1 Introduction
1.1 What is Industry 4.0?
Industry 4.0 (I4.0) is a term coined to represent the fourth industrial revolution based on the latest technological advances. While it represents an application of the concept of Cyber-Physical System (CPS) (Veera Ragavan & Shanmugavel, Reference Veera Ragavan and Shanmugavel2016), which is understood as its core (Lee et al., Reference Lee, Bagheri and Kao2015), it goes far beyond CPS, involving advanced data communication systems (Wollschlaeger et al., Reference Wollschlaeger, Sauter and Jasperneite2017), embedded intelligence (Wang et al., Reference Wang, Sun, Zhang, Thomas, Duan and Shi2016), and data semantic standardization (Fiorini et al., Reference Fiorini, Bermejo-Alonso, Goncalves, Freitas, Olivares Alarcos, Olszewska, Prestes, Schlenoff, Ragavan, Redfield, Spencer and Li2017).
I4.0, which was initiated at the beginning of this decade by national programs (Haupert et al., Reference Haupert, Bergweiler, Poller and Hauck2014) called Smart Manufacturing Leadership CoalitionFootnote 1 in the US and Industrie 4.0 Footnote 2 in Germany, has already proven to be a no-way-back trend that has the potential to take today’s Industry to a higher level of efficiency, performance, and productivity, as started to be used by companies such as ABB and Siemens (Drath & Horch, Reference Drath and Horch2014).
Indeed, I4.0 scenarios can present, for example, physical objects manipulated by means of their virtual representations which by their turn provide services that, at the end, support applications for highly detailed product customization, precise and timely accurate logistics supply chains, and efficient product delivery. Everything related to the production could be represented in the cyberspace, from the smallest and least significant raw material or component up to the complete product and all the machinery involved in its production (Rosen et al., Reference Rosen, Wichert, Lo and Bettenhausen2015). This setup relies on fast and efficient data transmission, supported by wireless communication technologies such as 5G (Rappaport et al., Reference Rappaport, Sun, Mayzus, Zhao, Azar, Wang, Wong, Schulz and Samimi2013), in which product subsystems could decide autonomously their best and most optimized production process, concurrently exchanging data with other components and elements of the industrial environment.
Hence, in an I4.0 scenario, the manufacturing process is the main activity and, among several equipments, autonomous robots are extensively used toward manufacturing performance and revenue improvements (Kattepur et al., Reference Kattepur, Dey and Balamuralidhar2018; Zhang et al., Reference Zhang, Li, Nicho, Ripperger, Fumagalli and Veeraraghavan2019). This helps to explain why power consumption related to motors represents two tier of the electrical power consumed by the industry sector (Saidur, Reference Saidur2010). Combined with currently available techniques of data analysis and cognition, this creates new possibilities of interoperability, modularity, distributed processing, and integration in real time with other systems for industrial processes. In fact, those possibilities constitute the core concept of I4.0 (Hermann et al., Reference Hermann, Pentek and Otto2016).
1.2 Technologies for Industry 4.0
I4.0 or smart factory (Kannengiesser & Muller, Reference Kannengiesser and Muller2013) is based on new and radically changed processes in manufacturing industry. It represents a number of contemporary automation, data exchange, and manufacturing technologies (Hermann et al., Reference Hermann, Pentek and Otto2016), such as virtual enterprise (Smirnov et al., Reference Smirnov, Sandkuhl and Shilov2010), cloud manufacturing (Xie et al., Reference Xie, Cai, Xu, Jiang and Bu2017), Internet of Things (IoT), also named by Cisco as Internet of Everything (Zheng et al., Reference Zheng, Martin, Brohman and Xu2014), and its emerging concepts Industrial Internet of Things (IIoT) (Civerchia et al., Reference Civerchia, Bocchino, Salvadori, Rossi, Maggiani and Petracca2017) or Industrial Internet as used in the US by General Electric (GE) to represent the realization of IoT for industrial applications.
In particular, data are gathered from suppliers, customers, and the plant/factory itself and evaluated before being linked up with real production. The latter is increasingly using new technologies such as data analytics, smart sensors, cloud computing, and next-generation robots (Haidegger et al., Reference Haidegger, Galambos and Rudas2019). This results in flexible and adaptive production processes that are fine-tuned, adjusted, or set up differently in real time (Hermann et al., Reference Hermann, Pentek and Otto2014).
Traditional industry relies on ISA-95, a well-defined five-layer automation architecture. The machine level (i.e. field devices such as sensors and actuators) is at the lowest level and sends/receives data via digital or analog signals to the control level, for example, the Programmable Logic Controller. Supervisor Control and Data Acquisition systems in the cell level perform (remote) control tasks. Manufacturing Execution Systems in the process control level allow users to perform complex tasks such as production scheduling. Top-level Enterprise Resource Planning or factory operation management level allows the management reporting and shares manufacturing data such as the order status with other systems (Wollschlaeger et al., Reference Wollschlaeger, Sauter and Jasperneite2017).
The fourth industrial revolution I4.0 represents a new paradigm shift from the centralized to the decentralized industry, relying on the cyber-physical-based automation, where sensors send data directly to the cloud and where services such as monitoring, control, and optimization automatically subscribe to the necessary data in real time. Hence, I4.0 involves flexible production networks that require horizontal integration across the company, while any production-related information exchanged in the network must be vertically forwarded to the corresponding service endpoint of the local production system (Wally et al., Reference Wally, Huemer and Mazak2017). The ultimate goal of this emerging technology is to improve the work conditions and to increase productivity, speed, precision, repeatability, reliability, flexibility, and competitiveness. In the coming years, these technologies will be seen as a viable alternative to the current manufacturing processes, and will enable mass customization, faster production, better quality, increased productivity, and improved decision-making (Da Xu et al., Reference Da Xu, He and Li2014).
It is worth noting that mass customization can allow the production of small lots at reasonable cost, due to the ability to rapidly configure machines in order to adapt to the customer-supplied specifications and additive manufacturing (Wang et al., Reference Wang, Sun, Zhang, Thomas, Duan and Shi2016). On the other hand, data-driven supply chains can speed up the manufacturing process by an estimated 120% in terms of time needed to deliver orders and by 70% in time to get products to market (Davies, Reference Davies2015). Thence, I4.0 technologies aim to improve the product quality and dramatically reduce the costs of scrapping or reworking defective products. Predictive maintenance and self-healing technologies in I4.0 intend to enable plants/factories to keep running in order to guarantee the productivity. I4.0 technologies could allow individuals and companies to share access to products, services, and experiences, enabling ‘sharing economy’ as a new business model. With access to factory and cross-market data, decision-makers can predict, response, and adapt to factory needs and market trends in an accurate and timely manner. Some estimates indicate that smart factory technology will have global market size of USD 62.98 billion by 2019 and USD 74.80 billion by 2022 (Markets and Markets, Reference Markets and Markets2016).
1.3 Challenges of Industry 4.0
I4.0 is opening the door for a new industrial revolution. In order to understand the contributions and challenges of I4.0, and how it will influence life at different levels of development, it is important to keep in mind how revolutionary industrial changes took place and their contribution to the evolution of technology since the first industrial revolution. Britain was the birthplace of the first technological revolution which emerged in the late 18th century with the invention of the steam engine and the introduction of new mechanical production facilities. The second industrial revolution encompassed at the end of the 19th century the development of electrical, chemical, and motor-vehicle engineering sectors, while the third industrial revolution came up with developments in the electronic and aerospace sectors, leading to the omnipresence of Information Technology systems and production automation (MacDonald, Reference MacDonald2016).
The fourth industrial revolution is initiating the use of CPS (Lee et al., Reference Lee, Bagheri and Kao2015) and is focused on the development of a new generation of intelligent and integrated technologies for smart manufacturing (Ivezic & Ljubicic, Reference Ivezic and Ljubicic2016), seeking to optimize its planning and usage across different industrial domains such as oil and gas industry (Du et al., Reference Du, Li, Shang and Wu2010), (Guo & Wu, Reference Guo and Wu2012), mining (Xue & Chang, Reference Xue and Chang2012), energy (Teixeira et al., Reference Teixeira, Silva, Pinto, Santos, Praca and Vale2017), steel production (Dobrev et al., Reference Dobrev, Gocheva and Batchkova2008), construction (Sorli et al., Reference Sorli, Mendikoa, Perez, Soares, Urosevic, Stokic, Moreira and Corvacho2006), aviation (Hoppe et al., Reference Hoppe, Eisenmann, Viehl and Bringmann2017; Lehmann et al., Reference Lehmann, Heussner, Shamiyeh and Ziemer2018), automotive industry (Phutthisathian et al., Reference Phutthisathian, Maneerat, Varakulsiripunth, Takahashi and Kato2013), electronic industry (Liu et al., Reference Liu, Wang, Morris and Kristiansen2005a), chemical industry (Natarajan et al., Reference Natarajan, Ghosh and Srinivasan2011), and process engineering (Wiesner et al., Reference Wiesner, Saxena and Marquardt2010). In addition, the concept of virtual production is considered to be the key factor for modeling production aiming for zero defects (MacDonald, Reference MacDonald2016).
Hence, the driving force behind the development of I4.0 is the rapidly increasing digitization of the economy and society, in the sectors of agriculture (Jayarathna & Hettige, Reference Jayarathna and Hettige2013), production (Meridou et al., Reference Meridou, Kapsalis, Papadopoulou, Karamanis, Patrikakis, Venieris and Kaklamani2015), and services, for example, banking (Atkinson et al., Reference Atkinson, Cuske and Dickopp2006), telecom (Agrawal et al., Reference Agrawal, Chafle, Goyal, Mittal and Mukherjea2008), tourism (Fang et al., Reference Fang, Jiaming, Yaohui and Mei2016), or insurance (Koetter et al., Reference Koetter, Blohm, Kochanowski, Goetzer, Graziotin and Wagner2019).
I4.0 integrates also the state of the art of communication technologies such as cloud (Xu, Reference Xu2012; Xie et al., Reference Xie, Cai, Xu, Jiang and Bu2017), IoT (Cagnin et al., Reference Cagnin, Guilherme, Queiroz, Paulo and Neto2018; Wan et al., Reference Wan, Chen, Imran, Tao, Li, Liu and Ahmad2018a) with the new trends of evolved intelligent industrial technologies, such as new-generation intelligent agents (Kannengiesser & Muller, Reference Kannengiesser and Muller2013), Internet of Robotics Things (Ray, Reference Ray2016), Augmented Reality. and Virtual Realty (Flatt et al., Reference Flatt, Koch, Rocker, Gunter and Jasperneite2015; Ivaschenko et al., Reference Ivaschenko, Khorina and Sitnikov2018).
Despite the benefits and advances promised by I4.0, the players in this arena have a wide range of challenges to cope with, from human–robot interaction (Jost et al., Reference Jost, Kirks and Mattig2017; Calzado et al., Reference Calzado, Lindsay, Chen, Samuels and Olszewska2018) to data analysis (Xu & Hua, Reference Xu and Hua2017; Li & Niggemann, Reference Li and Niggemann2018). On the other hand, wireless communication are also an important factor in I4.0. With 5G networks still under development (Nordrum & Clark, Reference Nordrum and Clark2017), other wireless technologies are being adopted in the meantime, leading to the need for networks’ coexistence solutions (de Moura Leite et al., Reference Leite, Canciglieri, Szejka and Canciglieri2017). Furthermore, I4.0 requires the understanding of data heterogeneity in the context of CPSs integration (Jirkovsky et al., Reference Jirkovsky, Obitko and Marik2017; Matzler & Wollschlaeger, Reference Matzler and Wollschlaeger2017) as well as the interoperability (Salminen & Pillai, Reference Salminen and Pillai2007; Nilsson & Sandin, Reference Nilsson and Sandin2018) within the agent-based ecosystem (Kao & Chen, 2010) for unambiguous communication (Zhang et al., Reference Zhang, Ahmad, Vera and Harrison2018), efficient collaboration (Olszewska, Reference Olszewska2017), and cooperation (Hildebrandt et al., Reference Hildebrandt, Scholz, Fay, Schroder, Hadlich, Diedrich, Dubovy, Eck and Wiegand2017). Thence, information and data used for smart manufacturing should follow a semantic standard (Macia-Perez et al., Reference Macia-Perez, Gilart-Iglesias, Ferrandiz-Colmeiro, Berna-Martinez and Gea-Martinez2009) throughout the whole industrial environment.
In particular, ontologies are a powerful solution to capture (Liandong & Qifeng, Reference Liandong and Qifeng2009) and to share the common knowledge (Hoppe et al., Reference Hoppe, Eisenmann, Viehl and Bringmann2017) among the distributed partners of the I4.0 technology, leading, for example, to Context-as-a-Service platforms (Hassani et al., Reference Hassani, Medvedev, Haghighi, Ling, Indrawan-Santiago, Zaslavsky and Jayaraman2018). Indeed, ontologies aim to make domain knowledge explicit and remove ambiguities, enable machines to reason, and facilitate knowledge sharing between machines and humans (Persson & Wallin, Reference Persson and Wallin2017) and in between machines (Olszewska & Allison, Reference Olszewska and Allison2018). Moreover, ontologies for the I4.0 are required to be business focused, that is promoting cooperation with customers and partners (Persson & Wallin, Reference Persson and Wallin2017) and, on the other hand, meet ontological, autonomous robotic requirements (Bayat et al., Reference Bayat, Bermejo-Alonso, Carbonera, Facchinetti, Fiorini, Goncalves, Jorge, Habib, Khamis, Melo, Nguyen, Olszewska, Paull, Prestes, Ragavan, Saeedi, Sanz, Seto, Spencer, Vosughi and Li2016). Furthermore, ontologies need to analyze and reuse domain knowledge by using present ontologies (Persson & Wallin, Reference Persson and Wallin2017).
Focusing on the abovementioned characteristics, this paper approaches the I4.0 theme through an ontological perspective, meeting the mandatory requirement of consistent and standardized data semantics. The goal of our work is to contribute toward the effort of unambiguously representing domain knowledge in order to assist I4.0 practitioners in the development of coherent and efficient systems. The contribution proposed in this paper is an ontological perspective of the I4.0 domain, with a highlight on the autonomous robotic facet of I4.0.
The rest of the paper is structured as follows. Section 2 presents existing ontologies for the I4.0 domain, along with a literature overview about relevant standardization efforts in the smart manufacturing field, with an emphasis on its autonomous robotic aspect. Section 3 describes real-world case studies providing potential applications for the use of I4.0 ontologies, while Section 4 concludes the work with reflections and future directions.
2 Industry 4.0 ontologies
2.1 Industry 4.0 ontological frameworks
Ontologies consist in a formal conceptualization of the knowledge representation and provide the definitions of the concepts and relations capturing the knowledge of a domain in an interoperable way (Wang et al., Reference Wang, Wong and Wang2010).
The domain of I4.0 or Factory 4.0 or Smart Manufacturing consists of concepts related, on the one hand, to business services (Wally et al., Reference Wally, Huemer and Mazak2017), encompassing automatization of the project management (Martin-Montes et al., Reference Martin-Montes, Burbano and Leon2017), organizational management (Izhar and Apduhan, Reference Izhar and Apduhan2017), customer satisfaction management (Kim and Lee, Reference Kim and Lee2013; Daly et al., Reference Daly, Grow, Peterson, Rhodes and Nagel2015), risk management (Atkinson et al., Reference Atkinson, Cuske and Dickopp2006), and virtualization of operations (Jiang et al., Smirnov et al., Reference Jiang, Peng and Mair2004; Reference Smirnov, Sandkuhl and Shilov2010), such as billing (Agrawal et al., Reference Agrawal, Chafle, Goyal, Mittal and Mukherjea2008), ticketing (Vukmirovic et al., Reference Vukmirovic, Szymczak, Ganzha and Paprzycki2006), generation of recommendations (Lorenzi et al., Reference Lorenzi, Loh and Abel2011), and decision-making aids (Koetter et al., Reference Koetter, Blohm, Kochanowski, Goetzer, Graziotin and Wagner2019).
On the other hand, production services (Wally et al., Reference Wally, Huemer and Mazak2017) involve abstractions of manufacturing processes (Brodsky et al., Reference Brodsky, Krishnamoorthy, Bernstein and Nachawati2016; Tang et al., Reference Tang, Li, Wang and Dong2018), such as production management (Yusupova et al.), product compliance (Disi & Zualkernan, Reference Disi and Zualkernan2009), resource reconfiguration (Wan et al., Reference Wan, Yin, Li, Celesti, Tao and Hua2018b), decision support (Arena et al., Reference Arena, Kiritsis, Ziogou and Voutetakis2017), and intelligence-based automatization of chain processes (Muller et al., Reference Muller, Hagenmeyer, Schmidt, Scholz and Elkaseer2018), such as assembly (Merdan et al., Reference Merdan, Koppensteiner, Zoitl and Hegny2008; Cecil et al., Reference Cecil, Albuhamood and Cecil-Xavier2018) and/or diassembly (Koppensteiner et al., Reference Koppensteiner, Hametner, Paris, Passani and Merdan2011), packaging (Wan et al., Reference Wan, Tang, Li, Imran, Zhang, Liu and Pang2019), shipping (Phutthisathian et al., Reference Phutthisathian, Maneerat, Varakulsiripunth, Takahashi and Kato2013) as well as system diagnosis (Bunte et al., Reference Bunte, Diedrich and Niggemann2016), product control (Bunte et al., Reference Bunte, Diedrich and Niggemann2016), safety control (Akbari et al., Reference Akbari, Setayeshmehr, Borsi, Gockenbach and Fofana2010), and security inspection (Mozzaquatro et al., Reference Mozzaquatro, Melo, Agostinho and Jardim-Goncalves2016).
For this purpose, in the last decade, ontologies have been developed for one specific industrial domain such as aviation (Keller, Reference Keller2016), aeropsace (Kossmann et al., Reference Kossmann, Gillies, Odeh and Watts2009), construction (Liao et al., Reference Liao, Liu and Zhao2009), steel production (Dobrev et al., Reference Dobrev, Gocheva and Batchkova2008), chemical engineering (Vinoth & Sankar, Reference Vinoth and Sankar2016; Feng et al., Reference Feng, Chen, Chen, Chen and Peng2018), oil industry (Du et al., Reference Du, Li, Shang and Wu2010; Guo & Wu, Reference Guo and Wu2012), energy (Santos et al., Reference Santos, Silva, Teixeira, Vale and Pinto2018), and electronics (Liu et al., Reference Liu, Wang, Morris and Kristiansen2005a). Other ontologies have been used for one specific manufacturing process such as packaging (Liu et al., Reference Liu, Wang, Morris and Kristiansen2005b), process engineering (Wiesner et al., Reference Wiesner, Saxena and Marquardt2010), process compliance (Disi & Zualkernan, Reference Disi and Zualkernan2009), risk management (Atkinson et al., Reference Atkinson, Cuske and Dickopp2006), safety management (Hooi et al., Reference Hooi, Hassan and Ci2012), customer feedback analysis (Kim and Lee, Reference Kim and Lee2013; Daly et al., Reference Daly, Grow, Peterson, Rhodes and Nagel2015), organizational management (Grangel-Gonzalez et al., Reference Grangel-Gonzalez, Halilaj, Auer, Lohmann, Lange and Collarana2016; Izhar and Apduhan, Reference Izhar and Apduhan2017), project management (Cheah et al., Reference Cheah, Khoh and Ooi2011), product development (Zhang et al., Reference Zhang, Zhou and Lu2017), maintenance (Haupert et al., Reference Haupert, Bergweiler, Poller and Hauck2014), resource reconfiguration (Wan et al., Reference Wan, Yin, Li, Celesti, Tao and Hua2018b), and production scheduling (Kourtis et al., Reference Kourtis, Kavakli and Sakellariou2019). Ontologies have also been focused on one service, for example, ticketing (Vukmirovic et al., Reference Vukmirovic, Szymczak, Ganzha and Paprzycki2006), or on one manufacturing concept, for example, information flow (Bildstein and Feng, Reference Bildstein and Feng2018), information security (Mozzaquatro et al., Reference Mozzaquatro, Melo, Agostinho and Jardim-Goncalves2016), and data integration (Yusupova et al.).
More recently, two ontological frameworks tending to cover the wider domain of smart manufacturing have been proposed. Hence, Cheng et al. (Reference Cheng, Zeng, Xue, Shi, Wang and Yu2016) provided a model of the production line using a combination of five ontologies, namely, device ontology (with concepts such as Machine), process ontology (with a taxonomy of the different Operations performed by the technical equipment), parameter ontology (with concepts such as Quality of Service), product ontology (with the product information), and the base ontology (integrated the four others and defining the concept Order). On the other hand, Engel et al. (Reference Engel, Greiner and Seifert2018) proposed a three-layer ontology for batch process plants. The first layer, or application layer, contains the operations; the second layer, or domain layer, the architecture, while the third layer, or upper layer, refers to an upper ontological model describing general system characteristics and relations.
These ontologies have been proven to bring some advances in the field, but they have a limited scope and/or a basic vocabulary. Hence, the effort to standardize the whole domain is a huge enterprise, and some current results of this standardization work are reported in Section 2.2.
2.2 Industry 4.0 ontological standards
2.2.1 Ontological standard effort
As I4.0 relies heavily on robotic agents which have to evolve and perform the main operations in smart manufacturing environment and which are solicited to communicate with human operators, customers, or with diverse distributed partners, the standardization of knowledge representation is a key element facing I4.0 development and is required to be addressed quickly and efficiently to avoid accumulated difficulties at later stages of the development. Hence, the ontological standardization effort for I4.0 builds upon the IEEE 1872-2015 Standard Ontologies for Robotics and Automation (IEEE-SA, Reference IEEE-SA2015), which establishes a series of ontologies about the Robotics and Automation (R&A) domain (Fiorini et al., Reference Fiorini, Bermejo-Alonso, Goncalves, Freitas, Olivares Alarcos, Olszewska, Prestes, Schlenoff, Ragavan, Redfield, Spencer and Li2017) that can be extended to the I4.0. by incorporating new I4.0-specific ontological concepts, as described in the next paragraphs.
CORA Ontology. The Core Ontology for Robotics and Automation (CORA) (Prestes et al., Reference Prestes, Carbonera, Fiorini, Jorge, Abel, Madhavan, Locoro, Goncalves, Barreto, Habib, Chibani, Gerard, Amirat and Schlenoff2013) developed within the IEEE 1872-2015 Standard Ontologies for Robotics and Automation (IEEE-SA, Reference IEEE-SA2015) is a core ontology for robotics. A core ontology specifies concepts that are general in a whole domain such as Robotics. In the case of CORA, it defines concepts such as Robot, Robot Group, and Robotic System. Its role is to serve as basis for other more specialized ontologies in R&A, currently developed within IEEE P1872.1 and P1872.2 standardization efforts, and focused on Robot Task Representation and Autonomous Robotics, respectively. Moreover, it determines a set of basic ontological commitments, which should help robot developers and other ontologists to create models about robots (Bayat et al., Reference Bayat, Bermejo-Alonso, Carbonera, Facchinetti, Fiorini, Goncalves, Jorge, Habib, Khamis, Melo, Nguyen, Olszewska, Paull, Prestes, Ragavan, Saeedi, Sanz, Seto, Spencer, Vosughi and Li2016).
ROA Ontology. The Ontology for Autonomous Robotics (ROA) (Olszewska et al., Reference Olszewska, Barreto, Bermejo-Alonso, Carbonera, Chibani, Fiorini, Goncalves, Habib, Khamis, Olivares, Freitas, Prestes, Ragavan, Sanz, Spencer and Li2017) defines robotic notions identified as fundamental (Ivezic & Ljubicic, Reference Ivezic and Ljubicic2016) for Autonomous Robotics. Hence, ROA provides the definitions of behavior, function, goal, and task concepts and reuses ontologies such as the Suggested Upper Merged Ontology (SUMO) upper ontology, the CORA core ontology, and specialized ontologies such as the Spatio-Temporal Visual Ontology (Olszewska, Reference Olszewska2011).
ORArch Ontology. The Ontology for Robotic Architecture (ORArch) elaborates notions related to hardware and software, as well as how these can be represented together in mixed architecture descriptions. Moreover, ROA aims to allow one to describe multiple architectural viewpoints of the same robot, which combines hardware and software devices.
Figure 1 depicts the main concepts of this ontology. The top concepts are part of the top-level ontology. The ontology divides the reality as endurants, perdurants, and abstracts. Endurant and perdurants are entities that are situated in time, while abstract entities are not. Perdurants have temporal parts like processes and events, while endurants have no temporal parts such as physical and social objects. Abstract entities are formal entities, for example, the logical and mathematical entities.
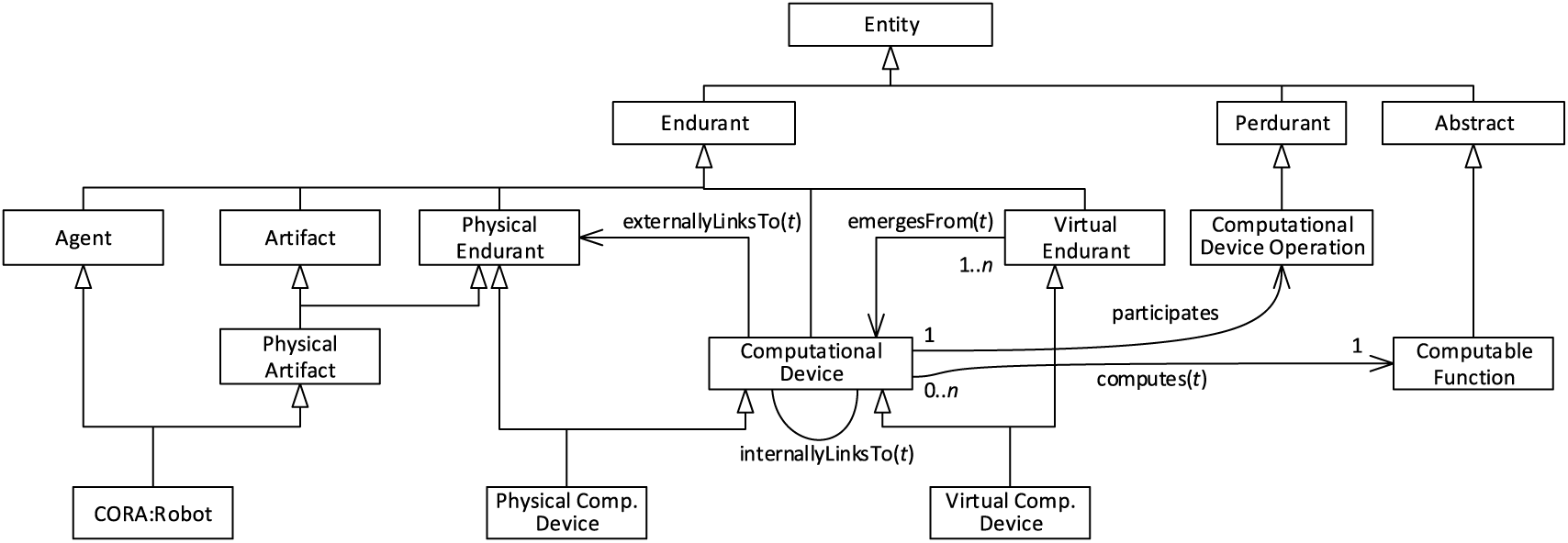
Figure 1 Ontological concepts of robotic hardware and software as conceived in ORArch and O4I4 ontologies
The main aspect of this ontology is the separation between physical and virtual endurants. Physical endurants are objects of everyday life. Virtual endurants emerge from computational devices in operation. Computation devices are entities that perform the computation of a computable function. Examples of virtual endurants are typical entities related to running software (e.g. processes, threads, components, objects, and procedures) and other virtual-reality entities.
The ontology also imports the notion of Robot from CORA. We introduced some concepts (and axiomatization) such as Artifact to align its meaning with CORA/SUMO.
The concepts dealing with the architecture are shown in Figure 2. ORArch includes descriptions and situations (DnS) ontology to describe the robot architecture. DnS allows the representation of descriptions without the need for second-order languages. It has two main concepts, namely Description and Situation. A Situation is an entity similar to a collection which aggregates (i.e. is setting for) some entities that should be taken into consideration together for a given reason. An example of situations is the plant or a navigation context for a robot. A situation satisfies one or more descriptions. A Description defines concepts and roles that classify elements of a situation. A description of a plant would define the concepts of type A product and type B product, which classify instances of products. A description of a robot context defines concepts such as objective and obstacle, which classify object and regions in different situations. It is important to note that instances of a concept are distinct of the concepts that form the ontology itself. Let’s consider, for example, the concept Mobile Robot. It might appear in the ontology as a subclass of CORA:Robot and also an instance of RobotType. Both these entities are treated as different. In ORArch, we consider that the notion of robot architecture has two sides. It can refer to a selection of components in a given, constructed robot, and it can also refer to an architectural model or description of an architecture that might be present in different robots. These two notions are captured by the concepts (Robot Architecture) Viewpoint and (Robot Architecture) Description in Figure 2.

Figure 2 Concepts about robot architecture viewpoint and description in ORArch
O4I4 Ontology. The Ontology for Industry 4.0 (O4I4) is dedicated to capture the I4.0-specific domain concepts, while reusing CORA, ROA, and ORArch ontologies for the robotic facet of smart manufacturing. It is worth noting that CORA used SUMO as the upper ontology. However, in the light of the requirements of the suite of standardization ontologies (Fiorini et al., Reference Fiorini, Bermejo-Alonso, Goncalves, Freitas, Olivares Alarcos, Olszewska, Prestes, Schlenoff, Ragavan, Redfield, Spencer and Li2017), it is planned that SUMO becomes optional as a top-level ontology in P1872.2. One reason is that some users of IEEE 1872 (IEEE-SA, Reference IEEE-SA2015) voiced their desire to use CORA with other top-level ontologies. On the one hand, SUMO is too big and complex for customizable projects. Hence, with O4I4 which aims to be a business-focused ontology, we began defining a minimal top-level ontology to support our development. Such top-level ontology is also optional, but also should be easier to map to other top-level ontologies, if need be.
The new I4.0-specific concepts appear in Figure 1 and their definition is as follows:
• Computable Function is an abstract entity representing a given computable function with defined input(s) and output(s).
• Computational Device Operation is a perdurant denoting the functioning of a computational device.
Moreover, in the O4I4 ontological standard, the concept of Computable Service is defined as a Computational Device Operation which captures the notion of the process in which an agent has to compute an external request (with a possible input) and to deliver a result (output). However, a computable service can only exist if the agent has the Capability of performing that service which includes the availability of a Physical Computational Device. Thence, the computable service exists from the moment in which the requester starts being served and not from the moment in which the agent is requested. It is worth noting that a computable service is a sort of service from a computational science point of view. Other classes of services could be developed in the future to cover notions related to robotic service, etc.
2.2.2 Ontological standard roadmap
To sum up, the standard design using formal models consists of (i) the development of standard vocabularies for robotic concepts; (ii) the development of a functional ontology for Autonomous Robotics; (iii) the validation of relationship using functions as a basis for relationship checking; and (iv) the use of developed vocabularies and ontologies for I4.0 applications.
The benefits of such design are twofold. On the one hand, academics can discuss concepts unambiguously on the topic which will pave way for further research and investigation on the topic (Bermejo-Alonso et al., Reference Bermejo-Alonso, Chibani, Goncalves, Li, Jordan, Olivares, Olszewska, Prestes, Fiorini and Sanz2018). On the other hand, Industry practitioners can use these ontologies to conceptualize implementation scenarios (Olszewska et al., Reference Olszewska, Houghtaling, Goncalves, Fabiano, Haidegger, Carbonera, Patterson, Ragavan, Fiorini and Prestes2019). Indeed, as every scenario considered within the framework of the I4.0 includes different entities which communicate and cooperate with each other, the main role of the presented ontological standard is to facilitate that exchange, as exemplified in Section 3.
3 Industry 4.0 scenarios
3.1 Smart-rapid prototyping scenario
In I4.0, 3D printing manufacturing is a key-technology enabler for smart factories. This technology is also known as rapid prototyping, digital fabrication, solid imaging, free-form fabrication, layer-based manufacturing, and laser prototyping. The process involves building prototypes or working models in a relatively short time to help the creation and the testing of various design features, ideas, concepts, functionalities, and in certain instances, the outcome and performance (Bagaria et al., Reference Bagaria, Rasalkar, Ilyas and Bagaria2011). Nowadays, there is a growing need and expectation of more rapid bespoke production, in order to both deliver the rapid prototyping of more products and variants and to support specialist products and obsolete parts globally and locally. Rapid prototyping provides a viable way to quickly and cost effectively deliver components or complete products as well as decrease the holding and transporting stock (and obsolescence concerns) (Burke et al., Reference Burke, Mussomeli, Laaper, Hartigan and Sniderman2015).
In a smart-rapid prototyping scenario (Figure 3), a customer with a predefined profile accesses a Web service to send a query to the manufacturing facility. This query contains the specifications of the part to be manufactured by the smart-rapid prototyping facility including the digital model uploaded by the customer or selected from an online digital model repository, as well as the material, the color, and the number of required units. The customer’s query is then parsed and directed to the rapid prototyping unit that generates or retrieves the solid model to be sent to the manufacturing modeler that creates the 3D physical model. Post-processing such as surface finishing is then applied to create the final prototype that is shipped to the customer via logistic 4.0 technologies such as connected trucks, autonomous ground/aerial vehicles. Moreover, the customer is able to track all the manufacturing steps from the receipt of the request to the delivery of the final prototype.

Figure 3 Overview of the smart-rapid prototyping scenario
In this scenario, the exchange of information and resources among those entities becomes crucial to obtain a good performance of the system as a whole, and the ontological approach can facilitate this exchange of information through the use of the defined concepts like Computable Function, Computational Device Operation, and Computable Service. Furthermore, the use of O4I4 ontology contributes toward the uniformization of the attributes required within the process as well as their unambiguous interpretation by both the machines and the customer.
3.2 UAV’s good delivery scenario
Another crucial element of I4.0 is the efficient good delivery. Thence, let’s consider a scenario where an operator has to supervise goods’ delivery via unmanned aerial vehicles (UAVs), assigning different UAVs to different delivery tasks. These UAVs have a fault detection system that can detect and inform the operator about degradation in performance. Based on that information, the operator has to infer if that particular drone can be kept in operation or it has to be brought back for maintenance. This kind of reasoning requires considerable amount of expertise, since it has to be precise and relatively quick. This might hinder the adoption of UAVs by non-specialized business, such as pizza delivery, for instance.
An ontological approach can help this type of human–robot systems in many aspects, and as a consequence, enables the business to grow. For example, the ROA ontology (Olszewska et al., Reference Olszewska, Barreto, Bermejo-Alonso, Carbonera, Chibani, Fiorini, Goncalves, Habib, Khamis, Olivares, Freitas, Prestes, Ragavan, Sanz, Spencer and Li2017) (described in Section 2.2) provides formal concepts such as Task, Function, and Behavior as well as spatio-temporal relations. In this scenario, this can aid, on the one hand, the robot to unambiguously communicate the status information about itself to a human operator and, on the other hand, this can aid the operator’s decision-making. Indeed, through automated reasoning, the robotic system can display more meaningful and simpler information. For instance, let’s consider that the malfunctioning UAV was designed with the function of delivering packages in confined places, such as corridors. As its motors degrade, it starts to display different behaviors, such as small, but sudden changes in its trajectory, which it is able to correct if enough space is available. In a non-intelligent system, the operator alone has to check if the displayed behavior is compatible with the designed function of the robot and decide about grounding it or not. Depending on the knowledge or workload of the operator, these can become expansive and/or dangerous operations. With an ontology representing the robot architecture, the system can autonomously classify the erratic movements and infer whether they fulfill the designed function of delivering pizza. This system can then inform the user directly of this fact, unloading the operator of having to decode low-level warning signals and decide the best course of action, which improves the operator’s general situation awareness.
4 Conclusions
The use of robotic agents in context of I4.0 has triggered, among others, the need to develop an interoperable communication model to interconnect them efficiently. Hence, an unambiguous, semantic-based knowledge representation of concepts for smart manufacturing domain is required to ensure a coherent and effective human–robot collaboration. For this purpose, ontologies have been identified as a possible solution for the representation of the vocabulary describing the key concepts related to this fourth industrial revolution. Thence, this paper presents the current state of ontologies for I4.0 and reviews both existing ontological frameworks and ontological standardization efforts in that field. Moreover, illustrative I4.0 scenarios have been provided to raise the awareness of practitioners about the potential of using ontologies for I4.0.
Acknowledgements
S. Veera Ragavan was supported by Monash University and MOHE grant FRGS/1/2015/TK08/ MUSM/02/1, entitled ‘Towards a model synthesis framework for conceptual modeling of cyber-physical systems’. Paulo J. S. Goncalves was partially supported by FCT, through IDMEC, uner LAETA, project UID/EMS/50022/2109 and project 0043-EUROAGE-4-E (POCTEP Programa Interreg V-A Spain-Portugal). J.I. Olszewska was partially supported by Innovate UK.