1 Introduction
People often make decisions on the basis of less information than might be available. One way in which researchers have studied this phenomenon is through the concept of maximizing, originally introduced by Reference SimonSimon (1955, Reference Simon1956, Reference Simon1957) and subsequently elaborated upon and popularized by Reference Schwartz, Ward, Monterosso, Lyubomirsky, White and LehmanSchwartz, et.al (2002). Reference Schwartz, Ward, Monterosso, Lyubomirsky, White and LehmanSchwartz et al. (2002) label people who extend their search to identify as many alternatives as possible and attempt to find the “best” one as maximizers. This contrasts with satisficers, who search until they find an alternative that is regarded as good enough.
Research on maximizing has proliferated in recent years. Yet, despite the manifest importance of judgments and decisions in work organizations (Reference Dalal, Bonaccio, Highhouse, Ilgen, Mohammed and SlaughterDalal et al., 2010), no extant research has examined this construct within an organizational setting. In the present research, we examine the extent to which maximizing predicts job satisfaction and job performance judgments—and whether these relationships differ as a function of the way in which maximizing is measured. In so doing, the present research attempts to answer several calls for researchers in the disciplines of Judgment and Decision Making (JDM) and organizational psychology/behavior to assess the extent to which theories and constructs from each discipline can enrich the other discipline (e.g., Reference Dalal, Bonaccio, Highhouse, Ilgen, Mohammed and SlaughterDalal et al., 2010; Reference Highhouse, Anderson, Ones, Sinangil and ViswesvaranHighhouse, 2001; Reference Moore and FlynnMoore & Flynn, 2008; Reference NaylorNaylor, 1984).
1.1 Maximizing
Reference SimonSimon (1955, 1956, 1957) proposed a theory that accommodates the typical real-world situation of imperfect knowledge about possible alternatives during the decision-making process. According to Simon, because it is often not possible to determine the objectively “best” alternative, humans seek to “satisfice”. That is, when individuals make choices, they explore alternatives until they find one that is good enough to meet their standards.
In this search for “good enough”, it may or may not be helpful to have a large number of alternatives. Although it may seem desirable to have more, rather than fewer, alternatives from which to choose, more alternatives may make the choice more difficult (Reference SchwartzSchwartz, 2000). As the number of alternatives increases, so does the difficulty of adequately evaluating every alternative and comparing it to every other alternative—and, in turn, so do decision regret and doubts regarding whether the “best” alternative has indeed been chosen (Reference Parker, Bruine de Bruin and FischhoffParker et al. ,2007; Reference Schwartz, Ward, Monterosso, Lyubomirsky, White and LehmanSchwartz et al., 2002). Extrapolating from decision situations involving more versus fewer alternatives to individual differences in preferences for more versus fewer alternatives, Reference Schwartz, Ward, Monterosso, Lyubomirsky, White and LehmanSchwartz et al. (2002) argued that the type of person who characteristically seeks out a large number of alternatives and then attempts to choose the best one should be more unhappy with the decision-making process and with life in general than the type of person who characteristically seeks out a smaller number of alternatives and then chooses one deemed to simply be good enough. The extent of empirical support for this assertion, however, appears to depend on the way in which individual differences in maximizing are measured.
1.2 Inconsistencies in maximizing research: Sensitivity to choice of scale
To assess whether an individual is a maximizer or a satisficer, Reference Schwartz, Ward, Monterosso, Lyubomirsky, White and LehmanSchwartz et al. (2002) developed a measure called the Maximization Scale (MS), composed of 13 items. These items focus on behavioral examples of maximizing and assess the accompanying high standards for finding the ostensibly best alternative. Using the MS, Reference Schwartz, Ward, Monterosso, Lyubomirsky, White and LehmanSchwartz et al. (2002) found support for their contention that maximizers (individuals who score high on the MS) experience higher levels of regret and depression, accompanied by lower levels of life satisfaction and optimism, than satisficers (individuals who score low on the MS). The general finding that the tendency to maximize is related to lower life satisfaction sparked work by several other researchers who sought to investigate the robustness of this relationship and the reasons behind it. Many studies that used the MS to measure maximization have replicated this relationship (Reference PolmanPolman, 2010; Reference Purvis, Howell and IyerPurvis et al., 2011). For example, a study of college students and their job searches during their final semester of school (Reference Iyengar, Wells and SchwartzIyengar et al., 2006) revealed that maximizers were more dissatisfied than satisficers with their job search outcomes.
Yet, the contention that maximizing is associated with lower life satisfaction has not gone unchallenged. Reference Diab, Gillespie and HighhouseDiab et al. (2008) argued that conceptual and psychometric problems associated with the MS called into question relationships obtained between the MS and life satisfaction. First, Diab et al. contended that the MS items are problematic because several items diverge from the definition of maximizing. For example, one item assesses whether the individual sometimes fantasizes about living a different life, which does not necessarily reflect a tendency to “pursue the identification of the optimal alternative” (Reference Diab, Gillespie and HighhouseDiab et al., 2008, p. 365). Furthermore, Diab et al. contended that the MS is multidimensional. Specifically, these authors contended that some items on the MS assess attitudes towards general decision-making (e.g., “I never settle for second best”) whereas others assess specific behaviors (e.g., “When I watch TV, I channel surf, often scanning through the available options even while attempting to watch one program”). Diab et al. moreover contended that even Reference Schwartz, Ward, Monterosso, Lyubomirsky, White and LehmanSchwartz et al.’s (2002) own data reveal that the MS is multidimensional. Finally, Diab et al. contended that the MS lacks both internal consistency reliability and construct validity.
To resolve these issues, Reference Diab, Gillespie and HighhouseDiab et al. (2008) developed the Maximizing Tendency Scale (MTS), a nine-item scale (three items of which were taken from the original MS) that not only exhibited higher reliability than the original MS but also improved prediction of scores on situational dilemmas that contrasted “pragmatic satisficing [with] more time- or labor-intensive options that would provide more complete information for optimizing the quality of the decision” (p. 366)—in other words, improved construct validity. Importantly, in their study comparing results from the original MS with those from the newly developed MTS, Diab et al. found that the negative relationship between maximizing and life satisfaction was greatly attenuated when the MTS, rather than the MS, was used. A subsequent study using the MTS (Reference Rim, Turner, Betz and NygrenRim et al., 2011) even found a moderately positive relationship between maximizing and life satisfaction. Thus, the obtained relationship between maximizing and life satisfaction appears to depend on the scale used to measure maximizing.
Reference Diab, Gillespie and HighhouseDiab et al. (2008) were not the only researchers to raise concerns about the MS. Reference Nenkov, Morrin, Ward, Schwartz and HullandNenkov, Morrin, Ward, Schwartz, and Hulland (2008) sought to examine the reliability, factor structure, and validity of this scale. Nenkov et al. opted both to drop some of the original items from the MS (recommending that only 6 of the original items be retained) and to replace the original unidimensional conceptualization with a multidimensional (specifically, three-dimensional) conceptualization. The alternative search dimension assesses the maximizer’s tendency to continue searching for the elusive “best” alternative despite the presence of acceptable alternatives, the decision difficulty dimension assesses the maximizer’s indecisive behavior during the decision-making process, and the high standards dimension assesses the maximizer’s attitude toward his or her decision alternatives such that only the best alternative will suffice.
Reference Nenkov, Morrin, Ward, Schwartz and HullandNenkov et al. (2008) contended that these dimensions are separate aspects of maximizing and, therefore, should predict outcomes differentially. For example, consistent with Reference Schwartz, Ward, Monterosso, Lyubomirsky, White and LehmanSchwartz et al.’s (2002) original work, Nenkov et al. found that maximizing, when treated as a composite score, is negatively related to life satisfaction, subjective happiness, optimism, and (the absence of) depression. However, when they disaggregated maximizing into the three dimensions, Nenkov et al. found that the relationships between maximizing and these other constructs were driven by the decision-difficulty dimension and, to a lesser extent, the alternative-search dimension. In the authors’ own words: “Our results…suggest that the real problem with maximizing is not having high standards, but rather, having high standards in a world of limitless choice alternatives that demands extensive search and creates decision difficulty” (Reference Nenkov, Morrin, Ward, Schwartz and HullandNenkov et al., 2008, p. 384).
It is also interesting to compare the Reference Nenkov, Morrin, Ward, Schwartz and HullandNenkov et al. (2008) scale to Reference Schwartz, Ward, Monterosso, Lyubomirsky, White and LehmanSchwartz et al.’s (2002) MS and Reference Diab, Gillespie and HighhouseDiab et al.’s (2008) MTS. Nenkov et al.’s high standards dimension, which was unrelated to constructs such as life satisfaction, was composed of items from the MS that were retained in the MTS. In contrast, Nenkov et al.’s decision-difficulty and alternative-search dimensions, which were primarily responsible for the negative relationship between maximizing and constructs such as life satisfaction, were composed of items from the MS that were excluded from the MTS. Differences in findings across the MS versus MTS, in other words, are likely to translate into differences in findings across the dimensions of the Nenkov et al. scale (i.e., the decision-difficulty and alternative-search dimensions versus the high-standards dimension). We therefore begin our discussion of job attitudes by comparing the MS and MTS. Subsequently, we reintroduce the Nenkov et al. scale into the discussion.
1.3 Evaluating maximizing as a predictor of job attitudes
Bivariate relationships.
Although maximizing has been a popular construct in the recent JDM literature, it has not yet been examined in the organizational literature. However, several of the observed correlates of maximizing are closely related to job attitudes or behavioral intentions examined in the organizational literature. For example, perhaps the best organizational analog of life satisfaction is job satisfaction; indeed, the two constructs are moderately to strongly intercorrelated, with observed (uncorrected) and corrected (for predictor and criterion unreliability) meta-analytic correlations of 0.40 and 0.48, respectively (Reference Bowling, Eschleman and WangBowling et al., 2010). As another example, an organizational analog of switching telephone companies (which maximizers have been found to do more often than satisficers; Reference LaiLai, 2011) is switching jobs—or, to use a construct frequently studied in the organizational literature, the intention to quit one’s current job in favor of another.
A considerable body of organizational research (e.g., Reference Arvey, Bouchard, Segal and AbrahamArvey et al., 1989; Reference Bowling, Eschleman and WangBowling et al., 2010; Reference Judge, Heller and MountJudge et al., 2002; Reference Staw, Bell and ClausenStaw et al., 1986; Reference Staw and RossStaw & Ross, 1985) has demonstrated a robust dispositional, even genetic, basis for job satisfaction. Although less organizational research has investigated a dispositional basis for switching jobs, there does appear to be empirical support for the idea of a “hobo syndrome”—a dispositional wanderlust that leads some individuals to repeatedly switch jobs (Reference GhiselliGhiselli, 1974; Reference Judge and WatanabeJudge & Watanabe, 1995; Reference WooWoo, 2011). In addition, virtually all extant theories include job satisfaction among the most important predictors of intentions to quit (e.g., Reference Tett and MeyerTett & Meyer, 1993; Reference SalgadoSalgado, 2002; Reference Donnelly and QuirinDonnelly & Quirin, 2006; for a review, see Reference Dalal, Weiner, Schmitt and HighhouseDalal, 2013). Consequently there is likely to also be an indirect dispositional basis for intentions to quit, one that operates through job satisfaction.
Thus, maximizing, which is conceptualized as a dispositional decision-making style, may correlate with job satisfaction and intentions to quit. However, extrapolating from the existing JDM research, we would expect maximizing to be more strongly negatively correlated with job satisfaction and more strongly positively correlated with intentions to quit when maximizing is measured via the MS rather than the MTS.
Hypothesis 1: Maximizing will have a stronger negative relationship with job satisfaction and a stronger positive relationship with intentions to quit when maximizing is measured using the Maximizing Scale (MS) than when it is measured using the Maximizing Tendency Scale (MTS).
Relative importance.
In addition to examining the bivariate relationships between maximizing and both job satisfaction and intentions to quit, it is important to examine the relative importance of maximizing vis-à-vis other dispositional individual-differences constructs that have traditionally been used by organizational researchers to predict job satisfaction and intention to quit. If maximizing is found to be more important than these traditional dispositional individual-differences constructs, organizational researchers would be well advised to import this JDM construct.
Among the dispositional individual-differences variables typically used by organizational researchers to predict job satisfaction and intention to quit are the personality factors of neuroticism and conscientiousness (Reference Judge, Heller and MountJudge et al., 2002). Individuals scoring high on neuroticism tend to view their life experiences in general, and their job experiences in particular, in a more negative light than those who are emotionally stable (Reference Judge, Heller and MountJudge et al., 2002). Neuroticism and associated traits (e.g., irritability, insecurity, and emotionality; Reference SalgadoSalgado, 2002) often contribute to difficulties in workplace relationships and productivity, thereby adversely affecting job satisfaction. Additionally, high conscientiousness contributes to job satisfaction because conscientious individuals tend to be more dependable and detail-oriented at work (Reference Judge, Heller and MountJudge et al., 2002). These traits contribute to the tendency to meet deadlines and produce higher quality work, which often result in both formal and informal rewards in the workplace. Conscientiousness “represents a general work involvement tendency and thus leads to a greater likelihood of obtaining satisfying work rewards” (e.g., promotions, positive feedback; Reference Judge, Heller and MountJudge et al., 2002, p. 531). Ultimately, these individuals have a more positive attitude towards their job and organization (Reference Judge, Heller and MountJudge et al., 2002).
Core self-evaluations (CSE), or “bottom-line evaluations that individuals hold about themselves” (Reference Judge and BonoJudge & Bono, 2001), have also been shown to predict job satisfaction (Reference Wu and GriffinWu & Griffin, 2012; Reference Judge, Bono and LockeJudge et al., 2000; Reference Judge and BonoJudge & Bono, 2001). CSE encompasses four specific traits: self-esteem, locus of control, generalized self-efficacy, and emotional stability (the opposite pole of neuroticism). According to Reference Judge, Heller and MountJudge et al. (2002), individuals high in CSE generally have better moods and may be happier at work “because they are more likely to achieve satisfying results at work” (p. 536). Additionally, Reference Wu and GriffinWu and Griffin (2012) argued that individuals scoring high on CSE engage in more self-verification and self-enhancement in the workplace. That is, they are more likely to seek out positive feedback from the workplace environment and focus on the positive aspects of their jobs to “confirm their positive self-view” (p. 331) and “inform judgments about their self-worth” (p. 339). In focusing on the positive aspects of the job, individuals high in CSE report higher job satisfaction.
Thus, the two traditional dispositional individual-differences predictors of job satisfaction considered in the present research are CSE (which incorporates neuroticism) and conscientiousness. Moreover, because job satisfaction is an important predictor of intentions to quit, we examine these two individual differences constructs as predictors (albeit more distal) of intentions to quit as well.
How is maximizing likely to compare in relative importance to these traditional predictors? Once again, the results are likely to depend on the scale with which maximizing is measured. Based on the JDM literature and our own Hypothesis 1, we do not expect maximizing to be meaningfully related to job satisfaction or intentions to quit when maximizing is measured using the MTS. Therefore, it seems likely that the traditional predictors of job satisfaction and intentions to quit will outperform maximizing when maximizing is measured using the MTS.
Hypothesis 2: When maximizing is measured using the Maximizing Tendency Scale (MTS), the relative importance of maximizing will be lower than that of core self-evaluations and conscientiousness when predicting job satisfaction and intentions to quit.
A priori, however, it is unclear whether the same pattern of relative importance will also hold true when maximizing is measured using the MS. We therefore examine this issue in an exploratory manner, using research questions rather than formal hypotheses.
Research question 1: When maximizing is measured using the Maximization Scale (MS), what is the relative importance of maximizing vis-à-vis core self-evaluations and conscientiousness when predicting job satisfaction and intentions to quit?
The Reference Nenkov, Morrin, Ward, Schwartz and HullandNenkov et al. (2008) scale.
The aforementioned hypotheses and research questions inform our speculation regarding the functioning of the Reference Nenkov, Morrin, Ward, Schwartz and HullandNenkov et al. (2008) scale. As noted previously, the decision difficulty and (to a lesser extent) alternative-search dimensions of this scale are expected to operate like the MS, whereas the high-standards dimension is expected to operate like the MTS. In other words, job satisfaction should be more strongly negatively related, and intentions to quit more strongly positively related, to the decision difficulty and alternative-search dimensions of maximizing than to the high-standards dimension; and when predicting job satisfaction and intentions to quit, the relative importance of maximizing should be lower than that of core self-evaluations and conscientiousness if maximizing is operationalized as the high-standards dimension.
1.4 Evaluating maximizing as a predictor of job performance
Although the main focus of JDM research on maximizing has been on the construct’s putative impact on life satisfaction (and similar constructs), some research has also examined the impact of maximizing on decision-making performance. After all, Reference Schwartz, Ward, Monterosso, Lyubomirsky, White and LehmanSchwartz et al.’s (2002) contention that maximizing is maladaptive would be particularly compelling if maximizing were found to exert deleterious effects on not just life satisfaction but also decision-making performance.
Some extant studies indeed suggest that maximizers make worse decisions than satisficers. For instance, one study examined how maximizing, as measured by the MS, affected performance in a gambling task (Reference PolmanPolman, 2010). This computerized game asked participants to select from four different decks of cards to win money. Maximizers were more likely to alternate among decks than satisficers, and were therefore more likely to pull from “bad” decks than satisficers. Similarly, Reference Bruine de Bruin, Parker and FischhoffBruine de Bruin, Parker, and Fischoff (2007) also measured maximizing via the MS and found that maximizers reported experiencing more negative decision outcomes than their satisficing counterparts. The negative decision outcomes studied by Bruine de Bruin et al. ranged in severity from relatively minor (e.g., ruining clothes in the laundry, bouncing a check) to major (e.g., being in jail overnight, getting divorced). In support of findings such as these, Reference Schwartz, Ben-Haim and DacsoSchwartz et al.(2011) theorized that, due to the incompleteness of information regarding alternatives and the difficulty of comparing alternatives, “robust satisficing”—that is, making decisions after judging whether the outcome will be “good enough … even if things go poorly” (Reference Schwartz, Ben-Haim and DacsoSchwartz et al., 2011, p. 212)—is a better way to make decisions than objectively trying to compare alternatives across a list of criteria (i.e., maximizing).
Conversely, however, there is some evidence that maximizers make better decisions than satisficers. A study of college students’ job searches during their final semester of school (Reference Iyengar, Wells and SchwartzIyengar et al., 2006) revealed that, although maximizers (as measured by the MS) were less happy than satisficers with their job search outcomes, they secured jobs with starting salaries that were on average 20% higher. That is, at least in this case, maximizers’ more extensive job searches did yield objectively better results.
Taken as a whole, then, the extant JDM literature has been equivocal about the impact of maximizing on decision-making performance. One reason for the equivocal findings may be that, in the process of seeking out as many alternatives as possible, maximizers uncover a larger number of both good and bad alternatives (Reference PolmanPolman, 2010). Subsequent performance would then be determined by whether a good or bad alternative is ultimately chosen. In other words, although maximizers attempt to seek out the best alternative, they may succeed merely in generating more alternatives. In support of this contention, Reference PolmanPolman (2010) found that maximizers (as measured by the MS) experienced both more positive and more negative life events than satisficers.
We turn now to the possible role of maximizing in determining performance in organizational settings. Organizational researchers often use in-role performance and income as proximal and distal performance outcomes. In-role (or task) performance refers to the execution of tasks required by one’s job, including completing assignments on time and complying with company rules (Reference Williams and AndersonWilliams & Anderson, 1991). Income represents a more distal indicator of performance because, all else being equal (e.g., level of education, parental socioeconomic status), individuals who perform better should on average receive a higher income.
As in the case of job satisfaction, there exist traditional dispositional individual-differences predictors of in-role performance in the organizational literature. For example, a meta-analysis by Reference Barrick and MountBarrick and Mount (1991) revealed that the personality trait of conscientiousness is a consistently strong predictor of in-role performance. Conscientious individuals tend to take their work more seriously, are better organized, and are ultimately more reliable than those who are not conscientious (Reference Judge, Heller and MountJudge et al., 2002). These individuals exhibit greater productivity and partake less in work avoidance/withdrawal behaviors than individuals low in conscientiousness. Importantly, conscientious individuals have been found to not only perform better at work but also to receive a higher income (Reference Spurk and AbeleSpurk & Abele, 2011).
More recent meta-analytic work has not only reiterated the role of conscientiousness in predicting job performance but has also revealed neuroticism to be a strong predictor. Specifically, job performance was higher for individuals scoring low on neuroticism than for individuals scoring high on this trait (Reference Hurtz and DonovanHurtz & Donovan, 2000). The authors reasoned that individuals who score low on neuroticism tend to be calm, secure, and well adjusted, and that these qualities contribute to higher in-role performance. Moreover, similar to conscientiousness, low neuroticism has also been associated with higher income levels (Reference Spurk and AbeleSpurk & Abele, 2011).
CSE—which incorporates neuroticism (typically discussed here in terms of its opposite pole, emotional stability)—is also relevant to task performance. In fact, CSE has been deemed “among the best dispositional predictors of job satisfaction and job performance” (Reference Judge and BonoJudge & Bono, 2001, p. 80). All four facets of CSE (emotional stability, self-esteem, locus of control, and generalized self-efficacy) were found to correlate at least moderately with performance (Reference Judge and BonoJudge & Bono, 2001; Reference Erez and JudgeErez & Judge, 2001; Reference JudgeJudge, 2009). Researchers believe that individuals who score high in CSE tend to maintain optimism in the face of failure and tend to persist through difficult tasks because they are confident in their general ability to perform (Reference Judge and BonoJudge & Bono, 2001). Regarding income, Reference Judge and HurstJudge and Hurst (2008) demonstrated that high CSE is associated with not only higher initial income but also steeper increases in income over time.
With these traditional individual-difference predictors (i.e., conscientiousness and CSE) in place, the relative importance of maximizing in predicting in-role performance and income is unclear. Moreover, the previously discussed conceptual and empirical differences between the MS and MTS suggest that the impact of maximizing on in-role performance and income may differ as a function of how the construct is measured. For these reasons, we use research questions rather than formal hypotheses to examine the: (a) possibility of differential impact of maximizing on in-role performance and income as a function of whether maximizing is measured using the MS or MTS, and (b) relative importance of maximizing vis-à-vis core self-evaluations and conscientiousness when predicting in-role performance and income, including whether the relative importance varies as a function of whether maximizing is measured using the MS or MTS.
Research question 2: Do the relationships exhibited by maximizing with in-role performance and income vary as a function of whether maximizing is measured using the Maximization Scale (MS) versus the Maximizing Tendency Scale (MTS)?
Research question 3: What is the relative importance of maximizing vis-à-vis core self-evaluations and conscientiousness when predicting in-role performance and income? Does the relative importance change when maximizing is measured using the Maximization Scale (MS) versus the Maximizing Tendency Scale (MTS)?
Finally, with regard to in-role performance and income, we would expect the decision difficulty and (to a lesser extent) alternative-search dimensions of the Reference Nenkov, Morrin, Ward, Schwartz and HullandNenkov et al. (2008) scale to behave similarly to the MS, and we would expect the high-standards dimension to behave similarly to the MTS.
2 Method
2.1 Participants and procedure
We collected data in two waves that were three weeks apart. We chose this short time gap between the two waves of data as a compromise between methodological advantages and theoretical expectations. A time gap between the measurement of predictors (including statistical controls) and criteria is methodologically advantageous as it allows for increased confidence in casual direction and less common method variance (see Reference Doty and GlickDoty & Glick, 1998). However, from a theoretical perspective, the impact of maximizing and the traditional predictors on job satisfaction and performance should be immediate (and enduring). To balance these two considerations, we chose a relatively short time period of three weeks. The sample size was 351 at Wave 1 and 252 at Wave 2 (retention rate across waves = 71.8%). Data from 15 individuals were excluded from further analysis due to concerns about quality (i.e., these individuals had a very fast completion time on either Wave 1 or Wave 2), leading to an effective sample size of 237. We compared respondents who completed both waves of data to those who completed only the first wave on the focal variables in the study (i.e., maximizing measured using the MS, maximizing measured using the MTS, the three dimensions of maximizing measured using the Nenkov et al., 2008, scale, job satisfaction, intentions to quit, in-role performance, and income) as well as on demographic characteristics (e.g., age, gender) using independent-samples t-tests. Results indicated no statistically significant differences on any of the focal variables. However, vis-à-vis demographic variables, respondents who completed both waves of data were significantly older, were significantly more likely to be male, had significantly lower parental socioeconomic status, had significantly lower educational attainment, and had significantly longer organizational tenure than those who completed only the first wave. We therefore used age, gender, parental socioeconomic status, educational attainment, and organizational tenure as statistical control variables in the analyses.
We recruited survey respondents from Mechanical Turk (MTurk), an online labor market provided by Amazon.com where “workers” (in our case, respondents) are recruited by “requesters” (in our case, researchers) for the completion of “human intelligence tasks” (in our case, surveys) in exchange for wages. MTurk has become a popular recruitment tool among social scientists (e.g., Reference Butler, Rosman, Seleski, Garcia, Lee, Barnes and SchwartzButler et al., 2012; Reference PolmanPolman, 2012; Reference Senecal, Wang, Thompson and KableSenecal et al., 2012; Reference Ulku, Dimofte and SchmidtUlku et al., 2012; Reference West, Meserve and StanovichWest et al., 2012). Research suggests that MTurk research participants provide data that are at least as reliable as data obtained via traditional methods (e.g., Reference Behrend, Sharek, Meade and WiebeBehrend et al., 2011; Reference Buhrmester, Kwang and GoslingBuhrmester et al., 2011; Reference IpeirotisIpeirotis, 2010; Reference Paolacci, Chandler and IpeirotisPaolacci et al., 2010). Moreover, findings (including seminal JDM findings such as the framing effect, conjunction fallacy, and outcome bias) from several well-known laboratory experiments using traditional samples have been replicated with MTurk samples (see Reference Goodman, Cryder and CheemaGoodman et al., 2012; Reference Horton, Rand and ZeckhauserHorton et al., 2010; Reference Paolacci, Chandler and IpeirotisPaolacci et al., 2010). Finally, the demographic makeup of MTurk samples is slightly more diverse than that of standard internet samples and considerably more diverse than that of typical university samples with respect to several variables (e.g., age, education level, employment status, profession; Reference Behrend, Sharek, Meade and WiebeBehrend et al., 2011; Reference Buhrmester, Kwang and GoslingBuhrmester et al., 2011; Reference IpeirotisIpeirotis, 2010), thereby increasing the external validity of the findings.
This greater demographic diversity notwithstanding, there is reason to be concerned about range restriction in income, occupation and educational attainment in the MTurk population (Reference IpeirotisIpeirotis, 2010). Consequently, we recruited a stratified random sample of respondents on the basis of educational attainment (under the assumption that greater variability in educational attainment would also be associated with greater variability in occupation and income). Moreover, because our criteria of interest were work-related, respondents were required to work full time (defined as 35 or more hours per week) to be eligible for participation. Finally, because one of our criteria was annual income, it was important to ensure at least approximate purchasing power parity; therefore, respondents were required to be located in the U.S. to be eligible for participation. The sample that completed both waves of the survey was 52.9% male and 79.7% Non-Hispanic White, had a mean age of 34.5 years (SD = 9.8 years), and had a median annual income range of $40,000 to $49,999. Fifty three percent of participants had attended at least some college. Respondents had been employed at their current organization an average of 4.8 years (SD = 4.3 years) and indicated that, when they were growing up, their parents were on average of mid-range socioeconomic status (M = 5.4 and SD = 2.0 on a 1-10 scale, with 1 = lowest and 10 = highest).
2.2 Measures
Maximizing.
Three scales were used to assess maximizing during Wave 1: the Maximization Scale (MS; Reference Schwartz, Ward, Monterosso, Lyubomirsky, White and LehmanSchwartz, 2002), the Maximization Tendency Scale (MTS; Reference Diab, Gillespie and HighhouseDiab et al., 2008), and a shortened version of the MS scale (Reference Nenkov, Morrin, Ward, Schwartz and HullandNenkov et al., 2008). As discussed previously, there is some overlap in the items included on each scale. Specifically, the short-form MS was composed of a subset of items from the MS, and the MTS used three items from the MS (along with six new items). For this study, participants completed each maximizing item only once, and we created scale scores by taking the average of each participant’s responses to the set of items included in each scale. All items used in these three maximizing scales appear in the Appendix.
The MS (Reference Schwartz, Ward, Monterosso, Lyubomirsky, White and LehmanSchwartz et al., 2002) consists of 13 items (Cronbach’s α = .67) for which respondents indicated degree of agreement on a 1 (completely disagree) to 7 (completely agree) scale. Although evidence arguing for the multidimensionality of the MS is available in Reference Schwartz, Ward, Monterosso, Lyubomirsky, White and LehmanSchwartz et al.’s (2002) own data as well as in subsequent research evaluating the MS (e.g., Reference Diab, Gillespie and HighhouseDiab et al., 2008; Reference Nenkov, Morrin, Ward, Schwartz and HullandNenkov et al., 2008), we use the overall composite score in our analyses because this is the practice advocated by Schwartz et al. and adhered to by other researchers who have used the MS to predict various outcomes (e.g., Reference Iyengar, Wells and SchwartzIyengar et al., 2006; Reference PolmanPolman, 2010; Reference Purvis, Howell and IyerPurvis, et al., 2011). We did, however, reanalyze the data using a multidimensional conceptualization of the MS; conclusions in this case were identical to those described subsequently for the Reference Nenkov, Morrin, Ward, Schwartz and HullandNenkov et al. (2008) scale.
The MTS (Reference Diab, Gillespie and HighhouseDiab et al., 2008) consists of 9 items (α = .84), three of which are retained from the MS. Respondents indicated degree of agreement on a 1 (strongly disagree) to 7 (strongly agree) scale. The reliabilities for the MS and MTS obtained in this study are similar to those obtained in previous research. For example, the alpha for the MS ranges from .54 to .75 (Reference Diab, Gillespie and HighhouseDiab et al., 2008; Reference Schwartz, Ward, Monterosso, Lyubomirsky, White and LehmanSchwartz et al., 2002; Reference Nenkov, Morrin, Ward, Schwartz and HullandNenkov et al., 2008), and Reference Diab, Gillespie and HighhouseDiab et al. (2008) obtained an alpha of .80 for the MTS.
We also assessed the 6-item short form of the MS recommended by Reference Nenkov, Morrin, Ward, Schwartz and HullandNenkov et al. (2008), in which each of the three dimensions is measured with two items. As in the original MS, respondents indicated degree of agreement on a 1 (completely disagree) to 7 (completely agree) scale. Obtained reliabilities (Cronbach’s α ) were .29 for alternative search, .56 for decision difficulty, and .65 for high standards. These obtained reliabilities are similar to those obtained by Reference Nenkov, Morrin, Ward, Schwartz and HullandNenkov et al. (2008), who analyzed 12 independent samples and reported reliabilities of .22–.58 for alternative search, .37–.63 for decision difficulty, and .52–.79 for high standards. Typical recommendations in the research literature, however, call for a Cronbach’s alpha of at least .80 (Reference Lance, Butts and MichelsLance et al., 2006) and indicate that an alpha lower than .70 is inadequate for almost any purpose (e.g., Nunnally, 1978, suggested that a reliability of 0.70 might be appropriate only if researchers are attempting to save time and energy in the early stages of research). Because an alpha of even 0.70 was never exceeded for the short form of the MS (either for the composite score or for any dimension score) in the current study and was almost never exceeded in the 12 samples reported by Reference Nenkov, Morrin, Ward, Schwartz and HullandNenkov et al. (2008), the results we present for this scale should be interpreted with caution.
Core self-evaluations.
Core self-evaluations, which encompass emotional stability (the opposite pole of neuroticism), were measured in Wave 1 using the 12-item Core Self-Evaluations Scale (CSES; Reference Judge, Erez, Bono and ThoresenJudge et al., 2003). Participants used a 1 (strongly disagree) to 5 (strongly agree) scale. The scale had an alpha of .90. Items on this scale include: “I am confident I get the success I deserve in life,” and “Sometimes I feel depressed” (reverse-scored).
Conscientiousness.
Conscientiousness was measured by the NEOPI-R (Reference Goldberg, Mervielde, Deary, De Fruyt and OstendorfGoldberg, 1999). Respondents were asked to indicate how accurately ten statements described them on a scale of 1 (very inaccurate) to 5 (very accurate). The scale had an alpha of .92. Although conscientiousness was a predictor of interest and would normally have been administered during the first wave of data collection, it was administered during Wave 2 to balance the length of the two waves of the survey and because, for an adult sample, personality scores would not be expected to change appreciably over a 3-week period (Reference Costa and McCraeCosta & McCrae, 1986). Sample conscientiousness items include: “Am always prepared” and “Waste my time” (reverse-scored).
Job satisfaction.
We administered Reference Judge, Bono and LockeJudge, Bono, and Locke’s (2000) abbreviated (5-item) version of the Reference Brayfield and RotheBrayfield and Rothe (1951) scale during Wave 2 (α = .94). Respondents indicated their agreement with each positive or reverse-scored item (e.g., “Most days I am enthusiastic about my work” and “I consider my job rather unpleasant”) using a scale ranging from 1 (strongly disagree) to 7 (strongly agree).
Intentions to quit.
Intentions to quit were assessed using Camman et al.’s (1979; as cited in Cook et al., 1981) 3-item scale during Wave 2 (α = .92). Participants first indicated their agreement with two items (i.e., “I often think about quitting” and “I will probably look for a job in the next year”) using a scale ranging from 1 (strongly disagree) to 7 (strongly agree) and then answered the question “How likely is it that you will actively look for a new job in the next year?” using a scale ranging from 1 (not at all likely) to 7 (extremely likely).
In-role task performance.
To assess in-role task performance, Reference Williams and AndersonWilliams and Anderson’s (1991) 7-item scale was administered during Wave 2 (α =.74). Respondents indicated how often they participated in each behavior, such as “Adequately complete assigned duties” and “Fail to perform essential duties” (reverse-scored), on a scale ranging from 1 (never) to 5 (always).
Annual income.
Total annual income before tax was assessed with one item (Reference Judge, Klinger and SimonJudge et al., 2010) during Wave 2: “What is your total annual income before tax, including all sources of income (salary, wages, commissions, tips, etc.)?” Response options were banded in $10,000 increments, with the lowest band being “Less than $10,000” and the highest being “$150,000 or more”). To preclude having to convert multiple currencies into U.S.$ and having to adjust for purchasing power parity across countries, we restricted our sample to people employed in the U.S.
Demographic and other control variables.
All control variables except social desirability were assessed with one item each. These control variables included age, gender, parental socioeconomic status (SES) while the respondent was growing up (modified from the MacArthur scale of subjective social status; Reference Adler and StewartAdler & Stewart, 2007), highest educational level attained, and organizational tenure. These variables, which were measured during Wave 1, were used as statistical controls in the relative importance analyses because: (1) scores on these variables differed significantly as a function of respondent attrition across the two waves of data collection, and (2) some of these variables are important predictors of the criteria of interest in their own right (e.g., age is a predictor of job satisfaction; age, education and parental SES are predictors of income; Reference JencksJencks, 1979; Reference Luchman, Kaplan and DalalLuchman et al., 2012).
The relative importance analyses were also conducted with dispositional social desirability as a control. Participants were asked to indicate whether each of 13 statements was true or false as it pertained to them personally, using Reference ReynoldsReynolds’ (1982) brief version of the Marlowe-Crowne scale. Dispositional social desirability was included as a statistical control because previous research (Reference Behrend, Sharek, Meade and WiebeBehrend et al., 2011) suggests that social desirability bias may be higher among MTurk workers than among other populations. Although we found that the relative importance of the focal predictor variables (maximizing, CSE, and conscientiousness) did not differ when social desirability was excluded, for the sake of completeness we report analyses that include social desirability.
3 Results
A correlation matrix is provided in Table 1. Relative importance analyses—performed to assess the simultaneous influence of maximizing, conscientiousness and CSE (along with several statistical control variables) on job satisfaction, intentions to quit, in-role task performance and income—are summarized in Tables 2-13.
Table 1: Correlations and reliabilities for focal constructs.
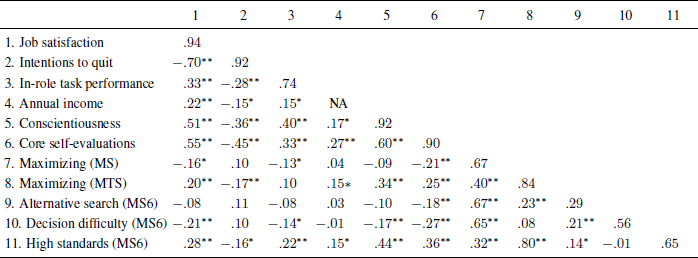
* p < .05
** p < .01
Note. N = 237. Internal consistency reliabilities (Cronbach’s alphas) for each measure are reported in the diagonals, in italics. Income was measured as a single item; consequently, internal consistency reliability could not be calculated for income. MS = Maximizing Scale (Reference Schwartz, Ward, Monterosso, Lyubomirsky, White and LehmanSchwartz et al., 2002). MTS = Maximizing Tendency Scale (Reference Diab, Gillespie and HighhouseDiab et al., 2008). MS6 = Short Form MS (6-item MS) (Reference Nenkov, Morrin, Ward, Schwartz and HullandNenkov et al., 2008)
Relative importance is defined as the contribution made by each predictor variable to the overall model R 2, considering both the predictor’s unique contribution and its contribution in the presence of other predictors (Reference LeBreton, Hargis, Griepentrog, Oswald and PloyhartLeBreton et al., 2007). When several correlated predictors are employed (as in the current case, where maximizing is correlated with CSE and conscientiousness), traditional approaches to assessing relative importance—for example, squared correlations or squared regression weights—may yield misleading estimates of importance (Reference BudescuBudescu, 1993). Instead, researchers interested in the relative contributions made by various predictors have been encouraged (e.g., Reference Van Iddekinge and PloyhartVan Iddekinge & Ployhart, 2008) to rely on assessments of relative importance. The present research assesses relative importance through a relative-weight analysis (Reference JohnsonJohnson, 2000), using Reference JohnsonJohnson’s (2001) “RWEIGHT” SPSS syntax.
In brief, a relative-weight analysis deals with predictor intercorrelations by: (1) transforming the original set of (correlated) predictor variables (X j) into a new set of predictor variables (Z k) that are uncorrelated with each other but maximally correlated (using a least-squares criterion) with the original set of predictor variables,Footnote 1 (2) regressing the criterion (Y) on the uncorrelated variables (Z k) to obtain standardized regression coefficients (βk), which are then squared, (3) regressing X j on Z k to obtain standardized regression coefficients (λjk), which are then squared, and, finally, (4) multiplying the two sets of squared standardized regression coefficients (Reference JohnsonJohnson, 2000; Reference Johnson and LeBretonJohnson & LeBreton, 2004). Relative weights (RW j) are estimated for each predictor variable (e.g., the relative weight for variable X 1 would be ).
Relative weights have the desirable property of summing to the model R 2 (i.e., they offer a complete decomposition of the overall predicted variance). Predictors with higher relative weights are associated with greater increases in R 2 than predictors with lower relative weights. This can be seen more clearly through the rescaled relative weights, which are calculated by dividing the relative weights by R 2, and which sum to 1.00. The rescaled weight for a predictor is interpreted as the proportion (or, when multiplied by 100, the percentage) of explained variance in the criterion that is attributable to that predictor—and, consequently, as the predictor’s relative importance with regard to the criterion (Reference Johnson and LeBretonJohnson, 2004). The predictor importance weights generated using the relative weights approach are virtually identical numerically to those generated using the other major approach to assessing relative importance—Reference BudescuBudescu’s (1993) “dominance analysis”—even though the two approaches are distinct conceptually (Reference Dalal, Baysinger, Brummel and LeBretonDalal et al., 2012; Reference JohnsonJohnson, 2000).
We turn now to tests of our hypotheses and research questions involving the MS and the MTS. Subsequently, we discuss results involving the third maximizing scale: the Reference Nenkov, Morrin, Ward, Schwartz and HullandNenkov et al. (2008) scale.
3.1 Job satisfaction and intentions to quit
Hypothesis 1 stated that maximizing would be more strongly negatively correlated with job satisfaction and more strongly positively correlated with intentions to quit when maximizing was measured using the MS as opposed to the MTS. As can be seen in Table 1 (or a combination of Tables 2 and 3), the correlation between maximizing and job satisfaction was negative when maximizing was measured using the MS (r = −0.16, p = 0.012) but positive when it was measured using the MTS (r = 0.20, p = 0.002). Meng et al.’s (1992) test for the difference between dependent correlation coefficients revealed that, in accordance with Hypothesis 1, the correlation between these two constructs was significantly more negative when maximizing was measured using the MS as opposed to the MTS (r difference = −0.37, p = 0.000).
Table 2: Job satisfaction as a criterion variable: Relative importance of maximizing (measured using the MS; Reference Schwartz, Ward, Monterosso, Lyubomirsky, White and LehmanSchwartz et al., 2002), conscientiousness, core self-evaluations (CSE), social desirability, age, gender, parental SES, education, and organizational tenure on intentions.

Note. Importance weights are univariate relative weights, which sum to the R 2 of the full model (the model containing all the predictors). Rescaled importance weights, which sum to unity, are calculated by dividing the (raw) importance weights by the full-model R 2. Maximizing, conscientiousness, and core self-evaluations are focal predictor variables; the remaining variables are statistical controls (see Method section for details). MS = Maximizing Scale (Reference Schwartz, Ward, Monterosso, Lyubomirsky, White and LehmanSchwartz et al., 2002). Consc. = Conscientiousness. CSE = Core Self-Evaluations. SES = socioeconomic status. Gender is scored such that higher scores denote men.
Table 3: Job satisfaction as a criterion variable: Relative importance of maximizing (measured using the MTS; Reference Diab, Gillespie and HighhouseDiab et al., 2008), conscientiousness, core self-evaluations (CSE), social desirability, age, gender, parental SES, education, and organizational tenure.
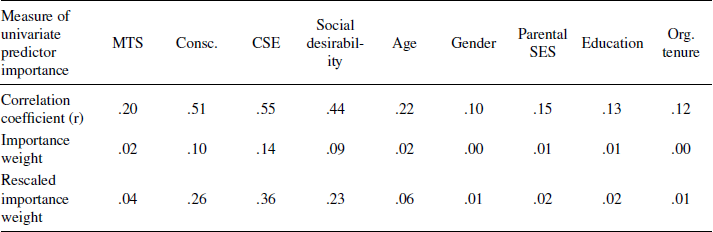
Note. Importance weights are univariate relative weights, which sum to the R 2 of the full model (the model containing all the predictors). Rescaled importance weights, which sum to unity, are calculated by dividing the (raw) importance weights by the full-model R 2. Maximizing, conscientiousness, and core self-evaluations are focal predictor variables; the remaining variables are statistical controls (see Method section for details). MTS = Maximizing Tendency Scale (Reference Diab, Gillespie and HighhouseDiab et al., 2008). Consc. = Conscientiousness. CSE = Core Self-Evaluations. SES = socioeconomic status. Gender is scored such that higher scores denote men.
Similarly, as can be seen in Table 1 (or a combination of Tables 4 and 5), the correlation between maximizing and intentions to quit was positive (albeit non-significantly so) when maximizing was measured using the MS (r = 0.10, p = 0.114) but negative when it was measured using the MTS (r = −0.17, p = 0.008). Meng et al.’s (1992) test revealed that, in accordance with Hypothesis 1, the correlation between these two constructs was indeed significantly more positive when maximizing was measured using the MS as opposed to the MTS (r difference = 0.28, p = 0.000).
Table 4: Intentions to quit as a criterion variable: Relative importance of maximizing (measured using the MS; Reference Schwartz, Ward, Monterosso, Lyubomirsky, White and LehmanSchwartz et al., 2002), conscientiousness, core self-evaluations (CSE), social desirability, age, gender, parental SES, education, and organizational tenure.

Note. Importance weights are univariate relative weights, which sum to the R 2 of the full model (the model containing all the predictors). Rescaled importance weights, which sum to unity, are calculated by dividing the (raw) importance weights by the full-model R 2. Maximizing, conscientiousness, and core self-evaluations are focal predictor variables; the remaining variables are statistical controls (see Method section for details). MS = Maximizing Scale (Reference Schwartz, Ward, Monterosso, Lyubomirsky, White and LehmanSchwartz et al., 2002). Consc. = Conscientiousness. CSE = Core Self-Evaluations. SES = socioeconomic status. Gender is scored such that higher scores denote men.
Table 5: Intentions to quit as a criterion variable: Relative importance of maximizing (measured using the MTS; Reference Diab, Gillespie and HighhouseDiab et al., 2008), conscientiousness, core self-evaluations (CSE), social desirability, age, gender, parental SES, education, and organizational tenure.
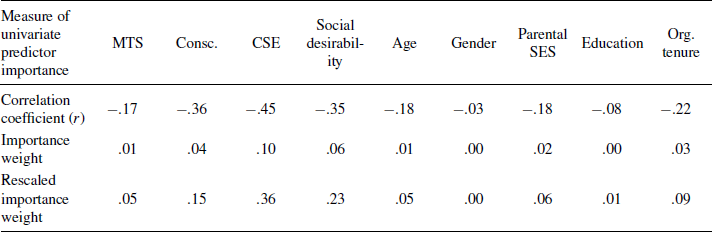
Note. Importance weights are univariate relative weights, which sum to the R 2 of the full model (the model containing all the predictors). Rescaled importance weights, which sum to unity, are calculated by dividing the (raw) importance weights by the full-model R 2. Maximizing, conscientiousness, and core self-evaluations are focal predictor variables; the remaining variables are statistical controls (see Method section for details). MTS = Maximizing Tendency Scale (Reference Diab, Gillespie and HighhouseDiab et al., 2008). Consc. = Conscientiousness. CSE = Core Self-Evaluations. SES = socioeconomic status. Gender is scored such that higher scores denote men.
In summary, Hypothesis 1 was fully supported. The negative relationship between maximizing and job satisfaction was obtained only when maximizing was measured using the MS. The same was true for the positive relationship between maximizing and intentions to quit.
Hypothesis 2 focused on the MTS alone, and stated that the relative importance of maximizing (as measured by the MTS) would be lower than that of CSE and conscientiousness when predicting job satisfaction and intentions to quit. Similarly, Research Question 1 focused on the MS alone, and pertained to the relative importance of maximizing (as measured by the MS) versus CSE and conscientiousness when predicting job satisfaction and intentions to quit.Footnote 2 Results for Research Question 1 are summarized in Tables 2 and 4, and results for Hypothesis 2 are summarized in Tables 3 and 5. In support of Hypothesis 2, the rescaled importance weights for maximizing as measured by the MTS (rescaled weights = 0.04 and 0.05 when predicting job satisfaction and intentions to quit, respectively) were indeed considerably lower than the rescaled importance weights for CSE (0.36 and 0.36, respectively) and for conscientiousness (0.26 and 0.15, respectively). Results were similar for Research Question 1: the rescaled weights for maximizing as measured by the MS (rescaled weights = 0.02 and 0.01 when predicting job satisfaction and intentions to quit, respectively) were considerably lower than the rescaled weights for CSE (0.36 and 0.37, respectively) and for conscientiousness (0.28 and 0.18, respectively). In summary, maximizing, regardless of whether it was measured by the MS or MTS, was outperformed by CSE and conscientiousness when predicting job satisfaction and intentions to quit.
3.2 In-role performance and income
Research Question 2 pertained to whether the relationships exhibited by maximizing with in-role (task) performance and annual income differed as a function of whether maximizing was measured using the MS versus the MTS.
As can be seen in Table 1 (or a combination of Tables 6 and 7), the correlation between maximizing and in-role performance was negative when maximizing was measured using the MS (r = −0.13, p = 0.049) but positive (albeit non-significantly so) when it was measured using the MTS (r = 0.10, p = 0.122). Meng et al.’s (1992) test revealed that the correlation between these two constructs was significantly more negative when maximizing was measured using the MS as opposed to the MTS (r difference = −0.23, p = 0.001).
Table 6: In-role (task) performance as a criterion variable: Relative importance of maximizing (measured using the MS; Reference Schwartz, Ward, Monterosso, Lyubomirsky, White and LehmanSchwartz et al., 2002), conscientiousness, core self-evaluations (CSE), social desirability, age, gender, parental SES, education, and organizational tenure.
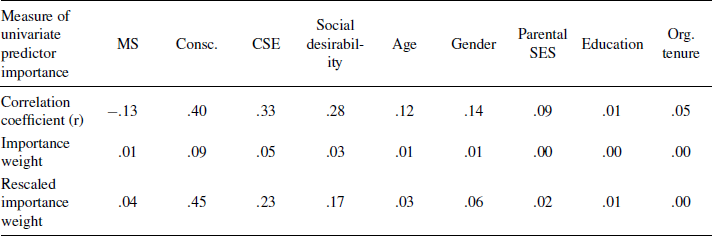
Note. Importance weights are univariate relative weights, which sum to the R 2 of the full model (the model containing all the predictors). Rescaled importance weights, which sum to unity, are calculated by dividing the (raw) importance weights by the full-model R 2. Maximizing, conscientiousness, and core self-evaluations are focal predictor variables; the remaining variables are statistical controls (see Method section for details). MS = Maximizing Scale (Reference Schwartz, Ward, Monterosso, Lyubomirsky, White and LehmanSchwartz et al., 2002). Consc. = Conscientiousness. CSE = Core Self-Evaluations. SES = socioeconomic status. Org. = Organizational. Gender is scored such that higher scores denote men.
Table 7: In-role (task) performance as a criterion variable: Relative importance of maximizing (measured using the MTS; Reference Diab, Gillespie and HighhouseDiab et al., 2008), conscientiousness, core self-evaluations (CSE), social desirability, age, gender, parental SES, education, and organizational tenure.

Note. Importance weights are univariate relative weights, which sum to the R 2 of the full model (the model containing all the predictors). Rescaled importance weights, which sum to unity, are calculated by dividing the (raw) importance weights by the full-model R 2. Maximizing, conscientiousness, and core self-evaluations are focal predictor variables; the remaining variables are statistical controls (see Method section for details). MTS = Maximizing Tendency Scale (Reference Diab, Gillespie and HighhouseDiab et al., 2008). Consc. = Conscientiousness. CSE = Core Self-Evaluations. SES = socioeconomic status. Org. = Organizational. Gender is scored such that higher scores denote men.
As can be seen in Table 1 (or a combination of Tables 8 and 9), the correlation between maximizing and annual income was positive regardless of how maximizing was measured; however, the correlation was non-significant when maximizing was measured using the MS (r = 0.04, p = 0.530) whereas it was significant when maximizing was measured using the MTS (r = 0.15, p = 0.026). Meng et al.’s (1992) test revealed that the correlation between these two constructs was not significantly different when maximizing was measured using the MS as opposed to the MTS (r difference = −0.10, p = .001).
Table 8: Annual income as a criterion variable: Relative importance of maximizing (measured using the MS; Reference Schwartz, Ward, Monterosso, Lyubomirsky, White and LehmanSchwartz et al., 2002), conscientiousness, core self-evaluations (CSE), social desirability, age, gender, parental SES, education, and organizational tenure.

Note. Importance weights are univariate relative weights, which sum to the R 2 of the full model (the model containing all the predictors). Rescaled importance weights, which sum to unity, are calculated by dividing the (raw) importance weights by the full-model R 2. Maximizing, conscientiousness, and core self-evaluations are focal predictor variables; the remaining variables are statistical controls (see Method section for details). MS = Maximizing Scale (Reference Schwartz, Ward, Monterosso, Lyubomirsky, White and LehmanSchwartz et al., 2002). Consc. = Conscientiousness. CSE = Core Self-Evaluations. SES = socioeconomic status. Org. = Organizational. Gender is scored such that higher scores denote men.
Table 9: Annual income as a criterion variable: Relative importance of maximizing (measured using the MTS; Reference Diab, Gillespie and HighhouseDiab et al., 2008), conscientiousness, core self-evaluations (CSE), social desirability, age, gender, parental SES, education, and organizational tenure.

Note. Importance weights are univariate relative weights, which sum to the R 2 of the full model (the model containing all the predictors). Rescaled importance weights, which sum to unity, are calculated by dividing the (raw) importance weights by the full-model R 2. Maximizing, conscientiousness, and core self-evaluations are focal predictor variables; the remaining variables are statistical controls (see Method section for details). MTS = Maximizing Tendency Scale (Reference Diab, Gillespie and HighhouseDiab et al., 2008). Consc. = Conscientiousness. CSE = Core Self-Evaluations. SES = socioeconomic status. Org. = Organizational. Gender is scored such that higher scores denote men.
In summary, tests of Research Question 2 revealed that the negative relationship between maximizing and in-role performance was obtained only when maximizing was measured using the MS. The same was true for the non-significant relationship between maximizing and annual income.
Research Question 3 pertained to the relative importance of maximizing versus CSE and conscientiousness when predicting in-role performance and annual income, as well as whether these relationships differed as a function of whether maximizing was measured using the MS versus the MTS. Results for Research Question 3 are summarized in Tables 6–9. As can be seen in Tables 6 and 8, the rescaled weights for maximizing as measured by the MS (rescaled weights = 0.04 and 0.02 when predicting in-role performance and annual income, respectively) were considerably lower than the rescaled weights for CSE (0.23 and 0.20, respectively) and for conscientiousness (0.45 and 0.05, respectively).
As can be seen in Table 7, when predicting in-role performance the rescaled weight for maximizing as measured by the MTS (rescaled weight = 0.02) was considerably lower than the rescaled weights for CSE (0.24) and for conscientiousness (0.44). As can be seen in Table 9, when predicting annual income the rescaled weight for maximizing as measured by the MTS (rescaled weight = 0.05) was considerably lower than the rescaled weight for CSE (0.17) and was roughly equivalent to the rescaled weight for conscientiousness (0.04).
3.3 Results from the Reference Nenkov, Morrin, Ward, Schwartz and HullandNenkov et al. (2008) scale
We now report on the findings from analyses operationalizing maximizing via the Reference Nenkov, Morrin, Ward, Schwartz and HullandNenkov et al. (2008) three dimensions, with a particular focus on comparing these results to those obtained using Reference Schwartz, Ward, Monterosso, Lyubomirsky, White and LehmanSchwartz et al.’s (2002) MS and Reference Diab, Gillespie and HighhouseDiab et al.’s (2008) MTS. In line with our expectations, the decision-difficulty dimension and (to a lesser extent) the alternative-search dimension operated similarly to the MS. As shown in Table 1, these two dimensions were correlated negatively with job satisfaction and in-role performance, were correlated positively with intentions to quit, and were effectively uncorrelated with annual income. Also in line with our expectations, the high-standards dimension operated similarly to the MTS: this dimension was correlated positively with job satisfaction, in-role performance and annual income, and was correlated negatively with intentions to quit. Finally, as can be seen in Tables 10-13, relative importance analyses once again revealed maximizing—as operationalized by all three Nenkov et al. dimensions—to be much less important than CSE or conscientiousness in predicting job satisfaction, intentions to quit, in-role performance, and annual income (with the sole exception being in the prediction of annual income, where the importance weight for the high-standards dimension was roughly equivalent to that for conscientiousness).
Table 10: Job satisfaction as a criterion variable: Relative importance of maximizing (measured using the short form MS; Reference Nenkov, Morrin, Ward, Schwartz and HullandNenkov et al., 2008), conscientiousness, core self-evaluations (CSE), social desirability, age, gender, parental SES, education, and organizational tenure.
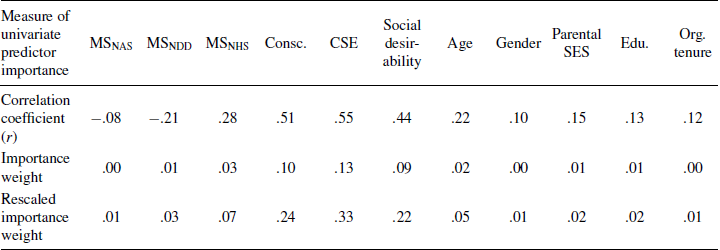
Note. Importance weights are univariate relative weights, which sum to the R 2 of the full model (the model containing all the predictors). Rescaled importance weights, which sum to unity, are calculated by dividing the (raw) importance weights by the full-model R 2. Maximizing, conscientiousness, and core self-evaluations are focal predictor variables; the remaining variables are statistical controls (see Method section for details). MSNAS = Reference Nenkov, Morrin, Ward, Schwartz and HullandNenkov et al.’s (2008) short form MS Alternative Search; MSNDD = Reference Nenkov, Morrin, Ward, Schwartz and HullandNenkov et al.’s (2008) short form MS Decision Difficulty; MSNHS = Reference Nenkov, Morrin, Ward, Schwartz and HullandNenkov et al.’s (2008) short form MS High Standards. Consc. = Conscientiousness. CSE = Core Self-Evaluations. Edu. = highest level of education attained. Org. = Organizational. SES = socioeconomic status. Gender is scored such that higher scores denote men.
Table 11: Intentions to quit as a criterion variable: Relative importance of maximizing (measured using the short form MS; Reference Nenkov, Morrin, Ward, Schwartz and HullandNenkov et al., 2008), conscientiousness, core self-evaluations (CSE), social desirability, age, gender, parental SES, education, and organizational tenure.
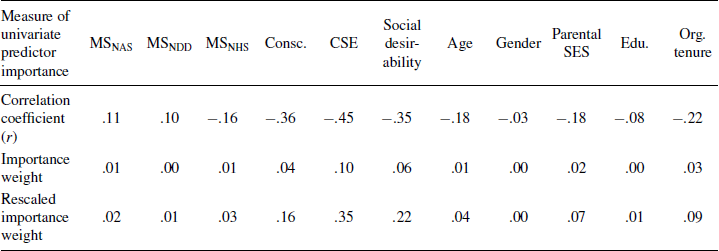
Note. Importance weights are univariate relative weights, which sum to the R 2 of the full model (the model containing all the predictors). Rescaled importance weights, which sum to unity, are calculated by dividing the (raw) importance weights by the full-model R 2. Maximizing, conscientiousness, and core self-evaluations are focal predictor variables; the remaining variables are statistical controls (see Method section for details). MSNAS = Reference Nenkov, Morrin, Ward, Schwartz and HullandNenkov et al.’s (2008) short form MS Alternative Search; MSNDD = Reference Nenkov, Morrin, Ward, Schwartz and HullandNenkov et al.’s (2008) short form MS Decision Difficulty; MSNHS = Reference Nenkov, Morrin, Ward, Schwartz and HullandNenkov et al.’s (2008) short form MS High Standards. Edu. = highest level of education attained. Org. = Organizational. SES = socioeconomic status. Gender is scored such that higher scores denote men.
Table 12: In-role (task) performance as a criterion variable: Relative importance of maximizing (measured using the short form MS; Reference Nenkov, Morrin, Ward, Schwartz and HullandNenkov et al., 2008), conscientiousness, core self-evaluations (CSE), social desirability, age, gender, parental SES, education, and organizational tenure.

Note. Importance weights are univariate relative weights, which sum to the R 2 of the full model (the model containing all the predictors). Rescaled importance weights, which sum to unity, are calculated by dividing the (raw) importance weights by the full-model R 2. Maximizing, conscientiousness, and core self-evaluations are focal predictor variables; the remaining variables are statistical controls (see Method section for details). MSNAS = Reference Nenkov, Morrin, Ward, Schwartz and HullandNenkov et al.’s (2008) short form MS Alternative Search; MSNDD = Reference Nenkov, Morrin, Ward, Schwartz and HullandNenkov et al.’s (2008) short form MS Decision Difficulty; MSNHS = Reference Nenkov, Morrin, Ward, Schwartz and HullandNenkov et al.’s (2008) short form MS High Standards. Edu. = highest level of education attained. Org. = Organizational. SES = socioeconomic status. Gender is scored such that higher scores denote men.
Table 13: Annual income as a criterion variable: Relative importance of maximizing (measured using the short form MS; Reference Nenkov, Morrin, Ward, Schwartz and HullandNenkov et al., 2008), conscientiousness, core self-evaluations (CSE), social desirability, age, gender, parental SES, education, and organizational tenure.

Note. Importance weights are univariate relative weights, which sum to the R 2 of the full model (the model containing all the predictors). Rescaled importance weights, which sum to unity, are calculated by dividing the (raw) importance weights by the full-model R 2. Maximizing, conscientiousness, and core self-evaluations are focal predictor variables; the remaining variables are statistical controls (see Method section for details). MSNAS = Reference Nenkov, Morrin, Ward, Schwartz and HullandNenkov et al.’s (2008) short form MS Alternative Search; MSNDD = Reference Nenkov, Morrin, Ward, Schwartz and HullandNenkov et al.’s (2008) short form MS Decision Difficulty; MSNHS = Reference Nenkov, Morrin, Ward, Schwartz and HullandNenkov et al.’s (2008) short form MS High Standards. Edu. = highest level of education attained. Org. = Organizational. SES = socioeconomic status. Gender is scored such that higher scores denote men.
4 Discussion
Because judgment and decision-making are ubiquitous in work organizations, it is important to assess the circumstances under which theories and constructs from the JDM literature can be integrated into the organizational literature (and vice versa). Recently, maximizing has been a particularly prominent construct in JDM research. The current paper sought to determine the usefulness of maximizing as a predictor of commonly studied organizational criteria: job satisfaction, intentions to quit, in-role task performance, and annual income.
Paralleling JDM findings vis-à-vis the relationship between maximizing and life satisfaction, we found that maximizing was negatively related to job satisfaction and positively related to intentions to quit when maximizing is measured using the MS—but not when it was measured using the MTS. Similarly, paralleling the more typical (albeit not universal) JDM finding vis-à-vis maximizing and decision-making performance (in which maximizing was typically measured using the MS), we found that maximizing was negatively related to in-role performance when maximizing is measured using the MS—but not when it is measured using the MTS.
Thus, in both the JDM research and our own organizational research, findings depend on which scale is used to measure maximizing. The widely touted malign influence of maximizing was obtained only when maximizing is measured using the MS. These findings suggest the need for conceptual clarity regarding what, precisely, is meant by “maximizing” (Reference Nenkov, Morrin, Ward, Schwartz and HullandNenkov et al., 2008), and whether it is in fact maladaptive for a person to be a maximizer. An exhaustive discussion of this topic is beyond the scope of the current paper. However, Reference SimonSimon’s (1955) original thoughts regarding pursuing the “best” alternative versus “satisficing” are most consistent with the dimension of “high standards” (Reference Nenkov, Morrin, Ward, Schwartz and HullandNenkov et al., 2008), which is much more similar both conceptually and in terms of empirical findings to the MTS than the MS.
Consequently, the original MS, in which the use of a composite score masks the functioning of the high-standards dimension, may be more difficult to interpret. It may therefore be inappropriate for researchers to conclude, on the basis of results generated solely from the original MS, that the construct of maximizing is maladaptive with regard to either satisfaction (in life overall or on the job in particular) or performance (on specific decision-making tasks or in the job-role). Indeed, maximizing as measured by the MTS (and the Reference Nenkov, Morrin, Ward, Schwartz and HullandNenkov et al., 2008, dimension of high standards) is correlated positively with both satisfaction and performance.
Why might this be the case? Although setting high standards may lead an individual to be somewhat critical of his or her alternatives and decisions, this tendency may be more strongly related to an adaptive form of perfectionism (e.g., making the most of one’s alternatives) than to a maladaptive form of perfectionism (e.g., striving for the perfect alternative; Reference Roets, Schwartz and GuanRoets et al., 2012; see also Reference Rim, Turner, Betz and NygrenRim et al., 2011). Instead, it may be the seemingly endless search for alternatives and the tiresome decision-making process that are maladaptive (Reference Nenkov, Morrin, Ward, Schwartz and HullandNenkov et al., 2008; Reference Rim, Turner, Betz and NygrenRim et al., 2011). Specifically, maximizers may sacrifice resources such as time and effort in their extensive search for alternatives, leading them to subsequently regret not merely the unselected alternatives but also the lost time and effort (Reference Dar-Nimrod, Rawn, Lehman and SchwartzDar-Nimrod et al., 2009). Maximizers may also find their decision-making process tedious: they may be unable to commit to their chosen alternatives, they may continually wish to retain the possibility of revisiting choices, and they may not experience the post-choice cognitive dissonance that would have permitted them to (over)value and therefore be satisfied with their choices (Reference Sparks, Ehrlinger and EibachSparks et al., 2012).
Yet, regardless of how maximizing is conceptualized (high standards versus decision difficulty and alternative search), it also seems fair to conclude, on the basis of the current results, that the construct is not a particularly strong predictor of satisfaction and performance—at least not in comparison with CSE and conscientiousness. One reason may stem from the fact that job satisfaction and job performance are broad criteria and may therefore be best predicted by broad dispositional predictors like conscientiousness and CSE (Reference Ones and ViswesvaranOnes & Viswesvaran, 1996). Future researchers interested in using maximization as a predictor in workplace settings may therefore wish to consider focusing on narrower criteria, such as the time and effort employees expend in generating alternatives in cases when they have complete autonomy to make workplace decisions (e.g., Reference Dar-Nimrod, Rawn, Lehman and SchwartzDar-Nimrod et al., 2009), the number of alternatives employees typically generate in such cases (e.g., Reference PolmanPolman, 2012), and the extent to which employees continually revisit their decisions in such cases (e.g., Reference Sparks, Ehrlinger and EibachSparks et al., 2012).
4.1 Limitations and additional suggestions for future research
As with all research, this study contains several limitations. First, unlike the other predictor and control variables, which were measured prior to the measurement of the criteria, conscientiousness was measured at the same time as the criteria so as to balance the length of the two waves of the survey. Although personality scores would not be expected to change appreciably over a three-week period in a sample of adults (Reference Costa and McCraeCosta & McCrae, 1986), future research should measure all predictor variables at a time prior to the collection of dependent variables.
Second, the composite maximizing score as well as the three dimension scores from the Reference Nenkov, Morrin, Ward, Schwartz and HullandNenkov et al. (2008) scale exhibited reliability levels that are generally considered unacceptably low (see Reference Lance, Butts and MichelsLance et al., 2006). Importantly, however, the reliabilities for the Nenkov et al. scale obtained in the current study were very similar to those reported by Nenkov et al. themselves across several independent samples. We therefore call for future research to continue to refine this scale in order to increase reliability (albeit without adding highly redundant items; Reference CattellCattell, 1978).
Third, during the course of this study (but after the data were collected), yet another maximizing scale—the Maximizing Inventory (Reference Turner, Rim, Betz and NygrenTurner et al.,2012)—was published. Future research should explore whether the Maximizing Inventory predicts common workplace attitudes and performance measures.
Finally, the exclusive reliance on self-report data for the measurement of all predictors and criteria may have led to inflated predictor-criterion relationships as a result of common-method variance. Yet, job satisfaction and intentions to quit are virtually always measured using self-reports, and income is also frequently measured using self-reports. In the case of in-role performance, where supervisor-reports are sometimes used, the key question is whether using self-reports is likely to have influenced substantive conclusions (Reference EdwardsEdwards, 2008; Reference Spector, Fox, Penney, Bruursema, Goh and KesslerSpector, 2006). In our view, there is no reason to expect the relative importance of a set of predictors to be influenced by reliance on self-report data (in other words, there is no reason to expect some predictor-performance relationships to be influenced appreciably more than others; Reference Dalal, Baysinger, Brummel and LeBretonDalal et al., 2012). Future research should nonetheless replicate our results using supervisor-reports or objective measures of in-role employee performance (e.g., average handle time in a call center).
In addition to addressing these limitations, future research should continue to “import” decision-making-related individual-differences constructs from the JDM literature to the organizational literature. For example, the avoidant decision-making style (Reference Scott and BruceScott & Bruce, 1995) may predict work withdrawal (Reference Spector, Fox, Penney, Bruursema, Goh and KesslerSpector et al., 2006), and decision-making competence (Reference Bruine de Bruin, Parker and FischhoffBruine de Bruin et al., 2007) may predict several forms of counterproductive/deviant work behavior (Reference Spector, Fox, Penney, Bruursema, Goh and KesslerSpector et al., 2006). Future research should also import broad individual differences constructs from the organizational literature (and the personality psychology literature) to the JDM literature. For example, consider CSE. High CSE is typically thought of in the organizational literature as a “good” thing, because it is associated with desirable scores on several criteria: for example, high job-satisfaction and performance. The JDM literature presents an opportunity to delineate the potential “dark side” of CSE by investigating whether high CSE is associated with certain forms of maladaptive decision-making (e.g., overconfidence, irrational escalation to commitment).
Appendix: Items used to measure maximizing

Note. All scales elicit extent of agreement on a 1-7 scale. Instructions for all scales were: “Please rate how much you agree with the following statements.”
* Because this item referenced job satisfaction (one of the criterion variables of interest), we conducted further analyses to determine whether to include it in the analyses. We found that the correlation between this item and job satisfaction was weak (r = −0.12, p = 0.059) and was close in magnitude to the median of the correlations between items on this scale and job satisfaction. Moreover, when predicting job satisfaction using a maximizing scale that did versus did not contain this item, the relative weights were identical up to two decimal places for maximizing and for all other predictors except Core Self-Evaluations (for which the rescaled weight changed by 0.01 when this item was excluded). Because this item did not change the substantive conclusions from the analyses, we decided to retain it in the scale.