1. Introduction
Customer Needs (CNs) are diversifying, and increasingly standardised mass-produced products fall short to answer these individual needs (Lin et al. Reference Lin, Yu, Zheng, Qiu, Wang and Xu2017). Besides the diverse customer requirements, increasing global competitiveness and dynamic market trends steer companies into the quest of serving individual customers at near mass-production costs (Paritala, Manchikatla, & Yarlagadda Reference Paritala, Manchikatla and Yarlagadda2017). In the past few decades, Mass Customisation (MC) has provided a solution by introducing modular and configurable products to answer the CNs better. Advancements in the production lines and supply chains has provided the necessary flexibility for MC to provide a variety of products at near mass efficiency (Tseng, Wang, & Jiao Reference Tseng, Wang and Jiao2017). Further advancements in industrial production and manufacturing systems create new possibilities and opportunities for product personalisation. The market desire for personalised products acts as a driving force in the paradigm shift towards smart manufacturing as envisioned in Industry 4.0, where manufacturing is on-demand, responsive and autonomous, using cyber-physical systems with advanced intelligence and flexibility (Lu, Xu, & Wang Reference Lu, Xu and Wang2020). The advancements in digital manufacturing technologies have enabled a more recent paradigm of mass personalisation (MP), which may be considered as a step further from MC (Tseng, Wang, & Jiao Reference Tseng, Wang and Jiao2017). MP differentiates from MC by expanding the design space, improving customer experience and enhancing creativity and innovation by customers (Sikhwal & Childs Reference Sikhwal and Childs2021). In the sense of product change and the needs addressed, MP is the extreme case of MC, as it aims to cater to the specific needs in a near-continuous design space (Wang et al. Reference Wang, Ma, Yang and Wang2017). Hence, it is beneficial where there can be value creation via exploiting personal needs and user experience, such as in consumer products. Besides, ideally, these needs should be addressed efficiently and cost-effectively. Therefore, the range of products within the applicability of MP is also affected by the availability and capability of digital manufacturing technologies. MP paradigm envisages changes in the basic design and structure of the product to meet the individual’s unique needs (Wang et al. Reference Wang, Ma, Yang and Wang2017). Digital manufacturing provides the necessary flexibility to allow such profound changes in the product, which enables tailoring products to customers with near mass production efficiency. As the products adapt more to customers, their involvement in the design process becomes more significant. The individual needs of the customer has to be transmitted to the design effectively. Therefore, the management of the variety provided through basic design and structure changes, and the effective customer involvement in design should be considered while designing products for MP.
The applications of MP are still limited to date; few successful applications are present in medical products, such as hearing aids and orthodontic aligners. However, it is still very uncommon in consumer products, while rare applications are present in footwear, jewellery or figurines (Bingham Reference Bingham2018). One of the limitations in the application of MP is the limited design knowledge in this domain (Sikhwal & Childs Reference Sikhwal and Childs2021). Dedicated design methodologies for MP are limited, and the majority of other relevant design methodologies are based on product family design and platform-based product development, which are extensively studied for MC (Pirmoradi, Wang, & Simpson Reference Pirmoradi, Wang and Simpson2014). However, these methodologies consider product development in the traditional manufacturing perspective, and their considerations may not apply in the digital manufacturing context. Commonly, the product variety is provided through varying modules or components on a common platform, and each varying module fulfils a specific requirement. On the other hand, digital manufacturing may allow the integration of multiple functions and their working principles in even one component (Spallek & Krause Reference Spallek and Krause2016). Therefore, the variety can be created by adapting the basic design of the product, and not necessarily with varying components. Hence, the variety and commonality should be considered differently in this context. Besides, the variety-induced complexity is much less of an issue with the design freedom provided by manufacturing flexibility, which allows MP to provide necessary variety to answer individual needs of the customers (Lei et al. Reference Lei, Yao, Moon and Bi2016). A similar challenge in applying the existing methodologies to MP is present with the standard configuration structures. These configuration structures are also based on the modularity and commonality of components, and they define hierarchical relationships of the optional or common components. Since the variety is defined differently in the MP context, the standard configuration structures are insufficient for managing this. Similarly, in a standard configuration scenario, eliciting individual needs through customer design is a challenge as well. Consequently, both the product development and configuration processes in the existing methodologies fall short to apply MP effectively. Therefore, the process of designing a personalisable product and facilitating the user in its design require a dedicated design methodology for MP in the digital manufacturing context, to effectively apply MP and exploit its benefits for customers.
The present work aims to develop a dedicated design methodology for MP in the digital manufacturing context. Addressing the challenges mentioned above, the work focusses on the utilisation of manufacturing flexibility in the process of developing a personalisable product; and an effective customer co-creation process that answers to specific needs. The main goals are providing guidance throughout the development process of a user modifiable design and facilitating the user design in an effective way.
The proposed methodology explores the development of a seed design that structures variety or commonality by the basic design features that vary through manufacturing, instead of a modular architecture that vary through assembly. Hence, multiple varying functions and parameters are integrated in a seed design that is the overarching architecture that ensures such flexibility and adaptability to user’s expectations. The user is also given more control over an interactive co-creation process to finalise the design. The underlying idea is to let customers set their own priorities in design decisions and hence get more design freedom for those priorities. Therefore, the proposed methodology intends to answer how to define the development process of a seed design realisable by digital manufacturing, and how to structure the co-creation process to answer the specific CNs better.
In the first part, the methodology describes the development process of a seed design that is used as a starting point for customer design. In the second part of the methodology, how users will interact with this template is explained, and a design solution algorithm is proposed to manage the interaction of the user with the design, to solve coupled design cases and to automate obtaining personalised designs. This bases on the real-time interaction of the users with a dynamic design space that updates with each user decision.
The proposed methodology contributes to design for MP by presenting a systematic way to personalise products in a mass manner. With a structured and systematic approach, the application of MP may be broadened to a wider range of consumer products. The methodology exploits the design freedom provided by manufacturing flexibility to answer specific CNs. In the following sections, first, background information on MP and relevant design approaches are presented. Then the proposed MP framework, the seed design development, and personalised design solution processes are explained. Finally, the proposed methodology is illustrated over saxophone mouthpiece and knitted footwear personalisation applications.
2. Background
Product differentiation to gain leverage in the market is not a new approach. Age-old artisanal practice of tailor-made products is an initial example. In mass production, this appears as market segmentation of products, where usually a single product is offered in each market segment. MC classifies customers into different segments and cluster the needs of the customers in the same market segment. Then, according to these clustered needs, customers are offered different product configurations within a predefined product family (Tseng, Jiao, & Merchant Reference Tseng, Jiao and Merchant1996). However, the limited variety of design options in MC often constraints answering CNs (Sikhwal & Childs Reference Sikhwal and Childs2021). In MP, CNs are fulfilled at the personal level. The basic product design and features may change to provide unique products tailored to each individual (Tseng, Jiao, & Wang Reference Tseng, Jiao and Wang2010). It is also important to point out the difference of personalisation and MP. Product personalisation dates long back to artisanal made-to-order products. The craftsmanship and additional labour of this process naturally result in a high price tag. Whereas, MP is about affordable personalisation, which makes it an interesting and viable strategy for businesses (Kumar Reference Kumar2007).
As the level of change in the product differs, the CNs addressed by different mass market strategies change as well. The CNs can be categorised as generic and specific needs. In mass production, generic CNs of a market segment are identified, and relatively important needs for the majority are implemented into the product (Ulrich & Eppinger Reference Ulrich and Eppinger2012). MP on the other hand, primarily addresses the specific, mainly affective and cognitive, needs. Besides the informed customer decisions, unexpected needs of individuals are exploited (Zhou, Ji, & Jiao Reference Zhou, Ji and Jiao2013). By giving customers an active and direct role in the design process, MP may meet CNs effectively (Sikhwal & Childs Reference Sikhwal and Childs2021).
The needs that MP addresses are not only related to the product, but also to the experience of the personalisation process. Since in MP, customers actively take part in the design process, by co-creating a personal product (Wang et al. Reference Wang, Ma, Yang and Wang2017). The product is unfinished when the customer involves, and the unknown and changing customer requirements necessitate an agile approach for MP (Aheleroff et al. Reference Aheleroff, Philip, Zhong and Xu2019). The real-time interaction of the customer with the design is necessary in the development, and this also contributes to the experience and satisfaction (Kumar Reference Kumar2007; Zhou, Ji, & Jiao Reference Zhou, Ji and Jiao2013). Aheleroff et al. (Reference Aheleroff, Philip, Zhong and Xu2019) also pointed out the importance of the real-time interaction in terms of informing the customer of any extra cost associated with a product specification. This co-creation activity is also a value creation process, via both utilitarian and hedonic innovation. Besides the augmented usability value of the product, there are also sensorial, emotional and symbolic values contribute to the user experience (Zheng et al. Reference Zheng, Yu, Wang, Zhong and Xu2017). Zhao et al. (Reference Zhao, McLoughlin, Adzhiev and Pasko2019) also suggest that a co-creation approach may increase perceived usefulness, enjoyment and satisfaction. The advanced customer participation also requires MP to be considered not only from a product development perspective, but also to be handled as a service design task (Hsiao et al. Reference Hsiao, Chiu, Chu and Chen2015).
MP aims for a market of one, and it relies more on value creation than efficiency. However, there is a limit of the created value outperforming the cost. Previous research confirms that personalisation indeed adds value for customers; however, their willingness to pay for this added value is up to 30% more compared to mass-produced counterparts (Boër & Dulio Reference Boër and Dulio2007; Abdul Kudus Reference Abdul Kudus2017). Therefore, the mass efficiency is still needed to be sustained. The major cost drivers of MP are the design personalisation process, and manufacturing. The flexibility of digital manufacturing is a prerequisite for MP, while automation of design personalisation is a major necessity to achieve mass efficiency.
Moving from mass production to MP, each new paradigm takes forward the concept of the previous and requires more responsive manufacturing systems (Hu Reference Hu2013). MC paradigm mainly relies on the traditional mass production methods, and flexibility in the supply chain and assembly processes allows different product configurations (Chen & Tseng Reference Chen and Tseng2007). It bases on the modularity and commonality of products to provide variance for customer (Albers et al. Reference Albers, Bursac, Scherer, Birk, Powelske and Muschik2019). In MP, the flexibility needed is at the lowest level of manufacturing. Industry 4.0 introduces information and communication technologies into manufacturing to develop smart factories with intelligent and adaptable processes. The Industry 4.0 roadmap includes the ability to produce affordable and highly personalised products with reasonable lead-time (Aheleroff et al. Reference Aheleroff, Philip, Zhong and Xu2019). The envisioned smart manufacturing enables mass personalised production with highly flexible processes (Gerrikagoitia et al. Reference Gerrikagoitia, Unamuno, Urkia and Serna2019). Lu, Xu, & Wang (Reference Lu, Xu and Wang2020) describe smart manufacturing as ‘fully-integrated, collaborative and responsive operations that respond in real-time to meet changing demands and conditions in the factory, in the supply network, and in customer needs via data-driven understanding, reasoning, planning, and execution of all aspects of manufacturing processes, facilitated by the pervasive use of advanced sensing, modeling, simulation, and analytics technologies’. Digital manufacturing technologies are key components of the smart manufacturing paradigm for data-driven and flexible production. Digital manufacturing includes both additive and substractive methods that are controlled with computer-based systems integrating processes such as CAD, simulation, visualisation and analytics (Chong, Ramakrishna, & Singh Reference Chong, Ramakrishna and Singh2018). The value of digital manufacturing becomes more evident as the product, and its manufacturing, gets more complex (Paritala, Manchikatla, & Yarlagadda Reference Paritala, Manchikatla and Yarlagadda2017). Changes in product are at the basic design level in MP, which makes digital manufacturing an essential enabler. Hence, the design considerations should also be accordingly to integrate design and manufacturing seamlessly. From the Industry 4.0 perspective, although smart factories can support personalised products with efficiency, to completely exploit the benefits, products should be designed for smart manufacturing (Marcus Vinicius Pereira Pessoa 2020).
2.1. Design for MP
MP is often considered in the same context as MC for design approaches. However, as outlined previously, there are specific challenges, and points of attention, emerging with the MP paradigm. Understanding these challenges, and catering to the product development accordingly, is crucial to design for MP (DfMP). DfMP should consider all key dimensions of MP in connection during the product development process. Bingham (Reference Bingham2018) points out the lack of method and conceptual framework to generate personalised designs and effective interaction with digital manufacturing.
Most of the DfMP literature are rather descriptive frameworks. As one of the earliest works on DfMP, Tseng, Jiao, & Wang (Reference Tseng, Jiao and Wang2010) proposed a technical framework composed of customer, functional, physical, process and logistics domains. They suggest connecting these domains by utilising a what-how mapping. The customer domain contains known and latent needs, which are translated into functional requirements (FRs) in the functional domain via customer co-creation. A product ecosystem is also defined within the functional domain, which acts as a design space for the customer. Zheng et al. (Reference Zheng, Xu, Yu and Liu2017) proposed a similar approach for the definition of design domains, but in an adaptable open architecture product platform. In their framework of a personalised product configuration system, customers, original equipment manufacturers (OEM), and companies are three stakeholders in the process, in which OEMs and open architecture product platform are at the centre. Sikhwal & Childs (Reference Sikhwal and Childs2021) also proposed a product ecosystem including an open hardware platform, personalised modules and active customer participation. In this case, a web-based system facilitates the user design, while the product is produced by standard part manufacturers and personalised module vendors. Kumar (Reference Kumar2007) suggests that real-time customer interaction with the design space would allow more reliable measurement of customer satisfaction. Zhou, Ji, & Jiao (Reference Zhou, Ji and Jiao2013) highlighted the importance of obtaining the latent needs and processing them in real-time in design and solution spaces. They also proposed a DfMP framework focussing on affective and cognitive needs elicitation. There are also other perspectives on DfMP frameworks. Hsiao et al. (Reference Hsiao, Chiu, Chu and Chen2015) proposed a service design approach, proposing a model between customer, service platform and service provider. Largely focussing on the customer journey, they also include a customer satisfaction evaluation as a final phase. Zheng et al. (Reference Zheng, Yu, Wang, Zhong and Xu2017) suggested a user experience-based MP development, based on value creation via use generation and meaning delivery. They proposed a circular framework between UX, cyber and physical models.
The design for MC bases on product family and platform design methodologies. A product family is a set of products that share some common components and functions, which is called a product platform. The variety among the product family is provided by the modularity of components interfacing the product platform (Pirmoradi, Wang, & Simpson Reference Pirmoradi, Wang and Simpson2014). While commonality among the product family provides cost savings and standardisation, variety allows covering the needs of more customers (Albers et al. Reference Albers, Bursac, Scherer, Birk, Powelske and Muschik2019). The effort in product family design is to find an efficient trade-off between these two conflicting terms (Fixson Reference Fixson2007). There is also an effort to adapt product family approach for personalisation by introducing personalised modules as a third kind, in addition to product platform and varying modules. The personalised modules are produced customer-specific for each order and combined with the platform and variant modules. They also share a standard interface with the modular architecture, and they are decoupled as far as possible from other components for the ease of configuration process (Greve et al. Reference Greve, Oltmann, Schwenke, Krause, Gebhardt and Spallek2017). Another type of modules is a scalable one, which is similar to personalised modules, but a more primitive version. A scalable module provides variety by stretching or shrinking some of its parameters within a continuous range or discretely (Gauss, Lacerda, & Cauchick Miguel Reference Gauss, Lacerda and Cauchick Miguel2021). Same as personalised modules, scalable modules are also decoupled as far as possible, and remain at the bottom of the configuration hierarchy. Both of these type of modules may provide a certain degree personalisation to the product. However, they are still considered in a modular design perspective, as they still have standard interfaces to the platform, and functionally and structurally independent of the rest. The configuration of a scalable module is simpler, but the variety it provides is limited, though it may be sufficient in certain cases. Personalised modules may help more to adapt the product to the customer. However, the design of a personalised module is not elaborate and foreseen as an individual effort for each customer; while also considered as a separate development process from the family architecture (Spallek & Krause Reference Spallek and Krause2016). Hence, this approach is valid for partially personalised products, yet how to develop a personalised module for a MP scenario is still an open question.
The majority of the product development methodologies follow a fundamentally similar path from customer to process domains, and the differentiation is in how the domains are connected in requirements, specification and solution steps. The common process is, very briefly, identifying CNs first, then translating them into FRs, identifying the design parameters (DPs) fulfilling the FRs, and finally determining process variables (PV) for production. Similar processes are demonstrated by established product development methodologies (Pahl et al. Reference Pahl, Beitz, Feldhusen and Grote2007; Ulrich & Eppinger Reference Ulrich and Eppinger2012). In the design for MC, the process is adapted for product family design, by clustering the DPs serving the same set of FRs, to provide a variability in design (Tseng, Jiao, & Merchant Reference Tseng, Jiao and Merchant1996). This involves limiting the spread of FRs, in other words limiting the variance as a trade-off to reduce the related costs. Gauss, Lacerda, & Cauchick Miguel (Reference Gauss, Lacerda and Cauchick Miguel2021) highlight the lack of interaction between functional and physical domains in the majority of product family design methods, as the FRs and DPs are not decomposed concurrently. Similarly, Pirmoradi, Wang, & Simpson (Reference Pirmoradi, Wang and Simpson2014) state the necessity of considering interdependencies among different design elements as a challenge of product family design. There is also a disconnection between customer and functional domains, since the majority of the methods derive FRs from existing solutions, but not from CNs (Gauss, Lacerda, & Cauchick Miguel Reference Gauss, Lacerda and Cauchick Miguel2021). Jiao, Simpson, & Siddique (Reference Jiao, Simpson and Siddique2007) also points to a lack of customer modelling and integration in product family design. The variance of design possibilities in MP is much greater, which results in higher complexity in design specification and solution steps (Loureiro, Ferreira, & Messerschmidt Reference Loureiro, Ferreira and Messerschmidt2020). The complexity arises from the variables in the customer, functional and physical domains, and the interdependence of these variables. The main gap of DfMP literature arises at this point of how to structure these domains and manage the interactions in between, as further discussed in the limitations section below.
2.1.1. Design specification
The design specification process translates the requirement domain into the physical domain. In MP, this process is to construct how each user will interact with the design. The DPs forming a design template are identified in this step. Using a design template as a starting point of design, customisation or personalisation is a common practice in MC/MP literature. In product family design, the design template appears in the form of a product platform and a set of modular components in a product family architecture (Jiao, Simpson, & Siddique Reference Jiao, Simpson and Siddique2007). Aheleroff et al. (Reference Aheleroff, Philip, Zhong and Xu2019) defined adapting the design over a design template as the most important and value added part of MP. Bingham (Reference Bingham2018) refers to it as ‘seed object’ or ‘seed design’, and describes it as ‘the starting point for all subsequent transformations and is specifically created to allow some form of personalisation by the intended end-user/customer’. A similar definition is also found as meta-design, where designer acts as a facilitator by enabling the user modifications within the open design framework (Boisseau, Omhover, & Bouchard Reference Boisseau, Omhover and Bouchard2018). Lipton et al. (Reference Lipton, Schulz, Spielberg, Trueba, Matusik and Rus2018) proposed MC of carpentry products over a design template to be used by experts, where the proposed method verifies the design and fabricates by robotic systems. Shugrina, Shamir, & Matusik (Reference Shugrina, Shamir and Matusik2015) suggested a method to convert any parametric model to a seed design for online product configurators with which the user interacts in real time. The user is exposed to a few parameters to interact with, and real-time feedback of validity of the designs and valid parameter bounds are given to the user. From here on in this paper, the ‘seed design’ term is used to refer to design templates that users interact with. The definitions and examples of seed design have been highlighted by several authors, but how it should be designed for MP was not covered.
Despite not conceived for MP, axiomatic design (AD) can support the transformation process from customer domain to functional and physical domains. AD theory brings a systematic method to the design specification process (Suh Reference Suh2001). With a top-down approach, the variables of design domain (customer, functional, physical and process) are decomposed to clarify the design task. The domains are mapped to each other with the reasoning of ‘what to achieve’ and ‘how to achieve it’. According to AD, to achieve the best design solution, the independence and information axioms must be followed. The independence axiom suggests maintaining the independence of FRs by appropriate selection of DPs. Only uncoupled or decoupled design cases satisfy the independence axiom. The aim is to reduce the complexity, and thus avoid unintended consequences in the design solutions. Suh (Reference Suh2001) defines the complexity here as ‘a measure of uncertainty in achieving the specified FRs’. If multiple designs are found according to the independence axiom, then the best design with the minimum information content is chosen according to the information axiom (Brown Reference Brown2020). These axioms are utilised at each step of the decomposition.
Jiang et al. (Reference Jiang, Zhao, Yang, Zhao and Tan2007) explored the use of AD in the functional and physical decomposition of the product family architecture. Tseng, Jiao, & Merchant (Reference Tseng, Jiao and Merchant1996) adopted AD principles for product family design in MC, by introducing a product family architecture, where building blocks are defined to be configured to individual products. Marchesi & Matt (Reference Marchesi and Matt2017) exampled the adoption of AD in design for MC, with a study on the conceptual design of prefabricated housing. Salonitis pointed out the lack of manufacturing considerations in the functional and physical domains of AD, and proposed a modified method focussed on design for additive manufacturing (DfAM) (Salonitis Reference Salonitis2016). In the proposed method, process domain is also included in the zigzag decomposition process of FRs and DPs to include manufacturing guidelines in the early design phase.
As MP is strongly linked to additive manufacturing, it is often considered with a DfAM perspective. In DfAM approach, the process selection is taken as the first step prior to the design process (Zhu et al. Reference Zhu, Pradel, Bibb and Moultrie2017). Spallek, Sankowski, & Krause (Reference Spallek, Sankowski and Krause2016) categorise the development processes as standard individualisation and specific adaptation. Standard individualisation divides the product to individualised and nonindividual components, similar to modular components and common platforms in product family design for MC. While nonindividual components are developed for mass production, individualised ones are developed with a DfAM approach. Specific adaptation is a personalisation case, first the product structure is designed with an individualisation scope, then the design process is repeated for each customer within a fixed solution space, where DfAM considerations are present. However, this is not in a mass context, since the necessity of customer-specific design effort is highlighted. Although the specific adaptation is stated as true product individualisation, the authors underline the disadvantages of the difficulty in developing a seed design and the lack of corresponding knowledge in the literature. Ko, Moon, & Hwang (Reference Ko, Moon and Hwang2015) proposed a computational approach to connect DfAM with custom products. In the proposed method, the interrelations between AM considerations, CNs and product features are identified using finite state automata and affordance. Designing for AM enables integration of multiple functions and their working principles in one part. However, this is in contradiction with the design for variety approach in modularisation, which structures the variety with one-to-one mapping between FRs and components (Spallek & Krause Reference Spallek and Krause2016). Besides, the major consideration of compromising between commonality and variety becomes insignificant with the design freedom AM offers (Lei et al. Reference Lei, Yao, Moon and Bi2016). Consequently, the product family design approach is principally limited in both exploiting the benefits of AM and effective product development for MP.
Design structure matrix (DSM) is another approach to structure interdependences between different domains (Eppinger & Browning Reference Eppinger and Browning2012). Instead of the top-down approach with zigzag decomposition in AD, a bottom-up approach is taken by clustering or reordering elements in the matrix to simplify the design task. DSM is used by some product family architecture methods to decouple the design or to identify different modules by clustering the components (Seol et al. Reference Seol, Kim, Lee and Park2007; Li et al. Reference Li, Cheng, Feng and Yang2013; Bonev et al. Reference Bonev, Hvam, Clarkson and Maier2015). Yu & Cai (Reference Yu and Cai2009) employed DSM to show the hierarchical dependencies among structures and design processes in product family architecture, which is used in the configuration process to create product variants rapidly. Careful consideration of dependencies and correlations among the elements affecting design is important to avoid suboptimal design solutions (Pirmoradi, Wang, & Simpson Reference Pirmoradi, Wang and Simpson2014). Based on DSM, Loureiro, Ferreira, & Messerschmidt (Reference Loureiro, Ferreira and Messerschmidt2020) proposed the design structure network (DSN), a design specification method for MP. The method demonstrates the interdependencies of FRs and DPs visually on a network, decomposes them and suggests principles to manage the network complexity. While being the only truly prescriptive method for MP, DSN explains how to carry out the specification process up to decomposed DPs. In other terms, the DPs to realise the design becomes evident, but how to define or manage the variety is not covered. The previous studies consider the specification process from MC or DfAM perspectives mostly, or propose rather descriptive frameworks.
2.1.2. Design solution
In the design solution step, the requirements of a specific user are transferred to the DPs and a personalised design is obtained. The DPs form a solution space where different requirements can be met. To achieve mass efficiency, effective management of the solution space for the automation of personalised design generation is necessary. Gembarski & Lachmayer (Reference Gembarski and Lachmayer2018) proposed an approach for complexity management of solution space in MC, regarding two dimensions of variety and uncertainty. The approach is based on finding a balance between CNs and portfolio capabilities by increasing or reducing the complexity. The authors also proposed a solution space development tool for geometry-based design tasks (Gembarski & Lachmayer Reference Gembarski and Lachmayer2018). The tool aims to lay out the dependencies between requirements, parameters and restrictions on a matrix to support design solution decisions. Skjelstad et al. (Reference Skjelstad, Thomassen, Sjøbakk, Bakås, Blazek and Partl2018) introduced manufacturing considerations in solution space management for MC to balance the customer and manufacturing perspectives. They also identified solution space archetypes according to product form, fit and function.
The variety of the solution space result in higher design complexity, and this is one of the challenges of MP. In DfMP, the main driver of this complexity is the coupling of the design (Loureiro, Ferreira, & Messerschmidt Reference Loureiro, Ferreira and Messerschmidt2020). While this is not a case in product family design, since the dependence between FRs and DPs can only be uncoupled or decoupled (Gauss, Lacerda, & Cauchick Miguel Reference Gauss, Lacerda and Cauchick Miguel2021). Instead, this variety-induced complexity mainly arises from increasing variants in the product family (Greve et al. Reference Greve, Oltmann, Schwenke, Krause, Gebhardt and Spallek2017). Development of algorithms and approaches to handle this complexity may enhance the configuration process. Multidisciplinary design optimisation is one of these approaches for reconfiguration of the product family to obtain the desired performance and optimal configuration (Pirmoradi, Wang, & Simpson Reference Pirmoradi, Wang and Simpson2014). The literature on how to build a solution space and how to manage it is very limited and more focussed on modular architectures in MC. Besides, as also discussed below, the existing literature focusses more on manufacturing and production planning, rather than the user involvement.
In design for MC, the product portfolio is predefined by customer requirement patterns, and not by considering individual customers, which creates the risk of suboptimal fulfilment of personal needs. This is the result of a solution space with discrete options. The design freedom and production flexibility of AM enables customer-specific designs within a continuous solution space (Greve et al. Reference Greve, Oltmann, Schwenke, Krause, Gebhardt and Spallek2017). The end-product configuration in product family design requires a configuration structure. The generic bill-of-material is a common method to structure the hierarchical relationships of the components (Gauss, Lacerda, & Cauchick Miguel Reference Gauss, Lacerda and Cauchick Miguel2021). It is defined as a tree structure with common and optional nodes to obtain different configurations.
2.1.3. Limitations
The product family design methodologies for MC are mostly developed in the traditional manufacturing technology context, and they propose solutions or optimise processes accordingly (Lei et al. Reference Lei, Yao, Moon and Bi2016). Hence, their considerations or challenges are not necessarily valid for digital manufacturing context. For instance, the assumptions on how commonality and variety affect cost or complexity are no longer valid when AM is used. Similarly, the decomposition of domains and configuration structure are based on modularity and one-to-one mapping between functions and modules. However, AM allows multiple functions in a consolidated part. Hence, the platform and modular architecture is insufficient to develop a seed design for MP in AM context. Although personalised modules may offer a solution to meet individual needs, they are still within a modular architecture, which prevents exploiting the advantages of AM. Lack of customer integration and elicitation of specific CNs are also highlighted in the literature, which is a major pitfall for MP. Moreover, these methodologies do not consider coupled design cases between FRs and DPs. While this is a reasonable approach for a modular architecture, it might be noteworthy to consider coupled cases in the AM context. As decoupling a design in this context might not be as simple or restrict the variety significantly. Consequently, all these drawbacks reflect on the overall development process, and it should be adapted for MP in digital manufacturing context.
The majority of the literature on MP are descriptive frameworks of how MP should be on the system level, and they mostly focus on manufacturing perspective. In terms of guiding DfMP, to the authors knowledge, there is only one prescriptive contribution (Loureiro, Ferreira, & Messerschmidt Reference Loureiro, Ferreira and Messerschmidt2020); however, focussed on only design specification. Therefore, an overarching gap exists in guiding designers in the product development process of the MP paradigm. Different domains subjected to the DfMP process are similar to the traditional product methodologies, and sufficiently well identified. However, constructing these domains and managing their interactions to obtain personalised designs are still present challenges. The lack of knowledge in connecting these domains and structuring a seed design were previously pointed out (Spallek, Sankowski, & Krause Reference Spallek, Sankowski and Krause2016; Bingham Reference Bingham2018). The use of a seed design as a starting point for customer design is stated by several authors; however, how to develop a seed design in MP context has not been elaborated either.
The importance of real-time customer interaction with the design in co-creation is highlighted by several authors (Kumar Reference Kumar2007; Zhou, Ji, & Jiao Reference Zhou, Ji and Jiao2013; Aheleroff et al. Reference Aheleroff, Philip, Zhong and Xu2019). This especially becomes evident in a coupled design case, where the design solution should be iterated. Existing configuration structures do not cover this case, and also limited to the options of modules and components. While some methods introduce personalised components, these are independent nodes in the hierarchical structure, and their variety is not managed in the configuration (Gauss, Lacerda, & Cauchick Miguel Reference Gauss, Lacerda and Cauchick Miguel2021). Multiobjective optimisation is one solution for a coupled case to find the optimal configuration for the user. However, this results in an uninformed design trade-off for the user. For an effective and informed interaction of the user and the design, the co-creation process should link to the physical domain in a dynamic way. The literature is very limited on this; though Shugrina, Shamir, & Matusik (Reference Shugrina, Shamir and Matusik2015) proposed a description, it is limited to the dimensional parameters of the product.
The higher complexity, due to coupled requirements or parameters, in reaching a design solution in MP is another present challenge. While there is also variety-induced complexity in product family design, this is not necessarily on variant configuration, but more of a manufacturing and managerial complexity (Greve et al. Reference Greve, Oltmann, Schwenke, Krause, Gebhardt and Spallek2017). Hence, the differences in considerations is also a major obstacle in applying design for MC methods or solution space management approaches to MP. The increased design freedom that MP provides the user may result in a complexity in the design configuration structure, and this requires a particular attention.
Achieving mass efficiency is an essential aspect of MP. This is well supported by production system automation in smart manufacturing concepts. However, achieving mass efficiency requires manufacturing automation to be supported by design automation as well. Therefore, how the differentiation of personalised designs may be handled for production is rather clear, but how to reach those designs in a mass manner is an open question.
The present work addresses the following gaps, limitations and challenges:
(i) Developing seed design for MP in the digital manufacturing context.
(ii) Creating and structuring variety through the design of a product incorporating varying functions and design features.
(iii) Structuring customer, functional and physical domains and to manage their interactions effectively for MP.
(iv) Facilitating real-time user interaction with the design for effective and informed decisions.
(v) Managing the complexity in the solution space being inclusive of coupled design cases.
(vi) Achieving mass efficiency in generating personalised designs.
There are more challenges and open questions in MP, which are not covered in this work. MP relies on value creation, by not only the product, but also the personalisation process. Therefore, design of the co-creation process focussing on enhancing the user experience is necessary. The level of personalisation or the co-creation methods should be compatible with the product and customer profile. The work on these topics are very limited and, hence, the challenge in customer satisfaction with MP is still present.
3. Proposed methodology
While designing for MP, the product undergoes profound changes in an open process where the design is started by the designer and completed by the user. Therefore, MP development is not only designing the product, but also managing a dynamic process. The active involvement of the user in the process, managing this involvement in a mass manner and the complexity of tailoring a product requires a novel approach.
The proposal in this work is a prescriptive methodology that aims at guiding designers to develop seed designs in digital manufacturing context, and to facilitate customer co-creation effectively to reach truly personalised designs. Addressing the limitations listed in the previous section, the methodology;
(i) proposes a seed design architecture that contains variety through variable design features in its structure;
(ii) structures and manages the interaction between different design domains considering the proposed seed design architecture and customer co-creation;
(iii) integrates the customer co-creation considerations in the development process;
(iv) actively involves customers in the process with real-time design decisions; and
(v) employs an iterative approach for design personalisation to solve coupled cases.
The proposed methodology explains the development process from identified CNs, and starts with expressing these in more technical requirements. It does not cover the complete product development process, but only how to develop it for MP. Hence, the starting point is an existing product, or a concept design.
The importance of the needs, hence product features, is also largely a personal choice. In the proposed methodology, as opposed to the traditional approach, the hierarchy or the relative importance of the needs, thus the priority of corresponding requirements, are left to the user. Therefore, the user can reach trade-offs between features, knowing that there is more design freedom on the primary decisions. This is enabled by the real-time interaction between the co-creation tool and the solution algorithm. However, the user does not have direct control over the DPs, but does have control over the requirements from the product. Hence, the design is driven by requirements derived from personal needs and realised by the DPs.
In the following section, a DfMP framework is introduced to explain the envisioned MP scenario, clarifying the roles of the designer and the user. Following that, the designer is guided through the seed design development to set the stage for the user. In the final section, design solution, how to facilitate the user co-creation in a mass manner is elaborated.
3.1. Design for MP framework
The proposed framework (Figure 1) is composed of two phases. The initial phase is where the designer develops a user-modifiable seed design, and the latter phase is where the seed design is completed by each user. The seed design can fulfil varying personal requirements (PRs) within the design space, which are realised by varying DPs in its structure. The predefined and assured ranges of DPs form the solution space. The design process is completed by the user, where the product is personalised within the design space, which is mapped to the solution space. Hence, the design changes are dictated by the requirements, and realised by the DPs. The interaction between design and solution spaces, with a real-time feedback to co-creation interface, is managed by a design solution algorithm. A final design is generated when all the parameters in the solution space are set. The aim is to automate the process of generating personalised products, defining the seed design once, and then enabling each customer to complete the design.

Figure 1. Design for mass personalisation framework.
3.2. Seed design development
The seed design includes a core part which is stable, consolidated for a given product, referred here as base architecture (Figure 2). However, it is not a set of common components in this case, but common design characteristics. It is a concept design where a seed design is built upon. The seed design has also a set of variable features that gives to the design flexibility and the possibility to be personalised (Figure 2). These are again not varying components, but certain design characteristics. Hence, the seed design does not consist of platforms or modular components, but it is more of an integral and flexible architecture that contains common and varying aspects in its design. It includes a base architecture and the information on how its design could be modified. The varying design characteristics provide personalised features of the product. This section explains the development of the variable design features of the seed design and the idea behind.

Figure 2. Seed design decomposition into base architecture and variable design features, corresponding product features and customer needs. The greyed out branch is out of the scope of the proposed methodology.
The seed design allows keeping control over complexity, still having an almost continuous variety, or flexibility of product offering. It allows to offer an almost continuous variety of alternatives which help to give the customer most suitable product according to their needs. The aim is to ensure the user a virtually infinite set of options to maximise the fulfilment of their needs, without bringing back the complexity that derives from the management of configurations.
MP development pipeline is shown in Figure 3. The first phase of the development is in a sense designing an unfinished product. A seed design is developed as a starting point for customer involvement in the second phase. The process of the first phase starts with understanding differentiating CNs. These specific CNs are then expressed as personal requirements (PRs). Afterwards, the DPs to satisfy the PRs are identified. Following these, the dependencies and constraints on DPs are identified to find the ranges of DPs, and these form the solution space. The dependencies between the requirements and parameters are demonstrated on a matrix. The solution space is mapped back to PRs to form the design space to find the ranges of PRs.

Figure 3. Development pipeline for seed design and final personalised design.
The decomposition process starts with only top-level CNs, and these are translated into PRs. Then, PRs and DPs are decomposed to lower levels. Following that, the identified levels of PRs to receive customer input are returned to the customer domain again to be expressed in the customer language for co-creation.
In the second phase, the user interacts with the design via a co-creation tool. The user input is translated to PRs and the solution algorithm sets the DPs. The key point here is that as the user modifies the design having real-time feedback on the valid space for further design choices. To achieve a design solution by setting all DPs to satisfy the PRs, an iteration algorithm is used. To satisfy PRs, DPs are iterated with the main objective of providing the largest design space at each step. This results in a dynamic design space, where each user decision updates the valid range of further decisions. The development process is finalised with a personalised design when all the DPs are set.
3.2.1. Customer needs
Identifying CNs is an essential step in all product development processes. In the traditional means, certain generalisations on the user data are made for each market segment. These generalised needs are then organised into a hierarchy and their relative importance is established (Ulrich & Eppinger Reference Ulrich and Eppinger2012). It is necessary to highlight that ‘needs’ in this context refer to any customer need or desire for the product (Roozenburg & Eekels Reference Roozenburg and Eekels1995). While designing for MP, it is important to understand the differentiating CNs, in other terms, the specific needs. In this case, product-specific clusters of specific needs should be identified, which later define the extent of personalisation offer. The offerings of MP should be unique and personal, hence while setting the personalisable features of the product, the considerations should be inclusive of those needs. Two main pillars of MP are increased customer involvement in design, and the manufacturing flexibility allowing this; the extent of personalisation offering is strictly related to these. The value presented by MP is not only about the personalised product, but also the sensorial, emotional and symbolic values created in the co-creation process (Zheng et al. Reference Zheng, Yu, Wang, Zhong and Xu2017). For a better user experience, providing the appropriate level of personalisation is important (Ozdemir et al. Reference Ozdemir, Van Goethem, De Buysscher, Delrue, Verburgh, Van Gastel and Verlinden2020). The level or extent of the personalisation scenario is also related to the cost and manufacturing feasibility. Hence, the level of personalisation affects both customers and providers, and reaching a trade-off with affordability is necessary for both parties (Aheleroff et al. Reference Aheleroff, Philip, Zhong and Xu2019).
In this context, the CNs are categorised as generic and specific needs. Generic needs are basic expectations of performance in a product. The generic product features answering to these needs are contained in the base architecture of the seed design (Figure 2). Specific needs refer to affective, cognitive or user experience related needs, which are the subjects of MP (Zhou, Ji, & Jiao Reference Zhou, Ji and Jiao2013). The specific needs are fulfilled by the personalisable product features, which may be functional, ergonomic or aesthetical ones. The variable elements of the seed design define these features according to specific needs. The focus of this methodology is on personalisable features, which cater to the individual needs and desires. Therefore, CNs in the following sections refer to personal, specific, needs.
The first step of the process is to identify the top-level specific CNs. These correspond to very broad categories, or descriptions, of possible personal needs. For instance, considering a case of eyewear frames, comfortable fit may be a top-level CN. Here, comfortable fit does not say what will change in the product. It is translated to PRs, and then these are decomposed to more specific requirements. Then these requirements determine what personalisation options will be offered to the user. How to identify these top-level CNs are not in the scope of this work.
3.2.2. Personal requirements
The CNs are in the customer language, and these needed to be expressed in technical descriptions to achieve quantitative relations in the design space. In the transition from customer domain to functional domain, the specific needs are mapped onto PRs. Cognitive task analysis, quality function deployment and association mapping present solutions to transfer these specific, affective-cognitive, needs to PRs in the MP context (Zhou, Ji, & Jiao Reference Zhou, Ji and Jiao2013).
A difference of PR definition in this context, in comparison to FR definitions, is that they do not describe what ‘has to be done’, but rather what ‘can be done’. The point of interest is exclusively specific needs, and PRs express a range of possible requirements. Since the personalised features of the product is defined after the general features, the product has already a base architecture. Therefore, the top-level PRs do not state the design objective of the whole product, and instead they state the personalisation objectives.
The product features corresponding to specific CNs may be categorised as ergonomic, functional and aesthetical (Figure 2). Since these features are defined through the PRs, the same categorisation may also be done for top-level PRs. An ergonomic requirement corresponds to a personal fitting need in this context. Moreover, while functional ones are related to the product performance, aesthetical requirements are to define the physical appearance of the product. While PRs in one of these categories is sufficient for a personalisation case, PRs in all three might be present as well, such as in the footwear example elaborated later in the paper. Whereas, in the saxophone mouthpiece example given later, there are only functional PRs. The reason of such categorisation is to cater these requirements accordingly, to structure better the design solution process, as illustrated later in Section 3.2. For instance, when present in a given MP scenario, the prior needs to meet are the ergonomic ones. Besides, the parameters corresponding to ergonomic requirements are likely to constrain the rest, as they define size or shape of the product. Such as, in a footwear personalisation case, if personal fit option is provided, it is inherently the primary requirement to be fulfilled. Therefore, the fit requirements should be at the top of the PR hierarchy.
Top-level PRs state design personalisation context derived from CNs. These PRs are decomposed to lower levels, based on the DPs, to define more specific design objectives to personalise the design. The customer input to the design in the co-creation process is done on the lower-level PRs. Therefore, the PRs should be decomposed, at least, until the level appropriate for customer input. These PRs getting the customer input define the design space, where each PR is a dimension with a range. The customer input is taken within these ranges, which define the extent of personalisation offering. Since the customer input cannot be in the language of PRs, the selected levels of PRs are mapped back to the customer domain to be expressed in the customer language again. This last process of defining how user input is taken is a part of the design of co-creation activity, which is not covered in this work.
To illustrate the decomposition process, a hypothetical eyeglass frame is taken. Some very broad CNs can be a comfortable fit of the frame and adaptation to face shape or skin tone. Comfortable fit need can be translated to a top-level PR as fitting to facial measures. Then the corresponding top-level DP would be the dimensions of the frame. This top-level PR can be further decomposed to lower levels to requirements of specific facial measures. These are then matched with relevant dimensional DPs of the frame. Here, the user input can be transmitted to the design through either the higher or lower level of PRs, depending on the co-creation scenario. For instance, in case there is a process of face scanning, then the user input is taken at the level of fitting to facial measures. Another case might be user self measuring or using the dimensions of a previous frame, and in this case, the user input would go through the lower-level PRs of specific facial measures.
3.2.3. Design parameters
The user input through PRs is realised by DPs in the physical domain. DPs define the variable design features of the seed design, and they are selected to fulfil the corresponding PRs. In the context of digital manufacturing, these DPs may provide variety through changing certain dimensions of the product, or through varying topologies, structures, material composition and so on. DPs should be decomposed to lower levels until PRs can be implemented into the design. A DP can have a range of quantities or a set of options. In the first option, the values the parameter can take would be a continuous range, while in the latter case, it would be a value for each option in the set.
The mapping between PRs and DPs can be expressed as a design equation, where r denotes the in-between dependency:

When identifying the DPs, the ideal scenario is that each PR is satisfied by one DP, which corresponds to an uncoupled design in AD. In this case, there is one-to-one mapping, and each PR is fulfilled by the corresponding DP. Another manageable form is a decoupled design, where the dependency matrix has a lower triangle form (Suh Reference Suh2001). In this case, PRs are satisfied in a hierarchy of least dependent to most dependent. A similar solution is also provided in DSM to provide an order for the definition variable values, by sequencing the rows and columns of the matrix to form an upper triangle (Eppinger & Browning Reference Eppinger and Browning2012). In the case of MP, the proposed method based on the principles of having sufficient design space for self-expression and implementing personal priorities in the design. Achieving an uncoupled design may require architectural changes in the product. Besides, a PR dependency hierarchy for the design solution in a decoupled design naturally narrows down the range of subsequent PRs. However, customers may have different priorities for the given PRs, and these priorities should set the solution hierarchy while achieving a personalised design. Therefore, a user-centric design solution algorithm is proposed in Section 3.2, along with a dependency matrix to visualise and simplify the design cases.
While setting DPs, it is still important to aim for less-coupled design to lower the complexity of the dependency matrix. The complexity in this context refers to the number of PR–DP dependencies in the matrix. A trade-off between complexity and the range of PRs satisfied is necessary to provide an adequate personalisation experience. Another downside of high complexity in PR–DP dependencies is the possibility of radical changes in the dynamic design space provided to the user during the co-creation process. This may lead to a negative user experience, as the initial state of the design space creates expectations, the valid state may lead to disappointment.
3.2.4. Constraints (C)
Cs are the limits or restrictions on the DPs (solution space) primarily, but indirectly restricting the PRs (design space) as well. The Cs may initially be divided into two groups based on the phases they are applied. The first group is used to set the boundaries of the initial design space. There are four categories of constraints to look for, which are explained below The second is the PR–DP dependency constraints that during the co-creation and design solution phase. The dependencies between PRs and DPs result in a temporarily valid design space in the co-creation process. These Cs are handled in the design solution algorithm.
The aim of constraining the design space is to ensure that the final design is reliable and manufacturable. This is a crucial prerequisite for automating personalised design generation. The initial and broadest ranges of DPs are set according to the product architecture. This implies the natural boundaries of the products, that exceeding these would be unnecessary or impractical. An example could be the DPs regarding the ergonomic fit of a product, which would be characteristically limited by anthropometries. The second step of Cs is the interdependencies of DPs. This step is to eliminate possibly counterproductive or unfeasible DP combinations.
In traditional product development, manufacturing considerations are in the process domain, after the design is defined (Ulrich & Eppinger Reference Ulrich and Eppinger2012). Whereas, in design for manufacturing, these considerations are present since the early design phases (Salonitis Reference Salonitis2016). Manufacturing flexibility is a main pillar of MP, and thus designing for MP largely relies on manufacturing and related variables. Therefore, a design for manufacturing approach is more suitable as manufacturing processes or materials are early phase design decisions, since they are related to the personalisation offering. Consequently, the proposed approach considers manufacturing-related variables while defining the solution space. The third step of Cs is related to the manufacturing and cost feasibility. At this step, restrictions regarding manufacturing methods, process and materials are applied to DPs, both to ensure manufacturability and to eliminate unpractical results. Furthermore, depending on the cost target, design solutions potentially resulting in extreme cost variation may be eliminated.
The final step to constraint DPs is regarding the personalisation offering. Depending on the CNs and co-creation scenario, the range of DPs may be restricted again, if at this step, the DP in the solution space exceeds the personalisation target. An example of this step would be restricting any DP that goes beyond the intended level of personalisation or create a very large design space, which may get confusing for the user and lead to a poor experience.
DPs along with the Cs, form the solution space where a personalised final design is achieved on the seed design. Therefore, DPs set the dimensions of the solution space, while Cs define the size of it. After all Cs applied, the final ranges of DPs are mapped back onto PRs to form the initial design space.
3.2.5. Design space
After the Cs are applied to the solution space, the consequent ranges of DPs define the possible ranges of PRs, and as a result the design space for the user. The dependencies between PRs and DPs are shown in Figure 4. In the dependency matrix, $ {r}_{mn} $ denotes for how
$ {DP}_n $ fulfils the
$ {PR}_m $. Therefore, PR is a function of DPs:


Figure 4. Dependency matrix of personal requirements (PRs) and design parameters (DPs). r denotes for dependency.
In case it is possible, at least in a certain range, to linearise the relationship between PRs and DPs, $ {PR}_m $ can be expressed as:

The extrema of the equation give the maximum range of $ {PR}_m $. The maximum range of a PR is established when all dependent DPs fulfil the PR. In the case where a DP affects multiple PRs, this creates a dependency between PRs. These dependencies would restrict the ranges of PRs. While defining the design space at any instance, these dependencies are ignored to provide the most design freedom to the user at the beginning. Since the range of each PR is set independently, they cannot have the largest ranges simultaneously, as some DPs are affecting multiple PRs. Following each user input during co-creation, the solution algorithm considers the dependencies and recalculates these ranges, and then returns to the user interface. Hence, the algorithm shows the largest range to the user at any instance, according to the DP values at that instance, but this is only valid for the following choice of the user. Hence, the PR to be fulfilled first would still have its largest range. Therefore, the user has to decide on personal priorities and reach a desired trade-off. The benefit here is that the user has the most freedom at any instance of decision and can get the most out of the desired performance aspect. How this process works is explained in the next section.
Consequently, the initial design space for customer co-creation is set by defining the maximum ranges of PRs regardless of the dependencies. With the formulation of a design space, the first design phase, seed design development, is completed.
3.3. Personalised design solution
Once a seed design is developed in the first phase, it is necessary to understand how the co-creation tool, hence the user, interacts with the seed design to develop a personalised final design. In this second phase, reaching a personalised design solution is explained.
The initial design space is formed by mapping back the solution space of DPs and Cs onto PRs. When there is user input to the system, the dependencies between PRs and DPs should also be considered. Each user input changes the valid ranges of PRs, and thus results in a dynamic design space.
The design solution procedure is shown at Phase 2 in Figure 3. The user input in co-creation is transmitted via PRs to the solution space. When a decision is made on a PR in the design space, it is fulfilled by the corresponding DP in the solution space. Afterwards, with the new state of the solution space, the design space is updated with the valid ranges of PRs at that instance. The user is then informed with the further design possibilities and then makes an input for the next PR. The process continues until all PRs are decided, and all DPs are set. In the case where the user prefers to exceed the valid ranges of any PR, still within the initial design space, the solution space is reset, and the design space is updated to the new state. This way, the user may get certain trade-offs between design decisions. Once the co-creation process is complete, all the DPs in the seed design are set, and a final personalised design is generated.
3.3.1. Clustering
DSM is a common tool to model, visualise and analyse the dependencies between the elements of complex systems (Eppinger & Browning Reference Eppinger and Browning2012). It also offers suggestions to simplify the design cases by sequencing the rows and columns to reach a systematic order for assigning a value to each variable. One of the suggestions is clustering, where matrix elements are clustered to identify minimally interacting subsets, hence eliminating or minimising the interdependencies. In a similar way, to reduce the complexity of the dependency matrix, certain PRs and DPs may be clustered, and considered as a cluster in the iteration for design solution. The clustering is useful in the following cases:
(i) DPs of an independent PR.
(ii) In case the dependency is on a higher level of PR, clustering the lower-level PRs.
(iii) DPs for ergonomic fit.
When a PR is independent, this implies that the corresponding DPs does not affect any other PR. Therefore, these DPs can be clustered and considered as a single element to fulfil the PR. If there is a dependency on a higher level of PR, the lower-level PRs in its subset can be clustered and iterated as a cluster. The clustering can be only up to the level where there is user input.
The last case is when the product is made-to-measure. DPs corresponding to requirements of fit would be geometric dimensions. Since the requirements would be dimensions as well in this case, these PRs and DPs would have one-on-one mapping. Therefore, ergonomic PRs, and corresponding fitting DPs, can be considered as a subsystem and clustered to simplify the case. Since these DPs change the size or shape of the product, they may still affect the valid range of other PRs. In such case, for the sake of simplicity, this dependency should be considered as between the fit cluster and the relevant PRs.
To illustrate the use of clustering, a dependency matrix of an arbitrary design case is shown in Figure 5. In the example, there are three top-level PRs and in the lower level there are nine PRs. $ {PR}_1 $,
$ {PR}_2 $ and
$ {PR}_3 $ are ergonomic fit requirements, and each of them are dependent on one DP. Therefore, these can be clustered to simplify the matrix.
$ {PR}_4 $–
$ {DP}_4 $ and
$ {PR}_5 $–
$ {DP}_3 $ have one-on-one mapping and independent of the rest. Therefore, these can be clustered as well. The third case of higher-level dependency is not present in this example. For instance, if
$ {DP}_3 $ and
$ {DP}_4 $ were both dependent on one PR, this would imply a higher-level dependency. In the simplified matrix (Figure 5), cluster two can be handled independently for the design solution. Cluster one is still dependent to PR6–PR7, but since it is an ergonomic fit cluster, it is fulfilled first as explained further in the following section. As a result, only the remaining four PRs and four DPs require an iterative solution.

Figure 5. Example clustering case on a personal requirement (PR)– design parameter (DP) dependency matrix. The matrix on the left side is the initial state, and the matrix on the right is the simplified one after clustering. Top-level PRs are marked with a superscript.
3.3.2. Solution algorithm
To manage the interaction between design and solution spaces, a solution algorithm for design personalisation is introduced in this section. The solution algorithm manages the dependencies between PRs and DPs, keeping control over the complexity, still providing an almost continuous variety. In the backend, it allows the real-time interaction of the user with the design. The algorithm dynamically controls the boundaries of these spaces and assigns values to DPs to fulfil PRs with an iterative approach. The objective is to offer the largest design space at each decision step and provide the most design freedom to the user on the preferred design aspects. The algorithm (Figure 6) works by iterating the DPs to fulfil the PR at each step. The fundamental principles of the algorithm are:
(i) The hierarchy of the PRs are decided by the user.
(ii) Least possible number of DPs used to satisfy an PR.
(iii) If present, independent DPs are changed first to fulfil the given PR.
(iv) Iteration from the most effective to the least effective DP for the given PR.
(v) If present, PRs of ergonomic fit are fulfilled first.
(vi) Solely aesthetical PRs are considered last.

Figure 6. Design solution algorithm.
When each DP affects only one PR, the complexity of the dependence matrix becomes the lowest, thus no iterations needed, and each PR can be satisfied with the corresponding DP. For the rest of the cases, where dependencies increase the complexity, the solution algorithm is applied. The steps below are followed for the personalised design solution:
(i) In case where the ergonomic fit is a PR, it is fulfilled first. This is because of the assumption that the primary expectation from a made-to-measure product is personalised fit. Besides, it would have a fixed input for each user, hence it is not a CN that the user could actively state preference. Therefore, PR related to the fit is fulfilled first and in case of a dependence, design space is updated, before further decisions are made in the co-creation process. Following this, functional PRs are fulfilled where design case is more likely to be coupled. Lastly, the aesthetical PRs are fulfilled, after the right fit and suitable set of functions are provided.
(ii) The process starts with the user input, and a value is assigned to the corresponding PR.
(iii) Then, the value is checked if in the valid range. If not, the solution space is reset.
(iv) In the following step, the most effective DP for the given PR is identified and checked if it has already been assigned a value.
(a) If yes, it goes back to the second most effective DP and repeat.
(b) If no, the DP is incrementally increased or decreased until either the boundary value is reached or the PR is satisfied.
(v) After this iteration, the PR is checked if satisfied.
(a) If not, it goes back to the DP selection process and continues with the next DP.
(b) If it is satisfied, the design space is updated for the valid ranges of remaining PRs.
(vi) The same process continues until all PRs are fulfilled.
(vii) Then, when all DPs are set, a personalised final design is generated.
All DPs initially start at a median value. According to the input PR value, they are iterated towards either the upper or the lower boundaries of their ranges. Since the design space is in a feedback loop, the input to the PR would be in the valid range, and the iteration of DPs will surely satisfy the PR. In case of an input outside the valid range, the solution space goes back to the initial state, and again the fulfilment of the PR is ensured. The operation of dynamically controlling the dependencies and valid ranges also guarantees the reliability of the output final design. Hence, this process automates acquiring CNs and reflecting it to the design; and it may also provide personalised designs that are ready for manufacturing.
4. Illustrative applications
The proposed methodology focusses on the MP of consumer products that are realisable by digital manufacturing. This section presents two illustrative applications that demonstrate how the methodology can be applied to representative cases. In both cases, there is an existing demand for personalisation, and both are based on existing R&D topics that led to commercial solutions. Two very diverse products are chosen to demonstrate the range of applicability of the methodology. The first application is on 3D-printed saxophone mouthpieces, and there is a coupled design case while tailoring the performance of the mouthpiece. The second application on knitted footwear explores the personalisation of fit and appearance of the product, besides the functionality. In addition to 3D printing, there is also digital knitting in this case to illustrate the usage of another digital manufacturing technology. Each application is finalised with an example co-creation scenario; while in the mouthpiece application the front-end of the design and solution spaces’ interaction is demonstrated (Figure 11), in the knitted footwear application the back-end process of the algorithm during this interaction is presented (Figure 16).

Figure 7. Saxophone mouthpieces.

Figure 8. Personal requirement (PR) and design parameter (DP) decomposition for mouthpiece personalisation. The PRs getting user input are in rectangles with dashed lines.

Figure 9. Saxophone mouthpiece design parameters.

Figure 10. Dependency matrix between mouthpiece personal requirements (PRs) and design parameters (DPs). Independent DPs are shown with coloured background. X stand for dependency.

Figure 11. Interaction between design and solution spaces in a user co-creation scenario for mouthpiece personalisation. The ranges of design parameters (DPs) are shown on the radar charts on the left side; their values vary from inner to outer heptagon, increasing towards the outer.

Figure 12. Example knitted footwear with 3D printed sole (size EU37B).

Figure 13. Personal requirement (PR) and design parameter (DP) decomposition for knitted footwear personalisation. The PRs getting user input are in rectangles with dashed lines.

Figure 14. Dependency matrix between footwear personal requirements (PRs) and design parameters (DPs). Clusters are shown with coloured background. X stand for dependency.

Figure 15. Normalised effects of design parameters (DPs) on personal requirements (PRs).

Figure 16. Design solution example for knitted footwear personalisation.
4.1. Saxophone mouthpiece personalisation
The saxophone mouthpiece is a product attached to the upper end of the saxophone, and it is the interface between the player and the instrument (Figure 7a). Saxophone sound is produced in the mouthpiece, by the vibration of the reed attached to it. Hence, the design of the mouthpiece greatly affects the performance of the instrument. Therefore, saxophone players often try different mouthpieces to find the most suitable one for themselves. There are a range of different designs available in the market. The choice of a mouthpiece is a very personal decision, depending on playing habits, the music genre or the playing environment. There are also a number of artisans modifying existing mouthpieces to help players to reach closer to the desired performance. Thus, there is an existing demand for the personalisation of saxophone mouthpiece.
The majority of the mouthpieces in the market are produced with subtractive processes and finished by manual labour. Therefore, additive manufacturing presents an opportunity both in terms of ease of production and personalised designs. In this application, the personalised mouthpieces (Figure 7b) are 3D printed by Stereolithography technology, due to the high accuracy, resolution, precision and suitable materials that it provides (Ozdemir & Cascini Reference Ozdemir and Cascini2020).
This example application is a summary of a previous case study of the proposed methodology (Ozdemir & Cascini Reference Ozdemir and Cascini2020; Ozdemir et al. Reference Ozdemir, Chatziioannou, Verlinden, Cascini and Pàmies-Vilà2021). The starting point in this case is an existing mouthpiece, from which the features of the base architecture are derived. For instance, the overall dimensions of the mouthpiece, how it fits the saxophone, and how the reed is positioned are some elements of the base architecture. There are also some variable design features of the seed design that provides the personalisation. These are explained in the mouthpiece DPs section below.
4.1.1. Personal requirements for mouthpiece
The personal needs of a player with a mouthpiece can be broadly grouped as desired sound and compatibility with playing habits. Top-level PRs for these can be expressed as sound features and playability aspects, respectively (Figure 8). The sound features are usually very subjective and the perception of these are studied in psychoacoustics (Carron et al. Reference Carron, Rotureau, Dubois, Misdariis and Susini2017). However, the tone colour of the sound and the loudness of it can be related to quantifiable measures (Ozdemir et al. Reference Ozdemir, Chatziioannou, Verlinden, Cascini and Pàmies-Vilà2021). The spectral centroid of the sound gives an indication about the ‘brightness’ of the sound. Moreover, the loudness of the instrument can be measured by the sound pressure level. Therefore, two of the PRs related to sound features are the spectral centroid and the sound pressure level. In terms of playability, two important and measurable aspects are resistance and pitch flexibility. Resistance implies ease of blowing the mouthpiece while playing. It can be measured by the minimum blowing pressure required to oscillate the reed (oscillation threshold), which produces the sound. Hence, the oscillation threshold indicates the resistance of the mouthpiece. The pitch flexibility is the range of possible pitch modification on the mouthpiece, and it can be measured by the interval of the highest and lowest pitch produced.
4.1.2. Mouthpiece DP and dependency matrix
The sound is produced by the vibration of the reed, that is excited by the air passing through the inner cavity of the mouthpiece. The parameters defining the inner cavity affect the airflow characteristics, and the parameters interfacing the reed affect the vibration of the reed (Figure 8). Hence, these parameters, as shown in Figure 9, define the performance of the mouthpiece. These parameters vary among the existing mouthpieces, and it is known that they affect certain characteristics. However, there was no evidence on how and what they affect exactly. Since the proposed methodology requires quantitative relations between PRs and DPs, these were identified through a set of experiments in the previous study (Ozdemir et al. Reference Ozdemir, Chatziioannou, Verlinden, Cascini and Pàmies-Vilà2021).
Figure 10 shows how each parameter affects PRs. These relations between DPs and PRs were identified and quantified through experimentation with an artificial blowing machine (Ozdemir et al. Reference Ozdemir, Chatziioannou, Verlinden, Cascini and Pàmies-Vilà2021). As seen in Figure 10, there is a highly coupled case, where all PRs are defined by multiple DPs, and five of the DPs affect multiple PRs. Therefore, it is a complicated task to tailor the performance of the mouthpiece, and as the performance aspects are dependent through the DPs, certain trade-offs may be needed.
4.1.3. Constraints for mouthpiece and solution space
To set the boundaries of the solution space, a list of previously suggested constraints are to be considered. In the case of the mouthpiece, there are a considerable number of designs available on the market. Hence, a survey of these existing designs are used to set the initial boundaries of the solution space (Ozdemir et al. Reference Ozdemir, Chatziioannou, Verlinden, Cascini and Pàmies-Vilà2021). For instance among the existing designs, the reed interface parameters, lay length and tip opening, vary within 17–25 and 1.4–2.6 mm, respectively. This is used as a starting point to set the range of these parameters. It is also important to note that the existing designs have discrete values within these intervals, and most of the possible combinations of different parameter values do not exist. Hence, there is a large unexplored space of design possibilities, and consequently, unexplored performance characteristics. The proposed approach allows fine-tuning these parameters, and exploring the complete solution space to tailor the performance of the mouthpiece.
To constrain the design space further, interdependencies of the DPs are checked. Continuing on the same two parameters, lay length and tip opening are dependent parameters, and extreme combinations of these are either unplayable or very uncomfortable to play. Therefore, these combinations are also eliminated.
In terms of manufacturing or feasibility, there are not any significant constraints in this case. Since the product has a simple geometry, and the parameter changes are at a small scale. Nevertheless, there are still such constraints regarding the basic design of the mouthpiece. For instance, the selected material should not be toxic as the product stays in contact with the player for long time. Similarly, it should be resistant to moisture as it is significantly present while playing. There are surely more constraints to these to be considered for the basic design of the product. While the constraints here, and in the context of the methodology, are related to the possible changes in the product.
The final step of the constraints are related to the personalisation scenario. In this case, the users of the product are rather experts, and hence they might benefit and appreciate the largest possibilities. For instance, if the user target was narrower, such as only beginner players or classical music players, the parameters could have been restricted further to avoid possibly undesired results. Once all constraints are applied, the final ranges of the DPs are obtained. These ranges form the solution space, within which a personalised design can be created.
4.1.4. Design space for mouthpiece
The solution space set the boundaries of how the structure of the product may change. On the other hand, the design space includes possible PR for the product. In this case, these are the four performance aspects. The solution space is mapped back onto these to set their ranges. To be more specific, the DP combinations providing the extrema of the given performance aspect give the largest range of the PR. For instance, the highest pitch flexibility is obtained with the largest baffle height and the smallest chamber size and tip opening. With the given DP ranges, the pitch variation interval (flexibility) can get a value between 41 and 129 Hz, according to the results of the previous study (Ozdemir et al. Reference Ozdemir, Chatziioannou, Verlinden, Cascini and Pàmies-Vilà2021). The same procedure is then followed for all the PRs to find what can be offered to the user, and this forms the design space. The design space initially provides the largest ranges of PRs. But since this is a coupled design case, PRs are dependent and the user can not simultaneously decide on all four PRs. Therefore, the user has to reach a trade-off considering personal priorities and can obtain the most suitable performance from the mouthpiece.
4.1.5. Personalised design solution for mouthpiece
This example briefly illustrates how the user co-creates the product through the interaction between the design and solution spaces via the solution algorithm behind. Since the PRs are very technical and their values would not be meaningful to the users, they are renamed in the user language and sliders are provided for user input. In Figure 11, pitch flexibility refers to pitch flexibility interval; brightness refers to the spectral centroid; resistance refers to the oscillation threshold and loudness refers to sound pressure level. In the user study of this example, benchmarking mouthpieces were given to the users to understand the scale and have a reference for their decisions (Ozdemir et al. Reference Ozdemir, Chatziioannou, Verlinden, Cascini and Pàmies-Vilà2021).
A user co-creation scenario is shown in Figure 11. Step 1 shows the initial design space, where all four PRs have their largest ranges available. The first decision of the user is to set the pitch flexibility, moving the slider to the more flexible side. As the most effective DP in this case is tip opening, it is iterated to its lower limit; then passing to the second DP, the baffle height is increased, and a solution is found. In Step 2, it is seen that the design space and the default state of the PRs are changed. This is due to the DPs changed in the previous step. In this step, the user changes the brightness, and this requirement is satisfied by iterating the throat shape and the lay length, respectively. In the third step, four of the DPs are already set, and the remaining design space for the other two PRs are significantly smaller. In this case, the user changes the resistance, and this is satisfied by increasing the chamber shape. It should be noted that since the chamber shape is an independent DP, it does not affect the design space in the next step. In the last step, the user sets the loudness of the mouthpiece, and this is satisfied by iterating the throat shape first, as it is an independent DP. At this point, all PRs are decided by the user, and a design can be generated with the DP values at this instance. In the case desired, the user may continue looking for further trade-offs following the same process.
4.2. Knitted footwear personalisation
Personalisation in footwear is nothing new; it has been practised by shoemakers producing bespoke shoes. Inherently, the cost of an artisanal product is higher than a mass-produced counterpart. However, customer willingness to pay for a personalised shoe is only 10–30% more than a mass-produced one (Head Reference Head2012). A major reason of interest in personalisation is the customers’ need of proper fit and comfort. Standardised footwear with mass production results in a poor fit, which is found to cause several foot-related problems (Goonetilleke, Luximon, & Tsui Reference Goonetilleke, Luximon and Tsui2000). Another motivation personalisation is that footwear is also a fashion product and provides an opportunity of self-expression to customers. Therefore, the uniqueness and mass efficiency offered by MP is very suitable for footwear.
The proposed method is applied to a personalised footwear design case with a knitted upper and a 3D printed sole in this section (Figure 12). The advantages and application of digital knitting and 3D printing for footwear MP were explained in the previous study (Ozdemir, Cascini, & Verlinden Reference Ozdemir, Cascini and Verlinden2020). The reason for choosing these two technologies is that they both provide design personalisation opportunities, the flexibility needed for design variation and mass efficiency. The given example considers the development of only the shoe upper and the sole. In this case, the starting point is a concept design. The base architecture contains the style of the shoe through a defined shoe last. For instance, when the style in the base architecture is trainer shoes, the seed design can not vary to trekking shoes. Similarly, certain form elements, such as the form of the sole is also contained in the base architecture. The variable features of seed design here the sizing of the sole and upper for personalised fit; and functional and aesthetical features of the upper. These variable features of the seed design are explained in the development steps below.
4.2.1. Personal requirements for knitted footwear
In the first step of the design case, identification of top-level PRs requires broad descriptions of the specific CNs. For personalised footwear, these are identified as comfort and self-expression. The top-level PRs corresponding to these are personalised fit and shoe upper properties for comfort, and upper appearance for self-expression. These three top-level PRs correspond to ergonomic, functional and aesthetic features to be personalised in the shoe. To also exemplify the generic features, the shoe style might be given. The style, trainer shoes in this example, is predefined on the shoe last design, which may be considered as the mould in the footwear context.
Figure 13 shows the decomposition of PRs and the corresponding DPs. Flexibility, weight, permeability, and heat resistance are the lower-level PRs that define the functional features of the shoe upper. Graphical pattern and knit pattern are the lower-level PRs defining the visual appearance of the upper. The PRs framed in Figure 13 are the selected as convenient levels to get the user input. These are mapped back to the customer domain, to be expressed as design personalisation options in the co-creation process. Personalised fit and graphical pattern are decomposed one level further to be satisfied by the selected DPs. These lowest level PRs are not chosen as user input since they individually do not present any personalisation need.
4.2.2. Footwear DPs and dependency matrix
The identified DPs are shown in Figure 13. Yarn material, count (thickness) and knit pattern selections fulfil the comfort properties of the shoe upper. Yarn colour and knit pattern fulfil the upper appearance needs. Knit pattern is used both as a PR and a DP. Because it has both an aesthetic value and a structural function. In this case, it is assumed that there is a supply of yarns providing stable behaviour, and the knitting machine is able to produce some patters that behave substantially the same.
The shoe last design can be modified for personalised fit via sizing and grading parameters (Zhang et al. Reference Zhang, Luximon, Pattanayak and Zhang2012). These parameters define the geometry of the sole and the upper. The sizing is defined by the length, and the grading is by the girth measures of the user’s feet. The length and girth values are obtained from the foot measurements of the user.
The matrix in Figure 14 shows the dependencies between PRs and DPs. The length and girth requirements are clustered together since they are related to fit. The dependency between the graphical pattern cluster and the last parameters is related to dimensions. The last parameters define the size of the upper where a graphical pattern will be applied. This size information is a prerequisite for a graphical pattern input. Similarly, the sizing parameter should have a value before the grading parameter is set. Therefore, these two parameters should be clustered. The last parameters are defined by personalised fit requirements, which must be fulfilled first according to the method. Therefore, once the fit cluster is fulfilled, the graphical pattern cluster becomes independent. As a result, both clusters and the corresponding DPs are independent, and they can be fulfilled regardless of the other PRs. However, the remaining five PRs are coupled through three DPs, and this requires an iterative solution.
4.2.3. Constraints for knitted footwear and solution space
To define the solution space, the DP ranges go through the constraining steps. Initially, all DPs start at the largest intervals possible for the product architecture of the given case. For instance, the sizing and grading parameter ranges are bounded with the anthropometric foot data (Luximon & Luximon Reference Luximon and Luximon2013).
The second step is checking the interdependencies of DPs. A simple example of this is the limited availability of yarn colour and count options for a selected yarn material. In the third step, process and cost constraints are applied. Since a knitted upper is in the design proposal, the knitting parameters depend on the digital knitting machine. For instance, the range of yarn counts that can be used is limited with the machine gauge, and also the number of colours that can be used at the same time is limited with the number of carriers in the machine (Ozdemir, Cascini, & Verlinden Reference Ozdemir, Cascini and Verlinden2020).
The final step of the constraints is to limit the DPs which will define PR ranges in the initial design space. This step is according to CNs and the co-creation scenario. For instance, the number of knit patterns offered is limited to avoid over-complication for users. The boundaries of shoe sizes offered can be considered at this step as well.
Once all constraints are applied, the final ranges of DPs are obtained. These ranges, shown in Table 1, define the solution space. As stated before, yarn colour, last size and grading are independent parameters and get direct input from the customer domain. On the other hand, the options within the range of yarn material, count and knit pattern have different effects on the PRs. These effects are shown in Figure 15. To bring the effects to a common denominator, they are expressed as a value from 0 to 1. These ranges are mapped back onto PRs to define the initial design space in the next step.
Table 1. The ranges of design parameters (DPs) for the solution space.

4.2.4. Design space of knitted footwear
The combinations of the DPs seen in Figure 15 provide the max and min possible values of PRs that form the initial design space. For instance, the highest heat resistance can be obtained by wool yarn with Nm7–Nm2/14 having a single knit structure. The sum of the coefficients of these is 3. Likewise, the lowest heat resistance can be achieved by any material except wool, Nm6–Nm2/12, and half-cardigan rib or single-pique structure. The sum of coefficients, in this case, would be 1. Therefore, we can conclude that for heat resistance, the range is 1–3, and an input within this interval can be taken. The same is applied for the other PRs as well and shown in Table 2. These ranges shown in the table define the initial design space. It is important to highlight here that the interdependencies of PRs are not considered for these ranges. For instance, it is not possible to obtain both the highest flexibility and the highest heat resistance at the same time, due to the varying effects of DPs on each PR. But the first PR to fulfil, chosen by the user, will have the largest range.
Table 2. Final ranges of personal requirements (PRs) forming the initial design space.
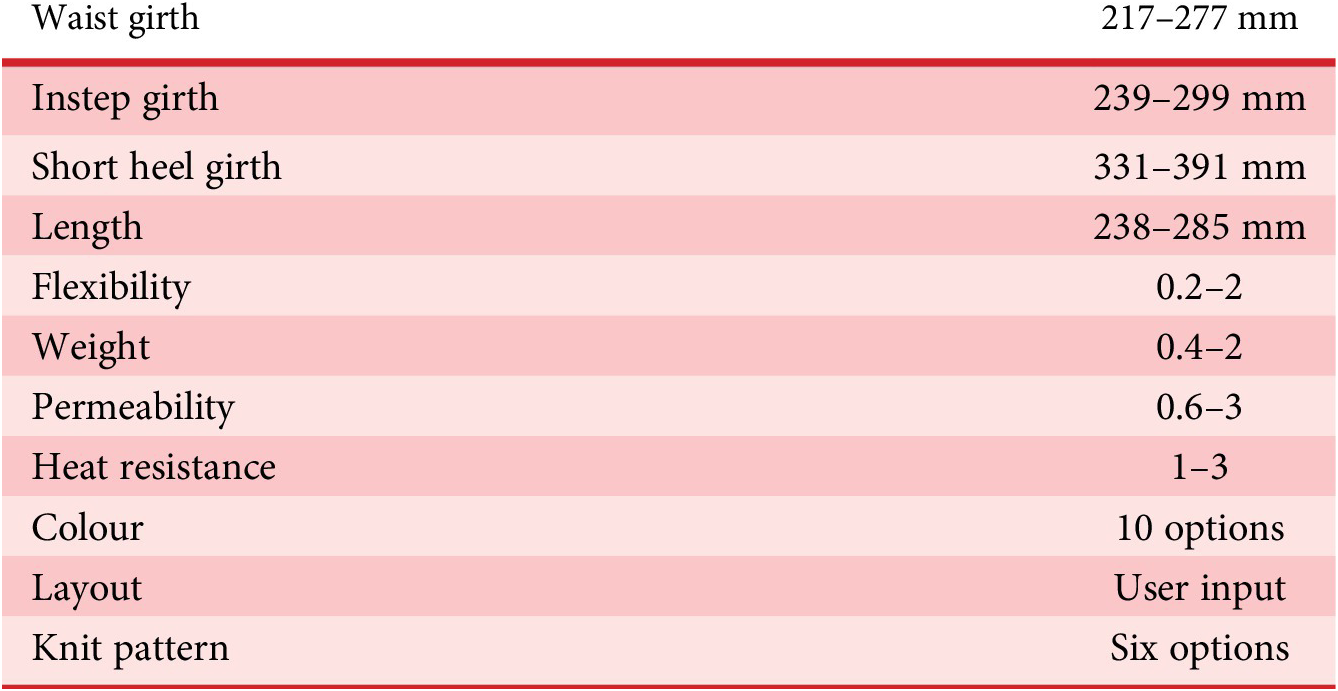
4.2.5. Personalised design solution for knitted footwear
An example design solution process is shown in Figure 16. The process starts with the user’s foot measurement input. First, the available shoe sizes are iterated for the given foot length. Afterward, the grading is iterated to match the user input with the obtained size. At the end of this process, 41.5C is found as the best fit, and the design space is updated with this information. As a second input, permeability = 1 is given. DP selection function chooses the yarn count parameter to be iterated first. At the end of the iteration, Nm7 is set for yarn count and this fulfils the permeability requirement. Updating the valid design space again, now it can be seen that the weight and heat resistance have narrower ranges, since the yarn count is set. The next input is heat resistance = 2.2. The DP selection function picks the yarn material and the iteration function picks wool as the most heat-resistant material. However, at this point, the heat resistance = 2, and the PR is not fulfilled. Therefore, the DP selection function runs again and picks the knit pattern this time and the iteration results in half-cardigan rib and single-pique as equal options to reach 2.2. At this point, all DPs are set, except the two options left for the knit pattern. Therefore, flexibility and weight have only one possible value in the updated valid design space. The final input is for the knit pattern, and single-pique is chosen. In case the user is not satisfied with the final output, the input for any PR can be set again to reach other trade-offs. On this occasion, the DPs chosen are reset, and the iteration process starts again. The final personalised design in the example is a 41.5C size shoe with Nm7 wool yarn having a single-pique structure. The graphical pattern is not included in the iteration, as it is independent and can be fulfilled directly at any step after sizing and grading.
5. Discussion
The two diverse illustrative applications presented the variability of applicable cases and the flexibility of the approach. The knitted footwear is a valuable example in the sense to show that the methodology is not limited to additive manufacturing, but it can be applied to digital knitting and other similar technologies. While the mouthpiece application focussed on the personalisation of the performance of the product, the knitted footwear also demonstrated the addition of personal fit and aesthetics.
The application of knitted footwear personalisation illustrated how to develop a seed design following the tasks in the proposed pipeline for an MP scenario under the suggested framework. Comfort and self-expression needs correspond to all three feature categories of the seed design, which is suitable to show the complete potential of the methodology. Demonstrating the functional personalisation of a coupled design case of the knitted upper contributes to the argument. However, although it does allow the user to prioritise certain performance, it is limited by the number of DPs and their options. Besides, it comes with a stable behaviour assumption of yarns and knitting patterns. Addition of new parameters or extending their ranges would strengthen the case. Besides, the functional personalisation might be more significant in high-performance applications such as shoes for athletes.
An important note on the knitted footwear example is that its seed design with two components or discrete design options of knitting should not be confused with a modular configuration case. Here, the variety is not created by changing components, but the design change provides the variety. The yarn parameters and knit pattern are also not components; they are the building blocks of a single component that provide multiple functions. Since in this case these parameters have discrete values, all possible combinations could be considered as variants of a module. Then we would end up with one module affecting four requirements, since decoupling is not possible. This becomes an even more complex problem, and still without a solution with a standard configuration structure.
The saxophone mouthpiece application illustrated a stronger functional personalisation case with a very coupled design. It is a single-component product that delivers variety on multiple performance aspects. Hence, it is also a stronger case to illustrate the benefit of the methodology. The mouthpiece is characterised by a coupled design and as long as its architecture is not changed, modularisation is not an option. In this case, the working principle of the mouthpiece makes the necessary architectural change to decouple the design virtually impossible. More in general, highly coupled designs require deep architectural changes to ensure modularity and consequent configurability. On the other hand, what is proposed here is to characterise the behaviour of the system even in the case of a coupled design to assign the most suitable value to each DP to fulfil PRs. In addition, this is a case where the user has expertise on the product. With the given design freedom, an expert user may even go beyond the foreseen scenario, and obtain a design that satisfies a very latent need.
In this work, the seed design definition flexibly adapts from cases very close to configuration to cases that allow flexible and continuous variation of all possible requirements. Hence, the seed design can be very simple, or very complex based on case or degree of investment. The key point is that the aim is to make a connection between the seed design and what the customer might want, facilitate the customer to finalise the design and obtain all the parameters for the digital fabrication of the product.
While constructing a seed design, the relationships between PRs and DPs may not be immediately evident in every case. These relationships can be identified depending on how much one can invest in the seed design development. While it is possible to linearise the relationships up to an extent to simplify the case, it is also possible to obtain a very precise map of how the product behaves with varying parameters through simulation or experimentation. The methodology makes a general claim on this, and linearisation is not mandatory as far as one can invest in characterising the design space in further details. For instance, in the application of mouthpiece personalisation, an experimental characterisation of the mouthpiece behaviour as a function of the different DPs was done, and in this case the relationships were not linear. While the experimentation might be limited by the sample size; if it was possible, an accurate simulation might have provided a more detailed characterisation. However, it is still important to note that the proposed algorithm assumes that it is possible with a reasonable effort to find the relationship between PRs and DPs. But it is clear that if the system is highly unstable, then it would be too complicated or too costly to build a detailed map for personalisation.
Another important consideration here is also on the proposed co-creation scenario. that the seed design is defined in a way that it is an unfinished product with open parameters within certain limits. With the co-creation process, the user finalises the product, rather than configurating it. More design freedom is provided to the user by allowing trade-offs between different requirements. The user is in the condition to make their own choices and priorities. In that perspective, the needs may, at least partially, remain latent. A user may even go beyond and satisfy a need that was not even intended to offer. Instead of a standard configuration structure, there is an iteration process to personalise the product. This results in a dynamic interaction with the user. The methodology includes a specific form of co-creation, where the user, in a way, negotiates with the algorithm behind to reach a desired trade-off. This both allows more design freedom to the user, and also streamlines the personalised design generation in coupled cases. But, it is important to inform the user well about how the system operates. A lengthy co-creation process may discourage users (Ozdemir, Cascini, & Verlinden Reference Ozdemir, Cascini and Verlinden2020). Therefore, trying to find a balance between the adequate design freedom of users and the complexity of the requirement-parameter dependencies is necessary. In addition, In this process, the user does not have direct control over the parameters but does have control over the performance of the product. Therefore, the personalisation process is driven by the requirements, and then parameters fulfil these.
The main advantage or improvement of MP over MC is expanding the design space, and consequently providing freedom to users for self-expression. Hence, the application of MP is suitable for products for which personal needs are present. While these personal needs might be already evident as in the saxophone mouthpiece example, they can be explored to create added value as in the knitted footwear example. The proposed methodology does not deal with the identification of the CNs, and it only decomposes the pre-identified top-level CNs. Therefore, the assessment of whether there is a customer need or desire for personalisation, or an opportunity of adding value to the product should be done beforehand. For the saxophone mouthpiece example, the categories of needs are rather evident with a more expert user profile. Hence, this is a very suitable case where users can benefit the design freedom to express their specific needs. Another limitation on the applicability of MP is that the mass efficiency is not achievable for all products to align the value creation with customer willingness-to-pay. In this sense, the design variability that AM enables is very suitable for saxophone mouthpiece, which has a simple construction, but high value. In the knitted footwear example, it may be arguable whether there is a need or desire towards the possible changes provided in the knitted fabric structure, as this case starts with an assumption of top-level CNs. However, the eventual aim is to demonstrate is how to exploit the flexibility of such digital manufacturing technology for design personalisation. The structural changes of the knitted fabric may allow many design opportunities in different contexts. In the footwear context, having a more generic user profile may limit the control given over the design. However, in a context with more experienced users, more design freedom can be provided, and this may yield to designs and uses of the product which have not even been anticipated.
6. Conclusion
MP may provide unique products and experiences for customers in an affordable way. Now, it is more real and feasible than ever with advancements in manufacturing and industrial production. Further advancements in digital manufacturing technologies will enable more profound changes in the product architecture, thus more products will be the subject of MP and personalisation will get more affordable. MP is compatible with on-demand smart manufacturing envisioned in Industry 4.0, and expected to play a major role in the future of consumer products market. The manufacturing automation envisioned via smart factories must be supported by design automation to truly enable personalisation with mass efficiency. Designers should get acquainted with the MP process and the necessary perspectives for successful implementation in product development.
In the aid of designers, this work presented a design methodology for MP that focusses on digital manufacturing context. It explains specifically the development and execution of a personal design generation process through defining a seed design as the overarching architecture that ensures such flexibility and adaptability to user’s expectations. The proposed methodology guides the designer through the design specification and solution processes for seed design development. It offers a tailored approach to the complexity management of solution space for MP. Other novelties are in how the design and solution spaces are constructed and in obtaining a final design having a real-time interaction with the user. The design and solution spaces are interactive and change according to the user input. Instead of setting a hierarchy of requirements prior to the design process, the choice is given to the users. The more customer-centric approach to design personalisation process aims to improve customer co-creation experience and satisfaction with personalised products. An algorithm is also proposed to manage real-time interaction of users and automate personalised design generation. Hence, the algorithm is aimed to be complementary to the smart manufacturing paradigm.The methodology is applicable to the design of consumer products where personal needs are present, personalisation would have added value, and application to digital manufacturing is feasible.
The proposed methodology provides a foundation for DfMP from a product development perspective, where the designer is not only the product developer, but also in a facilitator role for customer design. MP is still an emerging paradigm, hence both advancements in the enabling technologies and changes in customer demands and expectations may require the design approach to be adapted accordingly. The use of the methodology will be more significant with the advancements like varying topologies, voxel-based multiple materials among the same component, or with embedded 3D-printed electronics. A limitation of the study is that the methodology relies on digital manufacturing solely. Whereas in practice, some products may be partially mass-produced with conventional methods. Another limitation worth mentioning is in the user’s real-time interaction with the design. The design space changes with each user decision, and in case these changes are too radical, the user experience might be affected undesirably. Future work should focus on designing the co-creation activity and projecting that onto the seed design development. Besides the value offered by the personalisation of the product, the co-creation experience also presents value for the customer. Customers’ satisfaction with the final product and engagement with the co-creation process is also related to the experience provided. The different contributors such as co-creation process, product personalisation interfaces, user experience and customer journey through personalising a product may be considered together with a product and service design approach to provide the best processes and results for customers.