Impact statement
More than half of the world’s adult population suffers from hypertension (HTN), which is the single largest risk factor for human mortality. Despite available medications, susceptibility to develop HTN has not decreased because current knowledge on the risk assessment for susceptibility is severely limited. In this context, genome-wide association studies for HTN are factoring the genetic contributions toward the development of a polygenic risk score (PRS) for HTN. However, given that nongenetic factors also contribute to the etiology of HTN, PRS alone may be insufficient to account for the incidence and progression of HTN. One such nongenetic factor is gut microbiota, which is acquired at birth and demonstrated to be a definitive link to the etiology of HTN. Therefore, here we discuss the prospects for developing and integrating a microbiota-based ‘metagenomic risk score’ with PRS, and a clinical risk score to construct an artificial intelligence-based model for precision diagnosis and management of HTN.
Introduction
Despite improvements in health care, cardiovascular disease (CVD) remains the leading cause of human mortality globally (Vos et al., Reference Vos, Lim, Abbafati, Abbas, Abbasi, Abbasifard, Abbasi-Kangevari, Abbastabar, Abd-Allah, Abdelalim, Abdollahi, Abdollahpour, Abolhassani, Aboyans, Abrams, Abreu, Abrigo, Abu-Raddad, Abushouk, Acebedo, Ackerman, Adabi, Adamu, Adebayo, Adekanmbi, Adelson, Adetokunboh, Adham, Afshari, Afshin, Agardh, Agarwal, Agesa, Aghaali, Aghamir, Agrawal, Ahmad, Ahmadi, Ahmadi, Ahmadieh, Ahmadpour, Akalu, Akinyemi, Akinyemiju, Akombi, al-Aly, Alam, Alam, Alam, Alam, Alanzi, Albertson, Alcalde-Rabanal, Alema, Ali, Ali, Alicandro, Alijanzadeh, Alinia, Alipour, Aljunid, Alla, Allebeck, Almasi-Hashiani, Alonso, al-Raddadi, Altirkawi, Alvis-Guzman, Alvis-Zakzuk, Amini, Amini-Rarani, Aminorroaya, Amiri, Amit, Amugsi, Amul, Anderlini, Andrei, Andrei, Anjomshoa, Ansari, Ansari, Ansari-Moghaddam, Antonio, Antony, Antriyandarti, Anvari, Anwer, Arabloo, Arab-Zozani, Aravkin, Ariani, Ärnlöv, Aryal, Arzani, Asadi-Aliabadi, Asadi-Pooya, Asghari, Ashbaugh, Atnafu, Atre, Ausloos, Ausloos, Ayala Quintanilla, Ayano, Ayanore, Aynalem, Azari, Azarian, Azene, Babaee, Badawi, Bagherzadeh, Bakhshaei, Bakhtiari, Balakrishnan, Balalla, Balassyano, Banach, Banik, Bannick, Bante, Baraki, Barboza, Barker-Collo, Barthelemy, Barua, Barzegar, Basu, Baune, Bayati, Bazmandegan, Bedi, Beghi, Béjot, Bello, Bender, Bennett, Bennitt, Bensenor, Benziger, Berhe, Bernabe, Bertolacci, Bhageerathy, Bhala, Bhandari, Bhardwaj, Bhattacharyya, Bhutta, Bibi, Biehl, Bikbov, Bin Sayeed, Biondi, Birihane, Bisanzio, Bisignano, Biswas, Bohlouli, Bohluli, Bolla, Boloor, Boon-Dooley, Borges, Borzì, Bourne, Brady, Brauer, Brayne, Breitborde, Brenner, Briant, Briggs, Briko, Britton, Bryazka, Buchbinder, Bumgarner, Busse, Butt, Caetano dos Santos, Cámera, Campos-Nonato, Car, Cárdenas, Carreras, Carrero, Carvalho, Castaldelli-Maia, Castañeda-Orjuela, Castelpietra, Castle, Castro, Catalá-López, Causey, Cederroth, Cercy, Cerin, Chandan, Chang, Charlson, Chattu, Chaturvedi, Chimed-Ochir, Chin, Cho, Christensen, Chu, Chung, Cicuttini, Ciobanu, Cirillo, Collins, Compton, Conti, Cortesi, Costa, Cousin, Cowden, Cowie, Cromwell, Cross, Crowe, Cruz, Cunningham, Dahlawi, Damiani, Dandona, Dandona, Darwesh, Daryani, das, das Gupta, das Neves, Dávila-Cervantes, Davletov, de Leo, Dean, DeCleene, Deen, Degenhardt, Dellavalle, Demeke, Demsie, Denova-Gutiérrez, Dereje, Dervenis, Desai, Desalew, Dessie, Dharmaratne, Dhungana, Dianatinasab, Diaz, Dibaji Forooshani, Dingels, Dirac, Djalalinia, do, Dokova, Dorostkar, Doshi, Doshmangir, Douiri, Doxey, Driscoll, Dunachie, Duncan, Duraes, Eagan, Ebrahimi Kalan, Edvardsson, Ehrlich, el Nahas, el Sayed, el Tantawi, Elbarazi, Elgendy, Elhabashy, el-Jaafary, Elyazar, Emamian, Emmons-Bell, Erskine, Eshrati, Eskandarieh, Esmaeilnejad, Esmaeilzadeh, Esteghamati, Estep, Etemadi, Etisso, Farahmand, Faraj, Fareed, Faridnia, Farinha, Farioli, Faro, Faruque, Farzadfar, Fattahi, Fazlzadeh, Feigin, Feldman, Fereshtehnejad, Fernandes, Ferrari, Ferreira, Filip, Fischer, Fisher, Fitzgerald, Flohr, Flor, Foigt, Folayan, Force, Fornari, Foroutan, Fox, Freitas, Fu, Fukumoto, Furtado, Gad, Gakidou, Galles, Gallus, Gamkrelidze, Garcia-Basteiro, Gardner, Geberemariyam, Gebrehiwot, Gebremedhin, Gebreslassie, Gershberg Hayoon, Gething, Ghadimi, Ghadiri, Ghafourifard, Ghajar, Ghamari, Ghashghaee, Ghiasvand, Ghith, Gholamian, Gilani, Gill, Gitimoghaddam, Giussani, Goli, Gomez, Gopalani, Gorini, Gorman, Gottlich, Goudarzi, Goulart, Goulart, Grada, Grivna, Grosso, Gubari, Gugnani, Guimaraes, Guimarães, Guled, Guo, Guo, Gupta, Haagsma, Haddock, Hafezi-Nejad, Hafiz, Hagins, Haile, Hall, Halvaei, Hamadeh, Hamagharib Abdullah, Hamilton, Han, Han, Hankey, Haro, Harvey, Hasaballah, Hasanzadeh, Hashemian, Hassanipour, Hassankhani, Havmoeller, Hay, Hay, Hayat, Heidari, Heidari, Heidari-Soureshjani, Hendrie, Henrikson, Henry, Herteliu, Heydarpour, Hird, Hoek, Hole, Holla, Hoogar, Hosgood, Hosseinzadeh, Hostiuc, Hostiuc, Househ, Hoy, Hsairi, Hsieh, Hu, Huda, Hugo, Huynh, Hwang, Iannucci, Ibitoye, Ikuta, Ilesanmi, Ilic, Ilic, Inbaraj, Ippolito, Irvani, Islam, Islam, Islam, Islami, Iso, Ivers, Iwu, Iyamu, Jaafari, Jacobsen, Jadidi-Niaragh, Jafari, Jafarinia, Jahagirdar, Jahani, Jahanmehr, Jakovljevic, Jalali, Jalilian, James, Janjani, Janodia, Jayatilleke, Jeemon, Jenabi, Jha, Jha, Ji, Jia, John, John-Akinola, Johnson, Johnson, Jonas, Joo, Joshi, Jozwiak, Jürisson, Kabir, Kabir, Kalani, Kalani, Kalankesh, Kalhor, Kamiab, Kanchan, Karami Matin, Karch, Karim, Karimi, Kassa, Kassebaum, Katikireddi, Kawakami, Kayode, Keddie, Keller, Kereselidze, Khafaie, Khalid, Khan, Khatab, Khater, Khatib, Khayamzadeh, Khodayari, Khundkar, Kianipour, Kieling, Kim, Kim, Kim, Kimokoti, Kisa, Kisa, Kissimova-Skarbek, Kivimäki, Kneib, Knudsen, Kocarnik, Kolola, Kopec, Kosen, Koul, Koyanagi, Kravchenko, Krishan, Krohn, Kuate Defo, Kucuk Bicer, Kumar, Kumar, Kumar, Kumar, Kumaresh, Kurmi, Kusuma, Kyu, la Vecchia, Lacey, Lal, Lalloo, Lam, Lami, Landires, Lang, Lansingh, Larson, Larsson, Lasrado, Lassi, Lau, Lavados, Lazarus, Ledesma, Lee, Lee, LeGrand, Leigh, Leonardi, Lescinsky, Leung, Levi, Lewington, Li, Lim, Lin, Lin, Linehan, Linn, Liu, Liu, Liu, Looker, Lopez, Lopukhov, Lorkowski, Lotufo, Lucas, Lugo, Lunevicius, Lyons, Ma, MacLachlan, Maddison, Maddison, Madotto, Mahasha, Mai, Majeed, Maled, Maleki, Malekzadeh, Malta, Mamun, Manafi, Manafi, Manguerra, Mansouri, Mansournia, Mantilla Herrera, Maravilla, Marks, Martins-Melo, Martopullo, Masoumi, Massano, Massenburg, Mathur, Maulik, McAlinden, McGrath, McKee, Mehndiratta, Mehri, Mehta, Meitei, Memiah, Mendoza, Menezes, Mengesha, Mengesha, Mereke, Meretoja, Meretoja, Mestrovic, Miazgowski, Miazgowski, Michalek, Mihretie, Miller, Mills, Mirica, Mirrakhimov, Mirzaei, Mirzaei, Mirzaei-Alavijeh, Misganaw, Mithra, Moazen, Moghadaszadeh, Mohamadi, Mohammad, Mohammad, Mohammad Gholi Mezerji, Mohammadian-Hafshejani, Mohammadifard, Mohammadpourhodki, Mohammed, Mokdad, Molokhia, Momen, Monasta, Mondello, Mooney, Moosazadeh, Moradi, Moradi, Moradi-Lakeh, Moradzadeh, Moraga, Morales, Morawska, Moreno Velásquez, Morgado-da-Costa, Morrison, Mosser, Mouodi, Mousavi, Mousavi Khaneghah, Mueller, Munro, Muriithi, Musa, Muthupandian, Naderi, Nagarajan, Nagel, Naghshtabrizi, Nair, Nandi, Nangia, Nansseu, Nayak, Nazari, Negoi, Negoi, Netsere, Ngunjiri, Nguyen, Nguyen, Nguyen, Nguyen, Nichols, Nigatu, Nigatu, Nikbakhsh, Nixon, Nnaji, Nomura, Norrving, Noubiap, Nowak, Nunez-Samudio, Oţoiu, Oancea, Odell, Ogbo, Oh, Okunga, Oladnabi, Olagunju, Olusanya, Olusanya, Oluwasanu, Omar Bali, Omer, Ong, Onwujekwe, Orji, Orpana, Ortiz, Ostroff, Otstavnov, Otstavnov, Øverland, Owolabi, P A, Padubidri, Pakhare, Palladino, Pana, Panda-Jonas, Pandey, Park, Parmar, Pasupula, Patel, Paternina-Caicedo, Pathak, Pathak, Patten, Patton, Paudel, Pazoki Toroudi, Peden, Pennini, Pepito, Peprah, Pereira, Pereira, Perico, Pham, Phillips, Pigott, Pilgrim, Pilz, Pirsaheb, Plana-Ripoll, Plass, Pokhrel, Polibin, Polinder, Polkinghorne, Postma, Pourjafar, Pourmalek, Pourmirza Kalhori, Pourshams, Poznańska, Prada, Prakash, Pribadi, Pupillo, Quazi Syed, Rabiee, Rabiee, Radfar, Rafiee, Rafiei, Raggi, Rahimi-Movaghar, Rahman, Rajabpour-Sanati, Rajati, Ramezanzadeh, Ranabhat, Rao, Rao, Rasella, Rastogi, Rathi, Rawaf, Rawaf, Rawal, Razo, Redford, Reiner, Reinig, Reitsma, Remuzzi, Renjith, Renzaho, Resnikoff, Rezaei, Rezai, Rezapour, Rhinehart, Riahi, Ribeiro, Ribeiro, Ribeiro, Rickard, Roberts, Roberts, Robinson, Roever, Rolfe, Ronfani, Roshandel, Roth, Rubagotti, Rumisha, Sabour, Sachdev, Saddik, Sadeghi, Sadeghi, Saeidi, Safi, Safiri, Sagar, Sahebkar, Sahraian, Sajadi, Salahshoor, Salamati, Salehi Zahabi, Salem, Salem, Salimzadeh, Salomon, Salz, Samad, Samy, Sanabria, Santomauro, Santos, Santos, Santric-Milicevic, Saraswathy, Sarmiento-Suárez, Sarrafzadegan, Sartorius, Sarveazad, Sathian, Sathish, Sattin, Sbarra, Schaeffer, Schiavolin, Schmidt, Schutte, Schwebel, Schwendicke, Senbeta, Senthilkumaran, Sepanlou, Shackelford, Shadid, Shahabi, Shaheen, Shaikh, Shalash, Shams-Beyranvand, Shamsizadeh, Shannawaz, Sharafi, Sharara, Sheena, Sheikhtaheri, Shetty, Shibuya, Shiferaw, Shigematsu, Shin, Shiri, Shirkoohi, Shrime, Shuval, Siabani, Sigfusdottir, Sigurvinsdottir, Silva, Simpson, Singh, Singh, Skiadaresi, Skou, Skryabin, Sobngwi, Sokhan, Soltani, Sorensen, Soriano, Sorrie, Soyiri, Sreeramareddy, Stanaway, Stark, Ştefan, Stein, Steiner, Steiner, Stokes, Stovner, Stubbs, Sudaryanto, Sufiyan, Sulo, Sultan, Sykes, Sylte, Szócska, Tabarés-Seisdedos, Tabb, Tadakamadla, Taherkhani, Tajdini, Takahashi, Taveira, Teagle, Teame, Tehrani-Banihashemi, Teklehaimanot, Terrason, Tessema, Thankappan, Thomson, Tohidinik, Tonelli, Topor-Madry, Torre, Touvier, Tovani-Palone, Tran, Travillian, Troeger, Truelsen, Tsai, Tsatsakis, Tudor Car, Tyrovolas, Uddin, Ullah, Undurraga, Unnikrishnan, Vacante, Vakilian, Valdez, Varughese, Vasankari, Vasseghian, Venketasubramanian, Violante, Vlassov, Vollset, Vongpradith, Vukovic, Vukovic, Waheed, Walters, Wang, Wang, Wang, Ward, Watson, Wei, Weintraub, Weiss, Weiss, Westerman, Whisnant, Whiteford, Wiangkham, Wiens, Wijeratne, Wilner, Wilson, Wojtyniak, Wolfe, Wool, Wu, Wulf Hanson, Wunrow, Xu, Xu, Yadgir, Yahyazadeh Jabbari, Yamagishi, Yaminfirooz, Yano, Yaya, Yazdi-Feyzabadi, Yearwood, Yeheyis, Yeshitila, Yip, Yonemoto, Yoon, Yoosefi Lebni, Younis, Younker, Yousefi, Yousefifard, Yousefinezhadi, Yousuf, Yu, Yusefzadeh, Zahirian Moghadam, Zaki, Zaman, Zamani, Zamanian, Zandian, Zangeneh, Zastrozhin, Zewdie, Zhang, Zhang, Zhao, Zhao, Zheng, Zhou, Ziapour, Zimsen, Naghavi and Murray2020). The propensity to develop CVDs is fueled by chronically elevated blood pressure (BP) or hypertension (HTN). Among others, essential HTN is the most frequent type of HTN in adults (accounts for 95%). It is caused when there is sustained increase in the BP greater than 140/90 mmHg and when no etiology can be determined for the HTN (Gupta-Malhotra et al., Reference Gupta-Malhotra, Banker, Shete, Hashmi, Tyson, Barratt, Hecht, Milewicz and Boerwinkle2015). According to the World Health Organization, an estimated 1.28 billion adults of the age-group 30–79 years worldwide suffer from HTN (https://www.who.int/news-room/fact-sheets/detail/hypertension). Therefore, controlling the incidence of HTN is critical for improving the quality of life and prevention of premature death.
Research on HTN over the last few decades has established that the susceptibility to HTN is determined both by genetic and environmental factors. The estimated contribution of heritability of HTN is ~30%, while environmental factors contribute to ~70% (Biino et al., Reference Biino, Parati, Concas, Adamo, Angius, Vaccargiu and Pirastu2013). Despite the relatively lower contributions of genetics to HTN, there has been considerable focus on mining the genomic contributions to the genesis of HTN. There are two major factors propelling the momentum for understanding the genetics of HTN, (i) the desire to find novel druggable targets and (ii) advances in whole-genome sequencing, which alleviated the technical limitation of detecting human genetic variation on a large scale. Such efforts have thus far identified over 1,500 loci in human HTN (Evangelou et al., Reference Evangelou, Warren, Mosen-Ansorena, Mifsud, Pazoki, Gao, Ntritsos, Dimou, Cabrera, Karaman, Ng, Evangelou, Witkowska, Tzanis, Hellwege, Giri, Velez Edwards, Sun, Cho, Gaziano, Wilson, Tsao, Kovesdy, Esko, Mägi, Milani, Almgren, Boutin, Debette, Ding, Giulianini, Holliday, Jackson, Li-Gao, Lin, Luan, Mangino, Oldmeadow, Prins, Qian, Sargurupremraj, Shah, Surendran, Thériault, Verweij, Willems, Zhao, Amouyel, Connell, de Mutsert, Doney, Farrall, Menni, Morris, Noordam, Paré, Poulter, Shields, Stanton, Thom, Abecasis, Amin, Arking, Ayers, Barbieri, Batini, Bis, Blake, Bochud, Boehnke, Boerwinkle, Boomsma, Bottinger, Braund, Brumat, Campbell, Campbell, Chakravarti, Chambers, Chauhan, Ciullo, Cocca, Collins, Cordell, Davies, de Borst, de Geus, Deary, Deelen, del Greco, Demirkale, Dörr, Ehret, Elosua, Enroth, Erzurumluoglu, Ferreira, Frånberg, Franco, Gandin, Gasparini, Giedraitis, Gieger, Girotto, Goel, Gow, Gudnason, Guo, Gyllensten, Hamsten, Harris, Harris, Hartman, Havulinna, Hicks, Hofer, Hofman, Hottenga, Huffman, Hwang, Ingelsson, James, Jansen, Jarvelin, Joehanes, Johansson, Johnson, Joshi, Jousilahti, Jukema, Jula, Kähönen, Kathiresan, Keavney, Khaw, Knekt, Knight, Kolcic, Kooner, Koskinen, Kristiansson, Kutalik, Laan, Larson, Launer, Lehne, Lehtimäki, Liewald, Lin, Lind, Lindgren, Liu, Loos, Lopez, Lu, Lyytikäinen, Mahajan, Mamasoula, Marrugat, Marten, Milaneschi, Morgan, Morris, Morrison, Munson, Nalls, Nandakumar, Nelson, Niiranen, Nolte, Nutile, Oldehinkel, Oostra, O’Reilly, Org, Padmanabhan, Palmas, Palotie, Pattie, Penninx, Perola, Peters, Polasek, Pramstaller, Nguyen, Raitakari, Ren, Rettig, Rice, Ridker, Ried, Riese, Ripatti, Robino, Rose, Rotter, Rudan, Ruggiero, Saba, Sala, Salomaa, Samani, Sarin, Schmidt, Schmidt, Shrine, Siscovick, Smith, Snieder, Sõber, Sorice, Starr, Stott, Strachan, Strawbridge, Sundström, Swertz, Taylor, Teumer, Tobin, Tomaszewski, Toniolo, Traglia, Trompet, Tuomilehto, Tzourio, Uitterlinden, Vaez, van der Most, van Duijn, Vergnaud, Verwoert, Vitart, Völker, Vollenweider, Vuckovic, Watkins, Wild, Willemsen, Wilson, Wright, Yao, Zemunik, Zhang, Attia, Butterworth, Chasman, Conen, Cucca, Danesh, Hayward, Howson, Laakso, Lakatta, Langenberg, Melander, Mook-Kanamori, Palmer, Risch, Scott, Scott, Sever, Spector, van der Harst, Wareham, Zeggini, Levy, Munroe, Newton-Cheh, Brown, Metspalu, Hung, O’Donnell, Edwards, Psaty, Tzoulaki, Barnes, Wain, Elliott and Caulfield2018; Buniello et al., Reference Buniello, MacArthur, Cerezo, Harris, Hayhurst, Malangone, McMahon, Morales, Mountjoy, Sollis, Suveges, Vrousgou, Whetzel, Amode, Guillen, Riat, Trevanion, Hall, Junkins, Flicek, Burdett, Hindorff, Cunningham and Parkinson2019; Cabrera et al., Reference Cabrera, Ng, Nicholls, Gupta, Barnes, Munroe and Caulfield2019; Giri et al., Reference Giri, Hellwege, Keaton, Park, Qiu, Warren, Torstenson, Kovesdy, Sun, Wilson, Robinson-Cohen, Roumie, Chung, Birdwell, Damrauer, DuVall, Klarin, Cho, Wang, Evangelou, Cabrera, Wain, Shrestha, Mautz, Akwo, Sargurupremraj, Debette, Boehnke, Scott, Luan, Zhao, Willems, Thériault, Shah, Oldmeadow, Almgren, Li-Gao, Verweij, Boutin, Mangino, Ntalla, Feofanova, Surendran, Cook, Karthikeyan, Lahrouchi, Liu, Sepúlveda, Richardson, Kraja, Amouyel, Farrall, Poulter, Laakso, Zeggini, Sever, Scott, Langenberg, Wareham, Conen, Palmer, Attia, Chasman, Ridker, Melander, Mook-Kanamori, Harst, Cucca, Schlessinger, Hayward, Spector, Jarvelin, Hennig, Timpson, Wei, Smith, Xu, Matheny, Siew, Lindgren, Herzig, Dedoussis, Denny, Psaty, Howson, Munroe, Newton-Cheh, Caulfield, Elliott, Gaziano, Concato, Wilson, Tsao, Velez Edwards, Susztak, O’Donnell, Hung and Edwards2019). However, while they collectively define the genomic landscape for association with HTN in humans, individually, they are not druggable targets because each of these loci contribute very little to BP regulation.
In experimental studies using animal models, the genomic landscape for association with HTN was similar to that of humans. Animal model studies identified over 400 BP quantitative trait loci (https://rgd.mcw.edu/rgdweb/elasticResults.html?term=blood+pressure&chr=ALL&start=&stop=&species=Rat&category=QTL&objectSearch=true). Details on these investigations are documented in our previous review (Padmanabhan and Joe, Reference Padmanabhan and Joe2017) and updated in recent articles (Warren et al., Reference Warren, Evangelou, Cabrera, Gao, Ren, Mifsud, Ntalla, Surendran, Liu, Cook, Kraja, Drenos, Loh, Verweij, Marten, Karaman, Lepe, O’Reilly, Knight, Snieder, Kato, He, Tai, Said, Porteous, Alver, Poulter, Farrall, Gansevoort, Padmanabhan, Mägi, Stanton, Connell, Bakker, Metspalu, Shields, Thom, Brown, Sever, Esko, Hayward, van der Harst, Saleheen, Chowdhury, Chambers, Chasman, Chakravarti, Newton-Cheh, Lindgren, Levy, Kooner, Keavney, Tomaszewski, Samani, Howson, Tobin, Munroe, Ehret and Wain2017; Evangelou et al., Reference Evangelou, Warren, Mosen-Ansorena, Mifsud, Pazoki, Gao, Ntritsos, Dimou, Cabrera, Karaman, Ng, Evangelou, Witkowska, Tzanis, Hellwege, Giri, Velez Edwards, Sun, Cho, Gaziano, Wilson, Tsao, Kovesdy, Esko, Mägi, Milani, Almgren, Boutin, Debette, Ding, Giulianini, Holliday, Jackson, Li-Gao, Lin, Luan, Mangino, Oldmeadow, Prins, Qian, Sargurupremraj, Shah, Surendran, Thériault, Verweij, Willems, Zhao, Amouyel, Connell, de Mutsert, Doney, Farrall, Menni, Morris, Noordam, Paré, Poulter, Shields, Stanton, Thom, Abecasis, Amin, Arking, Ayers, Barbieri, Batini, Bis, Blake, Bochud, Boehnke, Boerwinkle, Boomsma, Bottinger, Braund, Brumat, Campbell, Campbell, Chakravarti, Chambers, Chauhan, Ciullo, Cocca, Collins, Cordell, Davies, de Borst, de Geus, Deary, Deelen, del Greco, Demirkale, Dörr, Ehret, Elosua, Enroth, Erzurumluoglu, Ferreira, Frånberg, Franco, Gandin, Gasparini, Giedraitis, Gieger, Girotto, Goel, Gow, Gudnason, Guo, Gyllensten, Hamsten, Harris, Harris, Hartman, Havulinna, Hicks, Hofer, Hofman, Hottenga, Huffman, Hwang, Ingelsson, James, Jansen, Jarvelin, Joehanes, Johansson, Johnson, Joshi, Jousilahti, Jukema, Jula, Kähönen, Kathiresan, Keavney, Khaw, Knekt, Knight, Kolcic, Kooner, Koskinen, Kristiansson, Kutalik, Laan, Larson, Launer, Lehne, Lehtimäki, Liewald, Lin, Lind, Lindgren, Liu, Loos, Lopez, Lu, Lyytikäinen, Mahajan, Mamasoula, Marrugat, Marten, Milaneschi, Morgan, Morris, Morrison, Munson, Nalls, Nandakumar, Nelson, Niiranen, Nolte, Nutile, Oldehinkel, Oostra, O’Reilly, Org, Padmanabhan, Palmas, Palotie, Pattie, Penninx, Perola, Peters, Polasek, Pramstaller, Nguyen, Raitakari, Ren, Rettig, Rice, Ridker, Ried, Riese, Ripatti, Robino, Rose, Rotter, Rudan, Ruggiero, Saba, Sala, Salomaa, Samani, Sarin, Schmidt, Schmidt, Shrine, Siscovick, Smith, Snieder, Sõber, Sorice, Starr, Stott, Strachan, Strawbridge, Sundström, Swertz, Taylor, Teumer, Tobin, Tomaszewski, Toniolo, Traglia, Trompet, Tuomilehto, Tzourio, Uitterlinden, Vaez, van der Most, van Duijn, Vergnaud, Verwoert, Vitart, Völker, Vollenweider, Vuckovic, Watkins, Wild, Willemsen, Wilson, Wright, Yao, Zemunik, Zhang, Attia, Butterworth, Chasman, Conen, Cucca, Danesh, Hayward, Howson, Laakso, Lakatta, Langenberg, Melander, Mook-Kanamori, Palmer, Risch, Scott, Scott, Sever, Spector, van der Harst, Wareham, Zeggini, Levy, Munroe, Newton-Cheh, Brown, Metspalu, Hung, O’Donnell, Edwards, Psaty, Tzoulaki, Barnes, Wain, Elliott and Caulfield2018; Giri et al., Reference Giri, Hellwege, Keaton, Park, Qiu, Warren, Torstenson, Kovesdy, Sun, Wilson, Robinson-Cohen, Roumie, Chung, Birdwell, Damrauer, DuVall, Klarin, Cho, Wang, Evangelou, Cabrera, Wain, Shrestha, Mautz, Akwo, Sargurupremraj, Debette, Boehnke, Scott, Luan, Zhao, Willems, Thériault, Shah, Oldmeadow, Almgren, Li-Gao, Verweij, Boutin, Mangino, Ntalla, Feofanova, Surendran, Cook, Karthikeyan, Lahrouchi, Liu, Sepúlveda, Richardson, Kraja, Amouyel, Farrall, Poulter, Laakso, Zeggini, Sever, Scott, Langenberg, Wareham, Conen, Palmer, Attia, Chasman, Ridker, Melander, Mook-Kanamori, Harst, Cucca, Schlessinger, Hayward, Spector, Jarvelin, Hennig, Timpson, Wei, Smith, Xu, Matheny, Siew, Lindgren, Herzig, Dedoussis, Denny, Psaty, Howson, Munroe, Newton-Cheh, Caulfield, Elliott, Gaziano, Concato, Wilson, Tsao, Velez Edwards, Susztak, O’Donnell, Hung and Edwards2019; Surendran et al., Reference Surendran, Feofanova, Lahrouchi, Ntalla, Karthikeyan, Cook, Chen, Mifsud, Yao, Kraja, Cartwright, Hellwege, Giri, Tragante, Thorleifsson, Liu, Prins, Stewart, Cabrera, Eales, Akbarov, Auer, Bielak, Bis, Braithwaite, Brody, Daw, Warren, Drenos, Nielsen, Faul, Fauman, Fava, Ferreira, Foley, Franceschini, Gao, Giannakopoulou, Giulianini, Gudbjartsson, Guo, Harris, Havulinna, Helgadottir, Huffman, Hwang, Kanoni, Kontto, Larson, Li-Gao, Lindström, Lotta, Lu, Luan, Mahajan, Malerba, Masca, Mei, Menni, Mook-Kanamori, Mosen-Ansorena, Müller-Nurasyid, Paré, Paul, Perola, Poveda, Rauramaa, Richard, Richardson, Sepúlveda, Sim, Smith, Smith, Staley, Stanáková, Sulem, Thériault, Thorsteinsdottir, Trompet, Varga, Velez Edwards, Veronesi, Weiss, Willems, Yao, Young, Yu, Zhang, Zhao, Zhao, Zhao, Evangelou, Aeschbacher, Asllanaj, Blankenberg, Bonnycastle, Bork-Jensen, Brandslund, Braund, Burgess, Cho, Christensen, Connell, Mutsert, Dominiczak, Dörr, Eiriksdottir, Farmaki, Gaziano, Grarup, Grove, Hallmans, Hansen, Have, Heiss, Jørgensen, Jousilahti, Kajantie, Kamat, Käräjämäki, Karpe, Koistinen, Kovesdy, Kuulasmaa, Laatikainen, Lannfelt, Lee, Lee, de Boer, van der Harst, van der Meer, Verweij, Linneberg, Martin, Moitry, Nadkarni, Neville, Palmer, Papanicolaou, Pedersen, Peters, Poulter, Rasheed, Rasmussen, Rayner, Mägi, Renström, Rettig, Rossouw, Schreiner, Sever, Sigurdsson, Skaaby, Sun, Sundstrom, Thorgeirsson, Esko, Trabetti, Tsao, Tuomi, Turner, Tzoulaki, Vaartjes, Vergnaud, Willer, Wilson, Witte, Yonova-Doing, Zhang, Aliya, Almgren, Amouyel, Asselbergs, Barnes, Blakemore, Boehnke, Bots, Bottinger, Buring, Chambers, Chen, Chowdhury, Conen, Correa, Davey Smith, Boer, Deary, Dedoussis, Deloukas, di Angelantonio, Elliott, Butterworth, Danesh, Langenberg, Deloukas, McCarthy, Franks, Rolandsson, Wareham, Felix, Ferrières, Ford, Fornage, Franks, Franks, Frossard, Gambaro, Gaunt, Groop, Gudnason, Harris, Hayward, Hennig, Herzig, Ingelsson, Tuomilehto, Järvelin, Jukema, Kardia, Kee, Kooner, Kooperberg, Launer, Lind, Loos, Majumder, Laakso, McCarthy, Melander, Mohlke, Murray, Nordestgaard, Orho-Melander, Packard, Padmanabhan, Palmas, Polasek, Porteous, Prentice, Province, Relton, Rice, Ridker, Rolandsson, Rosendaal, Rotter, Rudan, Salomaa, Samani, Sattar, Sheu, Smith, Soranzo, Spector, Starr, Sebert, Taylor, Lakka, Timpson, Tobin, Prins, Zeggini, van der Harst, van der Meer, Ramachandran, Verweij, Virtamo, Völker, Weir, Zeggini, Charchar, Hellwege, Giri, Edwards, Cho, Gaziano, Kovesdy, Sun, Tsao, Wilson, Edwards, Hung, O’Donnell, Wareham, Langenberg, Tomaszewski, Butterworth, Caulfield, Danesh, Edwards, Holm, Hung, Lindgren, Liu, Manning, Morris, Morrison, O’Donnell, Psaty, Saleheen, Stefansson, Boerwinkle, Chasman, Levy, Newton-Cheh, Munroe and Howson2020; Olczak et al., Reference Olczak, Taylor‐Bateman, Nicholls, Traylor, Cabrera and Munroe2021; Padmanabhan and Dominiczak, Reference Padmanabhan and Dominiczak2021). Meanwhile, research beyond genomic analyses has led to the profound realization that gut microbiota is an important nongenomic factor which was not previously accounted for in the etiology of HTN. Specifically, our group was the first to report the evidence of gut microbiota dysbiosis in both hypertensive animal models and patients (Mell et al., Reference Mell, Jala, Mathew, Byun, Waghulde, Zhang, Haribabu, Vijay-Kumar, Pennathur and Joe2015; Yang et al., Reference Yang, Santisteban, Rodriguez, Li, Ahmari, Carvajal, Zadeh, Gong, Qi, Zubcevic, Sahay, Pepine, Raizada and Mohamadzadeh2015). Following this pioneering discovery, associations between gut microbiota are reported between hypertensive and normotensive animal models and humans (Tables 1 and 2). In this article, we review the literature on gut microbiota and HTN and propose developing a gut metagenomic risk score (MRS) for HTN. Further, we discuss the value of combining MRS with polygenic risk score (PRS), CRS and artificial intelligence (AI) for clinical management of HTN (Graphical Abstract).
Table 1. The association observed between animal hypertension, gut microbiota and various interventions

Table 2. The association observed between human hypertension, gut microbiota and various interventions

From GWAS to PRS for HTN
Genome-wide association studies (GWAS) aim to analyze genetic variants across genomes to detect associations with complex traits (Dehghan, Reference Dehghan2018). GWAS for HTN began in 2007 with the first report of associations in the Wellcome Trust Case Control Consortium (Burton et al., 2007). GWAS for HTN soon outpaced all linkage analyses in humans (Figure 1b). Even so, the collective effect of all BP loci identified through GWAS accounts for ~3.5% of BP variance (Manolio et al., Reference Manolio, Collins, Cox, Goldstein, Hindorff, Hunter, McCarthy, Ramos, Cardon, Chakravarti, Cho, Guttmacher, Kong, Kruglyak, Mardis, Rotimi, Slatkin, Valle, Whittemore, Boehnke, Clark, Eichler, Gibson, Haines, Mackay, McCarroll and Visscher2009; Sung et al., Reference Sung, Winkler, de Las Fuentes, Bentley, Brown, Kraja, Schwander, Ntalla, Guo, Franceschini, Lu, Cheng, Sim, Vojinovic, Marten, Musani, Li, Feitosa, Kilpeläinen, Richard, Noordam, Aslibekyan, Aschard, Bartz, Dorajoo, Liu, Manning, Rankinen, Smith, Tajuddin, Tayo, Warren, Zhao, Zhou, Matoba, Sofer, Alver, Amini, Boissel, Chai, Chen, Divers, Gandin, Gao, Giulianini, Goel, Harris, Hartwig, Horimoto, Hsu, Jackson, Kähönen, Kasturiratne, Kühnel, Leander, Lee, Lin, ’an Luan, McKenzie, Meian, Nelson, Rauramaa, Schupf, Scott, Sheu, Stančáková, Takeuchi, van der Most, Varga, Wang, Wang, Ware, Weiss, Wen, Yanek, Zhang, Zhao, Afaq, Alfred, Amin, Arking, Aung, Barr, Bielak, Boerwinkle, Bottinger, Braund, Brody, Broeckel, Cabrera, Cade, Caizheng, Campbell, Canouil, Chakravarti, Chauhan, Christensen, Cocca, Collins, Connell, de Mutsert, de Silva, Debette, Dörr, Duan, Eaton, Ehret, Evangelou, Faul, Fisher, Forouhi, Franco, Friedlander, Gao, Gigante, Graff, Gu, Gu, Gupta, Hagenaars, Harris, He, Heikkinen, Heng, Hirata, Hofman, Howard, Hunt, Irvin, Jia, Joehanes, Justice, Katsuya, Kaufman, Kerrison, Khor, Koh, Koistinen, Komulainen, Kooperberg, Krieger, Kubo, Kuusisto, Langefeld, Langenberg, Launer, Lehne, Lewis, Li, Lim, Lin, Liu, Liu, Liu, Liu, Liu, Loh, Lohman, Long, Louie, Mägi, Mahajan, Meitinger, Metspalu, Milani, Momozawa, Morris, Mosley, Munson, Murray, Nalls, Nasri, Norris, North, Ogunniyi, Padmanabhan, Palmas, Palmer, Pankow, Pedersen, Peters, Peyser, Polasek, Raitakari, Renström, Rice, Ridker, Robino, Robinson, Rose, Rudan, Sabanayagam, Salako, Sandow, Schmidt, Schreiner, Scott, Seshadri, Sever, Sitlani, Smith, Snieder, Starr, Strauch, Tang, Taylor, Teo, Tham, Uitterlinden, Waldenberger, Wang, Wang, Wei, Williams, Wilson, Wojczynski, Yao, Yuan, Zonderman, Becker, Boehnke, Bowden, Chambers, Chen, de Faire, Deary, Esko, Farrall, Forrester, Franks, Freedman, Froguel, Gasparini, Gieger, Horta, Hung, Jonas, Kato, Kooner, Laakso, Lehtimäki, Liang, Magnusson, Newman, Oldehinkel, Pereira, Redline, Rettig, Samani, Scott, Shu, van der Harst, Wagenknecht, Wareham, Watkins, Weir, Wickremasinghe, Wu, Zheng, Kamatani, Laurie, Bouchard, Cooper, Evans, Gudnason, Kardia, Kritchevsky, Levy, O’Connell, Psaty, van Dam, Sims, Arnett, Mook-Kanamori, Kelly, Fox, Hayward, Fornage, Rotimi, Province, van Duijn, Tai, Wong, Loos, Reiner, Rotter, Zhu, Bierut, Gauderman, Caulfield, Elliott, Rice, Munroe, Morrison, Cupples, Rao and Chasman2018). This begs the question: ‘What is the expectation from continued investments in GWAS for clinical management of HTN?’ From the perspective of disease risk prediction, continued research in GWAS for HTN is essential for developing, defining and refining the predictive power for HTN using a genomic index, which is known as PRS (Choi et al., Reference Choi, Mak and O’Reilly2020; Lewis and Vassos, Reference Lewis and Vassos2020; Padmanabhan and Dominiczak, Reference Padmanabhan and Dominiczak2021). It is computed as the sum of an individual’s genome-wide genotype that is weighted by corresponding genotype effect size estimates (or Z scores) generated from a relevant GWAS data (Lewis and Vassos, Reference Lewis and Vassos2020). Although PRSs often explain only a small portion of trait variance, their link with genetic liability, the single biggest source of phenotypic variation, has rendered PRS as an attractive prediction tool in biomedical research (Choi et al., Reference Choi, Mak and O’Reilly2020). PRS is used to assess shared etiologies between phenotypes and to investigate the clinical applicability of genetic information for complex diseases (Choi et al., Reference Choi, Mak and O’Reilly2020). Previously, a PRS constructed utilizing genome-wide important single nucleotide polymorphisms from GWAS for BP showed a significant relationship with heart failure, left ventricular mass, coronary artery disease and stroke (Studies, 2011; Ference et al., Reference Ference, Julius, Mahajan, Levy, Williams and Flack2014). Currently, there is considerable excitement in the field for developing reliable PRS for HTN as evident from multiple reports of PRS indices from different cohorts (Steinthorsdottir et al., Reference Steinthorsdottir, McGinnis, Williams, Stefansdottir, Thorleifsson, Shooter, Fadista, Sigurdsson, Auro, Berezina, Borges, Bumpstead, Bybjerg-Grauholm, Colgiu, Dolby, Dudbridge, Engel, Franklin, Frigge, Frisbaek, Geirsson, Geller, Gretarsdottir, Gudbjartsson, Harmon, Hougaard, Hegay, Helgadottir, Hjartardottir, Jääskeläinen, Johannsdottir, Jonsdottir, Juliusdottir, Kalsheker, Kasimov, Kemp, Kivinen, Klungsøyr, Lee, Melbye, Miedzybrodska, Moffett, Najmutdinova, Nishanova, Olafsdottir, Perola, Pipkin, Poston, Prescott, Saevarsdottir, Salimbayeva, Scaife, Skotte, Staines-Urias, Stefansson, Sørensen, Thomsen, Tragante, Trogstad, Simpson, Aripova, Casas, Dominiczak, Walker, Thorsteinsdottir, Iversen, Feenstra, Lawlor, Boyd, Magnus, Laivuori, Zakhidova, Svyatova, Stefansson and Morgan2020; Sapkota et al., Reference Sapkota, Li, Pierzynski, Mulrooney, Ness, Morton, Michael, Zhang, Bhatia, Armstrong, Hudson, Robison and Yasui2021; Sato et al., Reference Sato, Fudono, Imai, Takimoto, Tarui, Aoyama, Yago, Okamitsu, Mizutani and Miyasaka2021; Fujii et al., Reference Fujii, Hishida, Nakatochi, Tsuboi, Suzuki, Kondo, Ikezaki, Hara, Okada, Tamura, Shimoshikiryo, Suzuki, Koyama, Kuriki, Takashima, Arisawa, Momozawa, Kubo, Takeuchi and Wakai2022; Parcha et al., Reference Parcha, Pampana, Bress, Irvin, Arora and Arora2022; Quintanilha et al., Reference Quintanilha, Etheridge, Graynor, Larson, Crona, Mitchell and Innocenti2022). Recently, Weng et al. (Reference Weng, Liu, Yan, Liang, Zhang, Xu, Li, Xu and Gu2022) included 391,366 participants from the UK Biobank database and established a PRS for HTN assessing the combined effect of genetic susceptibility and air pollution on incident of HTN. They demonstrated that long-term exposure to air pollution is associated with increased risk of HTN particularly in individuals with high genetic risk (Weng et al., Reference Weng, Liu, Yan, Liang, Zhang, Xu, Li, Xu and Gu2022). Another study from Finland revealed that a BP (systolic and diastolic) PRS could predict HTN in the FINRISK cohort, a Finnish population survey on risk factors on chronic, noncommunicable diseases (Vaura et al., Reference Vaura, Kauko, Suvila, Havulinna, Mars, Salomaa, Cheng and Niiranen2021, https://thl.fi/en/web/thl-biobank/). This study highlights the potential of PRS as a predictive tool that may be better than the established clinical risk factors for the prediction of HTN (Vaura et al., Reference Vaura, Kauko, Suvila, Havulinna, Mars, Salomaa, Cheng and Niiranen2021). But both studies are limited by their reliance on genetic data from European ancestries, which could limit the predictive power of a PRS in other populations. With the availability of recent, large multi-ethnic and non-European GWAS of BP phenotypes, such as those from the Million Veteran Program, the UK Biobank and Biobank Japan (Kanai et al., Reference Kanai, Akiyama, Takahashi, Matoba, Momozawa, Ikeda, Iwata, Ikegawa, Hirata, Matsuda, Kubo, Okada and Kamatani2018; Giri et al., Reference Giri, Hellwege, Keaton, Park, Qiu, Warren, Torstenson, Kovesdy, Sun, Wilson, Robinson-Cohen, Roumie, Chung, Birdwell, Damrauer, DuVall, Klarin, Cho, Wang, Evangelou, Cabrera, Wain, Shrestha, Mautz, Akwo, Sargurupremraj, Debette, Boehnke, Scott, Luan, Zhao, Willems, Thériault, Shah, Oldmeadow, Almgren, Li-Gao, Verweij, Boutin, Mangino, Ntalla, Feofanova, Surendran, Cook, Karthikeyan, Lahrouchi, Liu, Sepúlveda, Richardson, Kraja, Amouyel, Farrall, Poulter, Laakso, Zeggini, Sever, Scott, Langenberg, Wareham, Conen, Palmer, Attia, Chasman, Ridker, Melander, Mook-Kanamori, Harst, Cucca, Schlessinger, Hayward, Spector, Jarvelin, Hennig, Timpson, Wei, Smith, Xu, Matheny, Siew, Lindgren, Herzig, Dedoussis, Denny, Psaty, Howson, Munroe, Newton-Cheh, Caulfield, Elliott, Gaziano, Concato, Wilson, Tsao, Velez Edwards, Susztak, O’Donnell, Hung and Edwards2019), PRS predictions are now expanded to other demographics, which is a promising outlook for the construction of multi-ethnic PRS for HTN risk prediction (Cavazos and Witte, Reference Cavazos and Witte2021). More recently, the Trans-Omics in Precision Medicine Initiative program (Stilp et al., Reference Stilp, Emery, Broome, Buth, Khan, Laurie, Wang, Wong, Chen, D’Augustine, Heard-Costa, Hohensee, Johnson, Juarez, Liu, Mutalik, Raffield, Wiggins, de Vries, Kelly, Kooperberg, Natarajan, Peloso, Peyser, Reiner, Arnett, Aslibekyan, Barnes, Bielak, Bis, Cade, Chen, Correa, Cupples, de Andrade, Ellinor, Fornage, Franceschini, Gan, Ganesh, Graffelman, Grove, Guo, Hawley, Hsu, Jackson, Jaquish, Johnson, Kardia, Kelly, Lee, Mathias, McGarvey, Mitchell, Montasser, Morrison, North, Nouraie, Oelsner, Pankratz, Rich, Rotter, Smith, Taylor, Vasan, Weeks, Weiss, Wilson, Yanek, Psaty, Heckbert and Laurie2021; Taliun et al., Reference Taliun, Harris, Kessler, Carlson, Szpiech, Torres, Taliun, Corvelo, Gogarten, Kang, Pitsillides, LeFaive, Lee, Tian, Browning, das, Emde, Clarke, Loesch, Shetty, Blackwell, Smith, Wong, Liu, Conomos, Bobo, Aguet, Albert, Alonso, Ardlie, Arking, Aslibekyan, Auer, Barnard, Barr, Barwick, Becker, Beer, Benjamin, Bielak, Blangero, Boehnke, Bowden, Brody, Burchard, Cade, Casella, Chalazan, Chasman, Chen, Cho, Choi, Chung, Clish, Correa, Curran, Custer, Darbar, Daya, de Andrade, DeMeo, Dutcher, Ellinor, Emery, Eng, Fatkin, Fingerlin, Forer, Fornage, Franceschini, Fuchsberger, Fullerton, Germer, Gladwin, Gottlieb, Guo, Hall, He, Heard-Costa, Heckbert, Irvin, Johnsen, Johnson, Kaplan, Kardia, Kelly, Kelly, Kenny, Kiel, Klemmer, Konkle, Kooperberg, Köttgen, Lange, Lasky-Su, Levy, Lin, Lin, Liu, Loos, Garman, Gerszten, Lubitz, Lunetta, Mak, Manichaikul, Manning, Mathias, McManus, McGarvey, Meigs, Meyers, Mikulla, Minear, Mitchell, Mohanty, Montasser, Montgomery, Morrison, Murabito, Natale, Natarajan, Nelson, North, O’Connell, Palmer, Pankratz, Peloso, Peyser, Pleiness, Post, Psaty, Rao, Redline, Reiner, Roden, Rotter, Ruczinski, Sarnowski, Schoenherr, Schwartz, Seo, Seshadri, Sheehan, Sheu, Shoemaker, Smith, Smith, Sotoodehnia, Stilp, Tang, Taylor, Telen, Thornton, Tracy, van den Berg, Vasan, Viaud-Martinez, Vrieze, Weeks, Weir, Weiss, Weng, Willer, Zhang, Zhao, Arnett, Ashley-Koch, Barnes, Boerwinkle, Gabriel, Gibbs, Rice, Rich, Silverman, Qasba, Gan, Abe, Almasy, Ament, Anderson, Anugu, Applebaum-Bowden, Assimes, Avramopoulos, Barron-Casella, Beaty, Beck, Becker, Beitelshees, Benos, Bezerra, Bis, Bowler, Broeckel, Broome, Bunting, Bustamante, Buth, Cardwell, Carey, Carty, Casaburi, Castaldi, Chaffin, Chang, Chang, Chavan, Chen, Chen, Chuang, Chung, Comhair, Cornell, Crandall, Crapo, Curtis, Damcott, David, Davis, Fuentes, DeBaun, Deka, Devine, Duan, Duggirala, Durda, Eaton, Ekunwe, el Boueiz, Erzurum, Farber, Flickinger, Fornage, Frazar, Fu, Fulton, Gao, Gao, Gass, Gelb, Geng, Geraci, Ghosh, Gignoux, Glahn, Gong, Goring, Graw, Grine, Gu, Guan, Gupta, Haessler, Hawley, Heavner, Herrington, Hersh, Hidalgo, Hixson, Hobbs, Hokanson, Hong, Hoth, Hsiung, Hung, Huston, Hwu, Jackson, Jain, Jhun, Johnson, Johnston, Jones, Kathiresan, Khan, Kim, Kinney, Kramer, Lange, Lange, Lange, Laurie, LeBoff, Lee, Lee, Lee, Levine, Lewis, Li, Li, Lin, Lin, Lin, Liu, Liu, Liu, Luo, Mahaney, Make, Manson, Margolin, Martin, Mathai, May, McArdle, McDonald, McFarland, McGoldrick, McHugh, Mei, Mestroni, Min, Minster, Moll, Moscati, Musani, Mwasongwe, Mychaleckyj, Nadkarni, Naik, Naseri, Nekhai, Neltner, Ochs-Balcom, Paik, Pankow, Parsa, Peralta, Perez, Perry, Peters, Phillips, Pollin, Becker, Boorgula, Preuss, Qiao, Qin, Rafaels, Raffield, Rasmussen-Torvik, Ratan, Reed, Regan, Reupena, Roselli, Russell, Ruuska, Ryan, Sabino, Saleheen, Salimi, Salzberg, Sandow, Sankaran, Scheller, Schmidt, Schwander, Sciurba, Seidman, Seidman, Sherman, Shetty, Sheu, Silver, Smith, Smith, Smoller, Snively, Snyder, Sofer, Storm, Streeten, Sung, Sylvia, Szpiro, Sztalryd, Tang, Taub, Taylor, Taylor, Threlkeld, Tinker, Tirschwell, Tishkoff, Tiwari, Tong, Tsai, Vaidya, VandeHaar, Walker, Wallace, Walts, Wang, Wang, Watson, Wessel, Williams, Williams, Wilson, Wu, Xu, Yanek, Yang, Yang, Zaghloul, Zekavat, Zhao, Zhao, Zhi, Zhou, Zhu, Papanicolaou, Nickerson, Browning, Zody, Zöllner, Wilson, Cupples, Laurie, Jaquish, Hernandez, O’Connor and Abecasis2021) reported the assessment of PRS for HTN across major U.S. demographic segments. This included African Americans, Hispanic/Latino Americans, Asian Americans and European Americans in the assessment of PRS associations with HTN across the lifespan. The final HTN-PRS was compared with incident outcomes in the Mass General Brigham Biobank as well as with Multi-ethnic Independent Biobank that included 40,201 subjects, leading to associations that supported the links between PRS and HTN. The resulting PRS was also predictive of an elevated risk of type 2 diabetes, chronic renal disease, coronary artery disease and ischemic stroke (Kurniansyah et al., Reference Kurniansyah, Goodman, Kelly, Elfassy, Wiggins, Bis, Guo, Palmas, Taylor, Lin, Haessler, Gao, Shimbo, Smith, Yu, Feofanova, Smit, Wang, Hwang, Liu, Wassertheil-Smoller, Manson, Lloyd-Jones, Rich, Loos, Redline, Correa, Kooperberg, Fornage, Kaplan, Psaty, Rotter, Arnett, Morrison, Franceschini, Levy, Bis, Guo, Taylor, Lin, Haessler, Gao, Smith, Liu, Wassertheil-Smoller, Manson, Rich, Redline, Correa, Kooperberg, Fornage, Kaplan, Psaty, Rotter, Arnett, Franceschini, Levy, Sofer and Sofer2022). Based on these results, Kurniansyah et al. (Reference Kurniansyah, Goodman, Kelly, Elfassy, Wiggins, Bis, Guo, Palmas, Taylor, Lin, Haessler, Gao, Shimbo, Smith, Yu, Feofanova, Smit, Wang, Hwang, Liu, Wassertheil-Smoller, Manson, Lloyd-Jones, Rich, Loos, Redline, Correa, Kooperberg, Fornage, Kaplan, Psaty, Rotter, Arnett, Morrison, Franceschini, Levy, Bis, Guo, Taylor, Lin, Haessler, Gao, Smith, Liu, Wassertheil-Smoller, Manson, Rich, Redline, Correa, Kooperberg, Fornage, Kaplan, Psaty, Rotter, Arnett, Franceschini, Levy, Sofer and Sofer2022) proposed a new approach for tuning parameters for PRS construction including optimization of the coefficient of variation of the effect size estimates and combining PRS based on GWAS of multiple BP phenotypes into a single PRS. Collectively, the next phase of GWAS in HTN should focus on prediction rather than treatment, the implementation of which will depend on the accuracy for applicability of a PRS for HTN in a global setting. To this end, the ‘All of Us’ research program in the United States is enrolling a million individuals from diverse populations for building a repository that includes genomic data, along with variables such as lifestyle, socioeconomic factors, environment and biological factors (All of Us Research Program Investigators, 2019). The United States is a melting pot of diverse populations from around the world. It is therefore particularly interesting to explore this database for further enhancing the power of PRS for HTN.
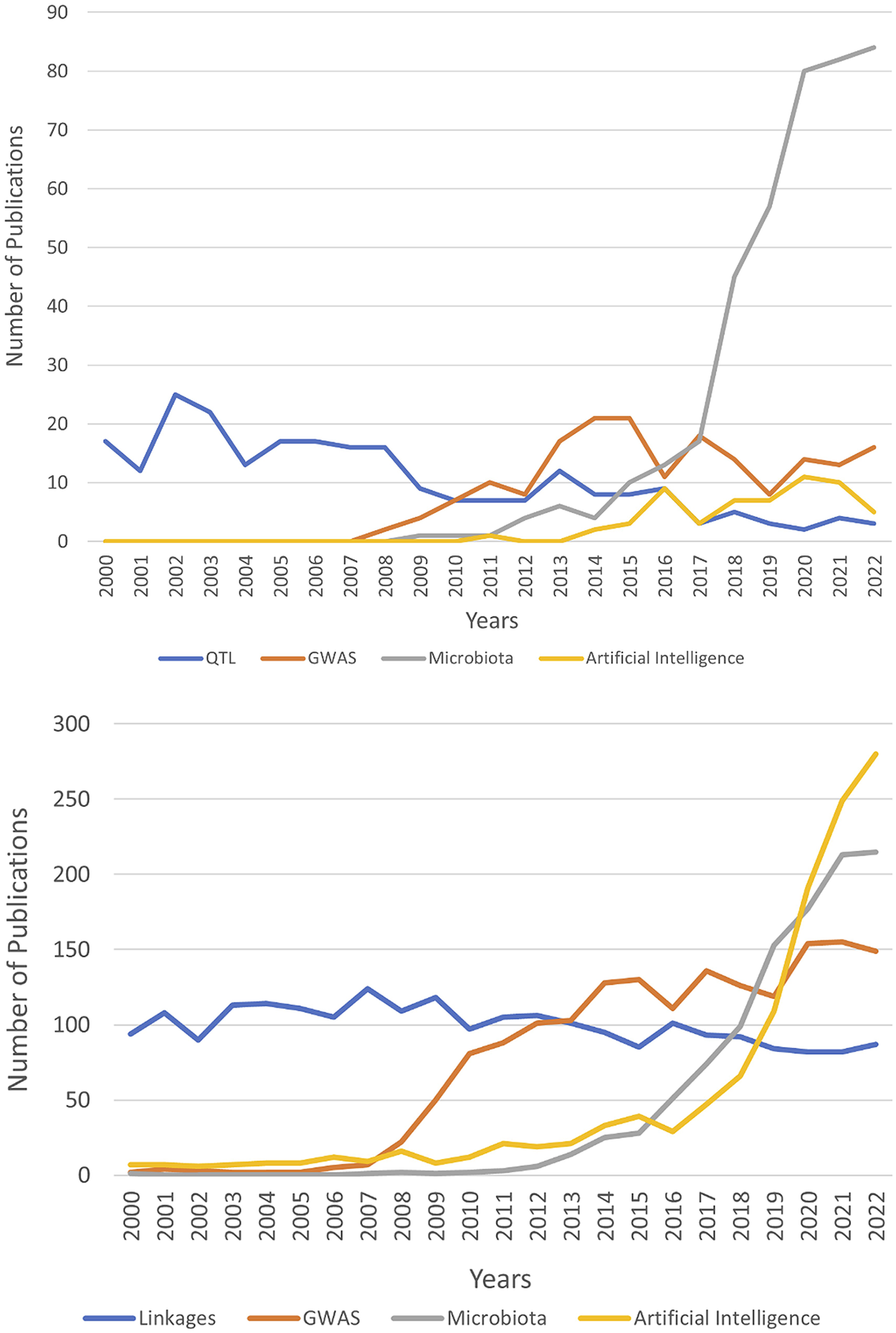
Figure 1. (a) The numbers of PubMed publications (2000–2022) related to quantitative trait locus (QTL), genome-wide association studies (GWAS), microbiota, artificial intelligence in rats and mice hypertension. The search keywords were QTL, hypertension, rats, mice, GWAS, microbiota and artificial intelligence. (b) The numbers of PubMed publications (2000–2022) related to linkage, genome-wide association studies (GWAS), microbiota and artificial intelligence in human hypertension. The search keywords were linkage, hypertension, humans, GWAS, microbiota and artificial intelligence.
Limitations for PRS-based predictions for HTN
Despite the promise and potential of PRS for HTN, there are clear barriers for its application in a clinical setting. One of the main concerns is the environmental component, which has larger effects than the genetic component on BP and may skew the prediction scores. Additionally, PRS analyses are not well-standardized and may lead to faulty interpretations (Choi et al., Reference Choi, Mak and O’Reilly2020). Thus, the focus must move from association with case–control status to individualized PRS for enhancing disease prediction (Lewis and Vassos, Reference Lewis and Vassos2020). Additionally, absolute risks for the disease should be converted from relative risks that compare people across the PRS continuum with a control group (Torkamani et al., Reference Torkamani, Wineinger and Topol2018; Sugrue and Desikan, Reference Sugrue and Desikan2019). When using PRS for HTN prediction, management and treatment, it is also required to rigorously differentiate between essential HTN and secondary HTN. Finally, as is the case with all diseases, there are ethical concerns regarding the application of PRS for HTN, which may escalate health inequities (Minari et al., Reference Minari, Brothers and Morrison2018; Martin et al., Reference Martin, Kanai, Kamatani, Okada, Neale and Daly2019; Vaura et al., Reference Vaura, Kauko, Suvila, Havulinna, Mars, Salomaa, Cheng and Niiranen2021).
Progress beyond GWAS: What are we missing?
As mentioned above, the premise of using PRS alone for HTN lacks power because of the environmental factors contributing to its etiology. In this context, it is important to note that a prominent, previously unknown, and relatively recent factor identified as contributing to BP regulation is the composition of gut microbiota. As shown in Figure 1a,b, the numbers of studies on microbiota and HTN is sharply rising in both animal models and humans. Interestingly, the sheer numbers of such studies currently surpasses that of GWAS studies, indicating its importance. In the following sections, we review these studies and propose that the inclusion of microbiota signatures and their functional readouts along with the genetic makeup of the host may enhance the power of PRS for HTN.
Gut microbiota and HTN
A large body of evidence has emerged in the last decade supporting the role of the microbiota in BP regulation. Our group has been at the forefront of this research. In 2010, it was shown that knockout of toll-like receptor 5 (Tlr5) in mice resulted in elevated BP (Vijay-Kumar et al., Reference Vijay-Kumar, Aitken, Carvalho, Cullender, Mwangi, Srinivasan, Sitaraman, Knight, Ley and Gewirtz2010). Tlr5 is a receptor for the bacterial protein flagellin, suggesting a link between gut microbiota and HTN. However, the major focus of this report was on metabolic syndrome, of which BP is a hallmark. The first evidence for a direct link between gut microbiota and BP regulation in a genetic model of HTN was reported in 2015 in Dahl salt-sensitive (DSS) rat (Mell et al., Reference Mell, Jala, Mathew, Byun, Waghulde, Zhang, Haribabu, Vijay-Kumar, Pennathur and Joe2015). Shortly thereafter, an association between gut dysbiosis and HTN in spontaneously hypertensive rats (SHR), angiotensin II-induced hypertensive rats, sleep apnea-induced hypertensive rats (Lloyd et al., Reference Lloyd, Durgan, Martini and Bryan2015) and hypertensive humans were reported (Yang et al., Reference Yang, Santisteban, Rodriguez, Li, Ahmari, Carvajal, Zadeh, Gong, Qi, Zubcevic, Sahay, Pepine, Raizada and Mohamadzadeh2015). Since these initial groundbreaking reports, multiple publications have demonstrated associations of gut microbiota with BP regulation in animal models and humans (Tables 1 and 2).
One question of assessing microbiota composition in rats is whether they are translationally relevant for humans. Human gut microbiota composition is more similar to rats than to mice (Flemer et al., Reference Flemer, Gaci, Borrel, Sanderson, Chaudhary, Tottey, O’Toole and Brugère2017), although this question is evolving with continued development of sequencing methods. Here, we access the commonalities in taxonomic rearrangements occurring during HTN in rats and humans. One of the themes emanating from BP association studies using rat as model organism is the application of the Firmicutes/Bacteroidetes (F/B) ratio in assessment of gut dysbiosis in HTN. Increased F/B ratio is regarded as a marker of gut dysbiosis and is consistently reported both in genetic and induced hypertensive rat models including the SHR (Yang et al., Reference Yang, Santisteban, Rodriguez, Li, Ahmari, Carvajal, Zadeh, Gong, Qi, Zubcevic, Sahay, Pepine, Raizada and Mohamadzadeh2015; Hsu et al., Reference Hsu, Hou, Chang-Chien, Lin and Tain2020; Li et al., Reference Li, Yang, Richards, Pepine and Raizada2020), DSS rats (Mell et al., Reference Mell, Jala, Mathew, Byun, Waghulde, Zhang, Haribabu, Vijay-Kumar, Pennathur and Joe2015; Waghulde et al., Reference Waghulde, Cheng, Galla, Mell, Cai, Pruett-Miller, Vazquez, Patterson, Vijay Kumar and Joe2018) high-fat diet fed rats (Hsu et al., Reference Hsu, Hou, Lee, Chan and Tain2019), NG-nitro-l-arginine methyl ester (l-NAME) treated rats (Robles-Vera et al., Reference Robles-Vera, Toral, de la Visitación, Sánchez, Romero, Olivares, Jiménez and Duarte2018) and angiotensin II induced HTN rats (Yang et al., Reference Yang, Santisteban, Rodriguez, Li, Ahmari, Carvajal, Zadeh, Gong, Qi, Zubcevic, Sahay, Pepine, Raizada and Mohamadzadeh2015). In further support, normalizing the F/B ratio by administration of the anti-inflammatory antibiotic, minocycline, alleviated angiotensin II-induced HTN (Yang et al., Reference Yang, Santisteban, Rodriguez, Li, Ahmari, Carvajal, Zadeh, Gong, Qi, Zubcevic, Sahay, Pepine, Raizada and Mohamadzadeh2015). This direct relationship between F/B ratio and BP has also been documented in various mouse models (Marques et al., Reference Marques, Nelson, Chu, Horlock, Fiedler, Ziemann, Tan, Kuruppu, Rajapakse, el-Osta, Mackay and Kaye2017; Toral et al., Reference Toral, Romero, Rodríguez-Nogales, Jiménez, Robles-Vera, Algieri, Chueca-Porcuna, Sánchez, de la Visitación, Olivares, García, Pérez-Vizcaíno, Gálvez and Duarte2018). Similarly, human studies (Mushtaq et al., Reference Mushtaq, Hussain, Zhang, Yuan, Li, Ullah, Wang and Xu2019; Silveira-Nunes et al., Reference Silveira-Nunes, Durso, Cunha, Maioli, Vieira, Speziali, Corrêa-Oliveira, Martins-Filho, Teixeira-Carvalho, Franceschi, Rampelli, Turroni, Brigidi and Faria2020; Joishy et al., Reference Joishy, Jha, Oudah, das, Adak, Deb and Khan2022) also support a direct relationship between F/B ratio and BP. In contrast, cold-induced HTN (Wang et al., Reference Wang, Liu, Lei, Xue, Li, Tian, Zhang and Luo2022) is one of the rare contexts wherein F/B ratio was not altered significantly. Nevertheless, more robust markers of gut dysbiosis should be developed to posit strong correlation between decreased microbial diversity and HTN.
Beyond the F/B ratio, remodeling of the overall composition of gut microbiota has been documented in the context of HTN. For example, enrichment in gut bacterial lactate producers such as Streptococcus and Turicibacter (Yang et al., Reference Yang, Santisteban, Rodriguez, Li, Ahmari, Carvajal, Zadeh, Gong, Qi, Zubcevic, Sahay, Pepine, Raizada and Mohamadzadeh2015; Toral et al., Reference Toral, Robles-Vera, de la Visitación, Romero, Sánchez, Gómez-Guzmán, Rodriguez-Nogales, Yang, Jiménez, Algieri, Gálvez, Raizada and Duarte2019b; Robles-Vera et al., Reference Robles-Vera, Toral, Visitación, Sánchez, Gómez-Guzmán, Muñoz, Algieri, Vezza, Jiménez, Gálvez, Romero, Redondo and Duarte2020b) and depletion of butyrate producers such as Coprococcus, and Pseudobutyrivibrio (Yang et al., Reference Yang, Santisteban, Rodriguez, Li, Ahmari, Carvajal, Zadeh, Gong, Qi, Zubcevic, Sahay, Pepine, Raizada and Mohamadzadeh2015; Durgan et al., Reference Durgan, Ganesh, Cope, Ajami, Phillips, Petrosino, Hollister and Bryan2016) are reported in hypertensive rodents. Importantly, Streptococcus and Coprococcus are also taxa similarly associated with human HTN (Yan et al., Reference Yan, Gu, Li, Yang, Jia, Chen, Han, Huang, Zhao, Li, Fang, Zhou, Guan, Ding, Wang, Khan, Xin, Li and Ma2017; de la Cuesta-Zuluaga et al., Reference de la Cuesta-Zuluaga, Mueller, Álvarez-Quintero, Velásquez-Mejía, Sierra, Corrales-Agudelo, Carmona, Abad and Escobar2018; Palmu et al., Reference Palmu, Salosensaari, Havulinna, Cheng, Inouye, Jain, Salido, Sanders, Brennan, Humphrey, Sanders, Vartiainen, Laatikainen, Jousilahti, Salomaa, Knight, Lahti and Niiranen2020; Verhaar et al., Reference Verhaar, Collard, Prodan, Levels, Zwinderman, Bäckhed, Vogt, Peters, Muller, Nieuwdorp and van den Born2020), and normalizing abundance of these with minocycline and captopril (Yang et al., Reference Yang, Santisteban, Rodriguez, Li, Ahmari, Carvajal, Zadeh, Gong, Qi, Zubcevic, Sahay, Pepine, Raizada and Mohamadzadeh2015; Li et al., Reference Li, Yang, Richards, Pepine and Raizada2020) lowered BP, further supporting their associations with BP.
Dietary interventions have also been used to study the relationship between gut microbiota and BP. Dietary salt can modulate the composition of microbiota by depleting the abundance of beneficial microbiota including several Lactobacilli species (Mell et al., Reference Mell, Jala, Mathew, Byun, Waghulde, Zhang, Haribabu, Vijay-Kumar, Pennathur and Joe2015; Wilck et al., Reference Wilck, Matus, Kearney, Olesen, Forslund, Bartolomaeus, Haase, Mähler, Balogh, Markó, Vvedenskaya, Kleiner, Tsvetkov, Klug, Costea, Sunagawa, Maier, Rakova, Schatz, Neubert, Frätzer, Krannich, Gollasch, Grohme, Côrte-Real, Gerlach, Basic, Typas, Wu, Titze, Jantsch, Boschmann, Dechend, Kleinewietfeld, Kempa, Bork, Linker, Alm and Müller2017; Bier et al., Reference Bier, Braun, Khasbab, Di Segni, Grossman, Haberman and Leibowitz2018; Yan et al., Reference Yan, Jin, Su, Yin, Gao, Wang, Zhang, Bu, Wang, Zhang, Wang and Zhang2020). An association between the depletion of Lactobacillus and HTN has also been noted in response to maternal and post-weaning high-fat diet, and it suggested that Lactobacillus may be beneficial in curbing developmental HTN (Tain et al., Reference Tain, Lee, Wu, Leu and Chan2018; Hsu et al., Reference Hsu, Hou, Lee, Chan and Tain2019). Although the mechanisms remain to be clarified, administration of Lactobacillus murinus prevented the expansion of proinflammatory IL-17A-producing CD4+ TH17 lymphocytes in small intestine, colon, and the splenic lamina propria (Wilck et al., Reference Wilck, Matus, Kearney, Olesen, Forslund, Bartolomaeus, Haase, Mähler, Balogh, Markó, Vvedenskaya, Kleiner, Tsvetkov, Klug, Costea, Sunagawa, Maier, Rakova, Schatz, Neubert, Frätzer, Krannich, Gollasch, Grohme, Côrte-Real, Gerlach, Basic, Typas, Wu, Titze, Jantsch, Boschmann, Dechend, Kleinewietfeld, Kempa, Bork, Linker, Alm and Müller2017). Data from human studies with Lactobacillus are however conflicting, as they may be enriched or depleted in hypertensive patients (Wilck et al., Reference Wilck, Matus, Kearney, Olesen, Forslund, Bartolomaeus, Haase, Mähler, Balogh, Markó, Vvedenskaya, Kleiner, Tsvetkov, Klug, Costea, Sunagawa, Maier, Rakova, Schatz, Neubert, Frätzer, Krannich, Gollasch, Grohme, Côrte-Real, Gerlach, Basic, Typas, Wu, Titze, Jantsch, Boschmann, Dechend, Kleinewietfeld, Kempa, Bork, Linker, Alm and Müller2017; Palmu et al., Reference Palmu, Salosensaari, Havulinna, Cheng, Inouye, Jain, Salido, Sanders, Brennan, Humphrey, Sanders, Vartiainen, Laatikainen, Jousilahti, Salomaa, Knight, Lahti and Niiranen2020; Silveira-Nunes et al., Reference Silveira-Nunes, Durso, Cunha, Maioli, Vieira, Speziali, Corrêa-Oliveira, Martins-Filho, Teixeira-Carvalho, Franceschi, Rampelli, Turroni, Brigidi and Faria2020; Wan et al., Reference Wan, Jiang, Lu, Tong, Zhou, Li, Yuan, Wang and Li2020; Liu et al., Reference Liu, Jiang, Liu, Shen, Ai, Zhu and Zhou2021b).
Gut metabolities, derived either from gut microbiota or involving both gut microbiota and host is of growing interest in the context of HTN. One such important class of microbial metabolites is short-chain fatty acid (SCFA). SCFAs such as acetate, propionate and butyrate are produced by bacterial fermentation of dietary carbohydrates and have been linked with BP regulation. It is reported that decreased SCFA production and the supplementation of SCFA lowered BP in rat and mouse HTN models, indicating the potential for antihypertensive therapy (Marques et al., Reference Marques, Nelson, Chu, Horlock, Fiedler, Ziemann, Tan, Kuruppu, Rajapakse, el-Osta, Mackay and Kaye2017; Kim et al., Reference Kim, Goel, Kumar, Qi, Lobaton, Hosaka, Mohammed, Handberg, Richards, Pepine and Raizada2018; Bartolomaeus et al., Reference Bartolomaeus, Balogh, Yakoub, Homann, Markó, Höges, Tsvetkov, Krannich, Wundersitz, Avery, Haase, Kräker, Hering, Maase, Kusche-Vihrog, Grandoch, Fielitz, Kempa, Gollasch, Zhumadilov, Kozhakhmetov, Kushugulova, Eckardt, Dechend, Rump, Forslund, Müller, Stegbauer and Avery2019; Robles-Vera et al., Reference Robles-Vera, Toral, la Visitación, Sánchez, Gómez-Guzmán, Romero, Yang, Izquierdo-Garcia, Jiménez, Ruiz-Cabello, Guerra-Hernández, Raizada, Pérez-Vizcaíno and Duarte2020c). In deoxycorticosterone acetate (DOCA)-salt-induced HTN, high-fiber diet lowered BP and enriched abundance of gut microbes producing acetate (Marques et al., Reference Marques, Nelson, Chu, Horlock, Fiedler, Ziemann, Tan, Kuruppu, Rajapakse, el-Osta, Mackay and Kaye2017). Interestingly, higher fecal levels of SCFA were associated with hypertensive individuals compared to normotensives (de la Cuesta-Zuluaga et al., Reference de la Cuesta-Zuluaga, Mueller, Álvarez-Quintero, Velásquez-Mejía, Sierra, Corrales-Agudelo, Carmona, Abad and Escobar2018; Huart et al., Reference Huart, Leenders, Taminiau, Descy, Saint-Remy, Daube, Krzesinski, Melin, de Tullio and Jouret2019; Calderón-Pérez et al., Reference Calderón-Pérez, Gosalbes, Yuste, Valls, Pedret, Llauradó, Jimenez-Hernandez, Artacho, Pla-Pagà, Companys, Ludwig, Romero, Rubió and Solà2020). Further, increased fecal SCFA was accompanied by decreased plasma SCFA and depleted butyrate-producing bacteria which suggests dysregulated production of SCFA in HTN condition (Calderón-Pérez et al., Reference Calderón-Pérez, Gosalbes, Yuste, Valls, Pedret, Llauradó, Jimenez-Hernandez, Artacho, Pla-Pagà, Companys, Ludwig, Romero, Rubió and Solà2020). The translational relevance and progress of animal as well as clinical studies in SCFA and BP have resulted in a clinical trial to determine the full efficacy of SCFA to treat HTN (Australian New Zealand Clinical Trials Registry ACTRN12619000916145). This phase II clinical trial used two SCFAs, acetate and butyrate which were supplemented with high-amylose maize, and the patients receiving the treatment showed 24-h BP lowering effect with the increase in gut microbes producing SCFA (Jama et al., Reference Jama, Rhys-Jones, Nakai, Yao, Climie, Sata, Anderson, Creek, Head, Kaye, Mackay, Muir and Marques2023), which is another evidence of the promising potential of targeting gut microbiota in HTN treatment. Another notable microbial metabolite is trimethylamine-N oxide (TMAO). Trimethylamine is produced by gut microbiota, and subsequently oxidized in the liver to form TMAO. Studies have shown the associations between higher plasma levels of TMAO and CVDs (Koeth et al., Reference Koeth, Wang, Levison, Buffa, Org, Sheehy, Britt, Fu, Wu, Li, Smith, DiDonato, Chen, Li, Wu, Lewis, Warrier, Brown, Krauss, Tang, Bushman, Lusis and Hazen2013, Reference Koeth, Lam-Galvez, Kirsop, Wang, Levison, Gu, Copeland, Bartlett, Cody, Dai, Culley, Li, Fu, Wu, Li, DiDonato, Tang, Garcia-Garcia and Hazen2019; Wang et al., Reference Wang, Tang, Buffa, Fu, Britt, Koeth, Levison, Fan, Wu and Hazen2014). In a meta-analysis involving human studies, higher circulating TMAO concentration was positively associated with an increased risk of HTN (Ge et al., Reference Ge, Zheng, Zhuang, Yu, Xu, Liu, Xi, Zhou and Fan2020). TMAO feeding further increased BP and promoted vasoconstriction in angiotensin II-induced hypertensive mice (Jiang et al., Reference Jiang, Shui, Cui, Tang, Wang, Qiu, Hu, Fei, Li, Zhang, Zhao, Xu, Dong, Ren, Liu, Persson, Patzak, Lai, Wei and Zheng2021). Besides SCFA and TMAO, there are many microbiota-derived metabolites such as indole, indole-3-acetic acids and secondary bile acids among others, that may have significant roles in BP regulation (Huć et al., Reference Huć, Nowinski, Drapala, Konopelski and Ufnal2018; Chakraborty et al., Reference Chakraborty, Lulla, Cheng, McCarthy, Yeo, Mandal, Alimadadi, Saha, Yeoh, Mell, Jia, Putluri, Putluri, Sreekumar, Wenceslau, Kumar, Meyer and Joe2020a). The knowledge on the effects of these microbial metabolites is growing, however, the precise underlying working mechanisms remain largely unknown.
Gut microbiota restructured in HTN: Cause, consequence or adaptation?
While an association between the reprogramming of gut microbiota and HTN is established, whether gut dysbiosis is a cause or a consequence of HTN is an important question to focus on. Initial experiments were designed to address this question by using antibiotics to eliminate endogenous gut microbiota. However, such studies did not provide conclusive evidence for cause or consequence because different antibiotics affected BP differently depending on both the type of antibiotic and the rodent strain (Galla et al., Reference Galla, Chakraborty, Cheng, Yeo, Mell, Zhang, Mathew, Vijay-Kumar and Joe2018, Reference Galla, Chakraborty, Cheng, Yeo, Mell, Chiu, Wenceslau, Vijay-Kumar and Joe2020). More convincing evidence for microbiota to cause a BP effect was obtained using germ-free Sprague Dawley (SD) rats. We showed that these rats which lack microbiota are hypotensive, with a prominent loss of vascular tone (Joe et al., Reference Joe, McCarthy, Edwards, Cheng, Chakraborty, Yang, Golonka, Mell, Yeo, Bearss, Furtado, Saha, Yeoh, Vijay-Kumar and Wenceslau2020). These findings are the first to clearly demonstrate that the host requires gut microbiota for BP homeostasis and maintenance of vascular tone (Joe et al., Reference Joe, McCarthy, Edwards, Cheng, Chakraborty, Yang, Golonka, Mell, Yeo, Bearss, Furtado, Saha, Yeoh, Vijay-Kumar and Wenceslau2020). One caveat to these studies is that the model used is not hypertensive. To establish the cause-effect relationship between HTN and gut microbiota, animal models such as germ-free hypertensive rat models can be developed. Such hypertensive germ-free rats will allow for testing the hypothesis that lack of microbiota will render them resistant to HTN. Currently, the lack of germ-free hypertensive rats as tools is a technical barrier to understand whether microbiota cause HTN.
To examine the causality of gut dysbiosis, Adnan et al. (Reference Adnan, Nelson, Ajami, Venna, Petrosino, Bryan and Durgan2017) performed gut microbiota cross-transplants between WKY and spontaneously hypertensive stroke-prone rats (SHRSP, a rodent model of HTN associated with high incidence of stroke. They observed that a stable transplant of SHRSP gut microbiota to normotensive WKY recipients, by oral gavage, led to a significant elevation in BP (Adnan et al., Reference Adnan, Nelson, Ajami, Venna, Petrosino, Bryan and Durgan2017). Similar trend was observed in another study after fecal microbiotal transplantation from SHR to WKY (Toral et al., Reference Toral, Robles-Vera, de la Visitación, Romero, Sánchez, Gómez-Guzmán, Rodriguez-Nogales, Yang, Jiménez, Algieri, Gálvez, Raizada and Duarte2019b). As an alternative approach to oral gavage transplants, which involve exposure to antibiotics, Nelson et al. (Reference Nelson, Phillips, Ganesh, Petrosino, Durgan and Bryan2021) swapped WKY and SHRSP gut microbiota using a cross-fostering protocol. By fostering newborn rat pups with a dam of the opposite strain, SHRSP rats were populated with a WKY gut microbiota and vice versa. Under these conditions, WKY rats harboring SHRSP gut microbiota developed a significantly elevated BP in adulthood compared to WKY rats with native WKY microbiota. Conversely, adult SHRSP rats harboring the WKY gut microbiota presented with significantly lower BP compared to SHRSP with their native SHRSP microbiota (Nelson et al., Reference Nelson, Phillips, Ganesh, Petrosino, Durgan and Bryan2021). These data signify that initial colonization of gut microbiota is critical and has long-lasting consequences on host pathophysiology.
As highlighted above, research focus has shifted to better understanding the mechanisms of host-microbiota interactions. Emerging studies address molecular mechanisms and demonstrate how BP may be regulated by bacterial metabolites via effects on the aryl hydrocarbon receptor (Natividad et al., Reference Natividad, Agus, Planchais, Lamas, Jarry, Martin, Michel, Chong-Nguyen, Roussel, Straube, Jegou, McQuitty, le Gall, da Costa, Lecornet, Michaudel, Modoux, Glodt, Bridonneau, Sovran, Dupraz, Bado, Richard, Langella, Hansel, Launay, Xavier, Duboc and Sokol2018; Liu et al., Reference Liu, Miao, Deng, Vaziri, Li and Zhao2021a), and G-protein coupled receptors (Marques et al., Reference Marques, Mackay and Kaye2018; Xu and Marques, Reference Xu and Marques2022) among others, which may impact end organ functions of the kidney, vasculature, brain and heart. Emerging work from Durgan et al. shows that a new mechanism by which signals derived from the gut microbiota (i.e., metabolites, neurotransmitters, endotoxins) may be distributed throughout the host via packaging into outer membrane vesicles (OMVs). These OMVs are lipid-bound vesicles (as known as bacterial liposomes) released from the gut microbiota that are capable of crossing the gut barrier and entering the systemic circulation. Bacterial OMVs can carry a wide range of ‘cargo’ including proteins, lipids, and small RNA, that can be delivered to and exert effects on distant host cells. They have shown that OMVs from the SHRSP microbiota have unique protein and lipid cargo as compared to OMVs from the WKY microbiota. Additionally, they find that SHRSP OMVs gavaged to WKY rats leads to significant elevations in BP (Shi et al., Reference Shi, Shi, Phillips, Zhang, Ayyaswamy, Bryan and Durgan2021a).
Previous reports showed no gut dysbiosis in pre-hypertensive SHR (Santisteban et al., Reference Santisteban, Qi, Zubcevic, Kim, Yang, Shenoy, Cole-Jeffrey, Lobaton, Stewart, Rubiano, Simmons, Garcia-Pereira, Johnson, Pepine and Raizada2017; Yang et al., Reference Yang, Li, Oliveira, Goel, Richards, Pepine and Raizada2020), suggesting that gut dysbiosis may arise as a consequence of HTN. However, these studies demonstrated colonic changes in pre-hypertensive SHR indicating a dysregulated gut barrier before developing HTN. Future studies should address this more specifically. Nevertheless, transplant experiments show that gut dysbiosis contributes to HTN and that manipulation of gut microbiota can alleviate HTN, suggesting that gut microbiota could be a potential therapeutic target.
Gut microbiota as therapeutic targets
There is considerable excitement of targeting gut microbiota for translational applications as evident from ongoing clinical trials for microbiota-guided therapies for HTN (https://clinicaltrials.gov/ct2/results?cond=hypertension&term=minocycline&cntry=&state=&city=&dist=). In preclinical studies, our group recently proposed Faecalibacterium prausnitzii as a novel probiotic to attenuate chronic kidney disease (CKD) conditions, following demonstrated depletion of F. prausnitzii in CKD patients in eastern and western human hypertensive populations. Importantly, supplementation of F. prausnitzii in a CKD mouse model not only ameliorated renal dysfunction, renal inflammation, and the levels of uremic toxins, but also improved gut ecology and intestinal integrity (Li et al., Reference Li, Xu, Xu, Tang, Jiang, Li, Xia, Cui, Bai, Dai, Han, Li, Peng, Dong, Aryal, Manandhar, Eladawi, Shukla, Kang, Joe and Yang2022). Since F. prausnitzii has also been reported to be depleted in CVD (Jie et al., Reference Jie, Xia, Zhong, Feng, Li, Liang, Zhong, Liu, Gao, Zhao, Zhang, Su, Fang, Lan, Li, Xiao, Li, Li, Li, Li, Ren, Huang, Peng, Li, Wen, Dong, Chen, Geng, Zhang, Yang, Wang, Wang, Zhang, Madsen, Brix, Ning, Xu, Liu, Hou, Jia, He and Kristiansen2017; Aryal et al., Reference Aryal, Alimadadi, Manandhar, Joe and Cheng2020), and CVD and CKD are highly correlated, it is possible that enhancing F. prausnitzii could be beneficial for CVD, for which HTN is a major risk factor (Li et al., Reference Li, Xu, Xu, Tang, Jiang, Li, Xia, Cui, Bai, Dai, Han, Li, Peng, Dong, Aryal, Manandhar, Eladawi, Shukla, Kang, Joe and Yang2022). Supporting this notion, studies have shown that F. prausnitzii is significantly abundant in normotensive compared to hypertensive humans, demonstrating strong correlation of this specific microbe with BP (Yan et al., Reference Yan, Gu, Li, Yang, Jia, Chen, Han, Huang, Zhao, Li, Fang, Zhou, Guan, Ding, Wang, Khan, Xin, Li and Ma2017; Calderón-Pérez et al., Reference Calderón-Pérez, Gosalbes, Yuste, Valls, Pedret, Llauradó, Jimenez-Hernandez, Artacho, Pla-Pagà, Companys, Ludwig, Romero, Rubió and Solà2020). However, in contradiction, Faecalibacterium was more enriched in individuals with high BP (Joishy et al., Reference Joishy, Jha, Oudah, das, Adak, Deb and Khan2022). Therefore, there is a need to directly examine the potential of F. prausnitzii in rigorous animal model studies.
In addition to being considered as therapeutic agents, gut microbiota may be involved in the modulation of our responses to antihypertensive medications (Kyoung et al., Reference Kyoung, Atluri and Yang2022). The efficacy of angiotensin-converting enzyme (ACE) inhibitors is reportedly modulated by the gut microbiota (Kyoung and Yang, Reference Kyoung and Yang2022; Yang et al., Reference Yang, Mei, Tackie-Yarboi, Akere, Kyoung, Mell, Yeo, Cheng, Zubcevic, Richards, Pepine, Raizada, Schiefer and Joe2022). Our group has recently demonstrated that quinapril, which is absorbed in the gut and metabolized by esterases in the liver to yield an active metabolite in circulation is prematurely catabolized in the gut by microbiota. This led to reduced availability of the active metabolite, quinaprilatin in circulation, which was associated with reduced BP responses to oral administration of quinalapril (Yang et al., Reference Yang, Mei, Tackie-Yarboi, Akere, Kyoung, Mell, Yeo, Cheng, Zubcevic, Richards, Pepine, Raizada, Schiefer and Joe2022). We further identified that a specific microbiota, Coprococcus comes, contains a bacterial form of esterase and may be one of the culprits for the premature quinalapril degradation and reduction in its efficacy as a BP-lowering agent. Interestingly, a higher abundance of C. comes is present in the African American hypertensive population (Yang et al., Reference Yang, Mei, Tackie-Yarboi, Akere, Kyoung, Mell, Yeo, Cheng, Zubcevic, Richards, Pepine, Raizada, Schiefer and Joe2022) who are known to respond poorly to ACE inhibitor treatments compared to Caucasian hypertensive patients (Yang et al., Reference Yang, Mei, Tackie-Yarboi, Akere, Kyoung, Mell, Yeo, Cheng, Zubcevic, Richards, Pepine, Raizada, Schiefer and Joe2022). This proof-of-concept study implicates that gut microbiota is a crucial factor defining individualized responses to hypertensive medications that should be addressed in future efficacy studies of antihypertensive drugs.
Current limitations in microbiome research for HTN
While such physiological studies are clearly important, drawing conclusions about the role of individual microbes in BP regulation, based on 16S analysis alone, can be problematic. The issue stems from the fact that multiple species can carry out the same function (e.g., generate the same metabolite), also referred to as functional redundancy. This redundancy likely contributes to the disparate candidate bacteria identified across hypertensive models and research facilities (Table 1). Another limitation of 16S analysis is that it captures a limited portion of the bacterial genome (Lewis et al., Reference Lewis, Nash, Li and Ahn2021). These limitations can be addressed by sequencing of whole bacterial genomes known as metagenomic sequencing, which is an emerging area in HTN research (Walejko et al., Reference Walejko, Kim, Goel, Handberg, Richards, Pepine and Raizada2018; Shi et al., Reference Shi, Zhang, Abo-Hamzy, Nelson, Ambati, Petrosino, Bryan and Durgan2021b). It should be noted that at the current stage, it is a misnomer to use the term ‘microbiome’ until metagenomes are reported. With the advent of rapid and cost-effective technologies, progress in reporting of metagenomes is anticipated to provide a platform for association studies of metagenomes with HTN. Metagenomics however falls short in assessing activity of the identified bacterial genes. Thus, combining metagenomics with an assessment of the functional output from the microbiota (i.e., proteomics, metabolomics, lipidomics) can be especially powerful. A recent study by the Durgan laboratory examined the role of gut dysbiosis in the SHRSP model by combining metagenomics with metabolomics analysis of the cecal bacterial content and the host plasma. While metabolomics revealed significant reductions in cecal and plasma primary and secondary bile acids in the SHRSP, metagenomics pinpointed that specific genes encoding bacterial enzymes involved in bile acid transformation were also reduced in the SHRSP microbiota. Thus, assessing changes in microbiota function will be useful in the development of targeted approaches for the treatment of HTN.
One other limitation in elucidation of functional consequences of host-microbiota interactions is addressing the complexity of such interactions. Gut microbiota and the host have evolved for centuries to live in complete symbiosis. This means that they are mutually dependent for survival and homeostasis. For example, humans are not capable of degrading fiber (Kaoutari et al., Reference Kaoutari, Armougom, Gordon, Raoult and Henrissat2013; Cockburn and Koropatkin, Reference Cockburn and Koropatkin2016). Gut bacteria aid the host by fermentation of fiber, thus generating SCFAs which are the main source of energy for the host colonic epithelium (Koh et al., Reference Koh, De Vadder, Kovatcheva-Datchary and Bäckhed2016; Baxter et al., Reference Baxter, Schmidt, Venkataraman, Kim, Waldron and Schmidt2019). Thus, the reduction of beneficial SCFA-producing bacteria, as seen in human and rodent HTN (Yang et al., Reference Yang, Santisteban, Rodriguez, Li, Ahmari, Carvajal, Zadeh, Gong, Qi, Zubcevic, Sahay, Pepine, Raizada and Mohamadzadeh2015; Gomez-Arango et al., Reference Gomez-Arango, Barrett, McIntyre, Callaway, Morrison and Dekker Nitert2016; Kim et al., Reference Kim, Goel, Kumar, Qi, Lobaton, Hosaka, Mohammed, Handberg, Richards, Pepine and Raizada2018; Yang et al., Reference Yang, Magee, Colon-Perez, Larkin, Liao, Balazic, Cowart, Arocha, Redler, Febo, Vickroy, Martyniuk, Reznikov and Zubcevic2019b; Calderón-Pérez et al., Reference Calderón-Pérez, Gosalbes, Yuste, Valls, Pedret, Llauradó, Jimenez-Hernandez, Artacho, Pla-Pagà, Companys, Ludwig, Romero, Rubió and Solà2020; Overby and Ferguson, Reference Overby and Ferguson2021), disrupts the symbiotic host-microbiota relationship leading to disease. In return, the bacteria have most likely evolved by adapting and responding to the host, as evidenced by the effects of genetic host manipulations on gut bacterial composition (Yang et al., Reference Yang, Ahmari, Schmidt, Redler, Arocha, Pacholec, Magee, Malphurs, Owen, Krane, Li, Wang, Vickroy, Raizada, Martyniuk and Zubcevic2017; Bartley et al., Reference Bartley, Yang, Arocha, Malphurs, Larkin, Magee, Vickroy and Zubcevic2018). Recent studies have attempted to address the complexity of host-microbiota interactions in symbiosis and dysbiosis. Of note, a recent study using isotope tracing found that the host can regulate the composition of gut bacteria by allowing the passage of host-circulating metabolites into the gut (Zeng et al., Reference Zeng, Xing, Gupta, Keber, Lopez, Lee, Roichman, Wang, Neinast, Donia, Wühr, Jang and Rabinowitz2022). These host/gut cometabolites were found to be beta-hydroxybutyrate (BHB), lactate and urea, among other, which are preferentially utilized as nutrients by certain bacterial communities. Future studies should investigate how these cometabolites contribute to regulation of gut microbiota eubiosis and how this interaction reflects on BP regulation. We have recently shown that the circulating BHB and gut microbiota are both salt-responsive (Chakraborty et al., Reference Chakraborty, Galla, Cheng, Yeo, Mell, Singh, Yeoh, Saha, Mathew, Vijay-Kumar and Joe2018). Moreover, we found that circulating BHB was decreased with high salt feeding, and that supplementation with BHB alleviated salt-sensitive HTN, but the contribution of gut microbiota to BHB generation or the potential direct effect of BHB on gut microbiota in BP regulation remains unknown. Thus, gut microbiota may have coevolved with the host to produce, utilize and respond to a variety of the same metabolite-substrate-effectors, reflected in the expression of some of the same genes by the bacteria and the host (Bartley et al., Reference Bartley, Yang, Arocha, Malphurs, Larkin, Magee, Vickroy and Zubcevic2018; Yang et al., Reference Yang, Richards, Pepine and Raizada2018, Reference Yang, Mei, Tackie-Yarboi, Akere, Kyoung, Mell, Yeo, Cheng, Zubcevic, Richards, Pepine, Raizada, Schiefer and Joe2022; Hsu et al., Reference Hsu, Yu, Lin, Tiao, Huang, Hou, Chang-Chien, Lin and Tain2022). Thus, the utilization of combined omics, employed at both microbiota and host levels, will lead to better predictions and targeting of host-microbiota interactions for therapeutics.
In conclusion, as noted through the numbers of studies reported in PubMed, microbiota is an emerging and important research area in HTN, surpassing that of GWAS and QTL studies of HTN (Figure 1a,b). Although research is still in early conception, given that the gut metagenomes co-evolve with the host and are critical for BP regulation, risk prediction for HTN using a PRS may be more informative in combination with new bacterial analysis approaches leading up to a ‘MRS’ that encompass both the metagenomic profiles and the functional bacterial readouts. The groundwork required for accumulating metagenomic signatures is admittedly daunting because of the fluctuating nature of microbiota, but collecting these data is important for its ultimate convergence with PRS for enhancing predictive strategies for HTN. Such an endeavor demands intense computational analyses that may only be addressable with AI strategies.
An application of AI and machine learning in HTN research
AI refers to methods for transferring human intellect to computers that can stimulate human learning and thought processes by using sophisticated algorithms and powerful computing capacity to process large amounts of data (Chaikijurajai et al., Reference Chaikijurajai, Laffin and Tang2020; Tsoi et al., Reference Tsoi, Yiu, Lee, Cheng, Wang, Tay, Teo, Turana, Soenarta, Sogunuru, Siddique, Chia, Shin, Chen, Wang and Kario2021). Machine learning (ML) and deep learning (DL) are the two subclasses of AI (Tsoi et al., Reference Tsoi, Yiu, Lee, Cheng, Wang, Tay, Teo, Turana, Soenarta, Sogunuru, Siddique, Chia, Shin, Chen, Wang and Kario2021). ML finds the association between the provided training datasets with variables and then performs predictive analyses on the new sets of data (Tsoi et al., Reference Tsoi, Yiu, Lee, Cheng, Wang, Tay, Teo, Turana, Soenarta, Sogunuru, Siddique, Chia, Shin, Chen, Wang and Kario2021). ML is further classified into supervised and unsupervised learning (Chaikijurajai et al., Reference Chaikijurajai, Laffin and Tang2020). Supervised ML relies on the labeled input–output paired data which is then used for the prediction of known output (Cheng et al., Reference Cheng, Manandhar, Aryal and Joe2011). It employs a variety of methods, including neural networks, support vector machines, random forest and naive Bayes (Cheng et al., Reference Cheng, Manandhar, Aryal and Joe2011). On the other hand, unsupervised ML employs unlabeled datasets to predict unknown outputs by detecting underlying patterns or correlations among the variables (Cheng et al., Reference Cheng, Manandhar, Aryal and Joe2011; Chaikijurajai et al., Reference Chaikijurajai, Laffin and Tang2020). The principal use of DL is pattern recognition, such as in voice and image analysis (Chaikijurajai et al., Reference Chaikijurajai, Laffin and Tang2020).
AI is increasingly being used in human HTN research (Figure 1b). Recent studies have shown how AI has the ability to reduce the worldwide burden of HTN and promote the development of HTN-related precision medicine (Golino et al., Reference Golino, Amaral, Duarte, Gomes, Soares, Reis and Santos2014; Ye et al., Reference Ye, Fu, Hao, Zhang, Wang, Jin, Xia, Liu, Zhou, Wu, Guo, Zhu, Li, Culver, Alfreds, Stearns, Sylvester, Widen, McElhinney and Ling2018; Lacson et al., Reference Lacson, Baker, Suresh, Andriole, Szolovits and Lacson2019; Kanegae et al., Reference Kanegae, Suzuki, Fukatani, Ito, Harada and Kario2020; López-Martínez et al., Reference López-Martínez, Núñez-Valdez, Crespo and García-Díaz2020; Soh et al., Reference Soh, Ng, Jahmunah, Oh, San and Acharya2020; Schrumpf et al., Reference Schrumpf, Frenzel, Aust, Osterhoff and Fuchs2021). As a result, the main goal of these investigations is to enhance the clinical management of HTN. Persell et al. conducted a randomized clinical trial of 297 persons with uncontrolled HTN to examine the impact of AI, smartphone coaching apps monitoring systolic BP and HTN-associated behavior. At the 6-month follow-up, the researchers did not discover decreased BP, but they did create a space for the possibility of different treatment effects among age subgroups (Persell et al., Reference Persell, Peprah, Lipiszko, Lee, Li, Ciolino, Karmali and Sato2020). Pan et al. (Reference Pan, He, Chen, Pu, Zhao and Zheng2019) coupled auscultatory waveforms data with ML to measure BP from Korotkoff sound recordings and examine the impact of movement disturbance on BP regulation. Among 40 healthy volunteers, their brand-new DL-based automatic BP measurement instrument showed encouraging accuracy in BP monitoring both when resting and not resting (Pan et al., Reference Pan, He, Chen, Pu, Zhao and Zheng2019). In 965 participants, Li et al. employed ML to identify genetic and environmental risk factors for HTN. To identify risk factors for HTN in the Northern Han Chinese population, they created two separate models for systolic BP (composed of age, body mass index, waist circumference, exercise [times per week], parental history of HTN [either or both], and 1 SNP (rs7305099)) and diastolic BP {composed of weight, drinking, exercise [times per week], triglyceride, parental history of HTN [either or both] and 3 SNPs (rs5193, rs7305099, rs3889728)} with an AUC (area under the curve) of 0.673 and 0.817 for the systolic BP and diastolic BP models respectively (Li et al., Reference Li, Sun, Liu, Li, Zhang, Liu, Wang, Wen and Zhou2019a). Future use of these AI/ML technologies to HTN may be combined to create a ‘clinical risk score’ (CRS).
To investigate the multifactorial causes of high BP, Louca et al. recently combined environmental, dietary, genetic, metabolite, biochemical and clinical data from two different cohorts. Then they applied the ML XGBoost algorithm to this multimodal domain. They included 4,863 TwinsUK subjects for the study and used 2,807 subjects from the Qatari Biobank to validate their findings. They discovered 30 overlapping features between the two groups, including age, BMI, sex, dihomo-linolenate, urate, cis-4-decenoyl cartinine, lactate, glucose, cortisol, chloride, histidine and creatinine to be associated with HTN. These BP biomarkers are crucial for prioritizing mechanistic investigations and for finding effective novel therapies for HTN (Louca et al., Reference Louca, Tran, Toit, Christofidou, Spector, Mangino, Suhre, Padmanabhan and Menni2022). Although this research examined a number of significant CRS and PRS domains to pinpoint the critical elements involved in the regulation of BP, gut microbiota features, which are crucial for building MRS, were not taken into account. Nakai et al. in their recent study performed the first gut microbiome multisite study involving 70 human subjects with HTN and without HTN. The authors combined ML with microbiome pathway analysis and reported differential microbial gene pathways between hypertensive and normotensive participants despite similar gut microbiota profile (Nakai et al., Reference Nakai, Ribeiro, Stevens, Gill, Muralitharan, Yiallourou, Muir, Carrington, Head, Kaye and Marques2021).
As one of the initial steps toward application of AI/ML in development of a MRS for HTN, our group had recently interrogated if the composition of microbiota may be used to classify patients with or without CVDs (Aryal et al., Reference Aryal, Alimadadi, Manandhar, Joe and Cheng2020). Due to the lack of information on the status of HTN in the American Gut Project, we resorted to classification of a broader group of patients. Using the top operational taxonomic unit features obtained from fecal 16S ribosomal RNA sequencing data of 478 CVD and 473 non-CVD human subjects, random forest, a supervised ML algorithm, was able to correctly classify between patients with CVD and without CVD, with an AUC value of 0.70. It denotes the prospective capacity of ML for case and control distinction (Aryal et al., Reference Aryal, Alimadadi, Manandhar, Joe and Cheng2020). Considering the wide range of variability in binning CVD as a single phenotype, an AUC of 0.7 further signifies that microbiota contribute to CVD, and that an association between disease and microbiota can be identified using AI. To move closer to the eventual objective of creating MRS for HTN, such data are required in the context of HTN.
Beyond its usage in healthcare, AI/ML can be used to understand GWAS results by spotting intricate underlying data patterns that make predictions easier. Such methodology improved the prediction of PRS for height, body mass index and diabetes (Paré et al., Reference Paré, Mao and Deng2017). Since there exist high-quality GWAS data for HTN, there is a possibility that similar AI/ML methodologies will be merged with CRS and MRS to improve the translational capacities of PRS for HTN (Figure 2).
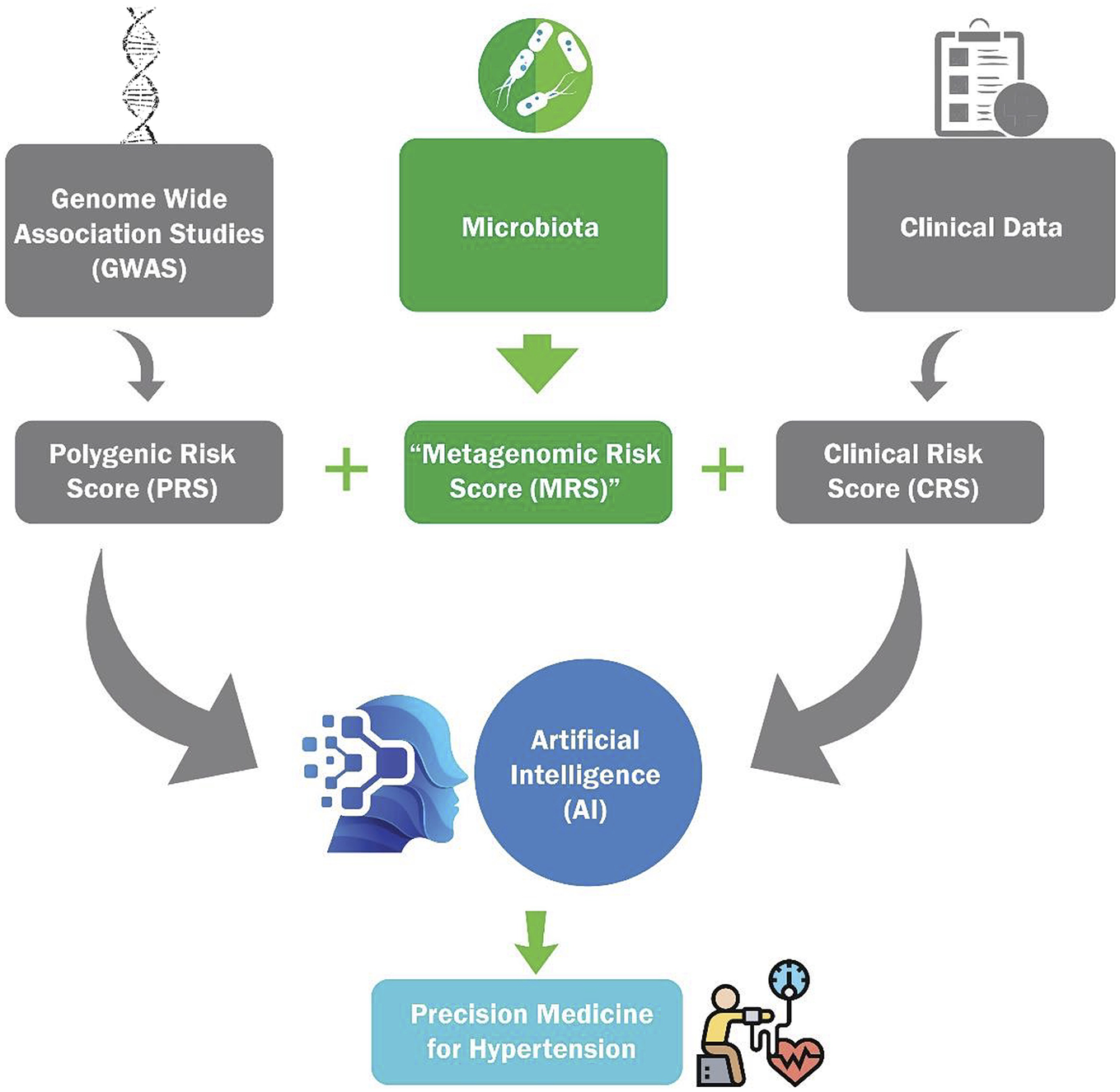
Figure 2. The integration of polygenic risk score, metagenomic risk score and clinical risk score using artificial intelligence is required for the precision medicine in hypertension.
Limitations of AI in HTN research
Although the use of AI in HTN has the potential to revolutionize risk prediction, this goal has significant constraints as listed below: (i) There are currently no standards for reporting AI investigations in HTN cases with sufficient rigor. In many publications, for instance, external validation datasets are not used. Very few research articles report the model calibration metrics and, bias brought about by algorithms is typically disregarded (Du Toit et al., Reference du Toit, Tran, Deo, Aryal, Lip, Sykes and Padmanabhan2023). (ii) There is a paucity of open-access databases that provide information on the genotypic and phenotypic characteristics of HTN. (iii) a major current limitation is that large cohort data containing both genomic and microbiota data are lacking. (iv) AI/ML operates in a ‘black box’ (i.e., it is unclear how it does what it does), which is claimed to be the main reason why physicians are reluctant to implement AI technology in clinical practice (Cheng et al., Reference Cheng, Manandhar, Aryal and Joe2011). (v) the interpretability of the AI models, the absence of cause-and-effect reasoning, the capacity to self-monitor errors, and the presence of societal biases are a few more drawbacks (Padmanabhan et al., Reference Padmanabhan, Tran and Dominiczak2021).
Some solutions for the limitations mentioned above could be to (i) develop easily interpretable AI models which can discern the relationship between the variables contributing to HTN, (ii) promote initiatives for setting up large-scale and rapid data-sharing of large cohort data specifically pertinent to HTN and (iii) development of standardized methodologies to control for rigor of human judgment into the AI systems for determining errors (Padmanabhan et al., Reference Padmanabhan, Tran and Dominiczak2021).
In conclusion, this review has summarized the mounting evidence that BP is closely correlated with the microbiota, which make up the second-largest genome after the host genome. In light of this borgeoning evidence, we propose exploiting such data for the development of a MRS as a predictive index for HTN. Additionally, we propose using MRS as part of a larger framework that incorporates PRS and CRS to build an AI-based model. Considerable research efforts to generate MRS may serve as a tool to enhance the existing, primarily insufficient predictive capability for the management of HTN.
Open peer review
To view the open peer review materials for this article, please visit http://doi.org/10.1017/pcm.2023.13.
Data availability statement
Data availability is not applicable to this article as no new data were created or analyzed in this study.
Acknowledgments
The authors thank Mr. Sarbesh Rijal, MS, Nepal for generating Figure 2. Graphical abstract was created with BioRender.com.
Author contribution
Conceptualization: S.A., I.M., B.J.; Drafting the original manuscript: S.A., I.M., B.S.Y., J.Z., D.J.D., M.V.-K., B.J.; Revision, editing and final approval of the manuscript: S.A., I.M., X.M., B.S.Y., R.T., P.S., I.O., J.Z., D.J.D., M.V-K., B.J.
Financial support
This work was supported by the National, Heart, Lung, and Blood Institute (NHLBI) (B.J., R01HL143082); the National Institutes of Health (NIH)-National Cancer Institute (NCI) grant (M.V.-K, R01CA219144); NHLBI and Research Corporation for Science Advancement (D.J.D., R01HL134838, RCSA27917); NHLBI (J.Z., R01HL152162); NHLBI (I.O., R00HL153896); American Heart Association Career Development Award (P.S., 855256) and American Heart Association Award (B.S.Y., 831112).
Competing interest
The authors declare none.
Comments
No accompanying comment.