided in the online supplementary material, Appendix 3).
5.3 Classifier’s prediction ability and mismatches
The classifier’s ability to identify the FinCORE registers is further illustrated in Figure 3 presenting a confusion matrix heatmap on the predictions of the XLM-R model. While the tables presented earlier in this section inform how well the register classes are predicted globally, Figure 3 gives further insight to the results by pointing to which classes the texts are actually predicted.

Figure 3. Confusion matrix heatmap presenting multiclass identification results. rows represent the true register classes and columns the predictions. numbers indicate the number of instances in the finCORE test data. hybrids are excluded for clarity.
As can be seen, when a text was misclassified, it was most often predicted to be a narrative – unsurprising considering that it is the largest class. Narrative texts were the most often predicted correctly. However, the most frequent misclassifications were predicted as informational description. This suggests that these registers could share some characteristics that might confuse the classifier. How-to/instructions were often misclassified as informational description.
These emerging misclassifications provide important information about the linguistic characteristics of the FinCORE registers (see Appendix 3 in the online supplementary material). A misclassification may take place when the classifier makes a mistake, but also when a text has linguistic features of another register. This can happen, as the basis of the register is the situational context, not its linguistic characteristics. Furthermore, some texts could be simple for a human to annotate, for instance based on the metadata surrounding the text on the Web page (if the site was still accessible during the annotation and the annotator visited it). The metadata, in turn, is not seen by the classifier, which can make the classification harder in some situations (see Appendix 3 in the online supplementary material, where the metadata has probably indicated to the human-annotator that the text should be labeled as an editorial).
6. Discussion and conclusions
The aim of this article was to introduce the FinCORE corpus and to present the registers of FinCORE, as well as to show, that they are sufficiently well-defined to allow for their automatic identification using machine learning.
As the first contribution, we released the full FinCORE corpus under a CC BY open licence. We explained the detailed solutions we made in terms of the FinCORE register taxonomy and how they compare to the English CORE, for which the taxonomy was originally developed. Second, we showed that this taxonomy allowed for a decent inter-annotator agreement, 80% prior to any discussions between the annotators. Thus, while many previous studies (Crowston et al., Reference Crowston, Kwasnik and Rubleske2011; Sharoff et al., Reference Sharoff, Wu and Markert2010) have indicated that web registers can be difficult to annotate due to their extreme variation and unclear boundaries, our results support the findings by Egbert et al. (Reference Egbert, Biber and Davies2015) showing that decent agreements can be reached with a well-defined scheme. While there is also a lack of data with manual annotations on the registers in languages other than English, FinCORE successfully contributes to filling this particular gap, as well.
Third, for the automatic register identification, we applied three machine learning methods: FinBERT, XLM-R, and CNN. Our results showed that the classifiers’ performance is sufficient for identifying registers from Finnish Web data. The highest performance was achieved by the XLM-R model with an F1-score of 79%. The analysis of register-specific classification showed that machine-translated/generated text was the best identified register class, but sports reports were also consistently identified by the classifier. High identification scores for individual registers, such as religious texts, reviews, discussion forums, question-answer forums, descriptions with intent to sell, and personal blogs, indicated that these registers are linguistically well-defined and possible to distinguish from the other registers. Together with the inter-annotator agreement of 80%, these results also support the application of the CORE scheme for Finnish.
The eventual goal of our study was to provide an overall linguistic description of register variation on the Finnish Internet. With over 10,000 texts reliably annotated for register information and the detailed descriptions of the registers presented in Section 4, FinCORE and our study allow for novel possibilities for both linguistics and NLP. Furthermore, the Web register identification results we presented in Section 5 offer promising avenues for challenging the lack of register information in Web corpora, in Finnish but also in other languages. As we showed, it is possible to develop machine learning systems for automatic Web register identification on the entire, unrestricted Web - especially now that we know what kinds of registers the Finnish Internet includes.
Supplementary material
For supplementary material accompanying this paper visit https://doi.org/10.1017/S0332586523000021
Acknowledgements
We thank for the financial support of the Emil Aaltonen Foundation. We also wish to acknowledge CSC - IT Center for Science, Finland, for computational resources.