Pre-diabetes is an intermediate phase preceding type 2 diabetes mellitus (T2DM), where glucose tolerance, fasting blood glucose and/or glycated haemoglobin (HbA1c) are impaired but not yet within the diabetic range(Reference Heianza, Arase and Fujihara1). Research efforts to date have primarily focused on interventions targeting patients with T2DM, owing to the growing burden on primary healthcare resources, and associated comorbidities including cardiovascular disease, kidney diseases, ocular dysfunction and mental illness(Reference Iglay, Hannachi and Joseph Howie2). However, evidence suggests that initiating treatment in pre-diabetes, where patients are earlier and less advanced in the diabetic disease pathway, may result in faster clinical improvements that are maintained long term(Reference Tuso3–Reference Chakkalakal, Galaviz and Sathish6). Pre-diabetes therefore represents a critical period for early intervention, reducing the risk of developing T2DM and associated healthcare costs(Reference Tabák, Herder and Rathmann7). While lifestyle forms a key part of this, diet and exercise interventions that target pre-diabetes are currently resource intensive, challenging to maintain over time and fail to address significant barriers to behaviour change experienced by those with pre-diabetes(Reference Barry, Roberts and Finer8,Reference Cheah, Goh and Zheng9) . There is therefore a pressing need to develop novel, scalable, cost-effective and targeted interventions.
Emerging evidence suggests that the gut microbiome and its metabolites represent a promising target for pre-diabetes interventions, playing a key role in modulating glycaemic, metabolic and inflammatory biomarkers(Reference Gurung, Li and You10–Reference Orly, Anastasia and Michal14). One of the key ways in which the gut microbiome is thought to exert these benefits is via microbial fermentation of dietary fibre in the colon, which typically results in the production of short-chain fatty acids (SCFA)(Reference Zhao, Zhang and Ding15,Reference Portincasa, Bonfrate and Vacca16) . SCFA, including butyrate, propionate and acetate, influence host health by maintaining intestinal barrier integrity(Reference Roediger17,Reference Roediger18) , modulating inflammation(Reference Soliman, Smith and Houdek19) and altering gene expression patterns in host tissue(Reference Boffa, Vidali and Mann20–Reference Davie22). In this manner, SCFA can regulate the expression of pro- and anti-inflammatory cytokines and influence glucose homeostasis and insulin sensitivity(Reference Barrera, Sandoval and D’Alessio23–Reference Khan, Nieuwdorp and Bäckhed26). As an important modulator of SCFA production, recent work has studied the effects of prebiotic fibres on glycaemic status, insulin sensitivity and inflammatory biomarkers in pre-diabetes. Many of these interventions have focused on single-ingredient supplements including resistant starch, resistant dextrin, fructo-oligosaccharides and oligofructose-enriched inulin(Reference Peterson, Beyl and Marlatt27–Reference Mitchell, Davy and Ponder32). While results are promising, it is clear that there are vastly different responses to interventions, with considerable individual variability(Reference Colantonio, Werner and Brown33). A possible source of underlying variability is the differences in microbial fibre fermentation capabilities(Reference Gurry, Nguyen and Yu34). In this sense, the degree of efficacy from a prebiotic fibre supplement is related to how well it will be fermented by specific microbiota to produce SCFA.
To overcome these constraints, studies conducted by us(Reference Gurry, Nguyen and Yu34) and others(Reference Zhao, Zhang and Ding15,Reference Frias, Lee and Carter35) have shown that exposure to a broad range of prebiotic fibres can compensate for inter-individual variability in microbial fermentation abilities. A diversified fibre supplement could therefore lead to improved clinical outcomes. Independent of microbiome-mediated effects, dietary fibres with viscous physicochemical properties (e.g. arabinoxylan and β-glucan) are well-known for their role in reducing acute postprandial glucose and insulin responses(Reference Zurbau, Noronha and Khan36). Interventions that leverage both prebiotic and postprandial glycaemic benefits represent a dual-pronged approach, acting via non-overlapping yet complementary mechanisms of action. In this study, we evaluated the effect of using a diverse prebiotic fibre supplement on metabolic, lipid and inflammatory biomarkers in patients with pre-diabetes.
Methods
Study design
This study was a parallel arm, individually randomised, single-blinded, placebo-controlled clinical trial with a 1:1 treatment allocation ratio. The study was approved by Berkshire B National Health Service Human Research Ethics Committee in the UK (reference: 22/SC/0363). The study was registered on ClinicalTrials.gov (registration number: NCT05593926). Written informed consent was obtained from all participants in accordance with the Declaration of Helsinki.
Study population
Eligible participants were men and postmenopausal women with a primary diagnosis of pre-diabetes but not receiving treatment for type 2 diabetes, with baseline HbA1c within the range of 5·8 % (40 mmol/mol) to 6·5 % (48 mmol/mol), who were willing to complete study requirements and had access to a smartphone or computer. The main exclusion criteria were participants receiving medication to treat type 1 or type 2 diabetes in the previous 6 months and current participation in a weight loss programme or planned in the next 16 weeks. Detailed eligibility criteria are available in Online Supplementary Note 1.
Study intervention
Eligible participants who met the eligibility criteria were randomised using a web-based system (Sealed Envelope) in a ratio of 1:1 to either the treatment arm of 20 g/d of a diverse fibre supplement (Online Supplementary Note 2 and Supplementary Table 1) or the placebo arm of 2 g/d of cellulose, both unflavoured powders. Previous work has demonstrated that prebiotic doses of approximately 10–20 g are well tolerated by participants(Reference Aliasgharzadeh, Dehghan and Gargari28,Reference Mitchell, Davy and Ponder32,Reference Frias, Lee and Carter35,Reference Broekaert, Cloetens and Courtin37) . The specific fibre blend used in this study contains 56 % prebiotic fibre (11·2 g) and 44 % viscous/low-fermentable fibres (8·8 g) (20 g in total). In this trial, we opted for the higher end of this range to yield more information about patient tolerance, adherence and side effects, as well as observing a clear signal in primary and secondary outcomes. The supplements were provided to the participants in single-serve sachets and were taken at any time of the day with water or mixed into food or drink of their choice (e.g. breakfast cereal, tea or coffee). To monitor adherence, participants received a daily text message, asking them to confirm that they had taken the supplement. If they failed to consume the supplement on any given day, they were prompted to provide a reason. Participants were advised to consume the intervention every day for 16 weeks. While our primary endpoint was assessed at week 16, we also included an exploratory endpoint at week 24. Participants were advised to continue taking the intervention daily until their final study visit at week 24.
Study assessments
Participants attended clinic appointments at baseline, week 16 and week 24 after 10–12 h of overnight fasting. They had blood pressure measurements, a fasting blood test and an Oral Glucose Tolerance Test for Insulin Sensitivity (ISI-OGTT) performed at baseline, week 16 and week 24. For the ISI-OGTT (baseline and week 16 only), blood samples were taken at −15, 0, 30, 60, 90 and 120 min for the measurement of plasma glucose and insulin concentrations. From blood samples, cytokines were measured by using a cytokine multiplexing assay from Meso Scale Discovery. This assay measures IL-6, IL-8, IL-10 and TNF-α together in the same well of a plate. The full lipid profile, high-sensitivity C-reactive protein (CRP), plasma glucose and HbA1c were all measured on an automated chemistry analyser (Siemens Advia 1800). Plasma insulin was measured on an automated immunoassay analyser (Siemens Advia Centaur XP). The insulin sensitivity index from the ISI-OGTT was calculated according to the equation derived by Matsuda and DeFronzo(Reference Matsuda and DeFronzo38) in which insulin sensitivity is estimated by dividing a constant (10 000) by the square root of the product of fasting blood glucose times, fasting plasma insulin (FPI) times, mean glucose times and mean insulin times. ISI-OGTT = 10·000/(FBG × FPI)(MG × MI). The index is strongly correlated with insulin sensitivity derived using the euglycaemic clamp technique(Reference Matsuda and DeFronzo38).
Primary and secondary endpoints
The primary endpoint was the change in HbA1c from baseline to week 16. Key secondary endpoints included changes in HbA1c from baseline to 24 weeks, insulin sensitivity (measured using ISI-OGTT) from baseline to week 16, and FPI, blood lipids (cholesterol (total, LDL-cholesterol, HDL-cholesterol), triglycerides), inflammatory biomarkers (IL-6, IL-8, IL-10, CRP and TNF-α) and diastolic and systolic blood pressure from baseline to 16 and 24 weeks. Previous work has shown that the extent of improvements observed from prebiotic supplementation could, in part, be explained by differences in baseline glycaemic status(Reference Mitchell, Davy and Ponder32,Reference Ďásková, Modos and Krbcová39) . As such, we also performed a sub-group analysis which included the effects of baseline HbA1c levels on the primary endpoint. Upon observing the data, approximately half of the participants had baseline HbA1c levels below 6·0 %, and thus, 6·0 % was used as the natural cut-off point for our sub-group analyses.
Tolerability and safety
Participants were asked to record whether they experienced any side effects or medical events since starting the intervention, recorded via online surveys at the end of weeks 1–4, and on a monthly basis for the remainder of the trial. If they answered yes, adverse events were documented, reported and reviewed for relatedness and expectedness within 24 h. Discontinuations were captured and presented in Fig. 1.
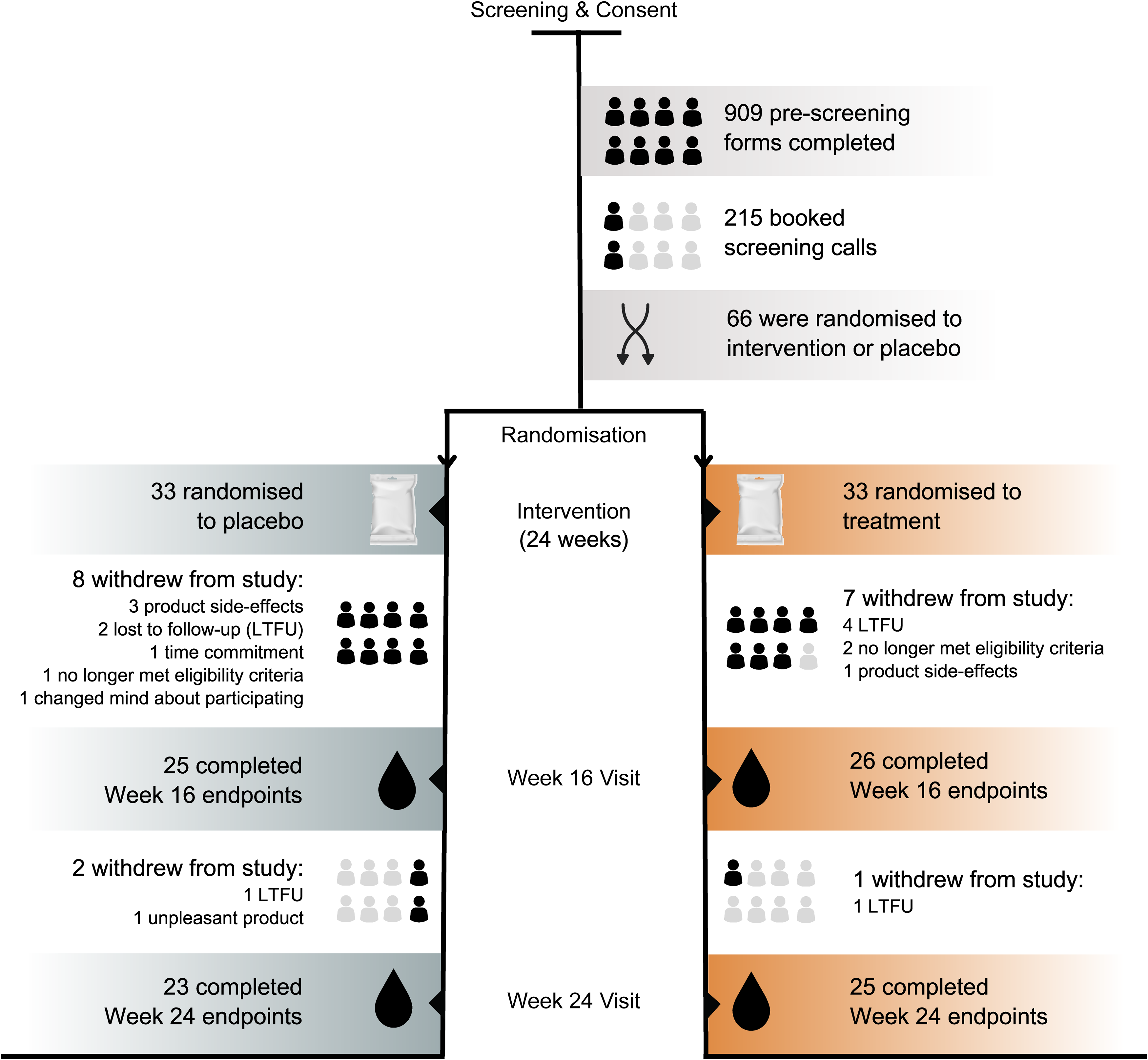
Fig 1. Clinical trial flow.
Statistical analysis
Data were analysed using R software. Statistical analyses were performed based on an intention-to-treat protocol. For the primary endpoint (HbA1c) and ISI-OGTT, an unpaired t test was used to compare the change from baseline to 16 weeks between the treatment and placebo. Two-way repeated-measures ANOVA with one within-subject factor (time) and one between-subject factor (randomisation) was used to compare changes in secondary endpoints from baseline to week 16, baseline to week 24 and week 16–24. Post hoc paired t tests (Bonferroni corrected) were performed on significant interaction effects to compare differences between and within groups over time. For non-normally distributed outcome variables, robust two-way ANOVA with trimmed means was used, and Wilcoxon signed-rank tests were used for non-parametric post hoc tests. Effects with P < 0·05 were considered statistically significant. As a pilot study, our sample size was informed from previous studies assessing fibre-based interventions in pre-diabetes and T2DM. Specifically, when using an observed effect size of 0·61 % (original units) from a study using a fibre supplement in T2DM(Reference Zhao, Zhang and Ding15), we determined that with a mean HbA1c value of 6·1 ± 0·4 %, a sample size of fourteen would be required to detect significance (assuming 80 % power and 0·05 alpha). However, given that our study was performed in a pre-diabetes population, as opposed to T2DM, we were less confident in our ability to determine accurate effect sizes from prior work. Therefore, we opted for a more conservative study size of 60. This sample size is consistent with previous studies assessing differences in HbA1c in a pre-diabetes cohort(Reference Zhao, Zhang and Ding15,Reference Peterson, Beyl and Marlatt27,Reference Guess, Dornhorst and Oliver40) . This also allows us to determine effect sizes that could be used to power subsequent studies appropriately. In addition, a sample size of sixty would enable us to investigate statistically meaningful differences in HbA1c in a sub-group analysis.
Results
Baseline patient characteristics
The study was conducted between 17 November 2022 and 9 October 2023. In total, 215 participants were screened, and sixty-six participants were randomised (thirty-three treatment and thirty-three placebo) (Fig. 1). The baseline characteristics were not significantly different across groups, with the exception of FPI and triglycerides, which were not matched variables for inclusion or randomisation (Table 1). A total of fifty-one and forty-eight participants completed the week 16 and week 24 visits, respectively. The various reasons for participant withdrawals are presented in Fig. 1.
Table 1. Baseline demographic and clinical characteristics
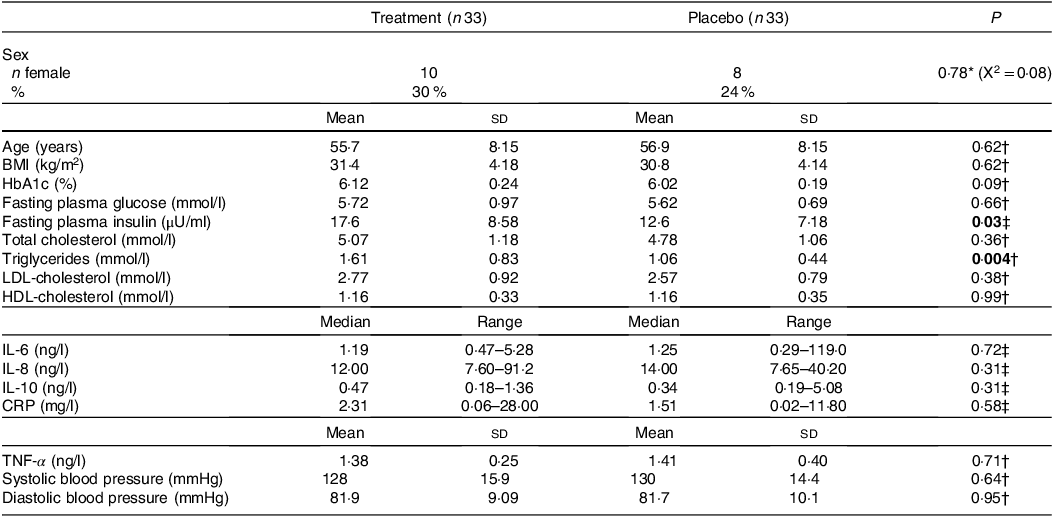
HbA1c, glycated Hb; CRP, C-reactive protein.
Mean ± sd; median (range).
P <0.05 are represented in bold.
* Pearson’s χ 2 test.
† Parametric t test.
‡ Wilcoxon signed-rank test.
Tolerability and safety
The intervention was well tolerated, with only two participants in the treatment group withdrawing from the study due to disliking the taste of the product. An additional three participants in the placebo group withdrew from the study due to product-related adverse events or not tolerating the product. There were no severe adverse events related to the treatment or placebo interventions. Adverse events reported were consistent with those typically seen in fibre-based interventions, including bloating, loose stools and abdominal pain (Online Supplementary Table 2).
Adherence
There was no statistical difference in adherence between the treatment and placebo arms (F 1,49 = 1·19, P = 0·28). From baseline to week 16, average adherence was 89·3 ± 2·54 % for the treatment group and 82·9 ± 3·79 % for the placebo (mean ± sd). However, from week 16 to the exploratory endpoint at week 24, we observed a significant decrease in adherence over time in both the treatment (–8·33 ± 7·83 %; t 26 = 2·70, P = 0·01) and placebo groups (–6·79 ± 6·82 %; t 26 = 2·24, P = 0·03) (Fig. 2(a)).
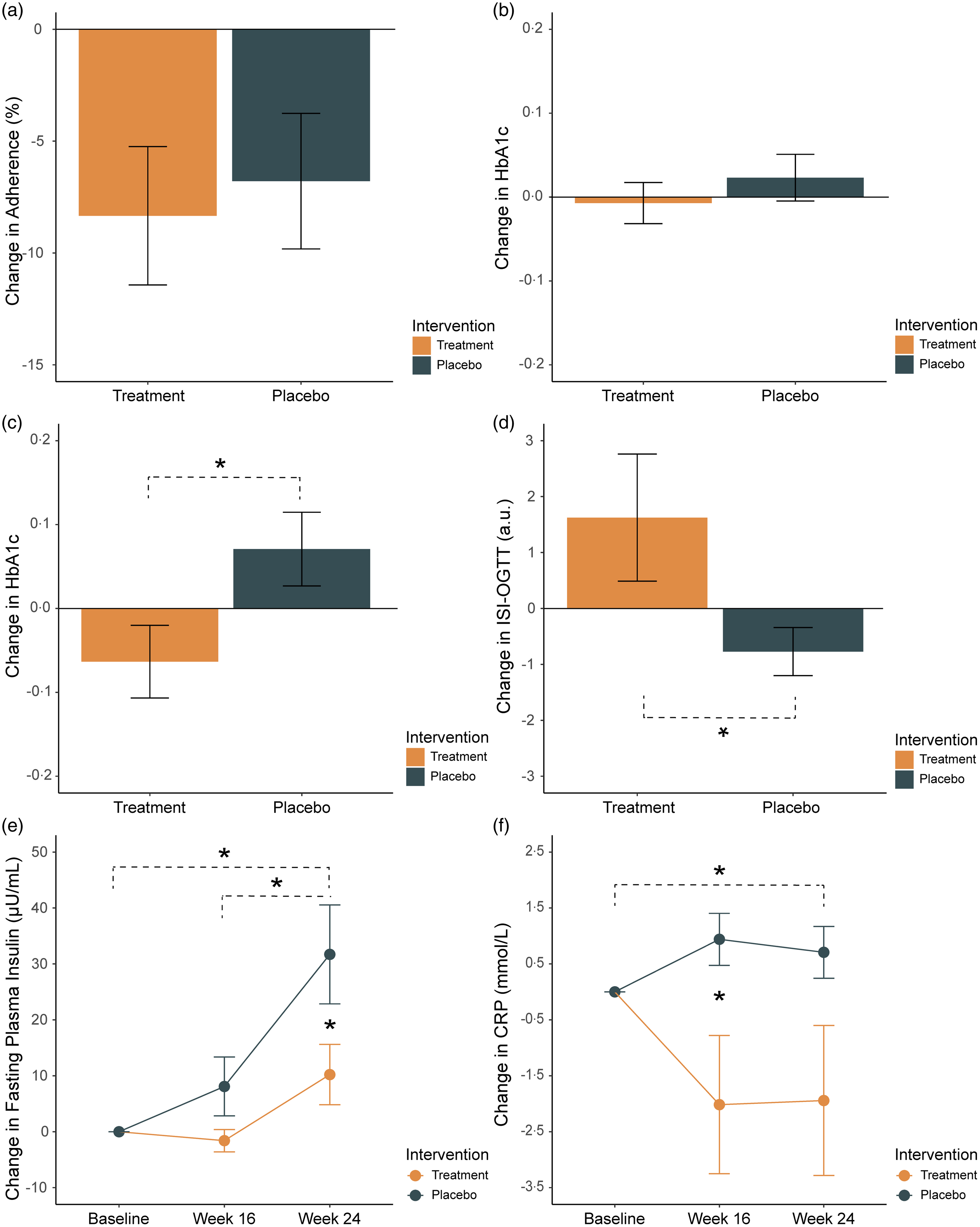
Fig 2. Change from baseline to week 16 in (a) adherence (%), (b) glycated Hb (HbA1c) (%), (c) HbA1c in a sub-group of participants with baseline HbA1c levels < 6·0 % and (d) insulin sensitivity (Oral Glucose Tolerance Test for Insulin Sensitivity (ISI-OGTT)) (a.u.) in the treatment (orange) and placebo (green) groups. (e) Change from baseline to weeks 16 and 24 for (b) fasting plasma insulin (µU/ml) and (c) C-reactive protein (CRP) mmol/l. Mean and standard errors are shown. * indicates P < 0·05.
Primary endpoint
The change in HbA1c from baseline to week 16 was not significantly different between the two groups (P = 0·37; treatment −0·02 ± 0·27 % v. placebo +0·04 ± 0·25 %, mean ± sd) (Fig. 2(b)). In participants with a baseline HbA1c < 6·0 % (i.e. earlier in the diabetic process), there was a significant reduction in HbA1c in the treatment group compared with the placebo (P = 0·05; treatment −0·17 ± 0·27 v. placebo 0·07 ± 0·29, n 23, mean ± sd) (Fig. 2(c)). This result was not observed in participants with baseline HbA1c ≥ 6·0 % (P = 0·48; treatment 0·06 ± 0·23 % v. placebo 0·001 ± 0·20 %, n 28, mean ± sd).
Secondary endpoints
Glycaemic outcomes
At week 16, insulin sensitivity, assessed via the ISI-OGTT, was significantly higher in the treatment group compared with the placebo (P = 0·03; treatment 1·62 ± 5·79 v. placebo −0·77 ± 2·11, mean ± sd) (Fig. 2(d)). For FPI, results of a two-way repeated-measures ANOVA showed a significant main effect of group (F 1,46 = 5·12, P = 0·03), time (F 2,92 = 13·31, P < 0·001) and interaction effects (F 2,92 = 3·07, P = 0·05) (Fig. 2(e)). In the placebo group, post hoc paired t tests (Bonferroni corrected) showed a significant change from baseline to week 24 (t 22 = −3·51, P FWE = 0·01) and week 16 to week 24 (t 22 = −2·94, P = 0·04). At week 24, the change in FPI in the placebo group was significantly higher compared with the treatment (t 12·6 = −2·02, P FWE = 0·05). There were no significant pairwise differences in FPI between baseline, week 16 and week 24 for the treatment group.
Inflammatory cytokines
For CRP, results of a robust two-way repeated-measures ANOVA using trimmed means showed a significant main effect of group (t = 6·37, P = 0·02) and an interaction effect (t = 6·85, P = 0·05) (Fig. 2(f)). Pairwise post hoc tests revealed that CRP was significantly lower at week 16 in the treatment group compared with placebo (W = 194, P FWE = 0·03). In the placebo group, there was a significant change in CRP from baseline to week 24 (W = 168, P FWE = 0·05). For TNF-α, there was a main effect of time (F 2,92 = 7·83, P = 0·001). No differences were observed for IL-6, IL-8 or IL-10 (Online Supplementary Fig. 1).
Lipid profiles
Results from two-way repeated-measures ANOVA in LDL-cholesterol and HDL-cholesterol showed a significant main effect of time (LDL-cholesterol, F 2,92 = 32·8, P < 0·001, and HDL-cholesterol, F 2,92 = 7·54, P = 0·001) (Online Supplementary Fig. 1). No significant differences were observed for total cholesterol or triglycerides.
Blood pressure
No significant differences were observed for systolic or diastolic blood pressure across groups (Online Supplementary Fig. 1).
Discussion
In this study, we showed a significant change in glucose metabolism and inflammatory responses in patients with pre-diabetes following a diverse prebiotic fibre intervention. Our primary outcome, the change in HbA1c from baseline to week 16, was not significantly different between groups (Fig. 2(b)). However, in participants with lower baseline HbA1c levels (< 6 %), we observed significant improvements in the treatment group compared with the placebo (Fig. 2(c)). Consistent with these results, a recent study in pre-diabetes found that improvements in glycaemic outcomes in response to inulin supplementation could be predicted by better baseline glycaemic status(Reference Ďásková, Modos and Krbcová39). This has been supported in previous work, showing that supplementation with inulin improved outcomes in a pre-diabetes population with lower baseline HbA1c values (5·4 ± 0·1 %, mean ± sd)(Reference Mitchell, Davy and Ponder32), while another study using 4 g oat β-glucan found no effects on HbA1c or secondary outcomes in a pre-diabetes population with higher baseline values (HbA1c 6·4 ± 0·4 %, mean ± sd)(Reference Christine, Fiona and Julian41). These studies are consistent with our results, showing that patients who are earlier in the pre-diabetic disease pathway may be more likely to respond metabolically to prebiotic fibre interventions. One study presents a possible explanation for this, highlighting that pre-diabetes individuals with HbA1c levels marginally below the threshold for T2DM (between 6·4 % and 6·5 %) face the highest risk of comorbidities, specifically adverse cardiovascular events(Reference Yahyavi, Snorgaard and Knop42). In this study, authors propose that in the absence of a T2DM diagnosis, this population is less likely to receive timely medical intervention, despite their HbA1c status being only marginally better than that of individuals with T2DM(Reference Yahyavi, Snorgaard and Knop42). While there are fewer studies on pre-diabetes, there are a number of studies on T2DM that show significant improvements in HbA1c following prebiotic interventions(Reference Zhao, Zhang and Ding15,Reference Colantonio, Werner and Brown33,Reference Frias, Lee and Carter35,Reference Dehghan, Pourghassem Gargari and Asghari Jafar-Abadi43) . However, the majority of patients in these studies were on stable anti-diabetic medication. The efficacy of prebiotic fibre interventions on HbA1c may therefore vary depending on the individual’s baseline glycaemic status, existing comorbidities and medication use.
While HbA1c remains a cornerstone for diabetes management, it may not fully capture early metabolic disturbances in pre-diabetes which can sometimes precede significant changes in HbA1c(Reference Varvel, Voros and Thiselton44). Our study suggests that secondary outcomes including insulin sensitivity and CRP may represent sensitive biomarkers to track responses to prebiotic fibre in a pre-diabetes population. Consistent with previous work, our data show that insulin sensitivity improves following prebiotic fibre intake(Reference Guess, Dornhorst and Oliver40,Reference Robertson, Wright and Loizon45) (Fig. 2(d)), although a number of studies have not observed this trend(Reference Mitchell, Davy and Ponder32,Reference Canfora, van der Beek and Hermes46–Reference Costa, França and Fonseca48) . We also observed a significant difference in fasting insulin at week 24 between groups, which appeared to be driven by significant increases over time in the placebo group (Fig. 2(e)). In the treatment group, increases in insulin sensitivity with unchanged fasting insulin levels may be attributed to improved insulin signalling pathways or increased responsiveness of insulin receptors in target tissues such as muscle, liver and adipose tissue(Reference Robertson, Wright and Loizon45). Unchanged fasting insulin levels alongside increased insulin sensitivity may also indicate altered glucose dynamics, including a reduction in hepatic glucose output or increased glucose uptake by peripheral tissues(Reference Thorburn, Muir and Proietto49). While not significant, an important consideration is that fasting insulin levels appear to slightly increase in the treatment group too, suggesting that the prebiotic treatment may have attenuated or slowed the progression of hyperinsulinaemia in unmedicated pre-diabetes patients. It is also important to note that the treatment group had significantly higher fasting insulin levels at baseline compared with the placebo group which may have influenced the rate of change over time. Our results should therefore be interpreted with caution.
Elevated levels of inflammatory cytokines are thought to be key contributors to β-cell impairment and insulin resistance(Reference Shoelson, Lee and Goldfine50,Reference Tsalamandris, Antonopoulos and Oikonomou51) . Our results showed a significant difference in CRP at week 16 between groups, supporting a potential link between prebiotic fibre, inflammation and metabolic health (Fig. 2(f)). CRP is recognised as an independent risk factor for cardiovascular disease(Reference Abdelmouttaleb, Danchin and Ilardo52,Reference Ridker, Glynn and Hennekens53) and more recently has been linked to an increased risk of later development of diabetes(Reference Pradhan, Manson and Rifai54,Reference King, Mainous and Buchanan55) . The reduction in CRP in the treatment group is consistent with a meta-analysis of fourteen randomised controlled trials showing a small (–0·37 mg/l) but significant reduction in circulating CRP after a dietary fibre or fibre-rich food intervention(Reference Jiao, Xu and Zhang56). In this current study, we observed a much larger reduction in CRP of –2·02 mg/l at week 16 (Fig. 2(f)).
In this study, the treatment had no effect on total cholesterol, triglycerides or HDL-cholesterol, and in fact, we observed increases in LDL-cholesterol in both the treatment and placebo groups (Online Supplementary Fig. 1). Given that participants were not currently medicated and were not advised to change their diet or lifestyle over the course of the intervention, it is plausible that their condition may naturally worsen over time. Thus, the increases in LDL-cholesterol that we observed over time may be attributed to the lack of efficacious intervention. Together, this demonstrates that the improvements in HbA1c, insulin sensitivity and CRP observed in this study are unlikely to be modulated by any changes in lipid profiles.
In our study, we used a diverse prebiotic fibre supplement which accounts for previously described inter-individual differences in microbial fermentation capabilities(Reference Gurry, Nguyen and Yu34). While beyond the scope of this investigation, a possible mechanism involves prebiotic fibre acting on metabolic and inflammatory outcomes via SCFA production. Locally, SCFA play a role in maintaining intestinal barrier integrity by acting as the dominant energy source for colonocytes(Reference Roediger17,Reference Roediger18) , promoting mucous production(Reference Pelaseyed, Bergström and Gustafsson57) and regulating the secretion of IL(Reference Soliman, Smith and Houdek19), all of which reduce intestinal inflammation. SCFA also act as histone deacetylase inhibitors and therefore alter gene expression patterns in host tissue(Reference Boffa, Vidali and Mann20–Reference Davie22). In this manner, SCFA influence the expression of pro- and anti-inflammatory cytokines, which further regulates both local and systemic inflammation. This is particularly relevant in pre-diabetes where the control and regulation of inflammatory cytokines are likely to play a role in the advancement of insulin resistance and type 2 diabetes(Reference Shoelson, Lee and Goldfine50,Reference Spranger, Kroke and Möhlig58,Reference Garcia, Feve and Ferré59) . Additionally, the SCFA butyrate promotes glucagon-like peptide 1 secretion in the colonic epithelium (via FFAR2/3 receptors) and intestinal gluconeogenesis, both of which may indirectly promote glucose homeostasis and insulin sensitivity(Reference Barrera, Sandoval and D’Alessio23,Reference Mazibuko, Muller and Joubert24,Reference De Vadder, Kovatcheva-Datchary and Goncalves60) . As this emerging field continues to develop, the importance of combining blood-based biomarkers with SCFA measurements and gut microbiome profiles will be critical to extend this work. Currently, meaningful quantification of SCFA from patient samples remains a challenge, particularly in the context of clinical trials.
The strengths of our investigation include the rigorous placebo-controlled randomised trial design, with high tolerability to the intervention, and no serious adverse events were reported. However, several caveats need to be considered. As a pilot study, our sample size is relatively small. Future studies will require larger sample sizes to confirm our findings. Another consideration is that for the placebo, we used 2 g of cellulose given that it is considered to have very little impact on microbial fermentation activities(Reference Wijnands, Appel and Hollanders61). However, adaptations of the gut microbiota over time have also been reported following cellulose ingestion, albeit with highly variable responses between individuals(Reference Gurry, Dannenberg and Finlayson62,Reference Tremaroli and Bäckhed63) . Future work should be carried out to understand the physicochemical properties of dietary fibres and their relationship to glucose homeostasis. As opposed to the more common choice of maltodextrin, the use of cellulose as a placebo could be considered a more conservative choice, potentially resulting in an underestimation of the quantitative impact of our prebiotic fibre supplement on HbA1c and insulin sensitivity. Due to the differences in intervention sizes (2 g v. 20 g for placebo and treatment, respectively), the study coordinators scheduled the participants’ study visits at different times/sessions. This was to avoid the risk of participants comparing experiences or sachet sizes in the waiting room. The study coordinators also needed to send the participants the intervention via post and would be able to see the difference in sachet size. It was therefore operationally impractical to conduct the study as a double-blind randomised controlled trial. The findings from this work will need to be replicated in a double-blinded study design to confirm our results. There were no significant differences in BMI between groups at baseline, nor did we anticipate that the prebiotic treatment would have significant impacts on BMI at week 16. However, we cannot rule out the possibility that changes in body weight throughout the intervention may have influenced the outcome measures. To substantiate the findings in this study, future work should assess weight changes across the trial period and include BMI as a confounding variable in the analysis of primary and secondary outcomes. Future extensions of this work should also include the collection of stool and plasma samples to link the observed glycaemic improvements with gut microbiota and SCFA changes. It is also important to note that at our exploratory endpoint at week 24, we did not observe a continued improvement in HbA1c, in the lower baseline HbA1c sub-group or the full cohort. This result may, in part, be related to the fact that adherence decreased from weeks 16 to 24 in both groups (Fig. 2(a)). This result suggests that ongoing high adherence is required to ensure long-term efficacy. From a practical perspective, ensuring that the supplement can be easily integrated into a patient’s existing daily routine will be important to determine the extent of improvements experienced by prebiotic fibre interventions.
Conclusion
Around 10–15 % of pre-diabetics will progress to diabetes annually(Reference Tabák, Herder and Rathmann7). Here, we showed that a diverse prebiotic fibre supplement improved metabolic and inflammatory biomarkers over a 16-week intervention. Critically, our results showed that participants with lower baseline HbA1c levels experienced greater improvements in HbA1c. These results provide initial indications that earlier stages of pre-diabetes may offer a window of opportunity to target diabetes-related biomarkers before they progress further. The broader improvements in insulin sensitivity, fasting insulin and CRP across the whole cohort also indicate potentially beneficial changes that warrant further exploration. Larger and adequately powered clinical interventional studies will be necessary to confirm these findings and explore the broader changes beyond HbA1c that impact longer-term disease progression and other health outcomes.
Acknowledgements
N/A
This project was sponsored and funded by Myota GmbH.
Conceptualisation and methodology, C. V. H., J. L. T. and T. G. Formal analysis: C. V. H. and T. G. Data curation: J. L. T. Resources, T. G. Writing—original draft, review and editing: all authors. Funding acquisition: T. G. Supervision: T. G. and M. S.
C. V. H. and T. G. are employees/shareholders of Myota GmbH.
Supplementary material
For supplementary material(s) referred to in this article, please visit https://doi.org/10.1017/S0007114524000904.