INTRODUCTION
When a technological area or a nascent industry is emerging, a group of competing firms will face multiple technological trajectories. In the presence of uncertainty about the potential gains of these trajectories, firms acquire information through both intra-firm experimentation and inter-firm imitation (Levitt & March, Reference Levitt and March1988; March, 1991; Wu, Wan, & Levinthal, Reference Wu, Wan and Levinthal2014). Intra-firm experimentation refers to the independent experiential learning from trial and error (Cyert & March, 1963; Denrell & March, Reference Denrell and March2001; Holmqvist, Reference Holmqvist2004), which exposes firms to distinct experiences and outcomes, giving rise to variations in choices in the industry (Lavie & Rosenkopf, Reference Lavie and Rosenkopf2006; McKnight & Zietsma, Reference McKnight and Zietsma2018). By experimentation, some firms may differentiate themselves from peers by finding the well-performing trajectories and thereby achieve competitive advantages (Benner & Tushman, Reference Benner and Tushman2013; Raisch, Birkinshaw, Probst, & Tushman, Reference Raisch, Birkinshaw, Probst and Tushman2009). Pursuing differentiation, however, may trap a firm in a technological periphery that peer firms or the market do not appreciate. Thus, intra-firm experimentation without considering peers’ choices may harm firms’ performance (Stettner & Lavie, Reference Stettner and Lavie2014). In contrast to experimentation, firms can also apply inter-firm imitation, which is also referred to as vicarious learning in the literature (Baum, Li, & Usher, Reference Baum, Li and Usher2000; Bruneel, Yli-Renko, & Clarysse, Reference Bruneel, Yli-Renko and Clarysse2010; Ingram & Baum, Reference Ingram and Baum1997). Imitation can greatly increase business performance by adapting quickly and efficiently, reducing uncertainties by enhancing legitimacy, and accelerating innovation diffusion and other network externalities (Haunschild & Miner, Reference Haunschild and Miner1997; Mazzucato & Mazzucato, Reference Mazzucato and Mazzucato2017).
The existence of this cross-level (intra-firm and inter-firm) learning confronts firms with two major paradoxes while orchestrating various technological alternatives. On the one hand, firms need to manage the traditional organizational ambidexterity paradox – i.e., balancing exploitation and exploration at the firm level (March, 1991; Raisch et al., Reference Raisch, Birkinshaw, Probst and Tushman2009). On the other hand, firms can balance their intra-firm learning (experimentation) and inter-firm learning (imitation), which seems to be related to the conformity-differentiation paradox for optimal distinctiveness as strategic balance (Deephouse, Reference Deephouse1999; Haans, Reference Haans2018; Zhang, Wang, & Zhou, Reference Zhang, Wang and Zhou2020; Zhao, Ishihara, Jennings, & Lounsbury, Reference Zhao, Ishihara, Jennings and Lounsbury2018; see Zhao, Fisher, Lounsbury, & Miller, Reference Zhao, Fisher, Lounsbury and Miller2017 for a review). In other words, experimentation can help a focal move ahead of rivals in the process of differentiation or divergence, while imitation can help the focal firm avoid falling behind rivals in the process of conformity or convergence.
A major gap in the extant literature is the lack of attention to the inherent link between the organizational ambidexterity paradox and the conformity-differentiation paradox. Focusing on the context of nascent industries, we seek to answer the following research question: How do firms manage the conformity-differentiation tension through cross-level learning behaviors in terms of exploration and exploitation? Specifically, we examine both the extent and from whom firms should learn to manage the conformity-differentiation paradox for the best performance. A mixed method approach is adopted to answer the research question. We first conduct a case study on the technological trajectory in the Chinese photovoltaic (PV) industry. The catch-up and growth of the Chinese multicrystalline silicon manufacturers in recent years demonstrates a clear pattern in the balance between conformity and differentiation, which is achieved through cross-level learning behaviors in interdependent technological domains along the value chain. With imitation, firms converge in the trajectory of the upstream bottleneck technologies, demonstrating conformity at the industry level. With experimentation, firms diverge across various technological trajectories for the development of distinctive products in the downstream market, demonstrating differentiation at the industry level.
To verify and extend the case findings, we draw on James March's exploitation-exploration model (Levinthal & March, Reference Levinthal and March1981; March, Reference March1991, Reference March2003) and adopt a computational experiment to reflect a learning setting in which a group of competing firms face various technological trajectories (Bitektine, Lucas, & Schilke, Reference Bitektine, Lucas, Schilke, Bryman and Buchanan2018; Raisch et al., Reference Raisch, Birkinshaw, Probst and Tushman2009). Following institutional theory and the notion of mimetic isomorphism (DiMaggio & Powell, Reference DiMaggio and Powell1983; Haunschild, Reference Haunschild1993; Haunschild & Miner, Reference Haunschild and Miner1997; Haveman, Reference Haveman1993), we conduct computational experiments in which organizations can engage in inter-firm learning using three possible imitation modes: imitate the best-performing firm (imitate the best); imitate the largest firm (imitate the big); and imitate the most-selected choice (imitate the crowd). Results suggest that while a firm can improve its performance under all three imitation modes, it achieves the best performance by learning from the firms with the best recent performance. Besides, over-reliance on imitation may deteriorate firms’ long-term performances.
The study theoretically contributes to the strategy and organization research streams by generating an important dialogue between the organizational learning literature and the optimal distinctiveness literature. First, we extend March's learning model by introducing inter-firm learning opportunities. March's original model focuses mainly on the tension between exploitation and exploration at the intra-firm level (March, 1991), but organizational interdependence is prevalent in almost any industry (Miller, Zhao, & Calantone, Reference Miller, Zhao and Calantone2006): while choosing a popular trajectory can potentially increase its return due to legitimacy, making identical choices can reduce profitability because of intensified competition (Deephouse, Reference Deephouse1999; Haans, Reference Haans2018; Haveman, Reference Haveman1993; Zhao et al., Reference Zhao, Ishihara, Jennings and Lounsbury2018). Second, the conformity-differentiation balance literature pays limited attention to the value of the learning model in terms of finding optimal distinctiveness (Zhao et al., Reference Zhao, Fisher, Lounsbury and Miller2017). Our study shows that the model of exploitation and exploration (March Reference March1991, Reference March2003, Reference March2010), with some extensions, can be applied effectively to the conformity-differentiation paradox. The research findings demonstrate that the balance between conformity and differentiation can be framed as the balance between exploitation and exploration across the levels of firm and industry. While imitation helps a focal firm explore new knowledge, it exploits the existing opportunities and knowledge pool at the industry level. Meanwhile, experimentation tends to exploit firm-level experiences, but it explores novel opportunities with diverging knowledge pool at the industry level. Knowledge accumulated and maintained by experimentation is diffused as a spillover to the industry via imitation.
Our findings also shed light on managerial practices. Besides the ‘success trap’ highlighted by March (1991), we suggest the need to pay attention to the ‘imitation trap’. When faced with technological uncertainties, firms are tempted to rely too much on imitating others because it is an effective strategy in the short run, but in the long run it is self-defeating. In addition, firms often tend to follow industrial giants. However, our findings challenge this approach and suggest that firms should learn from their peers that have the best recent performance, even though these firms may not be large players with a long-standing reputation in the industry.
The theoretical background of the study will be discussed in Section 2, followed by a case study of the Chinese PV industry in Section 3. Then, based on the case findings, we conduct a follow-up computational simulation in Section 4. We discuss the theoretical and practical implications and conclude the article in Section 5.
THEORETICAL BACKGROUND: BALANCING CONFORMITY-DIFFERENTIATION
Rooted at the intersection of institutional theory and the strategic management literature (Brewer, Reference Brewer1991; Deephouse, Reference Deephouse1999; Durand & Kremp, Reference Durand and Kremp2016; Zhao et al., Reference Zhao, Fisher, Lounsbury and Miller2017), the conformity-differentiation paradox implies that firms should, on the one hand, conform to the requirements imposed by external stakeholders to gain legitimacy and earn institutional resources (DiMaggio & Powell, Reference DiMaggio and Powell1983), while, on the other hand, differentiate from peers to reduce competition and gain competitive advantage (Deephouse, Reference Deephouse1999; Porter, Reference Porter1996). As both improved legitimacy and reduced competition enhance firms’ performance (Haans, Reference Haans2018; Haveman, Reference Haveman1993), how distinctly a firm should position itself in order to achieve optimal distinctiveness has become a focal research agenda, receiving increased attention in recent years (Durand & Jacqueminet, Reference Durand and Jacqueminet2015; Durand & Kremp, Reference Durand and Kremp2016; Zhang et al., Reference Zhang, Wang and Zhou2020; Zhao et al., Reference Zhao, Fisher, Lounsbury and Miller2017; Zuckerman, Reference Zuckerman, Pratt, Schultz, Ashforth and Ravasi2016).
The extant literature has investigated optimal distinctiveness in various empirical scenarios, such as corporate governance and social responsibility (Durand & Jacqueminet, Reference Durand and Jacqueminet2015; Zhang et al., Reference Zhang, Wang and Zhou2020); entrepreneurial resource acquisitions and new venture founding (Fisher & Lahiri, Reference Fisher and Lahiri2016); new entrants and platform strategies (Barlow, Verhaal, & Angus, Reference Barlow, Verhaal and Angus2019; Cennamo & Santalo, Reference Cennamo and Santalo2013; Zhao et al., Reference Zhao, Ishihara, Jennings and Lounsbury2018); business model design (Zott & Amit, Reference Zott and Amit2007); distinctiveness strategies in different population categories (Durand & Kremp, Reference Durand and Kremp2016; Haans, Reference Haans2018); and public and private family firms (Miller, Amore, Le Breton-Miller, Minichilli, & Quarato, Reference Miller, Amore, Le Breton-Miller, Minichilli and Quarato2018). The implications of these studies vary: while some suggest that moderate distinctiveness leads to improved business performance (Deephouse, Reference Deephouse1999), others argue that, by strategically positioning itself as moderately distinctive from its peers, a firm will largely damage its performance (Cennamo & Santalo, Reference Cennamo and Santalo2013; Haans, Reference Haans2018; Miller et al., Reference Miller, Amore, Le Breton-Miller, Minichilli and Quarato2018). Faced with the disparate and even contradictory findings, the recent literature highlights the importance of a synthesized understanding of the conformity-differentiation balance (Haans, Reference Haans2018; Zhao et al., Reference Zhao, Fisher, Lounsbury and Miller2017).
The balance between conformity and differentiation is traditionally regarded as solving a unidimensional problem (e.g., Deephouse Reference Deephouse1999). Recent literature recognizes that the conformity and differentiation paradox is, by its nature, multifaceted and multiplex (Durand & Jacqueminet, Reference Durand and Jacqueminet2015; Haans, Reference Haans2018; Mazzelli, Kotlar, & De Massis, Reference Mazzelli, Kotlar and De Massis2018; Zhao et al., Reference Zhao, Ishihara, Jennings and Lounsbury2018). Due to methodological limitations, however, extant empirical studies are based on settings in which organizations have simple choices that are inadequate to capture the multiplexity of the conformity-differentiation issue. Thus, studies with an updated view of the conformity-differentiation paradox, as well as new methodological approaches, are needed.
A major gap in the current research on optimal distinctiveness is that it neglects the fact that managing the conformity-differentiation paradox is inherently a learning process that involves proactive adaption based on one's own and others’ experiences (see Zhao et al., Reference Zhao, Fisher, Lounsbury and Miller2017 for a review). Specifically, Zhao et al. (Reference Zhao, Fisher, Lounsbury and Miller2017) call for a renewed research agenda by incorporating the perspective of organizational ambidexterity with that of conformity-differentiation balance, especially across the levels of firm and industry.
Institutional theory highlights another fundamental pressure that triggers organizational isomorphism and conformity (DiMaggio & Powell, Reference DiMaggio and Powell1983; Haunschild, Reference Haunschild1993; Haunschild & Miner, Reference Haunschild and Miner1997; Haveman, Reference Haveman1993). In the presence of uncertainties, organizations tend to imitate peer organizations’ experiences to reduce risks, lower costs, and improve legitimacy to gain institutional resources, leading to ‘mimetic isomorphism’ (DiMaggio & Powell, Reference DiMaggio and Powell1983; Haunschild & Miner, Reference Haunschild and Miner1997; Haveman, Reference Haveman1993). However, ‘new developments in institutional theory have redirected attention away from isomorphism and toward the theorization of organizational heterogeneity…As part of this redirection, institutional environments are conceptualized as fragmented, contested, and dynamic’ (Zhao et al., Reference Zhao, Fisher, Lounsbury and Miller2017: 99). In this sense, firms’ adaptability via learning will become highly salient in terms of gaining institutional capital (McKnight & Zietsma, Reference McKnight and Zietsma2018; Zhao et al., Reference Zhao, Fisher, Lounsbury and Miller2017).
According to the organizational learning literature, experiential learning and vicarious learning are two basic learning mechanisms (Baum et al., Reference Baum, Li and Usher2000; Denrell, Reference Denrell2003; Ingram & Baum, Reference Ingram and Baum1997). Experiential learning refers to a firm's independent experimentation, while vicarious learning refers to imitation of other firms’ choices (Baum et al., Reference Baum, Li and Usher2000; Bruneel et al., Reference Bruneel, Yli-Renko and Clarysse2010). When faced with an unknown field with various choices, both experiential learning and vicarious learning are present. Differentiation is a natural outcome of experiential learning because firms are exposed to distinct outcomes in their learning process. Yet, according to the strategic management literature, firms may also intentionally pursue distinctiveness from other firms to generate and sustain competitive advantages (Benner & Tushman, Reference Benner and Tushman2013; Junni, Sarala, Taras, & Tarba, Reference Junni, Sarala, Taras and Tarba2013; Luger, Raisch, & Schimmer, Reference Luger, Raisch and Schimmer2018; Raisch et al., Reference Raisch, Birkinshaw, Probst and Tushman2009; Stettner & Lavie, Reference Stettner and Lavie2014).
In experiential learning, firms are more inclined to engage in exploitation instead of exploring new opportunities and may fall into the familiarity trap (Baum et al., Reference Baum, Li and Usher2000; March, 1991). In contrast, vicarious learning by imitating others’ experiences often remedy the costs and uncertainties associated with exploration (Baum et al., Reference Baum, Li and Usher2000; Bruneel et al., Reference Bruneel, Yli-Renko and Clarysse2010; Ingram & Baum, Reference Ingram and Baum1997). As highlighted in the organizational learning literature, inter-firm learning is a common strategy to diffuse knowledge and innovation, deal with uncertainty, and earn adaptability (Levinthal & March Reference Levinthal and March1993, Levitt & March Reference Levitt and March1988). For example, Levitt and March (Reference Levitt and March1988), in an influential learning article, argue that isomorphism promotes adaptability and innovation diffusion:
The particular professions, policies, programs, laws, and public opinion… become powerful institutionalized myths that are adopted by organizations to legitimate themselves and ensure public support In the organizational literature, these three processes have been labeled coercive, mimetic, and normative (DiMaggio & Powell Reference DiMaggio and Powell1983). All three are involved in a comprehensive system of information diffusion. (Levitt & March, Reference Levitt and March1988: 330–331)
Despite the strong linkage indicated in the classic organizational learning and institutional theory literature, studies integrating organizational learning and the conformity-differentiation paradox are lacking. In particular, the above views are from the perspective of a single firm, which may differ from the industry-level perspective, so a cross-level approach is necessary. As both the conformity and differentiation strategies can be seen as results of organizational learning, we are motivated to explore how organizational learning across the intra- and inter-firm levels contributes to the proper balance between conformity and differentiation in terms of optimal distinctiveness as strategic balance (McKnight & Zietsma, Reference McKnight and Zietsma2018; Zhao et al., Reference Zhao, Fisher, Lounsbury and Miller2017).
CONFORMITY-DIFFERENTIATION IN THE CHINESE PHOTOVOLTAIC INDUSTRY
Case Background, Data Sources, and Data Analysis
Informed by the gap in the current conformity-differentiation literature and driven by our interest in nascent industries from emerging economies, we conducted a case study to investigate how the conformity-differentiation balance is achieved from the organizational learning perspective. The case study provides an in-depth investigation and analysis of emerging phenomena that the existing literature cannot explain. Additionally, it offers theory-building opportunities (Eisenhardt & Graebner, Reference Eisenhardt and Graebner2007; Siggelkow, Reference Siggelkow2007; Yin, Reference Yin2017). We chose the technological trajectory development of the multicrystalline silicon manufacturers in China's photovoltaic industry as our case.
The photovoltaic (PV) industry is one of the fastest-growing emerging industries in China. At present, China's PV industry has formed a competitive landscape with monocrystalline silicon (mono-Si) and multicrystalline silicon (mc-Si) as the two main component materials, which are also associated with distinct technological trajectories. Starting in 2007, along with the development of China's PV industry, mc-Si-based components gradually expanded and reached a 95% market share in 2014. However, since then, mono-Si based components have observed rapid growth in its market share, from 5% in 2014 to 15% in 2015, and then to 45% in 2018.
Challenged by the mono-Si-based technological trajectory and its rapid rise in market share, mc-Si manufacturers explored and quickly diffused the technological combination of ‘diamond wire sawing (DWS) + black silicon texturing + passivated emitter and rear contact (PERC) battery’ through interactive learning. The technological innovation in mc-Si slicing and batteries reduced the development and production costs of the entire mc-Si technological trajectory, improved the conversion rate of the mc-Si batteries, and strengthened the competitiveness of the mc-Si trajectory. While technology diffusion clearly benefited the mc-Si group, it also led to isomorphism and, inevitably, intensified the competition among mc-Si manufacturers. Thus, we find that the mc-Si manufacturers incorporated both adaptability considerations and individual distinctions, which served as a suitable setting for this research. Our case study adopts a multi-embedded units design. We focus on the technological development of the Chinese PV industry from 2014 to 2018 and chose companies such as GCL, CSIQ, Jinko, and Yingli as the multiple embedded units. The selected companies include industrial leaders, mainstream manufacturers, and technology followers.
We collected both primary and secondary data to enable data triangulation. Secondary data came from various sources. We conducted desk research on the annual industrial reports published by the China PV Industry Association (2016-2019) and the annual reports (2014-2018) of the top ten listed PV companies. We also followed the highlights and publications from nine industrial conferences and technology forums on DWS and black silicon texturing technology from 2014 to 2018 to strengthen our understanding. These secondary data sources provide rich information on the development of the PV industry and the technological trajectory adoption of PV manufacturers.
Regarding the primary data, from July 2018 to January 2019 we conducted field investigations and nine in-depth interviews with technological and business executives from four PV companies specializing in mc-Si. A summary of the interviews appears in Table 1. In addition, since 2018, one author has participated in two WeChat groups of the PV industry (each with 500 participants) to observe and conduct informal discussions with PV experts.
Table 1. Summary of interviews
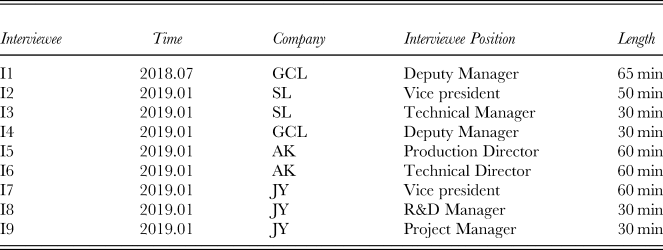
Coding and categorical analysis were adopted inductively in the data analysis (Gioia, Corley, & Hamilton, Reference Gioia, Corley and Hamilton2013; Miles & Huberman, Reference Miles and Huberman1994). We sorted and documented the primary and secondary data into a database consisting of sub-databases of individual mc-Si manufacturers. We first gained an overview of the development and innovation trend in the Chinese PV industry through desk research. We also identified the technological trajectory choices of individual mc-Si manufacturers. Two authors open coded our data independently according to critical events, technological trajectories, industry development, challenges, market share, etc. Then, they worked together to compare the open coding results and conducted axial coding together, categorizing and synthesizing open nodes into conceptual themes. We further identified second-level themes that are related to three core dimensions of our research goal: conformity, learning, and differentiation. We then scrutinized the underlying relationships and mechanisms across the theoretical constructs to generate theoretical propositions regarding how the conformity-differentiation balance is achieved through organizational learning behaviors. The three authors exchanged reflections and thoughts constantly to mitigate potential bias. The data coding scheme is shown in Table 2.
Table 2. Data coding scheme
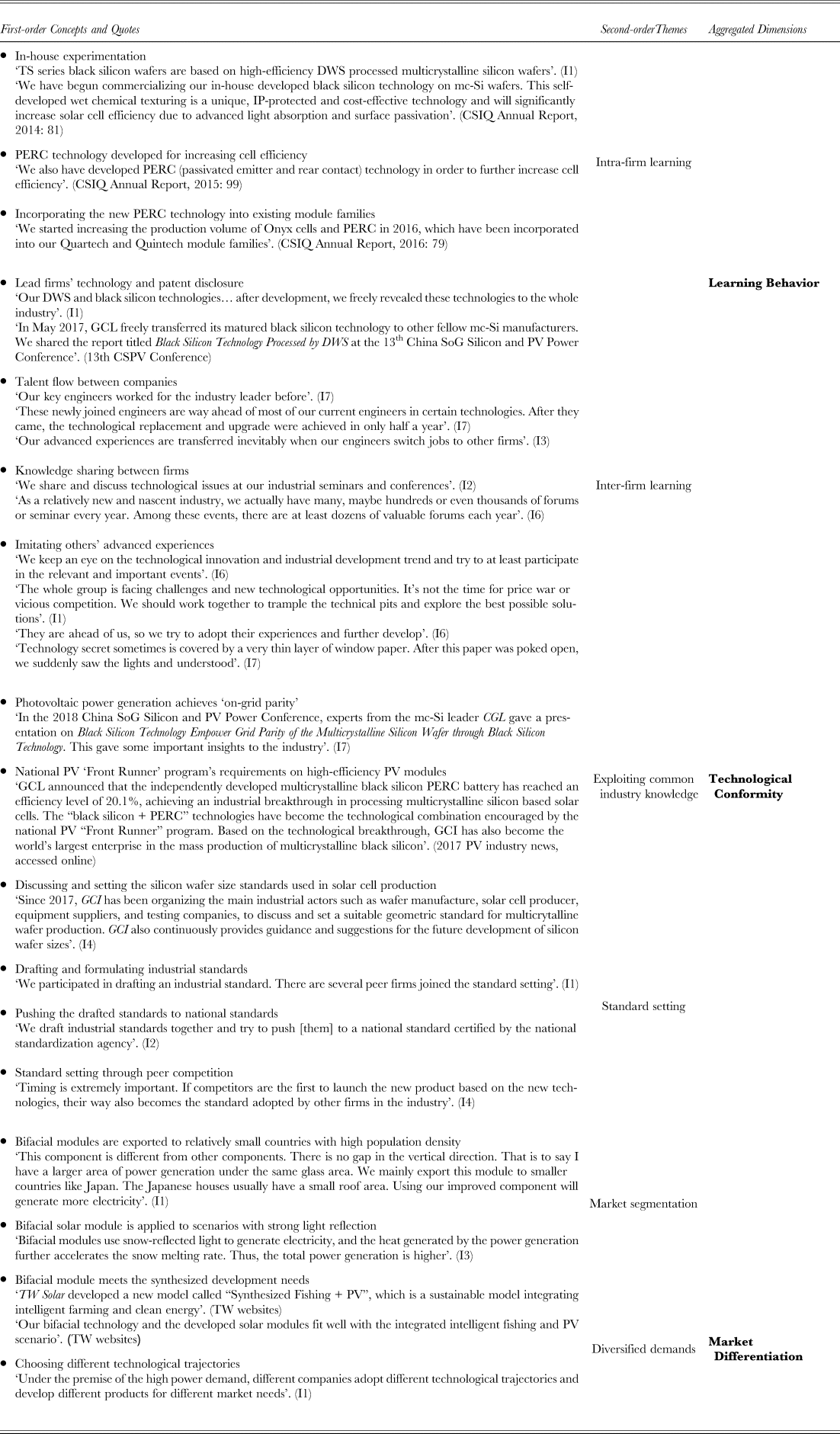
Findings
Technological conformity
An essential factor enabling the mono-Si group to challenge the mc-Si group's market share is the introduction of the diamond wire sawing technology in the wafer slicing process. Crystal silicon wafers account for 40% of the total costs of solar cell component production, and silicon squaring and wafer slicing are the most costly processes in wafer production. Therefore, reducing the cutting costs can contribute greatly to lowering the cost of the solar cell component. Conventional crystalline silicon slicing typically employs a slurry-based wafer sawing technique. Compared with slurry-based wafer sawing, DWS has three major advantages. First, the silicon consumption per production unit is greatly reduced, and the consumables per wafer are far lower. Second, the cutting speed and the wafer production capacity are greatly increased, which reduces the depreciation, electricity, and labor costs spent on wafer cutting. This leads to the rapid decline of non-raw material costs in wafer production. Third, replacing the difficult-to-process slurry can significantly reduce auxiliary costs and pollution during the slicing process.
The introduction of DWS technology to the Chinese PV industry is owing to the mono-Si leader, Longi Green Energy, and its research and development since 2013. A 100% replacement rate of the DWS technology in mono-Si slicing was reached in 2017. Confronted by the rise of the mono-Si group powered by DWS, the mc-Si group also started to investigate the possibility of adopting DWS. However, due to the lattice order features, adopting DWS technology in mc-Si slicing faces two major bottlenecks. First, the hard spots existing in the ingot crystal may cause broken lines during the cutting. Second, it is difficult to prepare the anti-reflection coatings with the traditional acid vapor texturization; thus, the reflectivity rate of processed mc-Si wafers is too high.
According to the Chinese Photovoltaic Association, the adoption rate of DWS among mc-Si manufacturers was only 5% in 2016 but rose to 35% in 2017. According to the 2017 prediction of the International Technology Roadmap for Photovoltaic, the adoption rate of DWS in mc-Si in 2018 was supposed to be around 50%. It was believed that texturing technology would be the major bottleneck for the popularity of DWS in mc-Si. However, beyond all expectations, the adoption rate of DWS in mc-Si jumped to 95% in 2018. The unexpected fast diffusion of DWS was due to the introduction and popularization of the black silicon texturing technology that solved the previously mentioned bottlenecks. Specifically, GSL, the leading mc-Si company, freely and fully disclosed the black silicon texturing technology to the industry in 2017. The wide application of DWS thereby increased the wafer output from 48 per kilo of the mc-Si ingot in 2016 to 63 per kilo in 2018, and it lowered the non-silicon cost of mc-Si by 0.8 RMB per slice.
Although adopting the ‘DWS + black silicon’ technological combination significantly improved productivity and lowered slicing costs of the Chinese mc-Si companies, the market share of the mc-Si group was still declining. The mc-Si companies needed to continuously improve the conversion efficiency of their PV products to remain competitive. Therefore, based on ‘DWS + black silicon’, mc-Si manufacturers further introduced and adopted the PERC battery technology. Although PERC technology was first adopted by mono-Si battery, it generated greater technological synergy with the mc-Si material after the breakthrough and diffusion of the bottleneck black silicon texturing technology solved the compatibility issue.
In 2017, mainstream mc-Si manufacturers, such as CSIQ, Yingli, GCL, and Risen Energy, started to apply the PERC technology to the mc-Si solar cells that are pre-processed by the black silicon texturing technique. The application of PERC technology, therefore, significantly increased the conversion efficiency of mc-Si cells from 18.5% in 2016 to 20.3% in 2018 and narrowed the gap with mono-Si cells in terms of conversion rates (see Figure 1). As the cost of mc-Si solar cells is significantly lower than that of mono-Si based products, the overall benefits and cost-efficiency of the mc-Si cells even outperformed the mono-Si cells.
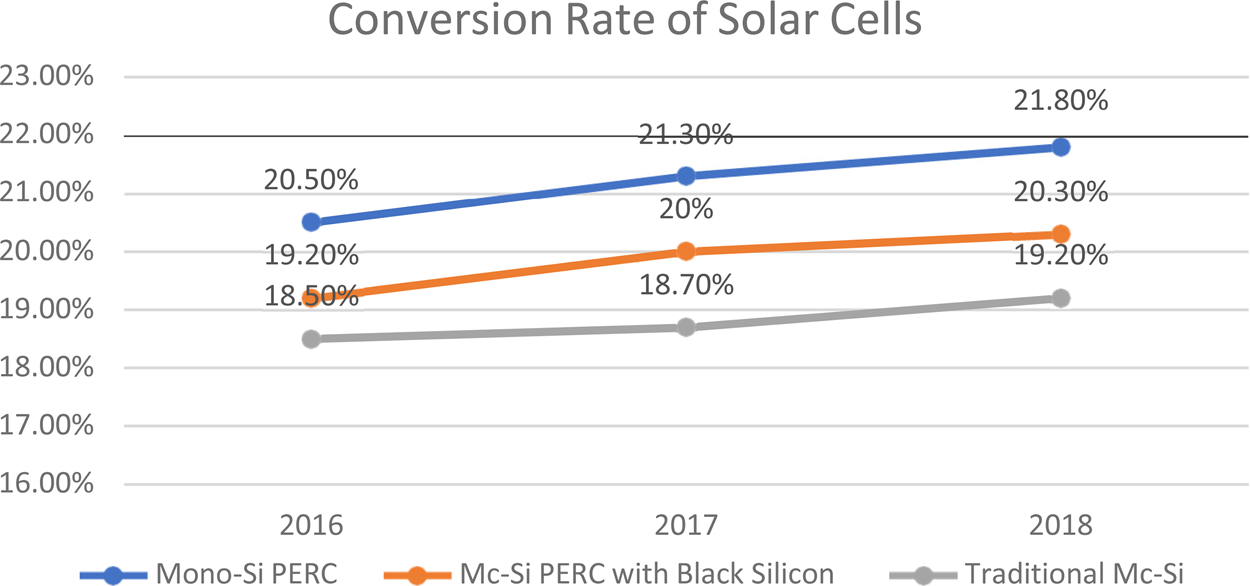
Figure 1. Comparison of conversion rates between solar cells with different silicon materials and module technologies
Market differentiation
The competition was concentrated mainly on the market application of the solar cells – namely, PV module development and the respective markets. In contrast to the convergence in solar cell technologies, mc-Si companies presented differentiated trajectory choices on the downstream module technologies to avoid patent protection, serve various market demands, and, thus, achieve differentiated market performances.
As described above, the mc-Si manufacturers have conformed to the technological combination of ‘DWS + black silicon + PERC’ in the upstream solar cell development. Yet in the application of solar cells – i.e., module development – the mc-Si manufacturers have demonstrated divergence. Currently, high-efficiency PV modules that are compatible with the black silicon and PERC include half-cell module, double glass bifacial module, shingled module, and multi-bus bar module (MBB). Each module trajectory has its advantages in coping with different optimization methods, application scenarios, and market demands. To pursue differentiated advantages, the Chinese mc-Si manufacturers demonstrate differentiated module technologies through licensing from or partnering with foreign leaders, or through independent R&D. For example, the shingled technology outperforms other module technologies due to its significant reduction in electrical losses and improvement of efficiency. However, the shingled technology patents are owned mostly by foreign leaders such as SunPower and Solaria. The only licensed Chinese companies are DZS, Seraphim, and GCL. Among them, DZS is a joint venture with Sunpower, and Seraphim has established a strategic partnership with Solaria. Chinese manufacturers such as Risen and Longi developed the shingled technology independently. Some other Chinese companies explored alternative technologies, such as Jinko's self-developed tiling ribbon technology, to avoid the patent restrictions.
Table 3 summarizes the mainstream mc-Si manufacturers’ adoption of upstream solar cell and downstream module technologies. Through the development of different module technological trajectories, Chinese PV manufacturers can compete differently, based on the technical isomorphism of ‘DWS + black silicon + PERC’. As the table shows, mastering the bifacial module technology and the self-developed TR technology, Jinko has led in global shipments since 2016. With the shingled module technology, Risen entered the top ten in 2017, and its ranking has been steadily increasing.
Table 3. Mc-Si manufacturers’ adoption and application of solar cell and module technologies

The differentiation and competition in the downstream market has also greatly promoted the overall performance of the mc-Si solar cell modules. As shown in Figure 2, the average power of traditional mc-Si modules was only 275 watts in 2018, whereas the average power output of black silicon PERC modules increased from 270 watts in 2016 to 295 watts in 2018, and the gap with the mono-Si modules has been narrowed.
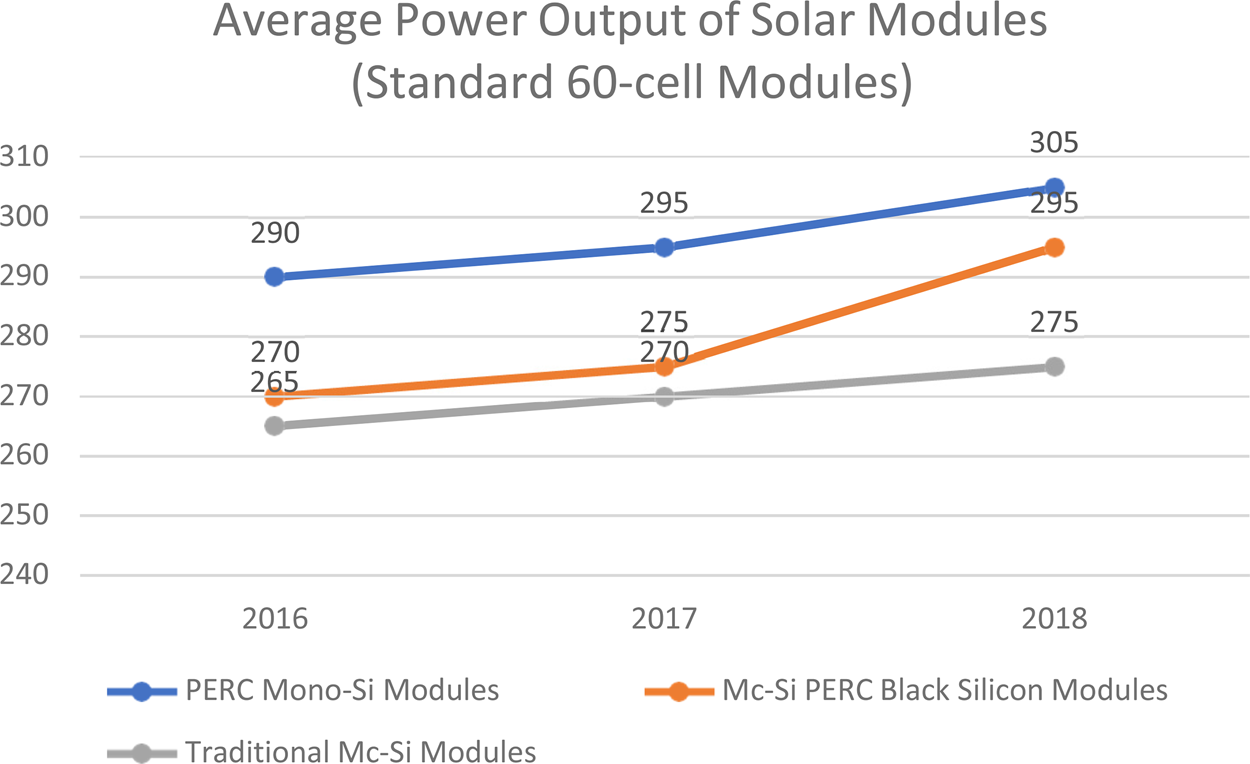
Figure 2. Overview of the average power output of different solar modules
Cross-level learning
The case depicts a setting in which PV manufacturers within a nascent industry are faced with various technological trajectories. Due to limited information on trajectories that perform better, firms are engaged in intensive experimentation across different options. Our coding results show the presence of cross-level learning: intra-firm experimentation and inter-firm imitation are both salient at interdependent technological domains along the value chain.
The fast development and diffusion of the ‘DWS + black silicon + PERC’ technological combination has been achieved primarily through imitation. ‘DWS + black silicon’ was first introduced by Japanese researchers and engineers at China's 2014 SoG Silicon and PV Power (CSPV) Conference. One year later, China's mc-Si leading manufacturers began to scale up production based on the new solar cell technologies. At the 2015 CSPV, Chinese mc-Si leaders such as CSIQ and GCL replaced the Japanese experts and took the lead in sharing technological advancements. In 2016, a considerable number of Chinese mc-Si manufacturers, such as JA and JK, began to engage in the R&D and application of DWS and black silicon texturing technologies. In the same year, the first technological seminar on ‘DWS + black silicon’ was held. Major mc-Si manufacturers, including GCL, CSIQ, JA, JK, CSG, and Suntech, openly shared their experiences with DWS and two major black silicon texturing methods: Reactive Ion Etching and Metal Catalyzed Chemical Etching. Interviews with business managers suggested that knowledge disclosure had greatly promoted the advancement and maturity of the new technologies, which benefited both the leaders and the imitators. A GCL manager explained:
It is beneficial to the promotion of our products and technology route. We will disclose this technology to everyone, and everyone can use it. This can also help solve the (potential) technological problems by utilizing others’ efforts. (I1)
Besides leaders’ purposeful knowledge disclosure, employee movement across different firms also enriched the industrial knowledge sharing and innovation diffusion. As a top executive from JY explained:
These newly joined engineers are way ahead of most of our current engineers in certain technologies. After they came, the technological replacement and upgrade were achieved in only half a year. (I7)
While inter-firm learning is salient in the bottleneck solar cell technologies, intra-firm learning is predominant in the downstream solar module development. There are two major reasons why firm-level experimentation became the dominant learning mode in downstream module development and market application. The first is competitive pressure from peer firms. To reduce direct competition in the same market, mc-Si manufacturers chose different technological trajectories in module development through various intra-firm learning efforts, such as in-house development or licensing from foreign leaders. Second, the final solar panel application scenarios imposed diversified and distinct market requirements, and different application scenarios were associated with distinct and tacit knowledge that was difficult to communicate and share. Thus, the mc-Si manufacturers chose to individually develop solar modules to fit the specific market segments. For example, based on the bifacial technological trajectory, TW Solar developed solar modules to fit the scenario of fishing grounds, proposing a sustainable model that integrated intelligent fishing and green energy.
Case Discussion
This case clearly shows a pattern of conformity and differentiation in a balance achieved through cross-level organizational learning behaviors. The underlying connections between the three core theoretical constructs – i.e., learning, conformity, and differentiation – are shown in Figure 3. In terms of the upstream solar cell technologies, the mc-Si companies conformed to the technology combination of ‘DWS + black silicon + PERC’. Achieving technological conformity depended mainly on imitation enabled by industrial leaders, industrial communications, and talent turnover.
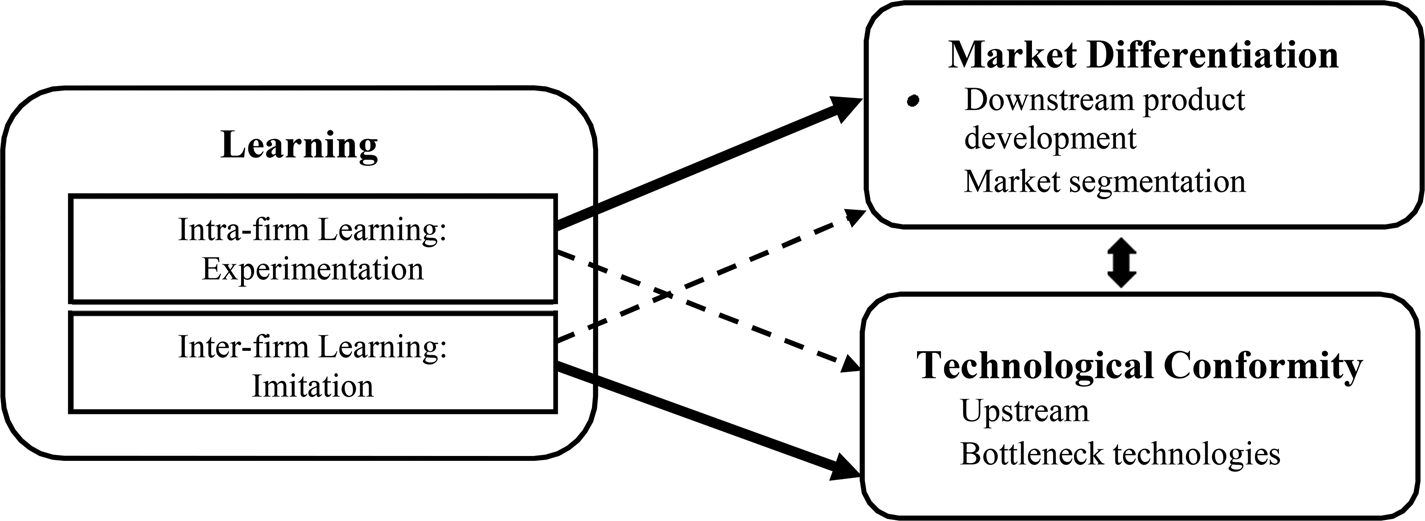
Figure 3. The conformity-differentiation balance can be achieved through cross-level learning. The solid arrows reflect the stronger relationships between the key theoretical constructs, while the dotted arrows reflect the weaker relationships.
The technological convergence increased the adaptability of promising technological trajectories, reduced technological exploration costs, and, therefore, improved the market share of the whole mc-Si group. For bottleneck technological areas that are costly to conquer, firms can exploit the common industry-level knowledge through imitation, so that they can explore new opportunities with lower costs and fewer uncertainties. Meanwhile, as a similar choice in nature may intensify competition, the mc-Si manufacturers differentiated themselves in the downstream market segmentation in module development through trial-and-error experimentation such as in-house R&D.
It is also worth noting that, though the case findings suggest inter-firm learning and technological conformity for bottleneck technologies, intra-firm learning was never absent. For example, the ‘DWS+Black Silicon+PERC’ combination was developed and constantly improved by industrial leaders and followers through independent experimentation. Similarly, peer comparison and conformity remained when differentiation was the dominant strategy in the downstream market. As Table 3 shows, though the mc-Si manufacturers demonstrated differentiated technological trajectory choices and showed diversified market performance, they shared consensus on several better-performing technological trajectories. The case findings lead to the following theoretical propositions:
Proposition 1: In a nascent industry, the conformity-differentiation balance can be achieved in interdependent technological areas with asymmetrical focuses: (1) conformity is reflected in the bottleneck or core technological areas with a primary dependence on inter-firm learning (i.e., convergence by exploiting others’ experiences), and (2) differentiation is reflected in downstream market applications with a primary dependence on intra-firm learning (i.e., divergence by exploring unique opportunities).
Proposition 2: The balance between conformity and differentiation can be framed as balancing exploration and exploration across the firm and industry levels.
Computational Simulation
The case findings show that while exploiting industry-level collective experiences through imitation leads to conformity firms’ own experimentation enriches the industry's knowledge pool. The extent to which firms should learn from others and from whom firms should learn to achieve best performances is still unclear. To investigate the degree and target of imitation that may lead to optimal distinctiveness, we conducted computational simulation to verify and generalize the case findings and theoretical propositions. Our investigation of the effect of organizational learning on the conformity-differentiation paradox is based primarily on the multi-armed bandit problem. March and other researchers have extensively used the bandit problem to study organizational learning (March, Reference March1996, Reference March2003, Reference March2010; Posen & Levinthal, Reference Posen and Levinthal2012; Reypens & Levine, Reference Reypens, Levine, Galavan, Sund and Hodgkinson2017).
Learning Model
In the original bandit problem, an organization chooses from a set of ! alternatives in each period t of the organization's lifespan T, seeking to maximize the flow of returns over time. To capture the conformity-differentiation tradeoff exhibited in a nascent industry, we follow the case findings and extend the original bandit problem in the following ways (technical details of the computational model can be found in the supplemental Appendix):
1. A firm faces a hierarchical bandit problem: it needs to first choose one of ! primary technological trajectories and then choose one of " complementary technologies. The primary trajectory (e.g., solar cell technology in the PV industry) requires a complementary technology (e.g., the downstream module technology) to realize synergy and to achieve commercialization. The payoff from choosing a combination of technologies is probabilistic and follows a normal distribution. Unknown to the organization in advance, the mean of the distribution is determined by the productivity of the trajectory and its complementary technology. The structure of the hierarchical bandit problem is shown in Figure 4.
2. Firms’ experiences are passed around in the industry, making inter-firm learning possible. Firms reveal only their primary technology, without information on their complementary technology choices.
3. Popular primary technologies enjoy enhanced adaptability. This adaptability comes from competence derived from a learning-by-doing process at the industry level, which decreases the costs of development, production, and marketing.
4. Firms choose the identical primary and complementary technologies to compete in the same market segment. The payoff that a firm receives from the bandit problem is discounted by the number of existing firms in the same market segment.
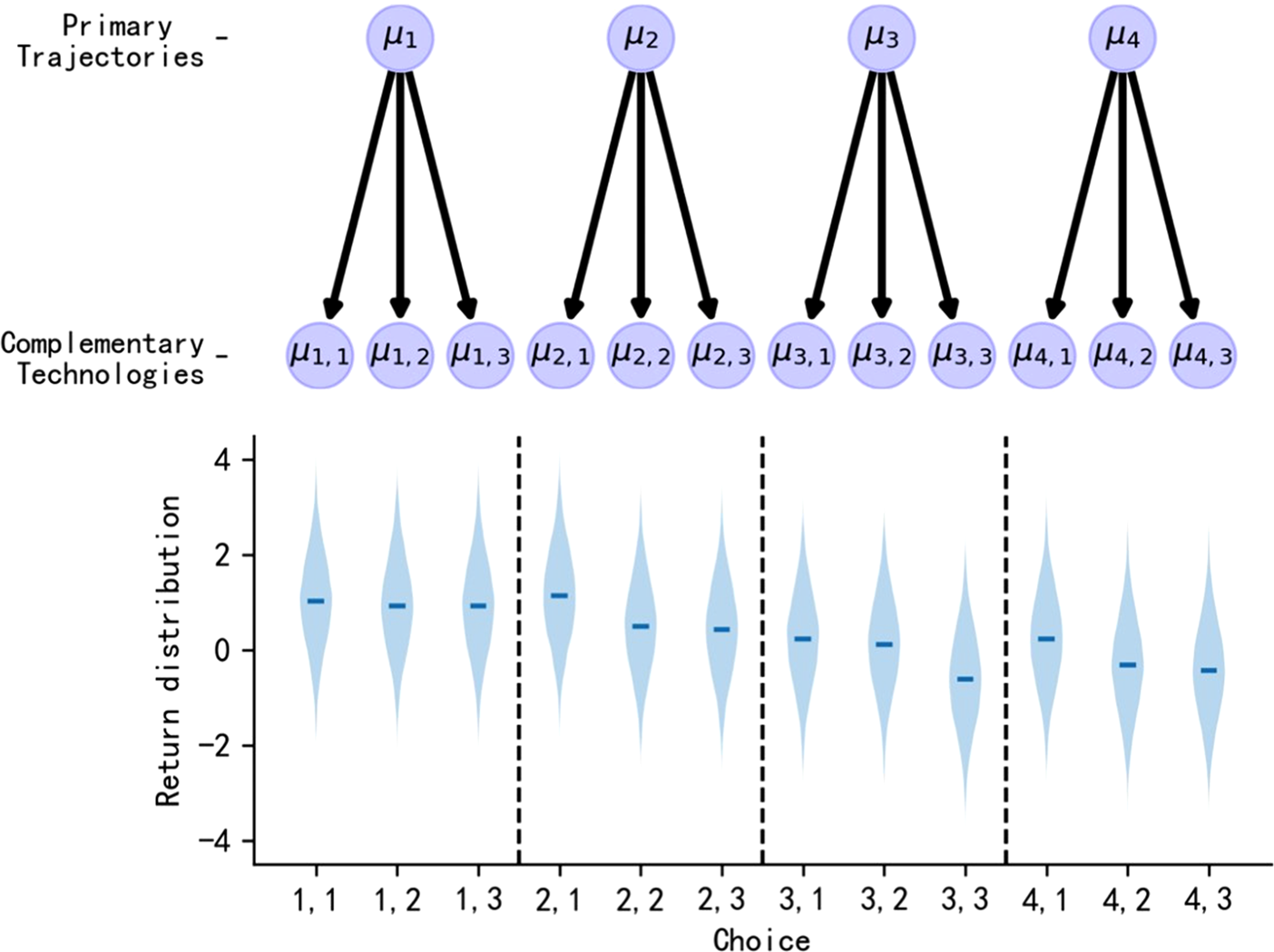
Figure 4. Illustration of hierarchical multi-armed bandit problem and the corresponding distributions of returns. A firm can choose different primary technological trajectories (1,2,3,…,A), and for each trajectory, there are B technologies of implementation that are complementary to the trajectory (1,2,…,B). The payoff from choosing the alternative (”, $) follows a normal distribution with mean &!,# and a unit variance, while &!,#~((&!,)%) and &!~((0,)%).
In the extended bandit problem, firms are interdependent because their choices inevitably influence the adaptability of technology and the intensity of competition. Through a learning-by-doing process, an organization, or the whole industry, develops competence in specific markets, technologies, and strategies. This learned competence at the industry level is the main source of adaptability (Denrell & March, Reference Denrell and March2001). The increased adaptability can, thus, reduce the potential costs of the diffusion and the adoption of a technology. At the same time, firms using identical technologies will compete with each other due to their confrontation in the same market segment or technological area.
This extension allows us to examine the underlying principle that conformity and isomorphism are associated with increased adaptability and competition. As shown in Figure 5, it is assumed that an inverted U-shaped relationship exists between the average return of adopting a technological trajectory and the number of firms choosing the same trajectory (Haans, Reference Haans2018). The combination of adaptability and competition determines that firms have to find a balance between conformity and differentiation (Haans, Reference Haans2018; Haveman, Reference Haveman1993): a popular technology is usually more mature and widely accepted by the market, but using the same technology as other firms leads to intense competition that undermines a firm's competitive advantage.
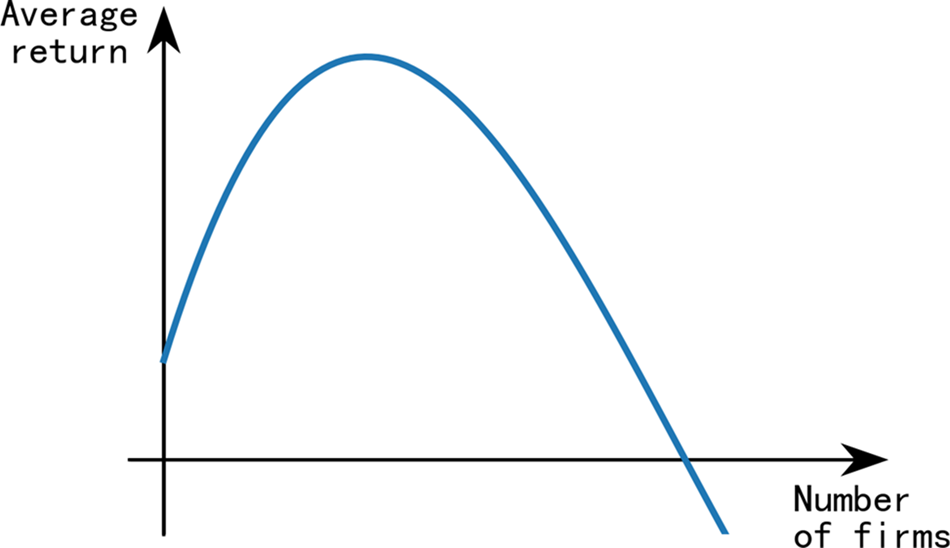
Figure 5. The conformity-differentiation paradox demonstrated as an inverted-U relationship between the average return of using one technology and the number of firms adopting the same technology.
Intra-firm experimentation
Following firms’ behavioral assumptions, we assume that firms adopt an experiential learning process to improve their performance in an adaptive way (Cyert & March, 1963; Denrell & March, Reference Denrell and March2001; March, 2010). Experiential learning was popularized by James March in his seminal studies (Cyert & March, 1963; Levitt & March, Reference Levitt and March1988), which claim that the propensity to select a particular alternative depends on the outcomes of this alternative when it was chosen previously.
Inter-firm imitation
Based on the case findings and the institutional theory literature (e.g., Haunschild, Reference Haunschild1993; Haunschild & Miner, Reference Haunschild and Miner1997; Haveman, Reference Haveman1993), we introduce three imitation modes to the experiential learning model: firms can imitate the trajectory chosen by most firms (imitate the crowd), by the best performing firm (imitate the best), or by the firm with the highest cumulative payoffs (imitate the big). In the no imitation treatment, firms focus on intra-firm learning and do not compare their choices with those of other firms. In the other treatments, a firm tries to conduct inter-firm imitation with probability # and implements the choice made by other firms if it believes that this choice could potentially bring a higher payoff. In the imitate the best treatment, the target is the firm with the best performance over the past five periods. In the imitate the big treatment, the target is the firm with the highest cumulative payoff. And in the imitate the crowd treatment, the imitating firm compares its payoff with the average industry payoff over the past five periods; it then implements the choice that has been most frequently chosen over the past five periods if it performed worse than the industry average. Firms actively share experiences with and knowledge of a primary technology to help its maturity and diffusion, but they seldom reveal information on the specifics of the complementary technologies. We base this assumption on observations derived from the case study (i.e., imitation largely happens in the upstream bottleneck technological area).
Computational Experiment
A firm's learning strategy can be characterized by its exploration tendency $ (the likelihood of engaging in exploration over exploitation) and its imitation tendency #. In the experiments, we set the default # as 0.02, and default $ as 0.1. The values for $ explored under the default # include {0.02,0.1,0.2,0.5,1}, and the values of # explored under the default $ include {0.02,0.1,0.2,0.5,1}.
The computational experiments with four treatments are seeded with 20,000 industries, each consisting of % = 30 firms. Firms are faced with multi-armed bandit problems with ! = 20 and " = 3, with timespan * = 500. Firms in the same industry face the same bandit problem and use the same exploration ($) and imitation (#) strategies. These industries are divided equally among ten sets of exploration($)-imitation(#) strategies and the four treatments.[Footnote 1]
In the following analysis, we first investigate how exploration and imitation tendencies influence performance under different imitation modes. We then investigate how imitation affects the conformity-differentiation tradeoff and follow that with a discussion of the results.
Exploration tendencies
We first briefly look at the exploration strategies to investigate whether the classical organizational ambidexterity paradox – the tradeoff between exploration and exploitation – applies to situations in the presence of competition and adaptability. Figure 6 demonstrates the average performance as a function of the exploration strategy ($), which visualizes the tradeoff between exploration and exploitation and the advantage of imitation. The advantage of imitation (imitate the best/big/crowd) is more salient with small $: imitate the best slightly outperforms imitate the big, while both lead to significantly better performance than imitate the crowd. These differences dissipate as $ increases.
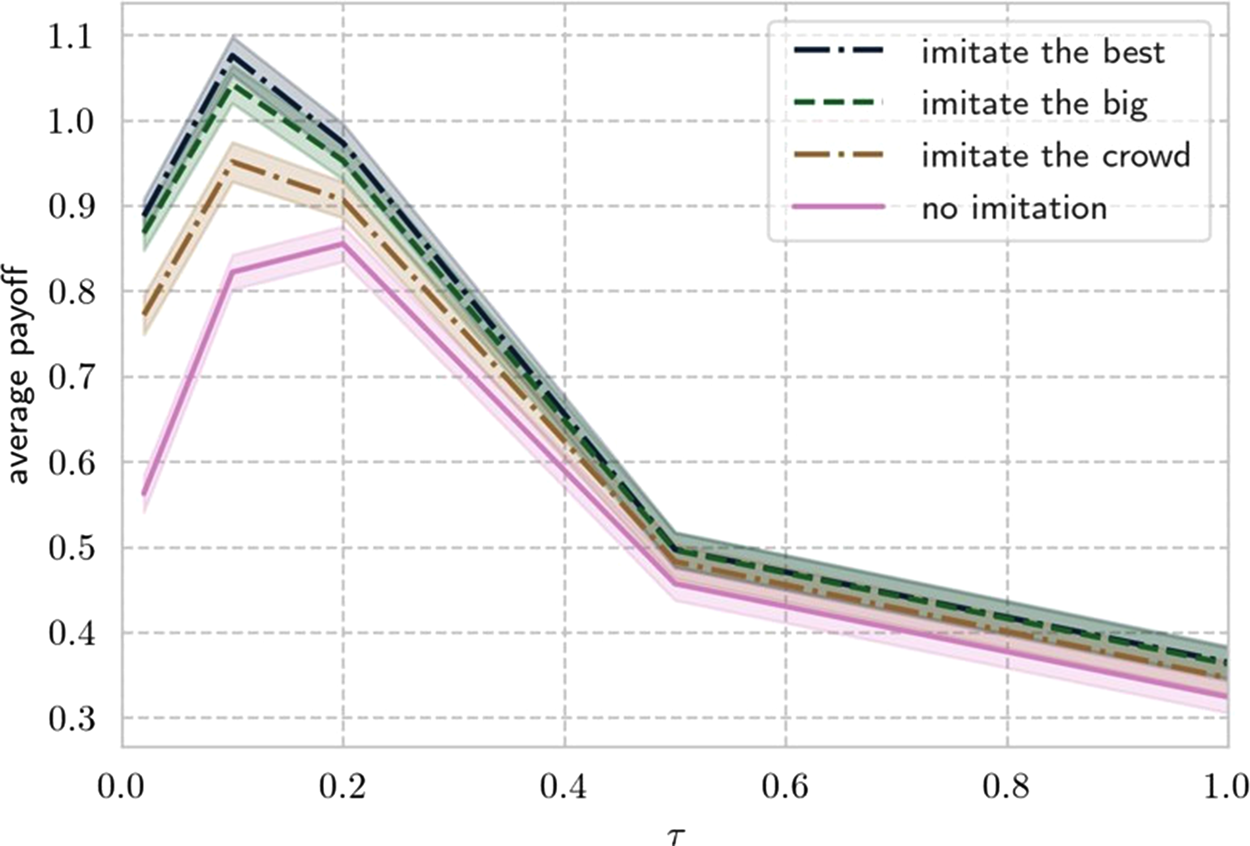
Figure 6. Average performance as a function of the exploration tendency (+). Shaded areas represent the corresponding 95% confidence intervals.
Even in the presence of organizational interdependence, we still observe the classical tradeoff between exploration and exploitation that has been documented in previous studies (Denrell & Fang, Reference Denrell and Fang2010; Levinthal & March, Reference Levinthal and March1993; March, 1991; Posen & Levinthal, Reference Posen and Levinthal2012; Raisch et al., Reference Raisch, Birkinshaw, Probst and Tushman2009). Under all imitation modes, the functions exhibit an inverted-U shape: exploring either too little or too much undermines performance. At low levels of exploration, the firm has little knowledge to exploit; at high levels of exploration, the firm has abundant knowledge, but it is never exploited to enhance performance.
It is interesting to observe that inter-firm learning reduces the level of exploration necessary to achieve the best performance: when only intra-firm learning is allowed, the best exploration strategy is $ = 0.2; when inter-firm imitation is possible, the best exploration strategy reduces to $ = 0.1. In a sense, revealing the choice of a better-performing firm substitutes for a firm's individual exploration. Revealing information improves performance because the most popular choice, as well as the choice of the best-performing firms, is likely to be the optimal choice, and imitation helps the firms to explore and identify the optimal choice. This holds true even when competition reduces the returns from a choice when a large number of firms make the same choice.
Imitation strategies
Figure 7 demonstrates the time series of the average payoffs under different imitation modes and imitation strategies #. Because inter-firm imitation is non-existent in the no imitation treatment, the imitation strategy does not influence behaviors and the function demonstrates a horizontal line. Moreover, the behaviors and performance in all treatments overlap when # = 0. Under all imitation modes and exploration strategies, we observe that the average payoff has an initial jump and then gradually stabilizes. It seems that as the probability of imitation # increases, the average payoff observes a sharper initial increase, but the long-run performance is undermined.
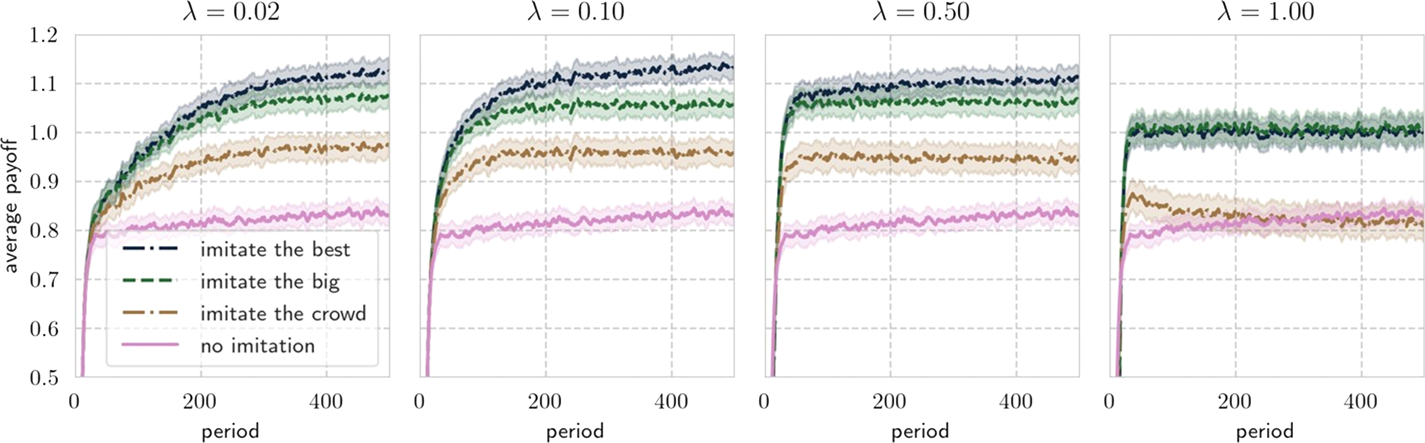
Figure 7. Average payoffs over time under different imitation modes and different imitation tendency. Shaded areas represent the corresponding 95% confidence intervals.
Figure 8 visualizes this tendency by showing the average performance as a function of the exploration strategy (#) separately for the first 50 periods and the last 50 periods. In both time spans, the function exhibits an inverted-U shape: either too much or too little imitation would negatively influence performance. Note that the imitation strategy that maximizes the expected payoff in the first 50 periods is # = 0.5 under all imitation modes, while the strategies that maximize the expected payoff in the last 50 periods is # = 0.02. This temporal comparison provides an important insight: active imitation without individual exploration is likely to be effective in the short run but self-defeating in the long run.

Figure 8. The average performance of the first 50 periods (left panel) and the last 50 periods (right panel) as a function of the imitation tendency. Shaded areas represent the corresponding 95% confidence intervals.
Conformity-differentiation tradeoff
Because of competition, inter-firm imitation and the ensuing conformity cannot guarantee a performance improvement. Figure 9 provides the distributions of choices in the last five periods of each simulation under different imitation modes, along with the optimal distribution of choices that brings the best industry performance.[Footnote 2]
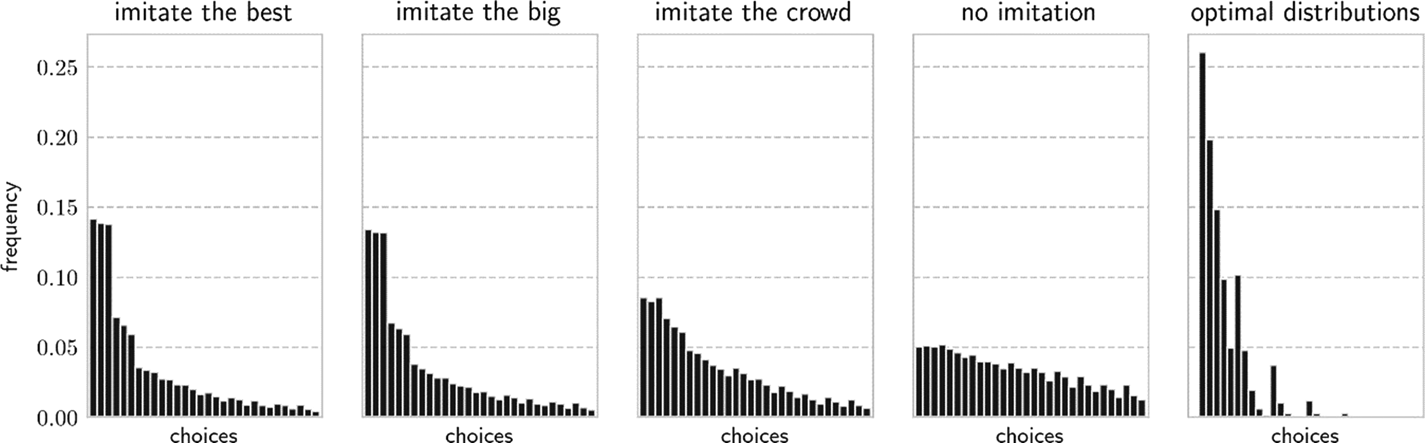
Figure 9. Average distribution of choices under different imitation modes in comparison to the optimal distribution.
Recall that the choices towards the left generally lead to higher expected payoffs, while too many firms adopting the same choice reduce their return because of increased competition. This optimal distribution, which takes into consideration the conformity-differentiation tradeoff, is almost impossible to achieve due to the uncertainty inherent in the bandit problems and the interdependence of firms’ decisions: when one firm switches to another choice, it significantly changes the average return of different choices due to the changed level of competition. It is obvious, however, that inter-firm learning significantly improves the balance between conformity and differentiation: while without imitation, firms place too much weight on the underperforming choices, inter-firm learning significantly shifts the distribution of choices towards the left, closer to the optimal distribution. This shift is more salient under the imitate the best and imitate the big modes than under imitate the crowd.
Figure 10 further demonstrates the expected payoffs given by the corresponding distributions of choices under different imitation modes. Although the expected payoffs with inter-firm learning still fall short of the optimal payoff, it significantly increases the expected payoff compared to intra-firm learning alone in the presence of the conformity-differentiation tradeoff.
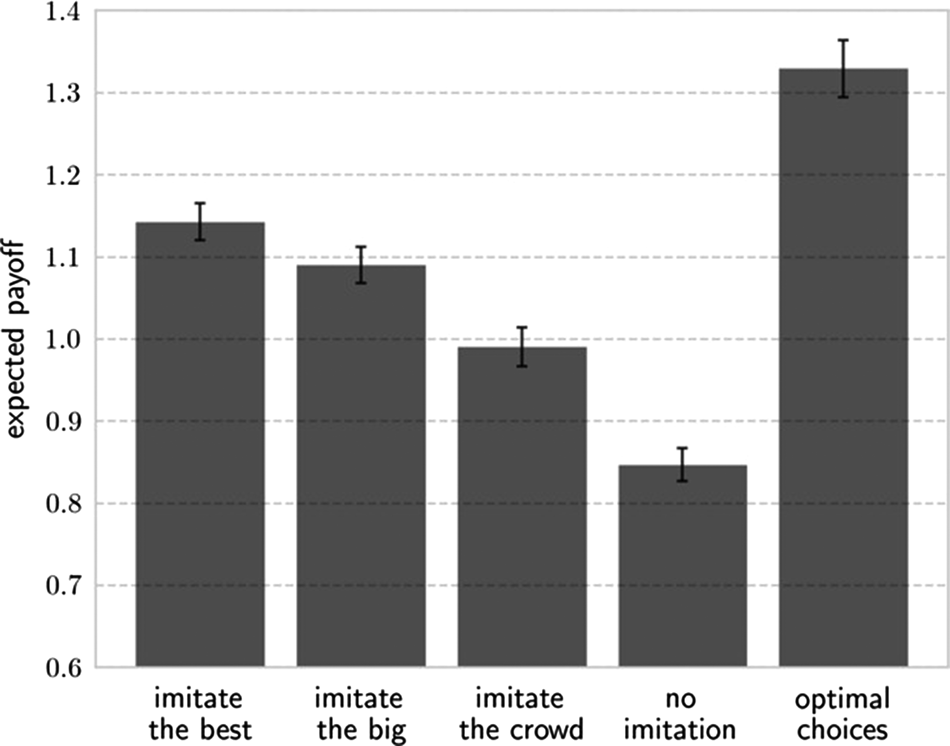
Figure 10. Expected payoffs calculated from the distribution of choices under different imitation modes in comparison to that of the optimal distribution. Error bars represent 95% confidence intervals.
Simulation Results
Our simulation extends previous organizational learning models (Levinthal & March, Reference Levinthal and March1993; March, 1991; Posen & Levinthal, Reference Posen and Levinthal2012) by adding inter-firm learning possibilities, adaptability concerns, and competition. We conduct additional experiments with different hierarchical structures of the bandit problem (! = 60, " = 1, and ! = 12, " = 5) and different specifications for competition. The general conclusions are similar, demonstrating the robustness of the results.
The computational experiments provide strong evidence that inter-firm sharing greatly facilitates the tradeoff between conformity and differentiation, greatly improving the overall performance of individual firms and the industry as a whole.
Several insights can be derived. First, the simulation results confirm Proposition 2: the tradeoff between experimentation and imitation is inherent in the conformity-differentiation paradox, and this tradeoff can be considered the classical exploitation-exploration tradeoff at both the firm and industry levels. A firm can achieve optimal distinctiveness through an appropriate balance between intra- and inter-firm learning. Imitation helps a firm utilize industry knowledge to improve its performance, but if all firms only resort to imitation without independent experimentation, the knowledge pool in the industry will be drained and become ineffective in generating advancement. As shown in Figure 8, overreliance on imitation can help a firm catch up to the leaders in the short-run but undermines its long-run performance.
Second, inter-firm imitation, when its frequency is kept low, helps firms and the industry balance conformity and differentiation. As we have emphasized all along, inter-firm imitation has two opposite effects: it both enhances adaptability and intensifies competition. Our experiment shows, however, that low frequency of imitation helps promote firms’ performance by achieving an appropriate level of distinctiveness. In comparison to the situation in which firms conduct only intra-firm learning (no imitation), when firms engage in inter-firm learning, they adopt technologies that bring higher returns while keeping the negative effect of competition low.
Third, the results show that imitate the best generally outperforms imitate the big. An important insight for managers is to try to identify the firm that recently performed well instead of the leaders with years of reputation or accumulated success. Finally, as Figure 6 shows, even after introducing the competition and adaptability concerns, the tradeoff between exploitation and exploration, as documented by March (March, 1991), still exists, demonstrating the generality and prevalence of this classical ambidexterity issue. We hereby propose:
Proposition 3: Inter-firm learning opportunities tend to help a focal firm achieve its optimal distinctiveness. Yet overreliance on exploiting existing industry knowledge may hurt the focal firm's performance in the long run.
Proposition 4: Imitating the leading firm with the best recent performance can better facilitate the optimal distinctiveness of a focal firm.
DISCUSSION
This study seeks to unfold the conformity-differentiation paradox through the perspective of organizational learning. We focus on a research setting in which, with little prior knowledge, nascent industry firms need to manage the classic organizational ambidexterity issue: i.e., experimenting between various technological trajectories in different technological areas. Meanwhile, as firms strive to strategically position themselves as distinctively as possible while remaining adaptable, they also face the challenge of balancing conformity with differentiation. The tension is probably more salient in nascent technological areas in which innovation diffusion and market acceptance are important for both individual performance and industry development.
The case evidence suggests that the key to managing the conformity-differentiation paradox is to balance exploitation with exploration at the industry level. Technological conformity is suggested at the upstream bottleneck technological domain. We find that the convergence in trajectory choice allows firms to exploit collective experiences at the industry level – just as it helped the mc-Si group to quickly improve its industrial performance and market share. Besides differentiating firms’ choices in the downstream market, firm-level experimentation has an unintended by-product of exploring novel opportunities and enriching the knowledge pool for the whole industry.
The follow-up computational simulation proves the case findings that when imitation opportunities are available, both individual firms and the whole industry can improve their performances. Yet the imitation tendency – or, in other words, the reliance on inter-firm learning – should be kept relatively low, and overreliance on imitation may deteriorate long-run performance. This is in line with Lazer and Friedman's (Reference Lazer and Friedman2007) study on how knowledge dissemination impacts the exploitation-exploration tradeoff under different network structures. Further, our simulation allows us to distinguish and compare different imitation modes and suggests that learning from and adopting the experiences of the organizations with the best recent performance can best help firms to reach optimal distinctiveness.
Reflection on Paradox
The paradoxical opposites – i.e., exploitation vs. exploration, and conformity vs. differentiation – in both the case and the simulation findings are shown to be both conflicting and complementary. For example, differentiation and intra-firm learning, without considering others’ choices and adaptability, may harm competitiveness, while overreliance on inter-firm learning may deteriorate performance. Thus, we argue that it is insufficient to treat the conformity-differentiation balance as an ‘either/or’ choice, or to simply apply the separation mechanism suggested by some paradox literature (e.g., Raisch et al., Reference Raisch, Birkinshaw, Probst and Tushman2009; Schad, Lewis, Raisch, & Smith, Reference Schad, Lewis, Raisch and Smith2016). The ‘either/or’ notion neglects the co-existent nature of paradoxical opposites and fails to leverage the complementarity between them.
As discussed above, most existing optimal distinctiveness studies treat a paradox as a unidimensional continuum: balancing a paradox is to find the optimal cutting point while walking along the tightrope (Deephouse, Reference Deephouse1999; Haans, Reference Haans2018; Zhao et al., Reference Zhao, Fisher, Lounsbury and Miller2017). In contrast, our findings and theoretical propositions suggest that the conformity-differentiation paradox and the exploitation-exploration paradox are multifaceted and multi-dimensional. The paradoxical opposites co-exist at both the intra-firm and the inter-firm levels, and the co-existence persists at different but interdependent technological domains.
The paradox of conformity and differentiation is then reconciled with asymmetrical focus on cross-level learning behaviors. Conformity, i.e., convergence in the upstream bottleneck technologies, relies largely on inter-firm learning; however, intra-firm learning still plays an important role. Similarly, differentiation in downstream product development and market segmentation relies mainly on intra-firm learning, but the achieved divergence is also based on a certain consensus. Demonstrating asymmetrical focuses at the upstream and downstream of the value chain is also in line with the suggestion of Mudambi, Li, Ma, Makino, Qian, and Boschma (Reference Mudambi, Li, Ma, Makino, Qian and Boschma2018).
Further, the study shows the inherent connection between the organizational ambidexterity paradox and the conformity-differentiation paradox: managing the conformity-differentiation paradox relies on balancing exploitation and exploration at cross levels. As March suggested (Levinthal & March, Reference Levinthal and March1993; March, 1991), learning is dynamic and evolutionary in nature. Thus, the conformity-differentiation paradox should also be scrutinized through a dynamic lens. As our simulation results show, firms’ imitation tendencies influence the dynamic interactions between firms in the same industry and give rise to contrasting performances in the short run vs. the long run. That is to say, the benefit of imitation and dominance of the conformity strategy may shift in the long run, demonstrating a transitional mechanism.
Our study, in general, suggests that the conformity-differentiation balance should consider both contradiction and complementarity, which is in line with Smets, Jarzabkowski, Burke, and Spee (Reference Smets, Jarzabkowski, Burke and Spee2015) and Lin, Lu, Li, and Liu (Reference Lin, Lu, Li and Liu2015). This is illustrated in the case and further proven by our simulation results. When considering the interdependence between adaptability and competition, there is an inverted U-shaped relationship between the industry-level exploitation-exploration tradeoff and business performance. The curvilinear relationship indicates that the two opponents of conformity and differentiation are contradictory for tradeoff and complementary for synergy (Li, Reference Li2014). This is also in line with the Chinese wisdom of Yin-Yang (Li, Reference Li2014; Li, Leung, Chen, & Luo, Reference Li, Leung, Chen and Luo2012). As in the Yin-Yang framework, the results from our study demonstrate both the tradeoff and synergy between paradoxical elements, and provide evidence in support of the asymmetrical, transitional, and curvilinear balancing mechanisms (Li, Reference Li2014), especially from the cross-level perspective.
Contributions and Theoretical Implications
Integrating the paradoxes of organizational ambidexterity and conformity-differentiation balance, this study identifies two learning mechanisms across the levels of firm and industry, i.e., intra-firm experimentation and inter-firm imitation. Such learning mechanisms can shed light on how to explain and manage the dynamic and multiplex features of both organizational ambidexterity and conformity-differentiation balance, especially concerning the optimal distinctiveness in strategic balance. Hence, this study contributes to the general research on organization by bridging the theoretical bifurcation between institutional theory and strategy management via organizational learning (McKnight & Zietsma, Reference McKnight and Zietsma2018; Zhao et al., Reference Zhao, Fisher, Lounsbury and Miller2017).
First, we enrich March's exploitation-exploration model by adopting the lens of cross-level learning. March's original model focuses primarily on intra-firm experiential learning, but it is imperative to recognize the salience of organizational interdependence and inter-firm interaction. We incorporate inter-firm learning into March's original model and examine the effects of learning at the cross-levels. Specifically, when experimentation and imitation are possible, the classical balance between exploitation and exploration may exhibit different effects at the firm and the industry levels: while experimentation is often exploitative at the firm level but exploratory at the industry level, imitation is often exploratory at the firm level but exploitative at the industry level. Hence, the enriched learning model helps us obtain a more comprehensive picture and deeper understanding of organizational ambidexterity by linking it with the conformity-differentiation paradox. This is the primary contribution of this study.
Second, the study makes a timely attempt to explain the conformity-differentiation paradox from the perspective of organizational learning. We find that the balance between conformity and differentiation can be reframed as the classical balance between exploitation and exploration across the levels of firm and industry, demonstrating the inherent connection between the paradoxes of organizational ambidexterity and conformity-differentiation balance. Specifically, this study reveals that intra-firm experimentation and inter-firm imitation serve as the two core mechanisms underlying the optimal distinctiveness in strategic balance. In this regard, the three specific mechanisms of yin-yang balancing (i.e., asymmetrical, transitional, and curvilinear balancing) shed light on how to achieve optimal distinctiveness in the conformity-differentiation paradox by specifying the thresholds of appropriate balancing under different boundary conditions. For example, both intra-firm experimentation and inter-firm imitation are like double-edged swords: conformity through inter-firm imitation can improve firms’ performance by facilitating adaptability up to a point, but beyond that point it will reduce firms’ performance by intensifying competition; differentiation through intra-firm experimentation can improve firms’ performance by fostering healthy competition up to a point, but beyond that point it will reduce firms’ performance by suppressing adaptability. Further, the curvilinear tradeoff between exploitation and exploration remains robust when extending March's original model to a cross-level and multi-dimensional setting with adaptability and competition considerations. Hence, March's model has the potential to explain and manage paradoxes other than organizational ambidexterity, such as the paradox of transaction cost and transaction value (Li, Reference Li1998; Li, Li, Liu, & Yang, Reference Li, Li, Liu and Yang2010).
In practical terms, this study also sheds light on how firms in nascent industries should strategize their learning behaviors when faced with both adaptability and competitive differentiation requirements. First, for a nascent industry with limited legacy and prior knowledge, knowledge sharing, even with competitors, can help individual firms, as well as the whole industry, to lower exploration costs and improve performance. Such inter-firm sharing is more relevant to bottleneck technologies or technological domains that are crucial for the formation of industrial standards.
Second, while March highlights the ‘success trap’ caused by staying in the firm's current comfort zone, our findings warn firms to avoid the ‘imitation trap’. When collective experiences are available to be shared, firms should keep the imitation tendency at a relatively low level to ensure long-run performance. Moreover, when few variations exist in an environment with few dominant leaders, lead firms may lure the whole industry into a trap that harms innovation and long-term performance. Although firms tend to follow the long-established giants with accumulated success, our findings suggest following the ‘rising stars’, firms with the best recent performance, regardless of their reputation or size. Take the development of the mobile phone industry as an example. Apple launched the first iPhone in 2007 and drew widespread attention, even though, at that time, Nokia's dominance in mobile phones was undoubtable. For a mobile phone manufacturer, the wise choice was to follow Apple's emerging smartphone trajectory rather than staying in the traditional cellphone camp with giants such as Nokia and Motorola.
Future Research Directions
The study focuses on one specific form of inter-firm learning: imitation. Imitation is closely related to the concept of vicarious learning in the organizational learning literature (Baum et al., Reference Baum, Li and Usher2000; Ingram & Baum, Reference Ingram and Baum1997). Besides, imitating or adopting others’ experiences is in line with the inbound or ‘outside-in’ mode of open innovation (Felin & Zenger, Reference Felin and Zenger2020; West & Bogers, Reference West and Bogers2014). Future research can investigate whom firms should imitate in open innovation and also the optimal degree of openness. This line of research can shed further light on the research streams of absorptive capacity (Cohen & Levinthal, Reference Cohen and Levinthal1990; Zahra & George, Reference Zahra and George2002) and dynamic capabilities (Teece, Reference Teece2007; Teece, Pisano, & Shuen, Reference Teece, Pisano and Shuen1997).
The positive effect of imitation depends on the diversity of the firms in the industry, and the level of diversity in turn depends on how firms balance between imitation and experimentation, as well as how they balance between exploration and exploitation during firm-level experimentation. In the current study, we assume that firms’ tendencies for exploration and imitation are exogenously determined. In order to deepen our understanding of these paradoxes, future studies can allow firms to actively adapt these tendencies while searching for the optimal distinctiveness.
Our computational experiments assume a relatively low intensity of competition across organizations. This is appropriate for an emerging industry such as the PV industry, in which competition across organizations is mild compared to their collective effort to establish adaptability. The Chinese photovoltaic industry is now striving for the adaptability of the whole industry – i.e., the grid-parity – so its leading firms are more willing to share their knowledge to promote the adaptability of both the technology and the industry. As an industry becomes more established, competition within that industry intensifies, the balance may be tilted towards differentiation, although the trade-off between conformity and differentiation still exists. Moreover, in the presence of intense competition, it is questionable that the lead firms of an industry that has already established industry-level adaptability would still be willing to voluntarily share their information and knowledge. Future research can consider the possibility that an organization can choose whether to share its information and how leading firms’ tendency to share can influence the effect of inter-firm learning. In addition, it could take into account the impact of environmental turbulence and investigate the effect of heterogeneity on firms’ learning capacity, imitation tendencies, and aspirational levels.
CONCLUSION
To answer the research question of how firms manage the differentiation-conformity tension through cross-level learning behaviors, we adopted a mixed-method design. We first conducted a case study on the Chinese PV industry to provide a contextual setting for technological trajectory choice and learning. We further conducted a computational simulation to investigate the extent to which firms should rely on inter-firm learning (imitation) and from whom they should learn, in order to manage the conformity-differentiation paradox for the best performance. This study demonstrates that it is highly fruitful to integrate the theoretical streams on institutional theory, strategy, and organizational learning. In particular, the organizational ambidexterity paradox can be linked with the conformity-differentiation paradox to shed light how to enrich the research streams on both paradoxes.
Supplementary Material
The supplementary material for this article can be found at https://doi.org/10.1017/mor.2021.35.