Introduction
Climate change increases weather hazards, like droughts and heavy rains, affecting European agriculture (Olesen et al., Reference Olesen, Trnka, Kersebaum, Skjelvåg, Seguin, Peltonen-Sainio, Rossi, Kozyra and Micale2011; Beillouin et al., Reference Beillouin, Schauberger, Bastos, Ciais and Makowski2022). Furthermore, climate change is expected to bring novel weather event combinations or extremes which can further increase yield volatility (Webber et al., Reference Webber, Lischeid, Sommer, Finger, Nendel, Gaiser and Ewert2020; Zscheischler et al., Reference Zscheischler, Westra, van den Hurk, Seneviratne, Ward, Pitman, AghaKouchak, Bresch, Leonard, Wahl and Zhang2018). Studies, like Albers et al. (Reference Albers, Gornott and Hüttel2017) showed that in Germany, weather risks account for over 40% of wheat yield volatility. Hence, farmers’ awareness of climate change and the importance of climate risk management is increasing (Finger and El Benni, Reference Finger and El Benni2021; Madhuri & Sharma, Reference Madhuri and Sharma2020). Addressing this issue, multi-peril crop insurances are emerging as a key risk management strategy for farmers, covering yield losses from all climate hazards (Bardají et al., Reference Bardají, Garrido, Blanco, Felis, María Sumpsi, García-Azcárate, Enjolras and Capitanio2016).
Despite strides by insurance companies in offering agricultural insurance for European farmers, challenges persist (Bucheli et al., Reference Bucheli, Conrad, Wimmer, Dalhaus and Finger2023). Insurance uptake hinges on farmer participation and payment capacity (Liebe et al., Reference Liebe, Maart, Mußhoff and Stubbe2012; Liesivaara and Myyrä, Reference Liesivaara and Myyrä2014; Möllmann et al., Reference Möllmann, Michels and Musshoff2019; Pérez-Blanco et al., Reference Pérez-Blanco, Delacámara and Gómez2016). Equally pivotal is farmers’ perception of the insurance tool’s efficacy against weather-related risks (e.g. Hanson et al., Reference Hanson, Dismukes, Chambers, Greene and Kremen2004; Ginder et al., Reference Ginder, Spaulding, Tudor and Randy Winter2009; Kumar et al., Reference Kumar, Barah, Ranganathan, Venkatram, Gurunathan and Thirumoorthy2011; Peng et al., Reference Peng, Zhao, Elahi and Peng2021; Yazdanpanah et al., Reference Yazdanpanah, Zamani, Hochrainer-Stigler, Monfared and Yaghoubi2013). From the supply side asymmetric information and the contract design of insurance products determine the success (Mahul, Reference Mahul1999; Odening et al., Reference Odening, Mußhoff, Shynkarenko and Angelucci2008). However, Europe’s high insurance premiums still deter many farmers (Santeramo et al., Reference Santeramo2018) prompting discussions on governmental support. Consequently, the 2013 Common Agricultural Policy (CAP) reform proposed risk management funding (EU regulation 2017/2393) (European Commission, 2013) to subsidise insurance premium. This is already adopted by France, Italy, and Spain (Bardají et al., Reference Bardají, Garrido, Blanco, Felis, María Sumpsi, García-Azcárate, Enjolras and Capitanio2016; Bucheli et al., Reference Bucheli, Conrad, Wimmer, Dalhaus and Finger2023; Santeramo, Reference Santeramo2018).
The investigation of the crop insurance market in the European Union (EU) remains incomplete (Santeramo et al., Reference Santeramo, Goodwin, Adinolfi and Capitanio2016) despite some studies that have been conducted. Studies primarily link crop insurance uptake to farm characteristics and farmer demographics, such as farmers’ age, education, and farm size (e.g., Enjolras et al., Reference Enjolras, Capitanio and Adinolfi2012; Enjolras and Sentis, Reference Enjolras and Sentis2011; Finger and Lehmann, Reference Finger and Lehmann2012; Liesivaara and Myyrä, Reference Liesivaara and Myyrä2014; Liesivaara and Myyrä, Reference Liesivaara and Myyrä2017; Santeramo et al., Reference Santeramo, Goodwin, Adinolfi and Capitanio2016; Santeramo, Reference Santeramo2018). Doherty et al. (Reference Doherty, Mellett, Norton, McDermott, O’Hora and Ryan2021) found a preference among Irish farmers toward an index-based insurance scheme over a traditional indemnity-based multi-peril crop insurance. Gassler and Rehermann (Reference Gassler and Rehermann2022) delved into the inclinations of Lithuanian farmers toward a subsidized multi-peril crop insurance, unveiling varied preferences for contract specifics. These recent studies underscore the need to acknowledge farmers’ heterogeneity when discussing the adoption of (multi-peril) crop insurance and related policies.
Diverse decision-making structures emerge due to attitudinal heterogeneity among farmers (Pennings and Leuthold, Reference Pennings and Leuthold2000), a noteworthy factor in understanding farmer risk management (e.g., Aderajew et al., Reference Aderajew, Du, Pennings and Trujillo-Barrera2020; Barnes et al., Reference Barnes, Islam and Toma2013; Martin and McLeay, Reference Martin and McLeay1998; Pennings and Leuthold, Reference Pennings and Leuthold2000; Tudor et al., Reference Tudor, Spaulding and Winter2014). Literature also points to heterogenous farmer opinions on governmental support aspects, like price support or environmental service (e.g. Gorton et al., Reference Gorton, Douarin, Davidova and Latruffe2008). Recently, Feindt et al. (Reference Feindt, Grohmann, Häger and Krämer2021) discerned three German farmer groups with differing attitudes toward a more environmentally focused Common Agricultural Policy (CAP), while Bethge and Lakner (Reference Bethge and Lakner2023) employed cluster analysis to identify subgroups of farmers with differing attitudes toward the future direction of direct payments in the CAP. This suggests also potential attitudinal variations about policy-supported (multi-peril) crop insurance. Farmers’ attitudinal heterogeneity suggests that policy responses will not be uniform across all farmers, but rather segment-specific. This poses a challenge for policy makers introducing insurance premium subsidies, as predicting participation from various farmer groups becomes uncertain. Overlooking this diverse mindset could hinder the policy’s effectiveness, possibly leading to inaccurate predictions of participation rates from different sub-groups. Thus, grasping current farmer attitudinal heterogeneity is paramount for crafting effective insurance-supporting policies. Despite ongoing research, there is a clear gap in comprehending farmers’ varied attitudes toward a CAP-backed, multi-peril crop insurance subsidy in Europe.
Farmer typologies or types effectively capture the diverse characteristics and circumstances of farmers and farms. A farm or farmer typology means “a systematic or taxonomic concept, whose decision based essential on similarities between various individuals” (Kostrowicki, Reference Kostrowicki1977, p. 36). Farm typologies represent a promising pathway to approach the heterogeneity of farmers’ behavior in specific study context. Initially, farmer typologies focus on structural characteristics (economic performance, socioeconomic characteristics) (Kostrowicki, Reference Kostrowicki1977), a recent shift to behavioral factors in the literature is observed (Bartkowski et al., Reference Bartkowski, Schüßler and Müller2022).Footnote 1 Most recently, Huber et al. (Reference Huber, Bartkowski, Brown, El Benni, Feil, Grohmann, Joormann, Leonhardt, Mitter and Müller2024) summarized three categories of variables farm typologies are based on: Social-environmental, farm structural, and farmers’ individual characteristic. Farmers’ individual characteristics refer besides sociodemographic characteristics like age and gender also to behavioral characteristics (e.g., farmer perceptions). Furthermore, the authors also systematically summarized purposes of farmer typologies including enhancing farms system understanding (e.g., understand and explaining heterogeneity) as well as informing policy formulation and implementation (e.g., ex ante-policy assessment). However, a farmer typology regarding the implementation of policy subsidizing a multi-peril crop insurance in an ex ante context is lacking in literature.
Our study aims to bridge this gap by classifying farmer typologies in the context of subsidy for a multi-peril crop insurance through the lenses of behavioral factors in an ex ante context. For this purpose, an online survey was conducted between February and May 2022, garnering 228 farmer responses. They were reached using an email list furnished by the Saxon State Ministry for Energy, Climate Protection, Environment, and Agriculture. The study targeted Saxony, Germany, because it currently emphasizes state-backed (multi-peril) crop insurance premium subsidies (State Government Saxony, 2019). Furthermore, Saxony as part of Eastern Germany has experienced extreme weather events like heat stress in the past (BMEL, 2022; Webber et al., Reference Webber, Lischeid, Sommer, Finger, Nendel, Gaiser and Ewert2020). Germany’s uptake of private sector multi-peril crop insurance remains limited (German Insurance Federation, 2016), with no nation-wide CAP-backed subsidy in place. Some states, like Bavaria, do subsidize premiums by up to 50% (Bucheli et al., Reference Bucheli, Conrad, Wimmer, Dalhaus and Finger2023). However, Saxony has not adopted this, making it a fitting representation of regions without such implementation.
Our study primarily introduces the ex-ante identification of farmer typologies related to policies subsidizing multi-peril crop insurance premium in Europe under the CAP framework using a cluster-analysis based on behavioral factors as classified by Huber et al. (Reference Huber, Bartkowski, Brown, El Benni, Feil, Grohmann, Joormann, Leonhardt, Mitter and Müller2024). Beyond this typology, we investigated farmers’ willingness to pay (WTP) for two multi-peril insurance designs – namely, the indemnity insurance founded on Actual Production History (APH, United States Department of Agriculture, 2022) as seen in the U.S., and an index insurance already available in Saxony, Germany. Farmers’ WTP were assessed using a modified payment cardFootnote 2 approach (Mitchel & Carson, Reference Mitchel and Carson1989; Carson & Hanemann, Reference Carson and Hanemann2005). Hence, this research complements works of Doherty et al. (Reference Doherty, Mellett, Norton, McDermott, O’Hora and Ryan2021) and Gassler and Rehermann (Reference Gassler and Rehermann2022) by aligning farmers’ WTP for two insurance models with the identified farmer typologies.
This study offers valuable insights for European, especially German, policy makers involved with the CAP. Recognizing distinct farmer typologies – based on attitudes, perceptions, and characteristics – facilitates targeted policy crafting, optimizing the use of public resources and enhancing farmer participation. By understanding these varied attributes, policy makers can anticipate which farmer groups are most likely to engage, fostering more efficient, resonant interventions and averting costly, ineffective strategies. Furthermore, farmers’ typologies can aid in the communication of the policy, ensuring that it meets the needs of different groups of farmers. Lastly, insurance firms contemplating multi-peril crop insurance can leverage these typologies to identify receptive farmers, refining their marketing strategies. Insights into farmers’ willingness to pay for such insurance provide crucial data to forecast demand and potential costs.
Material and methods
Data collection and survey design
Before the data collection started, the survey was deemed unobjectionable by the University’s Ethics Committee as well as the University’s Privacy Officer. The farmers were surveyed by using an online questionnaire from February to May 2022. The acquisition of the farmers was carried out via an e-mail distribution listFootnote 3 , provided by the Saxonian State Ministry for Energy, Climate Protection, Environment and Agriculture and with the help of the ministry’s social media accounts (Facebook, Instagram). The e-mail invitation is attached in the Appendix. The e-mail invitation highlights the survey context, relevance, and policy impact of the study and that the farmers’ participation contributes to informing policy makers on their view on the anticipated policy. Both in the e-mail and surveys’ introductory page, we assured the respondents of the anonymity of their responses and emphasized the importance of their honest opinions. In addition, we assured that there are no right or wrong answers, only an interest in their honest opinions on the introductory page again. Participation in the survey was rewarded with a €10 voucher and the chance to win one of five construction site radios. By taking these steps, we encouraged genuine responses by the farmers and reduced the hypothetical bias. A total of 6,000 farms were contacted, whose email addresses are stored at the ministry. This corresponds to approximately 92% of the farm population in Saxony, which has 6,480 registered farms (Statistik Sachsen, 2019).
The survey can be divided into four parts: In the first part, the farmers were asked for sociodemographic and farm-related characteristics. In the second part, farmers’ risk attitude as well as innovativeness were assessed. Farmers’ risk attitude and innovativeness were assessed using contextualized statements regarding their behavior on the farm which had to be evaluated using an 11-point scale based on Dohmen et al. (Reference Dohmen, Falk, Huffman, Sunde, Schupp and Wagner2011). The wording was adapted from Michels et al. (Reference Michels, Rieling and Musshoff2024).
In the third part, the farmers were presented with a detailed description of two multi-peril crop insurance designs (see Appendix). The indemnity insurance is based on the Actual Production History (APH) available in the USA (United States Department of Agriculture, 2022), which was chosen as an example due to the lack of established indemnity insurance in Europe and has long been a preferred product for farmers in the USA. The index insurance is based on an insurance product that is already offered on the market in Saxony, Germany. Afterwards, the farmers were asked to indicate their maximum WTP for each insurance type in terms of percentage of the value per hectare (expected price times expected return) using a range from 0 to 8% with one percent increments following a payment card approach (Mitchel & Carson, Reference Mitchel and Carson1989; Carson & Hanemann, Reference Carson and Hanemann2005). The payment card approach is well established in agricultural economics (e.g., Yu et al., Reference Yu, Gao and Zeng2014) and was also applied in the context of agricultural insurances (e.g., Zhang et al., Reference Zhang, Hu, Zhan and Chen2020). The payment card is particularly suitable in our case, as the respondents might have limited prior experience with such insurances and policies. The approach provides values that can assist participants in forming their estimations. This reduces cognitive burden and strategic bias, where respondents might overstate or understate their WTP, by anchoring responses in realistic ranges. The values of the range to be selected by the farmers to indicate their willingness to pay was drawn up on the basis of insurance expert discussions in order to correspond as closely as possible to the real insurance premiums. In the follow-up scenario, the WTP for both types of insurance was again questioned, with the state subsidizing 50% of the total price (=fair premium + loading).Footnote 4 The subsidy of 50% was chosen on the basis of the decisions of the German Conference of Agriculture Ministers (AMK, 2019). The presentation of the insurance designs was randomized.
In the fourth and last part, the farmers were asked to evaluate statements to capture their view on the introduction of a policy subsidizing a multi-peril crop insurance. Huber et al. (Reference Huber, Bartkowski, Brown, El Benni, Feil, Grohmann, Joormann, Leonhardt, Mitter and Müller2024) showed that one main source for farmer typologies are behavioral factors. Dessart et al. (Reference Dessart, Barreiro-Hurlé and Van Bavel2019) divided behavioral factors in the context of sustainable farming practices’ adoption in cognitive (e.g., perceived benefit), social (e.g., norms) and dispositional (e.g., risk attitude). Cognitive factors are closely related to the decision context and refer to how farmers perceive the decision context (Dessart et al., Reference Dessart, Barreiro-Hurlé and Van Bavel2019). Since the introduction of multi-peril crop insurance in the Netherlands in 2010 (van Asseldonk, Reference van Asseldonk2019), there was a marked surge in adoption rates (van Asseldonk et al., Reference van Asseldonk, van der Meulen, van der Meer, Silvis and Berkhout2018). van Asseldonk et al. (Reference van Asseldonk, van der Meulen, van der Meer, Silvis and Berkhout2018) findings suggest that multi-peril crop insurance might resonate as a compelling risk management option for European farmers. Furthermore, this shifted preference from hail insurance to multi-peril crop insurance (van Asseldonk et al., Reference van Asseldonk, van der Meulen, van der Meer, Silvis and Berkhout2018) potentially pointing toward dissatisfaction with earlier risk management strategies and traditional market-based tools. Consequently, the satisfaction levels of farmers with existing risk management, along with the prevailing market scenario in terms of risk management resources, become pivotal for the acceptance of any new agricultural insurance product. Hence, close to the research context in accordance with Dessart et al. (Reference Dessart, Barreiro-Hurlé and Van Bavel2019) is farmers’ satisfaction with their risk management and market supply of risk management tools. Likewise, close to the research context is farmers’ perception of agricultural insurance product itself (e.g., multi-peril crop insurance) on the one hand and the governmental support of the insurance market on the other hand. Hence, the statements to be evaluated are used to build the following constructs based on the aforementioned considerations:
-
Construct 1: Farmers’ satisfaction with the current risk management
-
Construct 2: Farmers’ satisfaction with the supply of market-based risk management tools
-
Construct 3: Farmers’ perceived benefits of multi-peril crop insurance
-
Construct 4: Farmers’ attitude toward governmental support of the insurance market
Liesivaara and Myyrä (Reference Liesivaara and Myyrä2017) demonstrated that a farmer’s WTP for crop insurance is influenced by the availability of governmental disaster relief funds, highlighting the importance of funding sources. In this context, we also explored farmers’ views on potential sources of state subsidies for multi-peril crop insurance. Hence, participants were presented with 16 randomized statements to gauge their opinions, using a 5-point Likert scale (1 = strongly disagree; 5 = strongly agree). Additionally, a free-text field allowed farmers to share personal feelings and perspectives on subsidized multi-peril crop insurance.
Data, reliability and cluster analysis
At first, descriptive statistics were assessed to describe the demographic and farm-related characteristics as well as the approval of the 16 statements. Furthermore, the average WTP for both insurance designs was estimated and compared using a non-parametric Wilcoxon signed-rank test. Second, the reliability of the four constructs was assessed. The reliability of the constructs was evaluated using Cronbach’s alpha (α) (Cronbach, Reference Cronbach1951). Due to the criticism of the strict assumptions of tau-equivalence for Cronbach’s α (Miller, Reference Miller1995) which are rarely met (Hayes and Coutts, Reference Hayes and Coutts2020; Raykov, Reference Raykov2001, Reference Raykov1997; Sijtsma, Reference Sijtsma2009), we also estimated the more robust Guttmann Lambda-2 (λ2) (Guttmann, Reference Guttmann1945) and Raykov’s rho (ρ) (Raykov, Reference Raykov2001, Reference Raykov1997), also known as McDonalds’ omega (ω) (McDonald, Reference McDonald2013, Reference McDonald1970), with the common cut-off level >0.7 for all reliability quality criteria. The constructs serve as the basis for the cluster analysis in the third step. The goal of the cluster analysis is to obtain homogeneous subgroups (Backhaus et al., Reference Backhaus, Erichson, Plinke and Weiber2016). The hierarchical cluster analysis applying the Ward method and squared Euclidean distance as the (dis)similarity measure identifies the various options for the number of clusters (Mooi et al., Reference Mooi, Sarstedt and Mooi-Reci2018). The number of clusters was determined by a systematic comparison of the cluster possibilities using a dendrogram and the difference in the variance ratio criterion values of each cluster solution (Caliński and Harabasz, Reference Caliński and Harabasz1974). Lastly, the identified cluster number from the Ward’s linkage analysis were used as a starting point in the final partitioning cluster method (k-means). The overlap between the cluster solutions should be more than 80% to confirm the selected number of clusters (Mooi et al., Reference Mooi, Sarstedt and Mooi-Reci2018). To assess the heterogeneity of the identified clusters non-parametric Kruskall–Wallis-Tests with a post hoc Dunn’s tests are applied.
Results and discussion
Descriptive results
Of the more than 6,000 contacted farms, 1,022 responded to the invitation and started the online survey. After filtering out questionnaires with illogical or incomplete information, 228 fully completed questionnaires were available for analysis. Of the 794 farmers who have not finished the survey, 597 farmers dropped out before completing the second part of the survey. This represents a sample size of 3.5% of the farms in the Free State of Saxony. The descriptive results of this sample are presented in Table 1. The sample is slightly biased toward relatively young farmers from larger farms with a relatively high proportion of farmers holding an academic degree. It can be assumed that many farmers who did not participate in the survey may not be interested in such insurance products. Therefore, the sample of younger, better-educated farmers from larger farms represents the group of farmers who are most likely to adopt a multi-peril crop insurance and participate in such a policy scheme, as shown by the literature reviewed in the introduction. Hence, it is important to assess their attitudes and perceptions toward this type of insurance. Still, results have to be seen in the light of bias of the sample.
Table 1. Descriptive statistics of the sample (N = 228)

Note: SD = Standard deviation.
a Dummy coded [0;1].
b Statistik Sachsen (2020a).
c Statistik Sachsen (2020b).
d WetterKontor (2022).
e Saxonian State Ministry for Energy, Climate Protection, Environment and Agriculture (2022).
f Related to farm manager.
g Based on a 11-point Likert scale (1 = not at all open to innovations; 11 = very open to innovations).
h Based on a 11-point Likert scale (1 = risk averse; 11 = risk seeking).
Table 2 shows the results for the statement evaluation which allow for the following implications: Farmers are relatively dissatisfied with their risk management. While there is also evident dissatisfaction with the current offerings in the market, there is potential for bridging this gap. Targeted training and education initiatives can enable farmers to better understand risk and effectively leverage existing risk management tools (Giampietri et al., Reference Giampietri, Yu and Trestini2020). For instance, Möllmann et al. (Reference Möllmann, Michels, von Hobe and Mußhoff2018) and Michels et al. (Reference Michels, Möllmann and Musshoff2019) showed that some risk management tools, such as commodity futures contracts, are not widespread among German farmers. Farmers perceive a multi-peril crop insurance basically beneficial. Despite the available index-based multi-peril crop insurance in Saxony, its adoption remains conspicuously low. This either points to a possible knowledge or awareness deficit or a lack of comprehension about the intricacies of such instruments. This finding also underscores the potential success of training farmer in risk management. The data reveals farmers’ ambivalence regarding governmental intervention in the insurance sector. They appear favorable to the idea of additional funds for insurance subsidies, but express reservations about reallocating funds, especially from established avenues like the CAP’s direct payments which is in line with Möllmann et al. (Reference Möllmann, Michels and Musshoff2019) and Michels et al. (Reference Michels, Möllmann and Musshoff2020). Likewise, using money from the 2nd pillar as it is intended in the CAP is not really approved by the farmers. Thus, farmers’ acceptance of a state-subsidized multi-peril crop insurance is contingent upon its source of funding. They seem to lean toward the infusion of fresh funds rather than the reshuffling of existing allocations
Table 2. Descriptive results on the statement evaluation (N = 228)
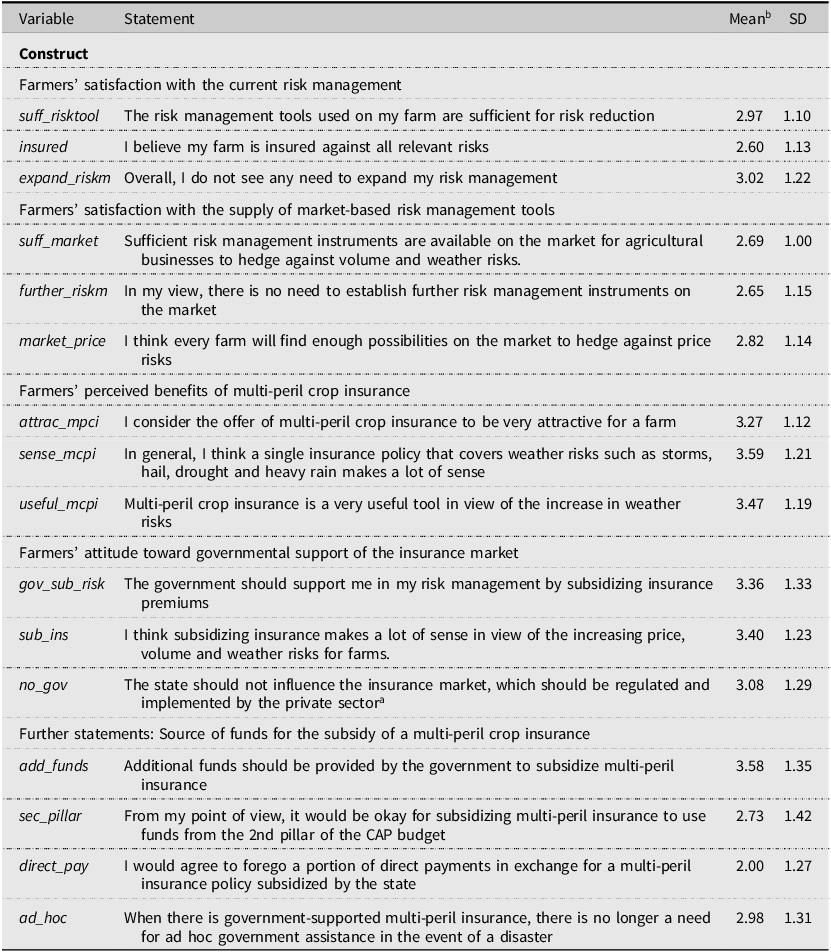
Note: Statements/indicators corresponding to one specific construct are shown below the corresponding construct. SD = Standard deviation.
a Reversed coded used for the reliability analysis.
b Likert scale 1 = fully disagree; 5 = fully agree.
Farmers were also given the chance to express their feelings in a free text field. The open-ended feedback from farmers uncovers deeper concerns. They are wary about subsidies potentially benefiting only specific subsets of farms or remaining with the insurance entities. Additionally, there’s a firm stance against any alterations to direct payments, emphasizing the importance farmers place on this financial support. However, farmers welcome state support with regard to the cost of an insurance and increasing (weather) risks they face. Curiously, a subset of agricultural producers interprets multi-peril crop insurance as a plausible compensatory mechanism for any prospective political impositions restricting the use of specific active ingredients (European Commission, 2022). This sentiment may find its roots in our presentation of an indemnity insurance framework (as detailed in the Appendix), which considers potential managerial oversights.
Table 3 illustrates the average WTP for two multi-peril crop insurance designs as a percentage of the value per hectare. The data show comparable WTP percentages for indemnity (2.61%) and index insurance (2.52%). Utilizing a non-parametric Wilcoxon signed-rank test, these percentages were found to be statistically indistinguishable, suggesting no marked preference among farmers for either indemnity or index insurance. This is a departure from the findings of Doherty et al. (Reference Doherty, Mellett, Norton, McDermott, O’Hora and Ryan2021) where Irish farmers demonstrated a preference for index-based schemes. Given that our index insurance mirrored a real product offering, the low uptake cannot be solely ascribed to potential misgivings farmers might have about the insurance’s structure. When contemplating a 50% insurance premium subsidy, WTP percentages for the indemnity and index insurance stand at 1.58% and 1.60%, respectively. These values, like those in the no-subsidy scenario, are statistically similar. A subsidy, therefore, does not seem to shift farmer preferences noticeably toward one insurance type over the other.
Table 3. Farmers’ willingness to pay for the two designs of the multi-peril crop insurance (N = 228)¥

Note:
† Average willingness to pay of farmers without state support (50% of the premium).
¥ Range from 0 to 8% of the value per hectare. SD = Standard deviation.
a No statistically significant differences according to Wilcoxon signed-rank test between indemnity and index insurance without a subsidy.
b No statistically significant differences according to Wilcoxon signed-rank test between indemnity and index insurance without a subsidy.
Two more remarkable results should be mentioned: First, WTP under the subsidy scenario is more than half of the non-subsidized scenario. This mirrors findings by Mußhoff et al. (Reference Mußhoff, Hirschauer, Grüner and Pielsticker2018), suggesting that when governmental subsidies support a particular scheme, farmers might interpret this as a seal of quality or endorsement. Second, though 14 and 17% of participants revealed zero WTP for indemnity and index insurance respectively, these figures, when considered against the survey’s low response rate, hint at a tepid interest in such insurance products. For the index insurance reflecting an actual market product, insurance specialists propose an average value per hectare at 5.90%, which considerably exceeds the average farmer WTP – even if government subsidies are factored in. This discrepancy indicates that only a minority of farmers might opt for such an offer, and even noteworthy state subsidies might not substantially amplify this uptake. This underscores a need for more substantial governmental support for wider adoption. In other words, larger additional governmental support as intended (50% is needed for a broad implementation.
Drawing from the insights of both Tables 2 and 3, several pivotal policy implications emerge: Consistent with the arguments made by Buckwell et al. (Reference Buckwell, Matthews, Baldock and Mathijs2017), governmental intervention, when introducing such policies, should be visualized not as a long-term crutch but as a short-term catalyst for private insurance markets. The evident gap between farmers’ WTP and the expert-recommended average underscores the need for a state-led initiative. However, this involvement should be time-bound to avert sustained market distortions and encourage the organic growth of private insurance offerings. While subsidies are vital, the data suggest that there might be more pronounced long-term gains in redirecting some of these funds toward farmer education and advisory services focused on risk management. By leveraging the findings of our study, government agencies and cooperatives can design tailored educational initiatives to amplify farmers’ awareness about extant market-driven risk management tools. Such an approach not only addresses immediate concerns but fosters a more informed and resilient farmer community. One intriguing avenue for future exploration is assessing the impact of governmental endorsements on farmers’ decision-making processes. Could a state recommendation or a “seal of approval” for specific risk management tools serve as a catalyst for higher adoption rates? Such endorsements might bridge trust deficits and facilitate more widespread use of these tools in the farming community.
Reliability and cluster analysis
Table 4 shows the reliability analysis of the constructs. Loadings of >0.55 are acceptable for a sample size of >100 respondents (Hair et al., Reference Hair, Black and Babin2010) as also applied by Greiner (Reference Greiner2015). Likewise, all values exceed the threshold of 0.7 for Cronbach’s α, Raykov’s ρ/McDonalds’ ω, and Guttmann λ2. Hence, reliability of the constructs is confirmed.
Table 4. Reliability analysis of the constructs via several quality criteria (N = 228)

Note: Statements/indicators corresponding to one specific construct are shown below the corresponding construct.
a Reverse coded.
b Cut-off level > 0.55.
c Cut-off level > 0.7.
Despite having a presumably younger, better educated farmers from larger farms, the procedure described in Section 2.2 lead to a four-cluster solution with distinct characteristics and attitudes. The minimum value of the differences in the variance ratio criterion (−4.11) is in favor of a four-cluster solution. Additionally, the validity of the four-cluster solution is confirmed by the overlap of 89% with the results provided by the k-means method (Mooi et al., Reference Mooi, Sarstedt and Mooi-Reci2018). Results of the cluster analysis are displayed in detail in Table 5 comprising sociodemographic and farm-related characteristics, results of all statements as well as farmers’ WTP for both multi-peril crop insurance designs. Some remarkable results should be highlighted:
Table 5. Comparison of the identified clusters (N = 228)

† Reverse coded.
ϕ Likert scale 1 = fully disagree; 5 = fully agree.
¥ Range from 0 to 8% value per hectare.
Minimum value of ω k = −4.11 at four cluster.
Overlap with k-means analysis 89.03%.
Kruskall–Wallis-Test *p < 0.1; **p < 0.05; ***p < 0.01.
Cluster sharing a letter are not statistical significantly different at the 10% level according to post-hoc Dunn’s test.
The first cluster “Seekers for risk management support without government intervention” contains 14% (N = 32) of the farmers. The subsample is characterized by farmers who are relatively dissatisfied with their current risk management. Farmers in this cluster are notably dissatisfied with their current risk management. Although they express a clear dissatisfaction with the market offerings of risk management tools, they do not perceive the multi-peril crop insurance as attractive and are not in favor of government intervention in insurance premiums. Their farms are predominantly characterized by grassland, and the cluster houses a very low proportion of part-time farmers. It is inferred that many of these full-time farmers might be livestock farmers, potentially explaining their disapproval of government intervention, particularly since most support discussions relate to weather risks linked with cash crop farming. They show a distinct desire to keep direct payments unchanged, especially ad hoc disaster payments. Their WTP for the indemnity design of a multi-peril crop insurance amounts to 2.62% and for the index design to 2.37%. The WTP does not differ statistical significantly from cluster 2 but from cluster 3 and 4.
The second cluster “Risk handlers in favour of multi-peril crop insurance” consists of 33% (N = 76) and represents the largest cluster. Farmers here seem relatively content with their current risk management tools and the market’s provisions. Given their satisfaction, it is no surprise they would endorse a multi-peril crop insurance, potentially even a subsidized one, to bolster their risk management arsenal. However, their WTP for a multi-peril crop insurance (2.65%, indemnity design; 2.51%, index insurance) is not statistically significantly different from cluster 1, but only from clusters 3 and 4, which indicate a higher WTP.
The third cluster “Advocates of governmental support in risk management” is the second largest cluster with 31% (N = 70) of the farmers. Farmers in this group express pronounced dissatisfaction with their current risk management tools and the available market alternatives. Their strong inclination toward a multi-peril crop insurance is evident, with a marked preference for governmental backing, especially through additional financial resources. This cluster’s farmers, predominantly full-time, have a noteworthy portion of leased land and have committed substantial investments in recent years. This could suggest high financial commitments on their part, making them inclined toward risk management tools, particularly when supported by the government. In addition, this cluster shows the highest WTP for both designs of the multi-peril crop insurance (3.15%, indemnity insurance; 3.26%, index insurance), which is also statistically significant from the other three clusters.
The fourth and last cluster “Satisfied market-orientated risk-manager” contains 22% (N = 50) of the farmers. Farmers in this cluster exhibit satisfaction with their existing risk management tools and what the market provides. They do not see a need for multi-peril crop insurance and are averse to governmental intervention in this domain. Overall, farmers in this cluster see no need for any change in risk management as well as corresponding policies, especially they demand the direct payments to be untouched. Another notable characteristic of this cluster is the relatively small amount of farm land, low share of rented farm land and the high share of part-time farmer. Accordingly, their risk exposure might be low. Their WTP for multi-peril crop insurance (1.78%, indemnity insurance; 1.60% index insurance) is statistically significantly lower than that of the other three clusters.
The cluster analysis highlights the diversity within the farming community, leading to varied reactions to policies like multi-peril crop insurance subsidies. Despite cluster 3’s inclination toward governmental support, their average WTP (3.15% for indemnity insurance and 3.26% for index insurance) is considerably lower than the 5.90% average stated by insurance experts. Thus, a 50% governmental insurance premium subsidy might only marginally increase acceptance among a specific subset of farmers. Many farmers in clusters 1, 2, and 3 seem discontented with their current risk management. This underlines the potential need for more investments in risk management education rather than premium promotions. The juxtaposition of a low WTP and relative dissatisfaction suggests a gap in farmers’ understanding of risk and the calculation of insurance premiums. Insurance companies, therefore, should enhance transparency concerning insurance premiums. Across all clusters, there is a consistent call to maintain the financial resources allocated for direct payments, reflecting their importance in farmers’ incomes as noted by Michels et al. (Reference Michels, Möllmann and Musshoff2020).
Limitations
As suggested by one anonymous reviewer, one of the primary limitations of this study could be its confined temporal scope. The data collection was limited to a two-month period within a single year. However, a shorter, defined period ensured consistency in external conditions, crucial for a clear, focused analysis. This approach minimized variance that might arise from longer-term fluctuations such as weather changes or market dynamics. Furthermore, considering the agricultural context in Germany, where harvest occurs only once a year, our survey timing strategically avoids the immediate biases of the harvest season. This timing is critical as it captures a period where farmers’ attitudes are less influenced by the direct pressures of harvest, thus providing a more stable and representative view of their perceptions and decisions. While the considered attitudes and perceptions reflect inherent latent traits that are less likely to strongly fluctuate within one year, it could still be of interest to observe them over a longer period of time. Hence, conducting similar surveys over multiple years could provide valuable insights into how farmers’ attitudes and decisions evolve over time, adding a longitudinal perspective to our understanding of agricultural insurance.
One notable limitation of our study, as highlighted in the results, is the bias in the sample. We contacted almost all famers in Saxony via the provided e-mail list. However, this could still skew our sample toward younger, more technologically adept farmers (e.g., younger, well-educated), as indicated in the descriptive statistics of our sample (Table 1). Such a demographic might have different risk perceptions, economic behaviors, and receptiveness to multi-peril crop insurance compared to the broader farming population. Consequently, our findings, especially regarding attitudes toward multi-peril crop insurance and willingness to pay, must be interpreted with this potential bias in mind.
Another limitation to be mentioned in the elicitation of the willingness to pay is the potential hypothetical bias. While we employed several strategies to minimize the hypothetical bias, it still needs to be mentioned, and further efforts are needed to reduce hypothetical bias in surveys and experiments.
Summary, implications, and outlook
Amid escalating weather risks, multi-peril crop insurance has emerged as a salient tool against varied weather challenges. Despite its potential, a fully-fledged private-sector market for this insurance in Europe remains underdeveloped, leading to discourse on potential premium subsidies amongst European policy makers and agricultural economists. Prior studies have delved into farmers’ WTP for (subsidized) multi-peril crop insurance within the CAP framework, but the diverse attitudes of farmers in the context of a subsidy for multi-peril crop insurance premium remain relatively uncharted. Recognizing the inherent heterogeneity among farmers, our study aimed to discern these differences, grouping farmers into distinct typologies based on their satisfaction with current risk management and market supply of risk management tools, their inclination toward multi-peril crop insurance, and opinions on government intervention in the insurance market. This endeavor was supplemented by assessing their WTP for this insurance, either in its indemnity or index form. To achieve this, a comprehensive online survey involving a segment of the farming population from the Free State of Saxony, Germany, was conducted in 2022. The online survey resulted in 228 (of over 6,000 invited) participating Saxonian farmers. Reliability analysis was used to establish the constructs which were used in cluster analysis to identify the targeted subgroup of farmers. These clustered insights are pivotal for policy makers and insurance providers, shedding light on the diverse perspectives within the farming community and how they might shape responses to new policies, especially those around multi-peril crop insurance. By understanding the heterogeneity stakeholders can craft more informed, effective policies to support farmers in their risk management.
Following implications can be given: Given the disconnect between the WTP and the market price of insurance products, insurance companies could focus on greater transparency. Explaining the pricing structure, factors that determine premiums, and potential benefits can foster trust and potentially increase uptake. While direct financial incentives, like subsidies, are a common approach, the study suggests they might not always be effective in increasing uptake. Policy makers could explore alternative incentives to promote multi-peril crop insurance adoption. In this regard, the possibility that farmers view government subsidies as an assurance of quality means that endorsements or certifications from government bodies for specific risk management tools or insurance products might boost farmer confidence and uptake. The dissatisfaction expressed by a noteworthy proportion of farmers regarding their current risk management strategies implies a need for enhanced education and outreach. Thus, policy makers and other stakeholders can alternatively evaluate to invest in programs that educate farmers about the nuances of available risk management tools, its benefits, and how they can be effectively integrated into their risk management toolbox.
However, if policy makers insist to implement such a policy, farmers’ heterogeneity should be considered. In specific, farmers are not a monolithic group but have diverse attitudes toward multi-peril crop insurance and government intervention. Findings of the study suggests that a one-size-fits-all policy approach may not be effective. This holds also true for the communication of such a policy to effectively reach all farmers by addressing the specific needs and concerns of different farmer clusters. Given the diverse nature of the farming community and farmers’ stated concerns, solutions need to be scalable and flexible to consider farmers’ heterogeneity also in their socio-demographic and farm-related characteristics.
For future research directions, extending this study to another EU member state could offer comparative insights and further solidify our findings, especially with a more representative sample. Furthermore, if could be of interest to investigate more in depth how different governmental intervention (e.g., recommendations, subsidies) influence the adoption of (multi-peril) crop insurance products.
Supplementary material
The supplementary material for this article can be found at https://doi.org/10.1017/aae.2024.8.
Data availability statement
Data available on request due to privacy/ethical restrictions.
Acknowledgements
We thank two anonymous referees and the editor for helpful comments and suggestions. Financial support by the German Research Foundation (DFG) is gratefully acknowledged.
Author contribution
Conceptualization, M.M., O.M.; methodology, M.M..; formal analysis, M.M..; data curation, M.M., H.W.; writing – original draft, M.M., H.W., O.M., writing – review and editing, M.M., H.W., O.M.; supervision, O.M..; funding Acquisition, M.M., O.M.
Competing interests
Marius Michels, Hendrik Wever, and Oliver Mußhoff declare none.