What is needed and why?
Theory in crisis
The field of language acquisition is prolific, with an extensive range of high-quality research published every year. However, there has been surprisingly slow progress in solving some long-standing controversies regarding the basic mechanisms that underlie language acquisition. For instance, do infants learn language primarily from extracting statistics over speech inputs (Romberg & Saffran, Reference Romberg and Saffran2010; Saffran & Kirkham, Reference Saffran and Kirkham2018), from examining cross-situational correlations over multisensory inputs (Smith & Yu, Reference Smith and Yu2008; Suanda, Mugwanya & Namy, Reference Suanda, Mugwanya and Namy2014; Yu & Smith, Reference Yu and Smith2017; Zhang, Chen & Yu, Reference Zhang, Chen and Yu2019), or by relying on social interactions and feedback (Tomasello, Reference Tomasello2003; Tsuji, Cristia & Dupoux, Reference Tsuji, Cristia and Dupoux2021; Yu & Ballard, Reference Yu and Ballard2007)? Do they learn by leveraging discrete linguistic categories or continuous sensory representations (Kuhl et al., Reference Kuhl, Conboy, Coffey-Corina, Padden, Rivera-Gaxiola and Nelson2008; McMurray, Reference McMurray2021)? Do they rely on language-specific or domain-general learning mechanisms (Elman, Bates & Johnson, Reference Elman, Bates and Johnson1996; Karmiloff-Smith, Reference Karmiloff-Smith1994; Pinker, Reference Pinker1994)? Such a lack of headway may be due in part to the ‘replication crisis’: the experimental study of human cognition in general and infant cognition, in particular, is inherently noisy and difficult (Frank, Bergelson, Bergmann, Cristia, Floccia, Gervain, Hamlin, Hannon, Kline & Levelt, Reference Frank, Bergelson, Bergmann, Cristia, Floccia, Gervain, Hamlin, Hannon, Kline and Levelt2017), slowing down cumulative progress. Here, we explore the possibility that there is, in addition, a ‘theory crisis’. To say it bluntly, perhaps, current theories have shortcomings that prevent us from even finding the right experimental setup to make progress on basic questions about learning mechanisms.
Several papers have already been devoted to the theory crisis in psychology in general; psychological theories have been claimed to be mere statistical model fitting (Fried, Reference Fried2020), too descriptive or fragmented (Muthukrishna & Henrich, Reference Muthukrishna and Henrich2019), or to not contribute in cumulative theory building (McPhetres et al., Reference McPhetres, Albayrak-Aydemir, Mendes, Chow, Gonzalez-Marquez, Loukras, Maus, O’Mahony, Pomareda and Primbs2021). In developmental psychology, Kachergis, Marchman, and Frank (Reference Kachergis, Marchman and Frank2021) called for a ‘standard model’ that would allow integration of results in a cumulative fashion. In this paper, we explore the possibility proposed in Dupoux (Reference Dupoux2018) that recent advances in machine learning could help address the theory crisis through systems that realistically simulate how infants learn language in their natural environment. Such learning simulations are computer models that would ideally learn from similar inputs as the ones available to infants (raw sensory data), and reproduce the broad spectrum of outcome measures as obtained in laboratory experiments or corpus studies. To the extent that these new computer models are powerful enough to address the complexity and variability of data available to infants during language development, they could help us make progress in some of the aforementioned controversies. At best, such learning simulations can provide proof of principle that a given hypothesis (e.g., the statistical learning hypothesis) can account for learning outcomes as observed in infants. In addition, they can help us go beyond said long-standing controversies by providing new insights into the learning process and a wealth of associated quantitative predictions.
In this paper, we first discuss how these new types of learning simulations are complementary to more familiar theoretical approaches in cognitive development and argue that they provide one step towards the needed cumulative integrative theories or standard models. We then present STELA, a recent learning simulation implementing the hypothesis that infants are statistical learners, and show how it provides insights into some long-standing controversies.
Varieties of theories in language acquisition
The theoretical landscape of language development is vast and complex. Even if one focuses on early language development, there are wild varieties of theoretical approaches that differ not only in scope (the range of phenomena they cover) but also in style (verbal, statistical, formal, computational). Here, far from making a comprehensive survey of these approaches, we attempt to classify them into types and sort them along dimensions that outline their respective strengths and weaknesses with regard to addressing basic questions/controversies about learning mechanisms. Familiar types are verbal frameworks (among others: The competition model: MacWhinney & MacWhinney, Reference MacWhinney and MacWhinney1987; WRAPSA: Jusczyk, Reference Jusczyk1993; Usage-based theory: Tomasello, Reference Tomasello2005; NML-e: Kuhl et al., Reference Kuhl, Conboy, Coffey-Corina, Padden, Rivera-Gaxiola and Nelson2008; PRIMIR: Werker & Curtin, Reference Werker and Curtin2005), which weave a narrative around a large body of experimental research using verbally defined concepts, sometimes complemented by box-and-arrow schemas (e.g., the ScALA framework from Tsuji et al., Reference Tsuji, Cristia and Dupoux2021). Correlational approaches (e.g., Fernald, Marchman & Weisleder, Reference Fernald, Marchman and Weisleder2013; Hart & Risley, Reference Hart and Risley1995; Swingley & Humphrey, Reference Swingley and Humphrey2018) aim to identify the main variables that predict language development outcomes through statistical models. Formal models (e.g., Jain, Osherson, Royer & Sharma, Reference Jain, Osherson, Royer and Sharma1999; Tesar & Smolensky, Reference Tesar and Smolensky2000) and computational models (e.g., Brent, Reference Brent1997) aim to study how algorithms can learn language through mathematical proofs or empirical study of the learning outcomes. All theoretical approaches of early language development recognise that infants receive inputs from their environment, and have a learning mechanism, which produces a linguistic competence that can be accessed through outcome measures. The differences between these theoretical approaches lie in the simplifying assumptions and degree of specifications they make about inputs, learning mechanisms and outcome measures. We distinguish four dimensions or axes to sort these theoretical approaches: Causal versus Correlational, Quantitative versus Qualitative, Realistic versus Abstract, and Broad Scope versus Narrow Scope.
Causal/Correlational
A theory is causal when it provides a specification/implementation of the learning mechanism underlying language acquisition; it is correlational when it focuses on the input/outcome relationship without specifying a learning mechanism. A correlational model can outline the important factors that drive learning and therefore provide insights into the development of learning mechanisms. However, only a causal model can provide proof of principle that a postulated learning mechanism is sufficient to reproduce a developmental outcome given an input. As a result, to the extent that they can be effectively implemented, causal models are better positioned to resolve disagreements about learning mechanisms than correlational models.
Quantitative/Qualitative
A theory is quantitative if it can produce numerical outcomes that can be compared to human performance. It is qualitative when it produces predictions about the possible presence of a significant effect without a numerical prediction about its strength. Qualitative models are useful to inspire novel experimental paradigms, and provide insights about learning mechanisms, but are hard to refute and difficult to compare to one another. Quantitative theories make very precise predictions and can be compared to one another by computing the degree of fit of their predictions against some observed outcome. As a result, they are better positioned to solve disagreements about learning mechanisms than qualitative theories.
Realistic/Abstract
A theory is realistic when its model of the environment is as close as possible to the actual sensory/motor environment of the child. It is abstract when the environment is specified through synthetic data, or human/categorical annotations of observed environments (e.g., textual transcriptions). Abstract theories are useful because they enable a high degree of control and interpretability and provide insights into what type of input information can yield particular outcomes. However, they cannot prove that their conclusions apply to real-world data as perceived by infants and are therefore not very informative when it comes to solving long-standing controversies. Realistic theories, in contrast, to the extent that they can be effectively implemented, are better positioned: because they directly reproduce the learning outcomes associated with a given input and learning mechanism.
Broad/Narrow Scope
A theory has a broad scope if it encompasses not one single linguistic level (phonetic, morphological, syntactic, semantic, etc.) or phenomenon but several at once. Narrow Scope theories are useful in focusing on learning specific representations, assuming all other representations are fixed. However, many controversies about learning mechanisms arise because of co-dependencies between linguistic levels, making it problematic to assume all levels are fixed except one. Being able to account for how infants can learn jointly all of these levels is at the heart of solving so-called ‘bootstrapping’ problems that are integral to language learning.
In Table 1, we position some familiar theoretical approaches in terms of these four axes. This characterisation may seem overly simplistic or reductionist, but we hope it will help outline the specific contribution of learning simulations. Verbal frameworks typically have a broad scope and embrace the complexities of the child’s real environment. They are causal to the extent that they mention specific learning principles but are not on the quantitative side. They are still the single most influential theoretical approach for infant language learning, providing insight into large quantities of experimental results. However, they resist empirical refutations or amendments because of their qualitative nature. Correlational models are on the quantitative side and integrate many variables and levels. When informed by a corpus of infant/caretaker interactions, they can reveal the relationships between input quality, quantity, and language outcome (Fernald et al., Reference Fernald, Marchman and Weisleder2013; Hart & Risley, Reference Hart and Risley1995). However, because they are not causal and rely on abstract variables derived from the input, they cannot directly speak to learning mechanisms. Computational/formal models (henceforth called learning simulations) are both causal and quantitative, but their ability to significantly impact controversies about learning mechanisms depends on the breadth of their scope and their degree of realism or abstraction. We discuss such models in more detail in the next section.
Table 1. Four dimensions along which theoretical approaches of language acquisition can be sorted

A brief history of learning simulations
For a long time, scientists with various backgrounds, from formal linguistics to developmental psychology and artificial intelligence, have contemplated the possibility of building mathematical models or computer simulations of language learning in infants. The hope was that building a simulated ersatz of the infant would reveal the formal conditions for learning (Pinker, Reference Pinker1979), would allow us to better formulate hypotheses about how infants actually learn (Frank, Reference Frank2011; Meltzoff, Kuhl, Movellan & Sejnowski, Reference Meltzoff, Kuhl, Movellan and Sejnowski2009) or would yield machines that learn in a graceful and robust fashion (Turing, Reference Turing1950). Here again, the diversity of the proposed models is too large to be reviewed (see Dupoux, Reference Dupoux2018, for an attempt). Instead, we classify the approaches based on the dimensions which we claim are central to answering key questions about learning mechanism: realism and scope.
As illustrated in Figure 1, all learning simulations consist of three components: a model of the environment, a model of the learner, and a model of the outcome measure. The model of the environment specifies the type of inputs/interactions available to the learner. The learner updates itself using a learning algorithm based on its interaction with the environment. The outcome measures of the learner are measured after exposure to speech. Where learning simulations differ is how they implement these three components.

Figure 1. General outline of a realistic learning simulation (centre) in relation to real infants (left) and traditional theoretical approaches (right). 1. Verbal frameworks inspire and help set up the entire language learning simulation by describing the environment, learner, and outcome models; 2. Corpus studies of children’s input help us build realistic models of the environment. In the best case, the model of the environment is a subset of a real environment, obtained through child-centred long-form recordings, for instance; 3. Machine learning provides effective artificial language learners. The learner model is relatively unconstrained as learning mechanisms used by the real learner (i.e., infants) remain largely unobservable; 4. Correlational models describe how the input should relate to the outcome measures; 5. Experimental and corpus studies of children’s outcomes show how we can evaluate learning outcomes of the artificial learner. The real versus predicted outcome measures allow us to compare humans to machines and provide new predictions for correlational models that relate input to outcomes in infants.
Focusing on AI-inspired models, the most visible trend historically has been on how to implement the learner. Early models (e.g., Anderson, Reference Anderson1975; Kelley, Reference Kelley and Levine1967) were rule-based. The second phase was probabilistic models (e.g., Brent, Reference Brent1996; de Marcken, Reference de Marcken1996), followed by connectionist and deep learning models (Brown et al., Reference Brown, Mann, Ryder, Subbiah, Kaplan, Dhariwal, Neelakantan, Shyam, Sastry, Askell, Agarwal, Herbert-Voss, Krueger, Henighan, Child, Ramesh, Ziegler, Wu, Winter, Hesse, Chen, Sigler, Litwin, Gray, Chess, Clark, Berner, McCandlish, Radford, Sutskever and Amodei2020; Elman, Reference Elman1990), each phase replacing hand-wired components with more and more powerful learning systems. As far as we are concerned, the way in which the learner is implemented is irrelevant. What counts is whether the learning mechanism actually reproduces the learning outcome or not, given infants’ inputFootnote 1. More relevant to our argument, another trend can be seen regarding the model of the environment, moving from synthetic data (e.g., Elman, Reference Elman1990; Vallabha, McClelland, Pons, Werker & Amano, Reference Vallabha, McClelland, Pons, Werker and Amano2007) to transcribed corpora (e.g., Bernard et al., Reference Bernard, Thiolliere, Saksida, Loukatou, Larsen, Johnson, Fibla, Dupoux, Daland and Cao2020) and, more recently, to raw audio and images or video recordings (Räsänen & Khorrami, Reference Räsänen and Khorrami2019; Schatz, Feldman, Goldwater, Cao & Dupoux, Reference Schatz, Feldman, Goldwater, Cao and Dupoux2021). Finally, the first models were focused on learning a single linguistic level (e.g., phonetic categories: Vallabha et al., Reference Vallabha, McClelland, Pons, Werker and Amano2007; word forms: Brent, Reference Brent1999; word meanings: Roy & Pentland, Reference Roy and Pentland2002; syntax: Pearl & Sprouse, Reference Pearl and Sprouse2013), and more recent approaches would learn several levels jointly (phonemes and words: Elsner, Goldwater & Eisenstein, Reference Elsner, Goldwater and Eisenstein2012; syntax and semantics: Abend, Kwiatkowski, Smith, Goldwater, Steedman, Reference Abend, Kwiatkowski, Smith, Goldwater and Steedman2017; phonetics, words and syntax: Nguyen et al., Reference Nguyen, de Seyssel, Rozé, Rivière, Kharitonov, Baevski, Dunbar and Dupoux2020).
In other words, thanks to recent progress in machine learning and AI (Bommasani et al., Reference Bommasani, Hudson, Adeli, Altman, Arora, von Arx, Bernstein, Bohg, Bosselut, Brunskill, Brynjolfsson, Buch, Card, Castellon, Chatterji, Chen, Creel, Davis, Demszky and Liang2021), learning models that are simultaneously of broad scope and able to ingest realistic data are around the corner. Obviously, a complete model that would feature maximal scope (integrating all relevant input and output modalities for language and communication) and maximal realism (using sensory data indistinguishable from what infants experience) is still out of reach. In the next section, we examine STELA, a recently proposed model (Lavechin, de Seyssel et al., Reference Lavechin, de Seyssel, Titeux, Bredin, Wisniewski, Peperkamp, Cristia and Dupoux2022c) and argue that even though it is limited both on scope and realism, this work can help us make nontrivial progress on some of the long-standing controversies regarding language learning mechanisms.
Before moving on, let us clarify that we are not claiming that broad-scope realistic simulations are the only valuable approach. Narrow-scope abstract models still have valuable contributions to make (e.g., Frank, Goodman & Tenenbaum, Reference Frank, Goodman and Tenenbaum2009; Kachergis et al., Reference Kachergis, Marchman and Frank2021). First, contrary to many realistic and broad-scope models, abstract and narrow models are interpretable and therefore allow building bridges with verbal frameworks. They are also more tractable and can be easily modified and experimented on in a way which is more difficult with larger models. Finally, one can view abstract learning simulations as “control” experiments: by comparing an abstract and a realistic learning simulation implementing a similar learning mechanism, we can gain knowledge on the role of specific abstractions made by the learner.
What has been achieved so far?
Among the competing hypotheses regarding the learning mechanisms that underlie early language learning, the one that seems the most natural to approach with learning simulations is the statistical learning hypothesis (Pelucchi, Hay & Saffran, Reference Pelucchi, Hay and Saffran2009; Saffran, Aslin & Newport, Reference Saffran, Aslin and Newport1996). It posits that infants learn at least some linguistic levels (phonetic, lexical and morphosyntactic) through a statistical or distributional analysis of their language inputs. The idea has a long history (Rumelhart, McClelland & MacWhinney, Reference Rumelhart, McClelland and MacWhinney1987; Skinner, Reference Skinner1957) and has generated many controversies (Chomsky, Reference Chomsky2013; Fodor & Pylyshyn, Reference Fodor and Pylyshyn1988) and mathematical investigation (Gold, Reference Gold1967; Jain et al., Reference Jain, Osherson, Royer and Sharma1999). But it is also the simplest hypothesis to implement in a learning simulation. If one equates language input to the auditory modality, the corresponding learning simulation would simplify the environment to audio recordings, and the learner to a probabilistic model that accumulates statistics paying no attention to other modalities or context, nor interacting with its environment.
Here, we present recent work on simulating a statistical learner for language acquisition (Lavechin et al., Reference Lavechin, de Seyssel, Métais, Metze, Mohamed, Bredin, Dupoux and Cristia2022b; Lavechin, de Seyssel et al., Reference Lavechin, de Seyssel, Titeux, Bredin, Wisniewski, Peperkamp, Cristia and Dupoux2022c). We present the simplifying assumptions made in these simulations and reflect on how simulated learners compare to infants. Then, we go over different use cases of such a simulation by showing how some of the skills the simulated learner has acquired through exposure can help shed light on some long-standing controversies in our understanding of language acquisition in infants.
We focus on a high-level description of this simulation as we believe it makes it easier to appreciate its lessons. However, readers interested in the technical details can refer to the original paper (Lavechin, de Seyssel et al., Reference Lavechin, de Seyssel, Titeux, Bredin, Wisniewski, Peperkamp, Cristia and Dupoux2022c). We will also list specific research use cases that the framework helped deepen. By doing so, we illustrate concretely how such realistic learning simulations can help future research, both in terms of proof of feasibility and inspiration for research.
Introducing STELA
Lavechin, de Seyssel et al. (Reference Lavechin, de Seyssel, Titeux, Bredin, Wisniewski, Peperkamp, Cristia and Dupoux2022c) introduced STELA (STatistical learning of Early Language Acquisition), a language learning simulation that tackles the problem of discovering structure in the continuous, untranscribed, and unsegmented raw audio signal. As said above, the scope of this simulation is restricted to the statistical learning hypothesis, where infants learn passively and uniquely by extracting statistical cues from what they hear (see Table 2). In this section, we present the model of the environment, the model of the learner, and the model of the outcome measures used in STELA.
Table 2. Non-exhaustive list of language learning assumptions for infants and whether they are included within the STELA simulation

The environment
STELA specifies the environment as raw audio speech recordings. For this to remain relevant, we need to restrict the quantity of speech within a plausible range of data. Current estimates of cumulative speech experiences by one year of age vary from around 60 hours (Cristia, Dupoux, Gurven & Stieglitz, Reference Cristia, Dupoux, Gurven and Stieglitz2019) to approximately 1,000 hours (Cristia, Reference Cristia2022). In STELA, the data comes either from open-source audiobooks with quantities varying from 50 to 3,200 hours covering the observed range. Admittedly, the infant’s language environment is different from audiobooks. On the one hand, audiobooks contain clearly articulated speech (read speech) and relatively good audio conditions, potentially facilitating learning for the model compared to the spontaneous and noisy speech available to infants (see Lavechin et al., Reference Lavechin, de Seyssel, Métais, Metze, Mohamed, Bredin, Dupoux and Cristia2022b). On the other hand, audiobooks may use larger vocabularies and more complex sentences than infants’ input, potentially putting the model in a more challenging situation than infants (Gleitman, Newport & Gleitman, Reference Gleitman, Newport and Gleitman1984). Nevertheless, this type of input is in the range of what infants could plausibly hear or overhear and is relatively easier to access in large quantities across languages than long-form recordings. Therefore, they are a good starting point, offering controlled conditions and replicability for the deployment and analysis of such simulations. Long-form recordings represent the extreme in realism that can be achieved in such simulations, but they are less accessible than audiobooks due to privacy concerns (Lavechin, de Seyssel, Gautheron, Dupoux & Cristia, Reference Lavechin, de Seyssel, Gautheron, Dupoux and Cristia2022a).
The learner
Elman (Reference Elman1990) was perhaps the first to introduce a practical implementation of a system that learns non-trivial linguistic representations by extracting regularities from language inputs: a simple recurrent neural network trained to predict future words or characters based on past ones. Since then, this idea has been expanded with more complex and larger neural networks trained on increasingly larger datasets. The resulting so-called “language models” can be viewed as models of the probability distribution of sentences and have been shown to generalise beyond the sentences in the training set (Baroni, Reference Baroni2020), reaching near human performances on many language tasks (Liu, He, Chen & Gao, Reference Liu, He, Chen and Gao2019). One major limitation of these models – as models of the infant learner – is that they only take as input words or characters, which are not entities accessible to a learning infant. However, recent breakthroughs in representation learning have made it possible to expand these models to work with raw audio inputs (Borsos et al., Reference Borsos, Marinier, Vincent, Kharitonov, Pietquin, Sharifi, Teboul, Grangier, Tagliasacchi and Zeghidour2022; Dunbar et al., Reference Dunbar, Bernard, Hamilakis, Nguyen, De Seyssel, Rozé, Rivière, Kharitonov and Dupoux2021; Lakhotia et al., Reference Lakhotia, Kharitonov, Hsu, Adi, Polyak, Bolte, Nguyen, Copet, Baevski, Mohamed and Dupoux2021). In a nutshell, these so-called ‘Generative Spoken Language Models’ replace text with their own discrete representations learnt from the audio and learn a probabilistic model of speech directly from raw inputs.
In Figure 2a, we present the model used in STELA, which has been selected from the class of Generative Spoken Language Models (Dunbar et al., Reference Dunbar, Bernard, Hamilakis, Nguyen, De Seyssel, Rozé, Rivière, Kharitonov and Dupoux2021) for its simplicity. From a high-level perspective, the learner can be described as the combination of two components, which are named according to the current practices in machine learning 1) an ‘acoustic model’ and 2) a ‘language model’Footnote 2. The acoustic model is fed with raw, continuous waveforms and trained using a form of predictive coding. It learns a vector representation for each slice of 10ms of signal by attempting to predict each of the twelve upcoming slices based on past ones, yielding a prediction over a 120ms time window. An exciting outcome of such a learning procedure is that the model learns representations that successfully abstract away from acoustic details and encode phonetic information. In STELA, we discretise these representations using clustering, yielding a discrete code each 10ms, which is passed onto the language model. This model is similar to Elman’s recurrent language model, only using an improved architecture (LSTMs) and more parameters. This model is trained to predict the next code based on past ones. Because the model’s output is not a single code, but a probability distribution over all the discrete codes, one can compute the probability of an utterance as the product of the conditional probabilities of each successive code (see Appendix A).

Figure 2. Overview of the STELA learner and outcome measures. a. (left): model of the learner; b. (right): add-on models for two types of outcome measures.
The outcome measures
Several types of outcome measures are used in infant development. Some are provided by caregivers (like the Child Development Inventory, or CDI: Fenson, Reference Fenson2007), who assess whether a word is known or produced by the child, some are linked to the production of the child as attested through transcription of naturalistic corpora (mean length of utterance such as used in Miller & Chapman, Reference Miller and Chapman1981 for instance), and some are obtained via in-lab experiments. Here we concentrate on the last type of measure. In principle, a maximally broad language learning simulation would include all linguistic and non-linguistic components (attention, memory, eye movement, etc.) and the artificial learner could just be virtually seated in a virtual lab and be subjected to the same experiments as real babies (Leibo et al., Reference Leibo, d’Autume, Zoran, Amos, Beattie, Anderson, Castañeda, Sanchez, Green and Gruslys2018). Here, STELA only simulates a subpart of infants’ linguistic competence and therefore has to specify a special add-on module to generate the equivalent of experimental outcome measures. Fortunately, experimental paradigms in infants are relatively simple and can be sorted into two main types: discrimination experiments and preference experimentsFootnote 3, yielding two types of add-on modules.
Discrimination experiments can vary in how they are conducted in the lab (ABX, AXB, AX, etc.). Still, they all rely on the ability of the learner to compute a perceptual distance between two stimuli (such as ‘bit’ versus ‘bet’). An add-on for ABX discrimination will just need to (a) extract a representation of a stimulus from the learner (typically the activation pattern of some layer) and (b) compute a distance over two representations (typically, the normalised dot product, or the angle between the vectors). In STELA (Lavechin, de Seyssel et al., Reference Lavechin, de Seyssel, Titeux, Bredin, Wisniewski, Peperkamp, Cristia and Dupoux2022c), this is used to measure phonetic knowledge through a machine ABX sound discrimination task (Schatz et al., Reference Schatz, Peddinti, Bach, Jansen, Hermansky and Dupoux2013) in which the learner has to choose two occurrences of, e.g., ‘bop’ as being closer than one occurrence of ‘bop’ and one occurrence of ‘bip’. The test is done over thousands of trials and over all possible contrasts of phonemesFootnote 4.
Preference experiments rely on the ability to compute a ‘preference’ or ‘probability’ associated with an input stimulus. Most learning algorithms learn by minimising an objective function, such as the error made in predicting the future based on the past. We can use the same objective function and apply it to test stimuli: if the stimulus is well represented or considered probable by the model, then the error should be low. Totally novel or unexpected stimuli should give a high error.
In STELA, this is used through the spot-the-word task developed in Nguyen et al. (Reference Nguyen, de Seyssel, Rozé, Rivière, Kharitonov, Baevski, Dunbar and Dupoux2020). Here, the model receives a spoken word (e.g., ‘apple’) and a spoken non-word (e.g., ‘attle’) matched for syllabic and phonotactic structure. We then look at the model’s probability of generating both words. The model is considered correct for the trial if the probability of generating the correct word is higher than the non-word. The same logic can be applied at the syntactic level using pairs of grammatical and ungrammatical sentences (i.e., ‘the brother learns’ versus ‘the brothers learns’), in which the model has to assign a higher probability to the grammatical sentence.
In the next section, we present case studies illustrating how meeting the four above-mentioned properties in a single simulation can help us make theoretical advances.
Results
Learning simulations can either be used as “proof of concept” for particular hypotheses about learning mechanisms or to offer novel predictions, never tested experimentally. Here, we focus on the first use case by addressing three long-standing controversies on language learning mechanisms as applied to the phonetic and lexical levels. In each instance, we use a design which enables us to conduct experiments that are both developmental (obtained by training the same learner on increasing quantity of speech, from 50 hours up to 3200 hours) and cross-linguistic (obtained by training and testing the models on two languages, French and English, deriving scores for the native and non-native language).
Could infants rely exclusively on statistical learning over speech inputs to bootstrap into language?
One of the major conceptual difficulties in accounting for early language acquisition is understanding how the young learner can learn several interdependent linguistic levels simultaneously and gradually. Statistical learning (Saffran et al., Reference Saffran, Aslin and Newport1996) seems a good hypothesis to address this, since it posits that infants gather information about the distribution of sounds. This would naturally yield gradual learning. As for simultaneous learning across levels, it could rest on the idea that probabilities can be gathered at several levels of descriptions simultaneously. Now, the evidence in favour of statistical learning is itself debated. Experimental evidence in infants only rests on simplified artificial languages (synthetic stimuli, small number of sounds), and it is not clear that this would translate to audio data in which speech sounds are highly variable according to phonetic context, speaker, speaking style and rate, in addition to being potentially contaminated by nonspeech background sounds.
In Figure 3, we highlight a few key results obtained by STELA when presented with raw audio from audiobooks (Lavechin, de Seyssel et al., Reference Lavechin, de Seyssel, Titeux, Bredin, Wisniewski, Peperkamp, Cristia and Dupoux2022c) and tested at the phonetic level (ABX discrimination) and lexical level (spot-the-word) using the tasks presented in a previous section. The results clearly show above-chance performance on native test stimuli and gradual and parallel learning at both phonetic and lexical levels, with the system being able to discriminate sounds better, and prefer words over nonwords more, as more data is presented to the model. This improvement is weaker when tested on a non-native language (actually, not present at all for the lexical task). Further tests (not shown in Figure 3) using a syntactic task (which is also carried out on the language model component presented in Figure 2) in which the system has to show a preference for legal versus illegal sentences revealed much weaker learning. Only the model trained on the largest quantity of speech available (that is, 3200 hours) was able to show preference on an adjective-noun order task (‘the nice rabbit’ versus ‘the rabbit nice’), with a slightly-above-chance 55% accuracy.

Figure 3. Phonetic (left) and Lexical (right) scores for native and non-native input at different quantities of training data. Phonetic score is expressed in terms of ABX accuracy, obtained by the discrete representations for native and non-native inputs. Lexical score is expressed in terms of accuracy on the spot-the-word task, on the high frequency words for native and non-native inputs. Error bars represent standard errors computed across mutually exclusive training sets. Two-way ANOVAs with factors of nativeness and training language were carried out for each quantity of speech. Significance scores indicate whether the native models are better than the non-native ones. Significance was only computed when enough data points were available to run sensical comparisons. Significance levels: na: not applicable, ns: not significant, * p<.05, **, p<.001, *** p<.0001. Figure taken from Lavechin, de Seyssel et al. (Reference Lavechin, de Seyssel, Titeux, Bredin, Wisniewski, Peperkamp, Cristia and Dupoux2022c).
In brief, the STELA simulation suggests that raw speech input only, combined with statistical learning, and more precisely predictive learning, is: 1) sufficient to bootstrap the phonetic, the lexical and only very weakly the syntactic levels; 2) sufficient to reproduce the gradual and overlapping developmental trajectory observed in infants at the phonetic and lexical levelsFootnote 5. It is the first time a simulation reproduces the gradual and multilevel learning observed in infants from audio signals, at least when audiobooks are used as input.
Do infants learn and perceive language in terms of linguistic categories?
A second debate concerns whether linguistic categories (phones, words) are necessary building blocks in early language acquisition. On the one hand, linguistic theories describe adult competence in terms of such categories. On the other hand, these categories are language-dependent and therefore need to be learned by infants, who have only access to continuous sensory information at the beginning. Schatz et al. (Reference Schatz, Feldman, Goldwater, Cao and Dupoux2021) recently proposed a learning simulation of phonetic learning from raw audio signals based on a probabilistic model using Mixtures of Gaussians. While reproducing observed native advantage effects in phonetic discrimination between Japanese and English phonemes, the learner used in this simulation did not learn phonemes or units that could be described linguistically. These results suggest that phonetic learning can occur without the existence of phonetic categories.
The STELA simulation reproduces this conclusion using a totally different learning algorithm, supporting once again the idea that phonetic categories are not necessary for phonetic learning (see also Feldman, Goldwater, Dupoux & Schatz, Reference Feldman, Goldwater, Dupoux and Schatz2022). To dive further into this, it is interesting to reflect on how the acoustic model behaves during training concerning the duration of the learnt representations. Pre-exposure (i.e., before the model has received any input) speech is represented within the model as a string of random units. As the model receives speech, it learns to structure this discrete representation: discrete units start repeating themselves, and the sound discrimination accuracy increases. An analysis of the duration of the discrete learnt units revealed that the latter are too short to correspond to phones (43 ms for the learnt units, versus 90 ms for a typical English phone), similarly to what has been found in Schatz et al. (Reference Schatz, Feldman, Goldwater, Cao and Dupoux2021). An example of how the discovered units compare to the original phones is presented in Figure 4, where units are clearly shorter than the phones. More surprisingly, the more speech the model receives, the lower the duration of the discrete units. It is essential to note that no constraint is applied to the duration of these units. The model could, in principle, converge to phone-length discrete units, but does no such thing. In other words, the model does not converge to phone-like representations, yet it can still pass phonetic, lexical and, to a certain extent, syntactic tests for which phoneme representations are still often considered a prerequisiteFootnote 6.

Figure 4. An example spectrogram of an English utterance, along with the corresponding phonemes (top tier) and the units discovered by a STELA model trained on 3200 hours of English. Transcription: “The valley was filled”
In STELA, it is also possible to ask the question of linguistic categories at higher linguistic levels. Surprisingly, even though the model can distinguish words from nonwords, we could not find an indication that the model represents words as such, or would represent the boundaries between words. Yet, the continuous activations found in the hidden layers of the recurrent model contained some approximate linguistic information, as a trained linear classifier was able to classify test words into function versus content words or verb versus adjective/adverb versus noun better than chance, and the separation increased with more input data. These results show that, although the model does not learn discrete and interpretable linguistic categories internally, linguistic information increasingly structures the learnt representations (for more in-depth analyses of the types of units yielded by such models, see de Seyssel, Lavechin, Adi, Dupoux & Wisniewski, Reference de Seyssel, Lavechin, Adi, Dupoux and Wisniewski2022; Nguyen, Sagot & Dupoux, Reference Nguyen, Sagot and Dupoux2022; Sicherman & Adi, Reference Sicherman and Adi2023). Thus, our simulation promotes the view that linguistic categories could be the end product of learning, not their prerequisite.
Can statistical learning alone account for early phonetic acquisition from ecological audio?
One of the largest controversies in language learning orbits around the poverty of the stimulus argument (Chomsky, Reference Chomsky1980). This argument states that the input available to infants is too scarce and too noisy to warrant language learning through a general-purpose learning algorithm. Therefore, only a learning algorithm with strong inductive biases would be able to reproduce human language learning. For a long time, this controversy has remained unsolved for lack of learning algorithms that can work even on rather simple inputs. With STELA, at last, we are able to address this controversy, at the level of phonetic and lexical learning. The preceding sections show that a relatively general-purpose system based on predictive coding is able to learn at both levels when fed with audiobooks, but this kind of input may not be realistic enough to correspond to the learning problem faced by infants. Indeed, the audio environment of infants, first of all, contains a majority of non-speech noises, and the little amount of speech that is heard may be under-articulated, reverberated and absorbed by the surrounding obstacles in the environment, and overlaid with various background noises. Could the relatively generic learner of STELA handle such noisy inputs?
One way in which one can revisit this simplifying assumption is by using child-centred long-form recordings, i.e., daylong recordings collected via child-worn microphones as people go about their everyday activities. Lavechin et al. (Reference Lavechin, de Seyssel, Métais, Metze, Mohamed, Bredin, Dupoux and Cristia2022b) exposed the STELA contrastive predictive coding algorithm to such ecological recordings of children’s language experiences and found that the discrimination gap between the native and the non-native models vanishes. It is only when supplemented with inductive biases in the form of filtering and augmentation mechanisms (restricting learning to speech parts, taking into account speaker invariance, and making the system resistant to reverberant noise) that the model could exhibit some form of perceptual attunement again (see Figure 5). In addition to this result, Lavechin et al. (Reference Lavechin, de Seyssel, Métais, Metze, Mohamed, Bredin, Dupoux and Cristia2022b) showed that, even in the presence of inductive biases, the learning speed of the learner was still negatively impacted by the presence of additive noise and reverberation in the training set and that this loss could not be recovered by adding more data.

Figure 5. Panel (a) shows native discrimination accuracy, as measured in an ABX discrimination task, obtained by American English and Metropolitan French CPC models (both models are evaluated on phonemes of their native languages). Panel (b) shows native advantage, computed as the average relative difference of the native model and the non-native model, obtained by the same pairs of models (a positive native advantage indicates that the native model is better at discriminating native sounds than the non-native model). Figure adapted from Lavechin et al. (Reference Lavechin, de Seyssel, Métais, Metze, Mohamed, Bredin, Dupoux and Cristia2022b).
Given the sparse, variable and noisy nature of the speech overheard by children, this simulation suggests that a statistical learning algorithm alone might not be sufficient to account for early phonetic acquisition. Given that linguistic input represents a small fraction of the audio environment of the child, and that even speech is itself overlapped with non-speech signals, any statistical learning algorithm will devote its resources to discovering the structure of the entire audio, thereby failing to capture the structure of speech sounds.
The three types of inductive biases that were introduced in this study are plausible and independently motivated by experimental evidence in infants: infants show an early preference for attending to speech versus non-speech sounds, and it is plausible that they would learn preferentially on such sounds. In addition, there is evidence that infants distinguish speakers and associate speakers to their voices at an early age; it is therefore plausible that their learning algorithm would be speaker-specific. Finally, the human learner has the benefit of an auditory system that has been fine-tuned by millions of years of evolution to accurately perceive sound sources in complex auditory scenes, and it is plausible that learning operates not on raw sensory data, but rather on sensory streams organised according to source and therefore resist additive noise and reverberation. It is important to note, however, that the inductive biases we implemented are not sufficient; as subsequent testing at the lexical level showed that, even with them, no lexical learning is evidenced in STELA when fed with long-form recordings. This indicates that, as far as phonetic and lexical learning is concerned, some form of poverty of the stimulus argument is valid, and that generic learning algorithms (at least the ones we tested) need to be supplemented with strong inductive biases.
In brief
We showed that realistic learning simulations could help address some of the key controversies within language acquisition. For instance, STELA shows that statistical learning can be sufficient to reproduce some key findings in infants (phonetic attunement, preference for words over nonwords) from raw audio inputs in the total absence of multimodal grounding or social feedback. It also shows that such learning patterns can arise in the total absence of interpretable linguistic categories. However, it also shows that it has to be supplemented with inductive biases in order to deal with the noise present in naturalistic recordings that are representative of what infants really hear. Of course, these findings are only theoretical results: and, as such, can demonstrate that mechanism A is sufficient (or not needed) to observe outcome B. Whether infants really use similar mechanisms remains to be further established.
What lies ahead?
So far, we have presented evidence that learning simulations, when scaled to incorporate realistic inputs and to model more than one linguistic level, can address some long-standing controversies regarding learning mechanisms in infants. However, our demonstration was limited to testing one hypothetical learning mechanism: statistical learning, and a particularly narrow version of it that is restricted to audio inputs. While STELA could perhaps be counted as the first successful learning simulation of early language acquisition in infants when trained on audiobook data, it struggles to learn with ecological data, even with inductive biases. This suggests two directions of future work: (1) improving STELA with more inductive biases; (2) build a model that incorporates other learning mechanisms (e.g., cross-situational learning, social feedback, etc.). Either way, there is work to be done for both the psycholinguistic and AI communities, which we review below.
Guidelines for psycholinguistics and AI communities
Modelling the environment
Concerning the learning environment, we believe that one challenge that lies ahead consists of collecting and characterising more ecological data. As demonstrated above, results are quite different when models are presented with audiobooks or long-form recordings. We foresee that moving towards more naturalistic training sets will increase the impact and relevance of language learning models.
As data is the crux of any language learning simulation, we believe constant efforts must be put in place to collect and share ecological learning environments. On this front, we would like to highlight important initiatives such as the privacy-preserving sharing platforms for long-form audio recordings (VanDam et al., Reference VanDam, Warlaumont, Bergelson, Cristia, Soderstrom, De Palma and MacWhinney2016) or video data (Simon, Gordon, Steiger & Gilmore, Reference Simon, Gordon, Steiger and Gilmore2015), and the DARCLE (DAylong Recordings of Children’s Language Environments, DARCLE.org, n.d.) community. We believe these initiatives must become standard practices as they can transform our understanding of language development by enabling incremental and reproducible science and fueling language learning simulations with realistic data.
In addition, most of what we know concerning language development comes from Western, Educated, Industrialised, Rich, and Democratic (WEIRD) populations (Henrich, Heine & Norenzayan, Reference Henrich, Heine and Norenzayan2010; Scaff, Reference Scaff2019), and this bias toward WEIRD populations reflects in the type of data computational modellers have access to. Current large-scale audio datasets – whether they contain child-centred recordings or audiobooks – are primarily collected in American English (Kearns, Reference Kearns2014; VanDam et al., Reference VanDam, Warlaumont, Bergelson, Cristia, Soderstrom, De Palma and MacWhinney2016). We believe this represents a significant limitation for language realistic learning simulations that can – and should – be run considering diverse socioeconomic and cultural backgrounds. Doing so would help us extract and understand universal constants taking place in the course of language development.
Finally, another challenge is to enrich the nature of the data provided to the learner by incorporating ecologically collected multimodal data, in order to address the importance of cross-situational learning in real life. Also, quantifying the nature and prevalence of social feedback (some of which is nonverbal) is very important as a first step towards building interactive models of the learning environment (Tsuji et al., Reference Tsuji, Cristia and Dupoux2021)
Modelling the learner
One key challenge on the learner side relates to the quantity of data needed to reach a certain level of linguistic performance. Today’s most performant text-based language models are trained on roughly one thousand times the amount of linguistic input afforded to a typical child (Warstadt & Bowman, Reference Warstadt and Bowman2022). Therefore, current language models are confronted with a data efficiency problem that is doomed to be even more critical when learning from the raw audio, where other sources of variations have to be considered (speaker’s identity, speech rate, acoustic conditions, etc.). Future research should focus on implementing algorithms that can reach human-like performances with the same input data available to an infant – that is, that can map the input and the output measures to those of the modelled human.
Related to this question is the challenge of improving perceptual constancy (on the difficulty of obtaining speaker-invariant representations, see van Niekerk, Nortje, Baas & Kamper, Reference Van Niekerk, Nortje, Baas and Kamper2021) for state-of-the-art learners of audio representations. As stated above, speech sounds, words and sentences can be realised in numerous ways depending on the speaker’s identity, the speech rate, or the acoustic environment. This problem is bypassed when considering the text as input, although text brings other simplifying assumptions irrelevant in the context of language acquisition. We believe normalising audio representations along all dimensions irrelevant to language represents one crucial step to bridging the performance gap between audio-based and text-based language models.
Finally, it is important to develop learners that go beyond the statistical learning hypothesis (Erickson & Thiessen, Reference Erickson and Thiessen2015; Romberg & Saffran, Reference Romberg and Saffran2010; Saffran et al., Reference Saffran, Aslin and Newport1996). Comparing this hypothesis with alternative ones (cross-modal grounding, social constructivism, etc.) will require developing learners with other learning mechanisms to play a more critical role. Reinforcement learning may, for instance, integrate social and interactive rewards, whereas supervised learning may integrate corrective feedback from caregivers. Admittedly, integrating multiple learning mechanisms and modalities in a single learning simulation requires collaborative work across fields, as has been analysed in Tsuji et al., Reference Tsuji, Cristia and Dupoux2021.
Modelling the outcome measures
The ultimate test of any language learning simulation is the comparison to humans. Dupoux (Reference Dupoux2018) proposed to aim at cognitive indistinguishability in that setup: “a human and machine are cognitively indistinguishable with respect to a given set of cognitive tests when they yield numerically overlapping results when run on these tests”. This critically assumes that cognitive tests that can be applied to the infant and the learner alike are available.
This is not an easy task, and much more can be done in this regard. As discussed above, outcome measures come in several flavours. Laboratory experiments require infants to cooperate with the setting, which is not a given. As a result, the outcome measures are loaded with non-linguistic factors. Infants’ performance depends on various factors that most simulations do not currently consider (e.g., memory or fatigue). This problem is even worse when considering babies for which measures are noisier (but see Blandón, Cristia & Räsänen, Reference Blandón, Cristia and Räsänen2021, who propose evaluations against meta-analyses). This measurement noise needs to be integrated into the outcome model before direct comparisons between infants and simulations can be done. We refer to this problem as the calibration problem. Some outcome measures are more ecological, and extracted directly from the speech of infants. This requires a learner that can also speak, which has not yet been developed. Other measures, like the CDI, depend on the judgement of a caretaker, which here again needs to be modelled specifically. Ultimately, the calibration of measures extracted from the machine to those extracted from the human (or vice versa) will have to be dealt with one measure at a time.
Similarly to HomeBank (VanDam et al., Reference VanDam, Warlaumont, Bergelson, Cristia, Soderstrom, De Palma and MacWhinney2016) or Databrary (Simon et al., Reference Simon, Gordon, Steiger and Gilmore2015), we believe both the AI and the psycholinguistics communities would greatly benefit from a privacy-preserving platform to share stimuli – as well as responses – used in psychology experiments. Such a platform would allow researchers to 1) re-use stimuli as new hypotheses arise; 2) revisit stimuli – or responses – to control for confounding factors, or in the context of meta-analytic studies; and 3) create benchmarks that aim at comparing humans and machines. Concerning the last point, we believe there are still too few works that directly compare human and machine performance on a common benchmark (but see Millet & Dunbar, Reference Millet and Dunbar2020 for a sound discrimination capability study). A stimuli-sharing platform would accelerate collaborative works across the AI and the psycholinguistics community and could also extend to other domains of psychology (including decision-making or social experiments, for instance).
Conclusion
The article’s main aim was to provide an extensive description of an emerging theoretical approach in the field of language acquisition: learning simulations, and especially realistic and broad-scope learning simulations. We proposed four criteria we believe are essential for such a simulation to address the current theory crisis and act as a cumulative and unifying theory of language acquisition. We then presented STELA, one such simulation, and showed how it could help shed light on long-standing controversies. Realistic learning simulations can – and should – integrate the large body of knowledge acquired by the different approaches that comprise the field of language acquisition. Such realistic learning simulations are by no means replacements for other approaches, as all are needed to reach a unified theoretical landscape. Indeed, verbal frameworks can inspire the design of artificial learners, computational models can provide hands-on algorithms, statistical models can exhibit relationships between input and learning outcomes, and corpus studies help describe the characteristics of language environments. Of course, there remain challenges ahead of us to build more complete realistic learning simulations, and we dedicated the last section to address some of them.
Competing interest declaration
The authors declare none.
Acknowledgements
We are particularly thankful to Dr. Daniel Swingley, Dr. Michael Frank and Dr. Abdellah Fourtassi for helpful insights on previous versions of the manuscript. We are grateful to CoML members for helpful discussions. All errors remain our own. E.D., in his academic role (EHESS), acknowledges funding from Agence Nationale de la Recherche (ANR-17-EURE-0017 Frontcog, ANR-10-IDEX-0001-02 PSL*, ANR-19-P3IA-0001 PRAIRIE 3IA Institute), a grant from CIFAR (Learning in Machines and Brains), and the HPC resources from GENCI-IDRIS (Grant 2020-AD011011829). M.S. acknowledges PhD funding from Agence de l’Innovation de Défense. M.S and M.L. contributed equally to this work. Authorship order was decided by a coin flip.
Appendix A: How to derive a probability from a Language Model?
Head-turn preference experiments (Nelson et al., 1995) provide a wealth of results regarding the type of stimuli infants prefer to listen to. However, mechanisms underlying this preference remain unobservable. Computational modelling provides complementary information by assessing hypotheses about how statistical information might be used to exhibit similar preference patterns as those exhibited by infants, or what underlying information processing problem is being solved. Language models, and probabilistic models in general, offer a natural way to extract a preference measure from an artificial learner: a stimulus A is preferred to a stimulus B if A is more probable than B.
But how does one compute the probability of a stimulus from a Language Model? First, the waveform goes through the Acoustic Model which returns a discrete representation of the audio:
$ {q}_1,{q}_2\dots, {q}_T $
. Then, the Language Model, which has been trained to predict the next discrete unit of a sequence given its past context, assigns a probability to the discrete sequence using the following chain-rule:

We compute the logarithm of the resulting probability which has the effect of increasing the difference between probabilities assigned to a minimal pair of stimuli (e.g., a word and a non-word that differ in a single phoneme). The logarithm is then normalised by the length of the input stimuli to enforce the model to not show a constant preference for the longest stimuli.
Appendix B: Overview of the learner used in STELA
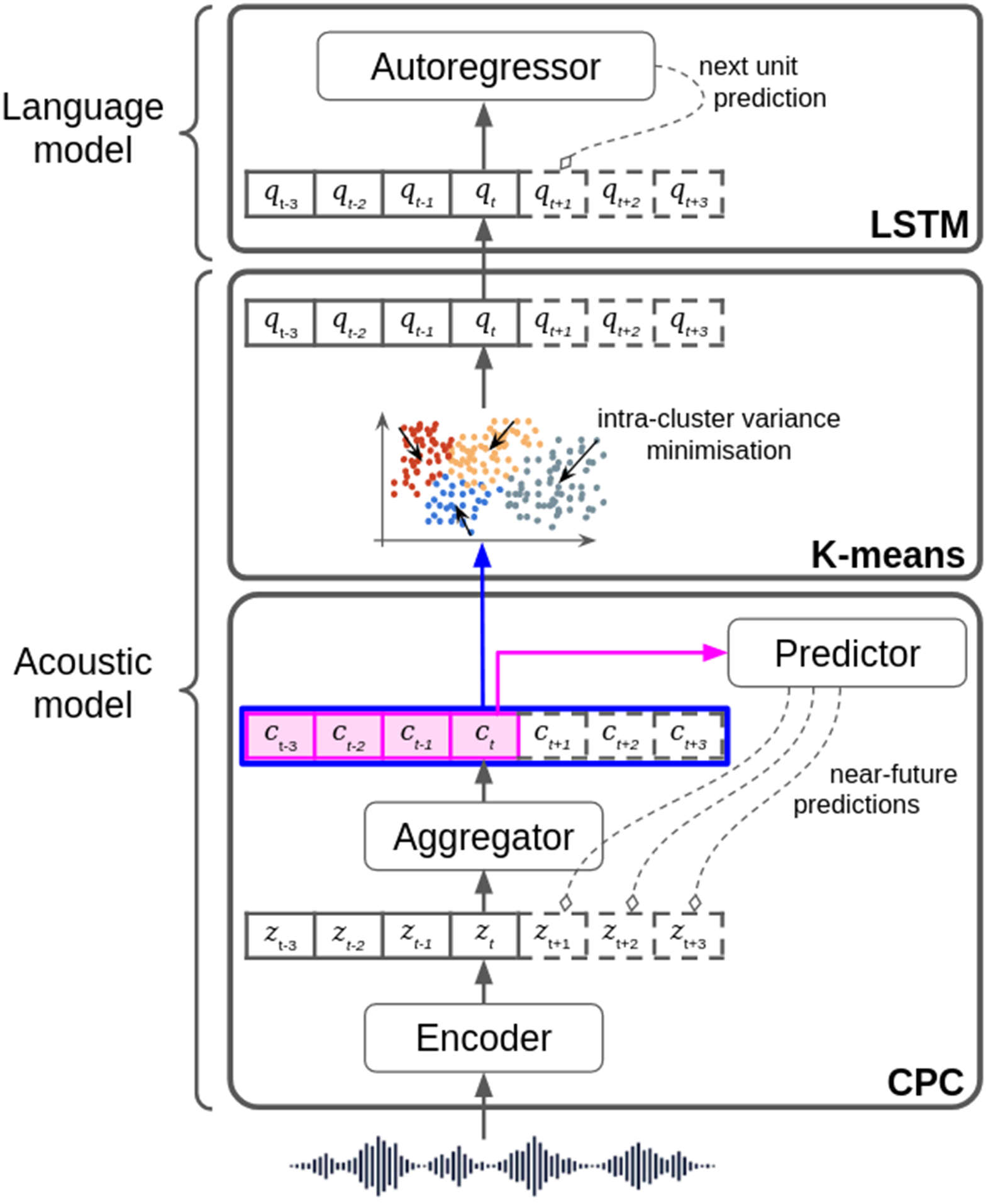
Figure A1. Model of the learner used in STELA. The Acoustic model is composed of a convolutional encoder which delivers a vector of continuous values z t every 10ms. This is sent to a recurrent network aggregator that integrates context and delivers vectors with the same time step. Contrastive Predictive Coding is trained to predict the outputs of the encoder in the near-future (up to 120 ms). The output of the aggregator is sent to a K-means algorithm that discretise the continuous representations c t into q t. Then, a language model (long-short term memory (LSTM) network) is trained to predict the next q t unit based on past ones.