Prehospital and Disaster Medicine (PDM) receives manuscripts in which sampling is used for data acquisition. The validity of the sampling method used for research is an important criterion for ranking quality of a study. This editorial provides an overview of data sampling methods that are common among submissions to PDM.
Sampling techniques are used to select a portion of individuals as a data source to represent the total individuals in a pre-identified population. An example of sampling would be using a process to select 50 disaster victims (study subjects) that are representative of 500 total victims (target population) for a research project. Important in population-based investigation is that a study sample is a valid representation of the total research target population. Sampling is used when it is not possible to be test every individual or element in a study target population.
An important concept in sampling is risk for sampling error, which occurs when the sample selected from among individuals in a general target population erroneously does not represent the entire population. Sampling error can occur due to lack of understanding who should be surveyed within a population (population error), stratification error when an inappropriate qualifier is used to select a sample (sample frame error), selection error in which those selected for a sample are not representative of the target population (selection bias), non-response error in which individuals selected for a sample elect not to participate, variation from or lack of representation of the study target population, and too few subjects in a sample to allow for precision in quantitative measures or complete development of themes.
Only when an entire target population cannot be tested is sampling appropriate. When compared to sampling, testing an entire population provides more valid and accurate data for developing research conclusions. To achieve validity for data obtained from a whole population, sampling must be done in a systematic manner, with an emphasis to avoid: (1) lack of detection of population variation, (2) lack of precision of mean and median measures, (3) lack of detection of important qualitative themes, (4) lack of detection of heterogeneity or homogeneity within the target population, (5) lack of accuracy in inferences made about a target population, and most important, (6) selection bias.
Sampling is used in both qualitative and quantitative research. Depending on the type of research or if mixed methods are used, sampling should be designed to achieve the study objective. Qualitative methods are intended to develop understanding of a population by saturation of themes or information. Quantitative methods are intended to achieve generalizability or conclusions that are representative of a study target population. For each type of research, standards for determining the number of subjects required for a sample to be valid vary. Quantitative methods use established formulae to avoid Type I and Type II errors. Established formulae cannot be applied to qualitative studies, and the number needed for a valid sample is determined by the type of analysis proposed, depth of detail expected, and whether homogeneity (needing smaller samples) or heterogeneity (needing larger samples) is being explored. Two categories of sampling exist, probability and non-probability sampling. An overview of these two sampling methods is provided below.
Probability Sampling
Probability sampling, which is often referred to as random sampling, is any method in which the probability is the same (equal) of including any individual within a target population in a study sample. Because probability sampling limits bias and provides an accurate selection of individuals who likely represent a study target population, it is preferred over non-probability sampling. Four types of probability sampling are common, as well as multi-stage random sampling that uses a combination of any of the four techniques.
Simple Random Sampling—This method of sampling is preferred for research that is designed to describe a target population. Study subjects are selected in a random manner. Most common is assignment of a number or identifier to each individual in the population with those whose number or identifier matches randomly selected numbers or identifiers being selected as study subjects. Random number generation computer programs and charts are readily available. Simple random sampling provides study subjects that are highly correlated to an entire target population. Simple random sampling allows for mathematical calculation of the number of study subjects needed to attain a preferred precision in quantitative study results. On the other hand, a disadvantage of simple random sampling is that each individual in the population must be identified (listed) making use of random sampling of large populations exceedingly tedious and often not possible.
Cluster Random Sampling—To address the disadvantage of identifying all individuals in large populations for simple random sampling, cluster random sampling allows systematically selecting study subjects. Cluster random sampling is commonly used in public health field assessment during health emergencies and disasters. In cluster random sampling, researchers randomly identify areas (such as residential blocks or postal codes) that contain the study target population. Individuals that are within the randomly identified clusters are then potential study subjects. All those within a cluster may be include in a study sample or study subjects may be selected from within clusters using simple random sampling. While more feasible than simple random sampling of large populations, cluster sampling requires that the areas from which clusters are derived are homogeneous with respect to the overall target population. For example, populations with a large degree of heterogeneity (for example in age, wealth, or ethnicity) may produce results with unintended selection bias unless clusters are well-dispersed and enough clusters are included in sampling.
Stratified Random Sampling—This sampling method relies on classifying members of a target population into mutually exclusive groups (such as male/female) and using simple random sampling to select study subjects from the groups. Important is that study subjects be selected based on the size representation of the group - for example if a population contains 60% females and 40% males, the sample should reflect a ratio 6:4 females to males. When done properly, stratified groups from which study subjects are randomly selected are highly representative of an overall target population. Stratified random sampling allows for sampling of a heterogeneous population, overcoming a shortcoming of cluster random sampling. On the other hand, a disadvantage of stratified random sampling is identifying all significant strata (groups) in proper proportion to allow for true representation of the target population.
Systematic Sampling—For systematic sampling, every “Nth” individual from the target population is chosen as a sample study subject. An example would be to form a numbered list of the target population and choose every 10th individual for inclusion in the sample. Another technique used for systematic sampling is to choose every person within a population that has a continuously issued, government-issued identification number that ends in a specific number or letter. Systematic sampling generates samples without need for random number generation, and while representative of a target population, the method is not based on random sampling and not considered as rigorous as randomization strategies. In addition, sample size is important when using systematic sampling to assure validity and precision of data.
Non-Probability Sampling
Non-probability sampling techniques include methods based on researcher judgement or researcher selection of those available and that are presumed to be able to provide data for a study. Therefore, non-probability sampling does not make use of random sampling such that each individual in a population has an equal probability of being included in a study sample. Non-probability sampling cannot be used to infer study findings to a general target population. On the other hand, non-probability sampling allows for identification of themes and patterns to develop an understanding of complex social, behavioral, or cultural phenomena. Essentially, non-probability sampling allows for study inferences relative to those selected as sample subjects. The sample subjects may or may not be representative of a group other than themselves. Non-probability sampling is at-risk for selection bias at a number of different layers, and simply showing demographic similarity of the sample to a general population fails to show that bias is not inherent in the sample. For example, those who self-select to participate in a study sample may have a grievance that is a motivation to participate that is not a common theme within the general population.
Purposeful Sampling Reference Palinkas, Horwitz, Green, Wisdom, Dan and Hanwood1 , Reference Optician, Musa and Alkassim2—Purposeful sampling is a method in which researchers use judgement to identify and select subjects that are knowledgeable about or experienced with a study question or phenomena. Sample subjects must also be available and willing to participate in the study. There are many purposeful sampling designs, with the most common examples as listed below:
Deviant Sampling: subjects or cases are selected in hope of discovering information that is uncommon and that can either show problematic or good findings. This is a type of sampling commonly used in process improvement programs.
Homogeneous Sampling: subjects are selected with the aim of showing similar patterns or characteristics that are dominate among the sample group (often subject experts) relative to the phenomenon of interest. This strategy is also often referred to as dominant pattern sampling. This form of sampling is employed when focus groups are used to generate study information.
Quota Sampling: the aim of this sampling strategy is to develop sample groups that are in the same proportion as a generalized population. The researcher then selects subjects from the groups making sure that the population proportions are maintained. Quota sampling allows for comparison of relationships between the groups selected. Quota sampling is similar to stratified random sampling with the difference that subjects are not identified using random selection, making determination of potential sampling error impossible. With inability to determine sampling error, it is not possible to make inferences to a general study population. Quota sampling groups must be mutually exclusive and possible for a researcher to identify.
Case Sampling: for this strategy, a researcher selects cases from one group with similar characteristics (such as medical cases with one or more of the same diagnostic codes). Cases are selected by the researcher for data extraction without use of randomization of all potential cases available. This strategy is not to be confused with a true case series which includes all available cases (a census strategy). As with other forms of non-probability sampling, case sampling has potential for unmeasurable sampling error.
Sequential (Consecutive) Sampling—This is a form of sampling commonly used for developing qualitative research themes. The technique involves inclusion of sequential subjects or cases until no new information or themes are revealed. This inclusion of subjects to the point that there are apparently no new themes or information to be discovered is commonly referred to as “saturation.” As with other forms of non-probability sampling, subjects or cases are not selected using randomization of all potential members of a population. Therefore, sampling error cannot be determined, which does not allow translation of study results obtained using the sampling method to a general target population. Also, without randomization to select sample subjects, there exist a possibility that new themes or information may not be discovered as the next case may provide more information that was not included in the sample after presumed saturation.
Theoretical Sampling—Theoretical sampling occurs as data collection proceeds. First, a researcher develops the research objectives and then identifies a group to discuss or be interviewed about the research question using pre-established discussion or interview criteria. Following the initial session for the sample (such as in a grounded theory study), the researcher will analyze and organize information obtained. Using the findings developed in the first session, the researcher will select a second sample of subjects to discuss and consider or be interviewed regarding the findings for the first session. The second sample may confirm or disconfirm the findings from the first session. Findings from the second and first sessions are compared and combined to refine study information and may be presented to a third sample or the original first session subjects for their confirmation. Theoretical sampling continues moving alternately from sampling, data collection, and analysis, until the researcher determines data saturation has occurred. A common type of theoretical sampling is the Delphi Method, which allows for a low-cost, less timely approach to a research objective. On the other hand, theoretical research is based on investigator judgement to identify and select sample subjects that are knowledgeable about or experienced a study topic, and are also available and willing to participate. As with purposeful sampling, this leads to potential sample error that cannot be measured.
Snowball Sampling—Snowball sampling uses individuals chosen by a researcher to refer acquaintances or other known potential subjects to develop a final sample. As with sequential sampling, the snowball process is continued until theme or information saturation is determined by the researcher. Snowball sampling depends on social connections, bibliographies, or social media rather than systematic selection of sample subjects. The method allows for research of groups that are not easily identified or represented in lists that are available to the researcher. An example of such a group would be illegal drug users likely to lose access to drugs and suffer withdrawal during a disaster event. As with other forms of non-probability sampling, snowball sampling is at-risk of sample error that cannot be measured.
Convenience Sampling Reference Optician, Musa and Alkassim2—This is a common form of non-probability sampling in which a researcher selects sample subjects based on the availability of potential participants or records. Often, an invitation to participate in a study is offered to a number of individuals who may meet study inclusion criteria. Those who volunteer to participate are included in the sample group (common with online or conference attendee recruitment of sample subjects). Convenience sampling is inexpensive and less time consuming than most sampling strategies, but the method is prone to selection bias and personal biases among those who respond to recruitment. There is also potential for confounding factors based on self-selection bias among individuals who volunteer to participate versus those who do not participate. Sampling error cannot be measured when using convenience sampling.
Summary
Table 1 summarizes the advantages and disadvantages for probability and non-probability sampling methods. When possible, probability sampling is preferred for studies aimed to generalize or infer the findings to a general target population. Probability sampling is usually more rigorous and difficult when compared to non-probability sampling. Sampling error can be estimated when using probability sampling to establish validity of study results.
Table 1. Probability and Non-Probability Sampling Advantages and Challenges
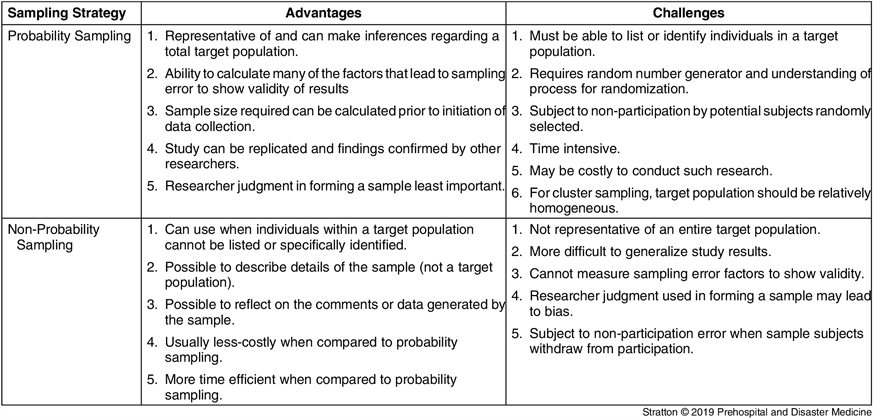
Non-probability sampling is often more cost effective and time efficient. When an entire study target population cannot be identified or listed for randomization, non-probability sampling may be the only way in which to address a study objective. Because of unmeasurable sampling error and uncontrolled confounding variables, data and conclusions derived from non-probability sampling are not considered adequate for describing a general target population, rather non-probability sampling data can only describe and allow conclusions for the study sample.