INTRODUCTION
The process of spoken word recognition requires the listener to match acoustic input to existing linguistic representations in memory. As a listener perceives a word’s initial acoustic information, multiple lexical candidates are concurrently activated and compete against one another for selection (see Cutler, Reference Cutler2012 for a review). During this early stage of lexical processing, a listener may also draw on knowledge of statistical regularities about the sound patterns of words (Dahan et al., Reference Dahan, Magnuson and Tanenhaus2001; McQueen & Cutler, Reference McQueen, Cutler, Hardcastle, Laver and Gibbon2010). For a second language (L2) learner, spoken word recognition in the L2 requires learning novel speech cues and building the statistical knowledge of how often those multidimensional cues occur and co-occur with other cues to form sound categories, phonotactics, and words (e.g., Ellis, Reference Ellis2002, Reference Ellis, Gass and Mackey2011; Escudero & Boersma, Reference Escudero and Boersma2004; Hayes-Harb, Reference Hayes-Harb2007; Holt & Lotto, Reference Holt and Lotto2006; Pajak et al., Reference Pajak, Fine, Kleinschmidt and Jaeger2016).
Previous L2 speech learning research investigating adult learners’ acquisition of novel speech sounds has identified challenges stemming from a late age of acquisition, a perceptual system attuned to first language (L1) input, and limited L2 input in a classroom setting (see Colantoni et al., Reference Colantoni, Steele, Escudero and Neyra2015 for a review). This research has established that L2 learners often struggle to phonetically discriminate novel speech sounds, particularly those produced by unfamiliar talkers whose acoustic realizations of speech may differ considerably from realizations previously encountered (e.g., Barcroft & Sommers, Reference Barcroft and Sommers2005, Reference Barcroft and Sommers2014; Hardison, Reference Hardison2003; Lecumberri et al., Reference Lecumberri, Cooke and Cutler2010; Lively et al., Reference Lively, Logan and Pisoni1993; Wade et al., Reference Wade, Jongman and Sereno2007). This inability to accurately discriminate new L2 speech sounds may cause learners to activate spurious lexical competitors, which ultimately results in slower or inaccurate L2 spoken word recognition (Weber & Cutler, Reference Weber and Cutler2004, Reference Weber and Cutler2006).
Given the challenges associated with recognizing L2 speech, statistical information about the probability of novel L2 speech cues may be highly beneficial for learners. In other words, information about the likelihood of sound occurrences and co-occurrences may facilitate word recognition in the absence of sophisticated L2 perception. Here we examine this claim with respect to a specific type of input learning, “dimension-based statistical learning” (Idemaru & Holt, Reference Idemaru and Holt2011, Reference Idemaru and Holt2014, Reference Idemaru and Holt2020), which reflects listeners’ ability to track multidimensional speech cues such as the statistical regularity of CV strings (where C and V refer to consonants and vowels, respectively) and the acoustic dimensions that define language units larger than the phoneme. Because talker variability can affect L2 learning (e.g., Barcroft & Sommers, Reference Barcroft and Sommers2005), we also examine how variability in the speech signal affects naïve listeners’ reliance on statistical information for L2 spoken word recognition. We additionally clarify whether explicit instruction on particular speech cues (e.g., Saito, Reference Saito2011, Reference Saito2015) is beneficial to L2 learners. An investigation into the effect of explicit instruction on speech sounds may also benefit L2 educators, particularly those who teach speech sounds that are typically difficult for adult learners to acquire.
To address these questions, this eye-tracking study tests (1) whether adult listeners unfamiliar with lexical tone learn statistical regularities of syllable-tone co-occurrences, (2) whether multitalker speech affects the reliance on statistical information for online L2 word recognition, and (3) whether explicit instruction on lexical tone contours facilitates this statistical learning.
BACKGROUND AND MOTIVATION
DIMENSION-BASED STATISTICAL LEARNING OF SYLLABLE-TONE CO-OCCURRENCES
Mandarin Chinese (hereafter “Mandarin”) provides a relatively consistent mapping from the word to the written form to the morpheme to the spoken syllable (Myers, Reference Myers2010; Packard, Reference Packard1999, Reference Packard2000). As an example, the word “horse” can be written with one character, 马, which corresponds to one morpheme, and which consists of one syllable: ma. Hereafter, we use “syllable” to refer to a segmental string irrespective of its tone. Because a syllable-tone combination like ma3 is the minimal Mandarin morpheme or word, we use “word” hereafter to refer to a specific syllable-tone combination.
Each of the nearly 400 (C)V(C) Mandarin syllables varies in terms of its token frequency where the frequency of an item is inversely proportional to its frequency rank in a natural corpus (e.g., the tenth most likely word appears .1 times as often as the most likely one; DeFrancis, Reference DeFrancis1986; Duanmu, Reference Duanmu2007, Reference Duanmu2009; Zipf, Reference Zipf1935, Reference Zipf1949). In Mandarin, for example, the 30 most frequent syllables account for roughly 50% of the tokens in a 46.8-million-character speech corpus (SUBTLEX-CH: Cai & Brysbaert, Reference Cai and Brysbaert2010). In contrast, the 100 least frequent syllables account for approximately 1% of the corpus’ tokens. Hereafter, references to frequency signify “token frequency.” Mandarin word recognition thus involves repeatedly activating a small subset of highly frequent syllables and occasionally activating mid- to low-frequency syllables. Native speakers learn from this asymmetric distribution of syllables in speech and tend to perceive and produce frequent syllables faster than infrequent syllables (Chen et al., Reference Chen, Chen and Dell2002, Reference Chen, Lin and Ferrand2003; Zhou & Marslen-Wilson, Reference Zhou and Marslen-Wilson1994) as do classroom L2 learners (Wiener et al., Reference Wiener, Lee and Tao2019; Wiener & Lee, Reference Wiener, Chan and Ito2020).
In speech, each Mandarin syllable can be produced with up to four different lexical tones (Gandour, Reference Gandour1983; Howie, Reference Howie1976). Figure 1 plots the four tones with their canonical fundamental frequency (F0) contours when spoken in isolation. Importantly, the segmental sequence composing each syllable constrains the probability of the four tones. For example, gei only forms a Mandarin word with the dipping third tone as gei3. Gei1, gei2, and gei4 are all nonwords. Another example is the syllable zhou, which forms a word with all four tones. Yet, roughly 90% of all spoken zhou tokens in SUBTLEX-CH appear with the first tone as zhou1. L1 Mandarin speakers’ linguistic knowledge therefore includes which syllable-tone combinations correspond to words, which combinations are nonwords, and which combinations are most likely to occur in speech (Fox & Unkefer, Reference Fox and Unkefer1985; Wang, Reference Wang1998; Wiener & Turnbull, Reference Wiener and Turnbull2016).
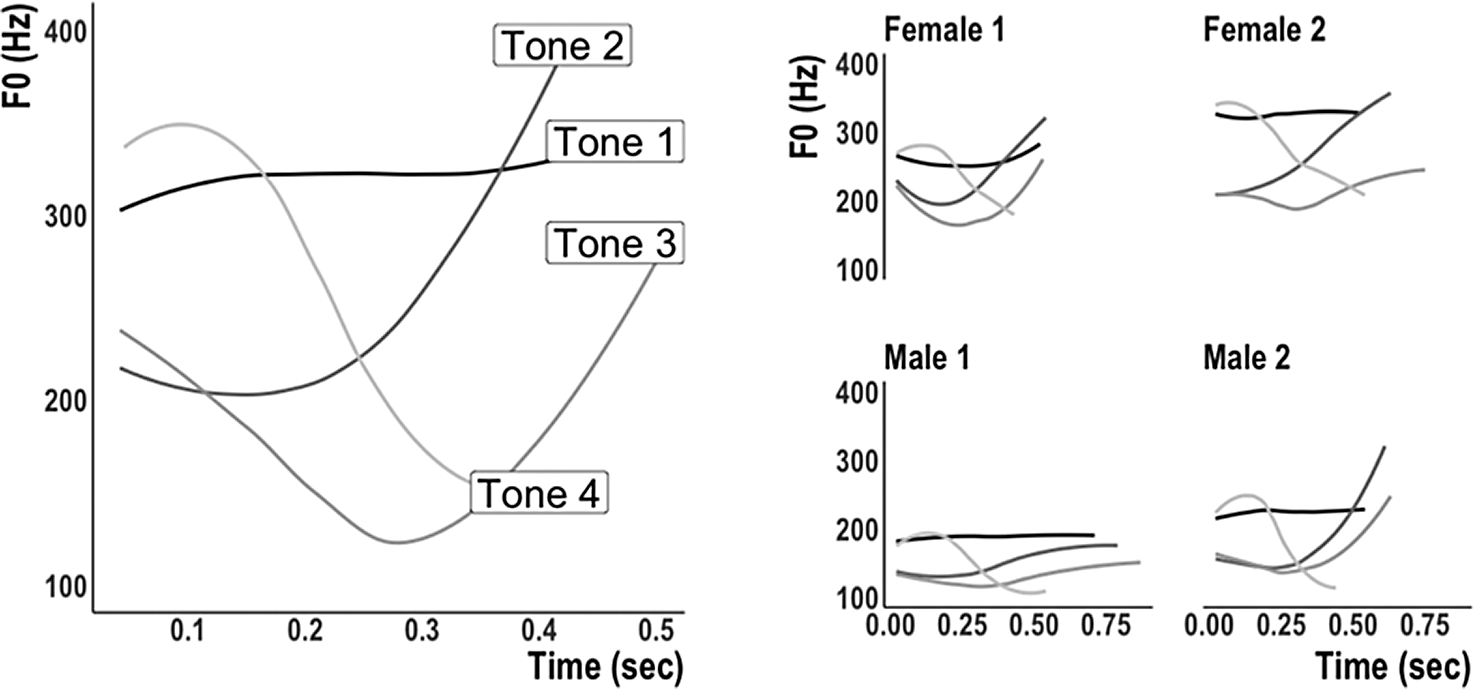
FIGURE 1. Four Mandarin tones spoken in isolation by a native female talker (left). Artificial language tones spoken in isolation by four talkers (right).
Such statistical information directly contributes to L1 Mandarin word recognition. In an eye-tracking study, Wiener and Ito (Reference Wiener and Ito2015) demonstrated that native Mandarin speakers draw on this syllable-tone co-occurrence probability information during the early stages of spoken word recognition. Participants were simultaneously presented with an array of four frequency-controlled Chinese characters and an auditory stimulus corresponding to one of them. The experimental manipulation involved a target (e.g., zhou2) and a tonal competitor, which shared the target’s syllable but differed in tone (e.g., zhou1). Upon hearing a syllable’s initial acoustic information, such as the onset of zhou, listeners initially looked to the character with the most probable tone (e.g., zhou1). For infrequent syllables, these predictive looks regularly occurred even if the tone of the competitor was incongruent with the incoming acoustic information (e.g., zhou2, which starts with a distinctively lower onset F0 than zhou1; see Figure 1). This effect of tonal probability, however, was short-lived—starting from roughly 300 ms to 700 ms after the onset of the syllable—and looks to the highly probable competitor were significantly higher only for infrequent target syllables. Wiener and Ito, consistent with previous research on homophony (e.g., Luce & Pisoni, Reference Luce and Pisoni1998; Vitevitch & Luce, Reference Vitevitch and Luce1998, Reference Vitevitch and Luce1999; Vitevitch et al., Reference Vitevitch, Luce, Pisoni and Auer1999), claimed that tone is less informative for the identification of highly frequent Mandarin syllables with a large number of homophonous morphemes. As an example, yi—the ninth most frequent syllable in SUBTLEX-CH—with the falling fourth tone can be written with nearly 100 semantically and orthographically distinct characters (Yin, Reference Yin1984). Tonal information may not help select specific yi lexical candidates because word identification for frequent syllables like yi typically requires additional context or morphemes (Packard, Reference Packard1999, Reference Packard2000; Zhou & Marslen-Wilson, Reference Zhou and Marslen-Wilson1994, Reference Zhou and Marslen-Wilson1995). In contrast, tone is more informative for the identification of infrequent syllables like zhou, which appear with far fewer homophones in sparse neighborhoods (e.g., Chen et al., Reference Chen, Vaid and Wu2009; Li & Yip, Reference Li, Yip, Leong and Tamaoka1998; Wiener & Ito, Reference Wiener and Ito2016).
Thus, L1 Mandarin speakers make use of syllable-tone dimension-based statistical information for online word recognition. Recent findings indicate that intermediate L2 classroom learners familiar with lexical tone appear to exhibit similar dimension-based statistical learning of a CV-syllable and tone (Wiener et al., Reference Wiener, Ito and Speer2018). L1 English adults enrolled in an intermediate L2 Mandarin language course took part in a multiday artificial tonal language-learning task (e.g., Caldwell-Harris et al., Reference Caldwell-Harris, Lancaster, Ladd, Dediu and Christiansen2015; Wewalaarachchi et al., Reference Wewalaarachchi, Wong and Singh2017). The syllable-tone input was designed to mimic Mandarin’s varying syllable frequency and conditional tone probabilities. After four consecutive days of training, L2 learners demonstrated comparable statistical experience-based early looks to more probable targets given the perceived syllable. Like the native speakers tested in Wiener and Ito (Reference Wiener and Ito2015), the L2 learners’ predictive looks were primarily observed for low-frequency syllables. These looks were rapidly shifted to the correct symbols if the initial probability-based prediction was incongruent with the acoustic information.
What remains unclear is whether knowledge of lexical tone is necessary for learners to track its statistical regularities. Statistical learning of a nonnative speech cue may only emerge only once learners are consciously aware of the novel cue’s importance in word recognition. The present study serves as a follow-up to Wiener et al. (Reference Wiener, Ito and Speer2018) to examine whether adult listeners unfamiliar with lexical tone, that is, the typical adult learner in a beginner Mandarin classroom language course, can learn statistical regularities involving syllable-tone co-occurrences with conscious attention.
TALKER VARIABILITY AND L2 TONAL WORD RECOGNITION
The present study additionally investigates how variability in the speech signal affects L2 learning and word recognition. With respect to Mandarin tones, previous research established that learners struggle to distinguish between low-F0-onset tones 2 and 3 and high-F0-onset tones 1 and 4, even after extended L2 classroom experience (e.g., Hao, Reference Hao2012, Reference Hao2018; Leather, Reference Leather1983; Pelzl, Reference Pelzl2019; Pelzl et al., Reference Pelzl, Lau, Guo and DeKeyser2019). For many L2 learners, this perceptual confusion increases when faced with multitalker speech. Figure 1 (right) illustrates how the same four syllable-tone productions vary across talkers in their F0 onset, contour, and range, a circumstance that could lead to tonal errors among L2 learners (e.g., Chang & Bowles, Reference Chang and Bowles2015; Lee et al., Reference Lee, Tao and Bond2009, Reference Lee, Tao and Bond2010, Reference Lee, Tao and Bond2013; Qin et al., Reference Qin, Tremblay and Zhang2019; Shen & Lin, Reference Shen and Lin1991).
Wang et al. (Reference Wang, Spence, Jongman and Sereno1999) first explored whether exposure to more varied, multitalker input improves beginner L2 Mandarin learners’ tone categorization. Participants heard Mandarin syllable-tone words from four talkers and were asked to categorize the speech according to its tone type. After 2 weeks of multitalker training, participants improved in their overall tone categorization. This learning even generalized to new tokens and speakers. Yet, follow-up multitalker studies (Perrachione et al., Reference Perrachione, Lee, Ha and Wong2011; Sadakata & McQueen, Reference Sadakata and McQueen2014) that controlled for individual tonal aptitude found that only learners with strong perceptual abilities benefited from greater talker variability—those learners with weak abilities saw little improvement (though see Dong et al., Reference Dong, Clayards, Brown and Wonnacott2019 for conflicting results).
Whereas greater talker variability may improve L2 tonal categorization, limited evidence suggests it may also cause uncertainty during L2 spoken word recognition. The L2 learners tested on Wiener et al. (Reference Wiener, Ito and Speer2018)’s artificial language demonstrated a greater reliance on tonal probability information when talker variability increased. Learners trained and tested on input from four talkers across the four days of training looked more to probable targets and recovered more slowly from incorrect predictions than those trained and tested on input from the same talker. The authors argued that as learners’ uncertainty about the tone category increased with multitalker input, they relied more on their statistical knowledge of syllable-tone co-occurrence to predict the intended target.
Thus, for intermediate L2 learners still learning to categorize tones, reliance on tonal probability information increased when faced with greater acoustic variability in the input. Because neither Wang et al. (Reference Wang, Spence, Jongman and Sereno1999) nor Wiener et al. (Reference Wiener, Ito and Speer2018) tested truly naïve listeners, the current study aims to clarify how multitalker input affects naïve listeners’ learning of syllable-tone words and their reliance on statistical information for spoken word recognition.
STATISTICAL LEARNING OF TONE AND EXPLICIT INSTRUCTION
The present study looks for evidence to support an innate statistical learning mechanism that facilitates language learning and processing using a process of extracting distributional information for sounds occurring in the continuous speech signal (Gómez & Gerken, Reference Gómez and Gerken2000; Maye et al., Reference Maye, Werker and Gerken2002; Saffran, Reference Saffran2003). Whereas the majority of research on the statistical learning of L2 speech sounds has focused on consonants and vowels (e.g., Escudero et al., Reference Escudero, Benders and Wanrooij2011; Wanrooij et al., Reference Wanrooij, Escudero and Raijmakers2013), we test whether this mechanism extends to the statistical learning of lexical tones.
Previous research on statistical learning of tone suggests that listeners can learn distributional regularities of words with tones, but it remains unclear how tonal information is represented in leaners’ lexicons. A study by Wang and Saffran (Reference Wang and Saffran2014) paired nine unique artificial tones with nine CV syllables to form three trisyllabic tonal words (e.g., bidatu with each syllable carrying a different tone contour). Adult participants were instructed to listen to a 9-minute continuous stream of speech containing these words. Learners could therefore track regularities involving the syllable-only, the tone-only, or the syllable-tone combinations as “words.” After the familiarization phase, participants heard a word from the training along with a new word that reversed the order of the syllables (e.g., tudabi). Participants had to indicate which of the two CV strings sounded more familiar. Monolingual English listeners performed the task at chance, whereas monolingual Mandarin listeners successfully discriminated previously heard words from nonwords (though the two monolingual groups did not differ statistically). Interestingly, two groups of bilinguals (Mandarin–English bilinguals and English and a nontonal L2 bilinguals) both discriminated words from nonwords better than the two monolingual groups. The authors concluded that experience with multiple languages—irrespective of whether the languages are tonal—resulted in an increased ability to discover patterns in new stimuli.
A follow-up study by Potter et al. (Reference Potter, Wang and Saffran2017) used the stimuli and task of Wang and Saffran (Reference Wang and Saffran2014) to test two groups of listeners: a monolingual English control group and an L1 English group currently learning Mandarin. The two groups discriminated words from nonwords with nearly identical accuracy levels of 55%. When tested 6 months later, the participants enrolled in the L2 Mandarin course improved to 66% while the monolingual control group remained slightly above chance (53%). Potter et al. argued that this improvement demonstrates that the L2 learners transferred their classroom experience to the statistical learning task. However, it is unclear what cues the participants were tracking: syllable regularities, tone regularities, or syllable-tone regularities.
Critically, the stimuli used in Wang and Saffran (Reference Wang and Saffran2014) and Potter et al. (Reference Potter, Wang and Saffran2017) presented syllables with the same tone, that is, there were no phonotactically identical sequences distinguished only by tone. Therefore, tones from their artificial language did not signal lexical contrast. Evidence from neuroimaging and eye-tracking data (Malins & Joanisse, Reference Malins and Joanisse2010, Reference Malins and Joanisse2012) suggests that lexical tone processing, where sound is associated to symbol and thus used for word identification, may fundamentally differ from nonlexical tone processing. The present study manipulates the tonal distribution separately from the syllable distribution to clarify whether novice listeners can extract statistical regularities of tone-syllable co-occurrences and use such information for online word recognition involving sound-symbol pairs. As we outline in the following text, this approach involves training participants on a larger set of items to set up the distribution of tone-syllable correspondences, and then testing participants with a smaller critical set of items to see the effect of frequency of co-occurrence.
The present study additionally aims to test the effect of explicit instruction on lexical tone acquisition and statistical learning. Previous research reports that adult performance in statistical learning tasks improves when explicit instructions to attend to the stimuli are given (e.g., Ong et al., Reference Ong, Burnham and Escudero2015; Saffran et al., Reference Saffran, Newport, Aslin, Tunick and Barrueco1997; Turk-Browne et al., Reference Turk-Browne, Scholl, Johnson and Chun2010). Ong et al. (Reference Ong, Burnham and Escudero2015) trained naïve L1 English listeners on Thai tonal minimal pairs and measured participants’ ABX discrimination before and after training. Participants improved their statistical learning of tone, but only if their auditory attention was encouraged using a cover task. Thus, statistical learning may be more effective if learners are instructed to attend to specific acoustic dimensions that matter for learning novel words. Ong et al.’s finding may also explain why the intermediate L2 participants tested in Wiener et al. (Reference Wiener, Ito and Speer2018) were able to track syllable-tone co-occurrences: These learners had more than a year of explicit Mandarin classroom training and were clearly aware of tonal cues in the stimuli. However, as with the Potter et al. (Reference Potter, Wang and Saffran2017) and Wang and Saffran (Reference Wang and Saffran2014) stimuli, the Ong et al. stimuli were not lexical in nature and participants’ learning was assessed with phonetic discrimination tasks only. Thus, listeners may not have categorized tones as lexical cues. The present study makes the lexical function of tone overt to listeners using sound-symbol pairs, and tests whether explicit instruction facilitates acquisition of lexical tone and its statistical regularities.
RESEARCH QUESTIONS
RQ1. To what extent does listeners’ experience with lexical tone affect their dimension-based statistical learning of syllable-tone co-occurrences? Can adult listeners unfamiliar with lexical tone learn syllable-tone statistical regularities?
RQ2. To what extent does multitalker input modulate the reliance on statistical information for online L2 spoken word recognition? Do learners exposed to multiple talkers rely on statistical knowledge to a greater degree than learners exposed to only one talker?
RQ3. To what extent does explicit instruction of lexical tone facilitate dimension-based statistical learning? Can naïve listeners who are not explicitly aware of lexical tone still track syllable-tone statistical regularities?
EXPERIMENT
METHOD
Participants
Eighty native speakers of American English with no previous experience in Mandarin or another tonal language participated in the study (M age = 20.9, SD = 4.0). All participants were undergraduates at a Midwestern university and had normal or corrected vision, normal speech and hearing, and no formal musical training. Tonometric (Mandell, Reference Mandell2015) was used to control for pure pitch perception.1 All participants had previously studied at least one nontonal European language during secondary school (e.g., French, German, Italian, Russian, or Spanish). Participants were asked to self-rate their L2 abilities on a 4-point Likert scale (1: beginner; 4: fluent: M speaking = 2.1, SD = 0.8; M listening = 2.1, SD = 0.7). No participant self-rated as a fluent speaker or listener of an L2 or studied another language at the time of testing. All participants were paid for their time.
Materials
Eight consonants /p, ph, f, k, kh, m, ts, ɹ/ and six vowels /a, i, ə, ia, iu, ai/ were used to construct 24 Mandarin-like CV syllables (see Supplementary Materials for full stimuli). Each syllable was paired with four tonal contours similar to those in Mandarin (Figure 1). Only 82 of the possible 96 syllable-tone co-occurrences were used to approximate the natural rate of syllable-tone gaps within the Mandarin lexicon (see Myers, Reference Myers2002, Reference Myers2010; Wang, Reference Wang1998). To introduce homophony within the artificial language, 48 syllable-tone homophones were added, resulting in 130 total nonce words—a rate of homophony approximating that of spoken Mandarin (see Duanmu, Reference Duanmu2007, Reference Duanmu2009). Each nonce word was given a unique symbol (Figure 2). These nonce symbols—like Chinese characters—served to disambiguate homophonous syllable-tone co-occurrences (see Wiener, Reference Wiener2015 for details on symbol creation). This meant that some syllable-tone combinations mapped to multiple symbols whereas other syllable-tone combinations only mapped to one symbol.
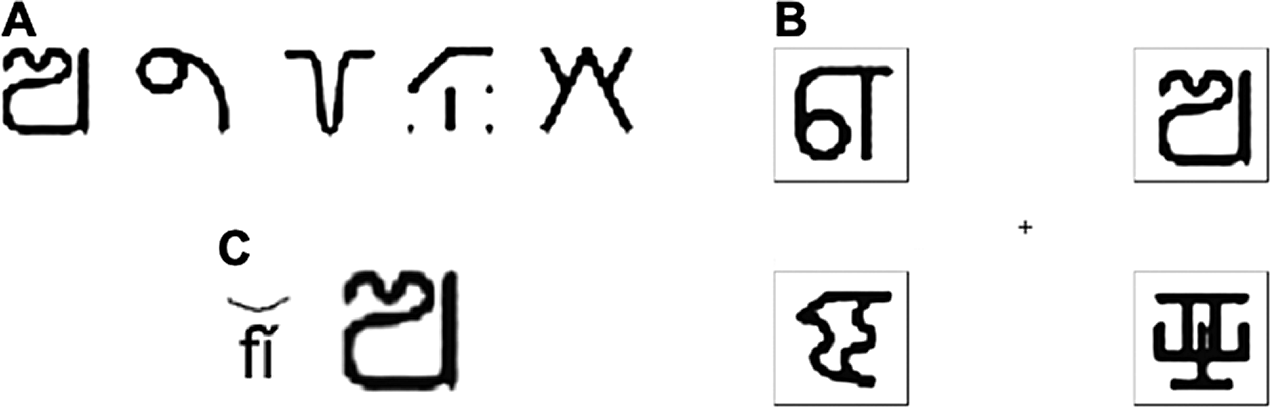
FIGURE 2. A: Example of five fi3 homophones. B: Sample 4-AFC slide; target (fi1, top-left), tonal competitor (fi3, top-right), rhyme competitor (ri1, bottom-right), and distractor (ka2, bottom-left). C: Example of explicit training slide for fi3.
To manipulate the statistical distribution of syllables and tones within the artificial language, 66 of the 130 items in the language served as fillers. The remaining 64 items served as critical items. Among the 64 critical items, two factors—syllable token frequency and syllable-conditioned tonal probability—were crossed to make four within-subject stimulus conditions. Syllable frequency was manipulated by increasing or decreasing the number of syllable tokens to which participants were exposed, irrespective of tone. Thirty-two of the 64 test items had high syllable frequency (F+: 28 tokens per day for a total of 896 tokens) while the other 32 items had low syllable frequency (F−: 16 tokens per day for a total of 512 tokens). For each syllable, a particular tone type was assigned to be the most probable (P+; occurring in 50% of the tokens) while another tone type served as the least probable (P−; occurring in roughly 10% of the tokens); the other two tones had identical mid-range probabilities. These frequency and probability values approximated those used in Wiener and Ito (Reference Wiener and Ito2015) while providing a reasonable amount of input for participants to learn the artificial language based on pilot results. Figure 3 illustrates the input to participants. Each syllable-tone input varied in the number of symbols to which it mapped, which presumably affected participants’ certainty about the identity of the target.
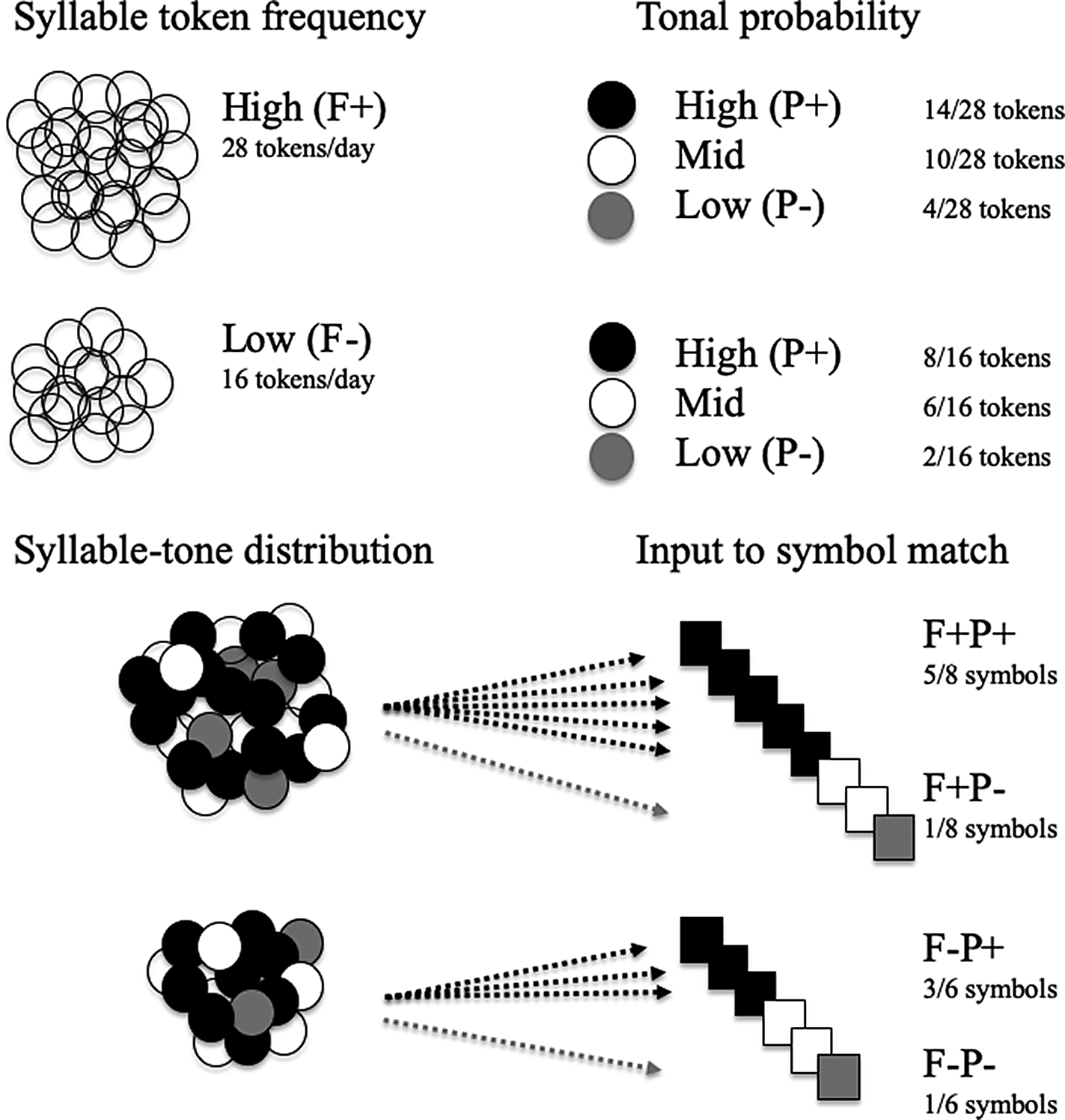
FIGURE 3. Illustration of syllable-tone input and match to symbols.
These frequency and probability manipulations resulted in four within-subject stimulus conditions: F+P+, F+P−, F−P+, F−P−. Filler homophones were used to manipulate tonal probabilities, which allowed for the target test items to visually appear the same number of times. For example, on each day of training and testing, the syllable-tone combination fi3 was presented 10 times using the four rightmost nonce symbols in Figure 2A. By continually showing participants these four symbols throughout the experiment, the probability of the syllable fi appearing with tone 3 greatly increased. The leftmost nonce symbol in Figure 2A functioned as the test item and visually appeared four times each day, which is the same number of times as the three respective critical item symbols for fi1, fi2, and fi4. Thus, the critical test items’ symbols appeared with identical frequency while the phonological occurrence of a syllable with a particular tone varied through manipulation of filler homophone symbols.
All auditory stimuli were recorded by four (two male and two female) native Mandarin talkers at 16 bits/44,100 Hz. Figure 1 (right) shows an example of fi1, fi2, fi3, and fi4 produced by each talker. Acoustic analysis of the stimuli confirmed previously reported temporal differences; tones 2 and 3 were longer in duration than tones 1 and 4 (Ho, Reference Ho1976; Moore & Jongman, Reference Moore and Jongman1997; Zee, Reference Zee1980). Mean duration of each tone type was comparable across the four talkers: tone 1 (F(3,96) = 0.166, p = 0.69); tone 2 (F(3,96) = 0.825, p = 0.37); tone 3 (F(3,96) = 3.28, p = 0.08); and tone 4 (F(3,96) = 0.114, p = 0.73).
Design and Procedure
To control for the potential influence of particular sound-symbol pairs on learning, participants were randomly assigned to one of two presentation lists that counterbalanced sound-symbol mapping. Participants took part in 30-minute training-testing sessions on four consecutive days thereby allowing for overnight consolidation of word learning (Qin & Zhang, Reference Qin and Zhang2019). Participants performed the tasks in the same order each day: passive listening, shadowing, naming (with feedback), and 4-alternative forced-choice (4-AFC) with eye-tracking (with feedback).2 Figure 4 shows the daily training and testing routine.

FIGURE 4. Daily training and testing routine.
Participants first completed a self-paced passive listening task (131 trials). They were seated in a sound-attenuated booth with a computer monitor and given headphones. A symbol and its audio label were simultaneously presented. Participants were told to remember the sound-symbol pair and mouse click to advance to the next trial. Participants next shadowed the speech (131 trials; because the results are beyond the scope of this article, see Wiener et al., Reference Wiener, Chan and Ito2020 for discussion). After a sound-symbol pair was presented, participants were asked to repeat the perceived word as clearly and accurately as possible. The symbol remained on screen until the participant mouse clicked to advance to the next trial. After completing the shadowing task, participants performed a naming task (64 trials). These trials, which only tested the 64 critical items in the language, first presented a symbol on screen. Participants were told to produce the symbol’s syllable-tone as accurately as possible and to guess if they were unsure. The symbol remained on screen until the participant mouse clicked, at which point feedback about the correct audio label was presented using headphones. Participants ended each day of training with the 4-AFC task, which is the focus of this article.
In the 4-AFC task, participants’ eye movements were first calibrated using the Clearview 5-point calibration program. At the start of each trial, participants fixated on the center of the screen while listening to a carrier phrase “I will say…” (“wo3 yao4 shuo1” spoken in Mandarin), followed by simultaneous presentation of four symbols and the target symbol’s audio label, that is, a slide was not previewed. Participants were told to click on the symbol that matched the perceived audio while their eye movements were continuously recorded at 50 Hz using a Tobii 1,750 system. After clicking, correct targets were highlighted with a red box so that feedback could guide learning. There were 48 total trials (16 target trials with four in each stimulus condition and 32 filler trials) with a 2 second intertrial interval.
The 16 target trials (consisting of symbols only from the 64 critical items) showed the target and three other trained test items: a tonal competitor, a rhyme competitor, and a distractor. Figure 2B shows a sample trial from the high token frequency, low-probability condition (F+P−) with fi1 (P−) as the target (top left). The three other on-screen items included a tonal competitor, which shared the same syllable but had the opposite tonal probability (e.g., P+ fi3; top right); a rhyme competitor, which shared the same vowel and tone but had a different onset (e.g., ri1; bottom right); and a distractor, which had a wholly dissimilar syllable and tone (e.g., ka2; bottom left). Position of the target and competitors was fully counterbalanced across days and trials.
To test the effect of talker variability, half of the participants were trained and tested on a single female talker (single-talker condition). Participants in this condition heard speech from Female 1 during all four tasks: the 4-AFC test consisted of a familiar syllable-tone exemplar spoken by a familiar talker. The other half of the participants were trained and tested on four different talkers (multitalker condition): the 4-AFC test consisted of a familiar syllable-tone learned in the first three tasks but spoken by a particular talker for the first time. For instance, participants heard fi1 in the first three training tasks spoken by Male 1. Participants were then tested in the 4-AFC task on Female 2’s production of fi1.
To test the effect of instruction, half the participants were given no instructions regarding lexical tone whereas the other half were explicitly trained. At the start of each daily training session, participants in the explicit training condition took part in a 5-minute, self-paced computerized lesson on the four tones using the ma tone quadruplets as practice sounds. This lesson was presented in English and emphasized the four tones’ F0 contours following modern L2 classroom pedagogy for introducing isolated monosyllables (e.g., Shen, Reference Shen1989; Xing, Reference Xing2006; Yang, 2017). Participants were first told to “pay attention to the pitch or tone of the syllable.” Participants then simultaneously heard a syllable tone while its pinyin was displayed onscreen with tone diacritics. After participants mouse clicked on the screen, the syllable-tone was presented again over headphones while the syllable’s rendered F0 contour was displayed on screen (generated through Praat; Boersma & Weenink, Reference Boersma and Weenink2016). Thus, participants were trained to associate the four F0 contours with the four tonal categories. At the conclusion of the lesson, participants were told that the “pitch or tone of the syllable is important for symbol learning.”
For the explicit group, nonce symbols were displayed alongside the symbol’s syllable-tone label in pinyin and the tone’s rendered F0 contours during the passive learning and shadowing phases of daily training. Figure 2C shows an example for the fi3 target containing the pinyin and tone contour. Participants in the no-instruction group were only shown the nonce symbol. Hereafter, we refer to the between-subject variables talker variability (single-talker/multitalker) and phonetic instruction (explicit/nonexplicit) as training conditions while the within-subject variables syllable token frequency (F+/F−) and tonal probability (P+/P−) as stimulus conditions.
Predictions
We predict higher 4-AFC mouse-click accuracy for those participants trained with explicit instruction to attend to F0 contours, which should increase awareness of the phonological role of tones (e.g., Chun et al., Reference Chun, Jiang, Meyr and Yang2015; Godfroid et al., Reference Godfroid, Lin and Ryu2017; Liu et al., Reference Liu, Wang, Perfetti, Brubaker, Wu and MacWhinney2011; Saito & Wu, Reference Saito and Wu2014). Explicit training may interact with talker variability such that explicit tone instruction is effective only for highly familiar or low variability speech. In this outcome, participants explicitly trained on single-talker input may demonstrate the highest overall mouse-click accuracy. Participants trained on multitalker input may show little to no effect of explicit instruction given the challenge associated with multitalker tones (e.g., Lee et al., Reference Lee, Tao and Bond2010; Wang et al., Reference Wang, Spence, Jongman and Sereno1999).
Participants’ eye movements within the first 1,000 ms will reveal whether syllable-tone co-occurrence probabilities were used during online spoken word recognition. In particular, we test whether participants activate the most probable (P+) tone upon hearing a syllable’s initial acoustic information (RQ1). This will be shown in anticipatory fixations to the more probable (P+) symbol as compared to the less probable (P−) symbol. If talker variability challenges participants’ perception of tone (e.g., Wiener et al., Reference Wiener, Ito and Speer2018), participants exposed to multitalker input may rely on probabilistic information to a greater degree and look to the symbol with the more probable (P+) tone (RQ2). If explicit instruction facilitates statistical learning of tone distributions, participants explicitly trained on tone may respond with more anticipatory eye movements to more probable (P+) visual candidates (RQ3).
RESULTS
4-AFC MOUSE-CLICKS
Mean 4-AFC mouse-click accuracy was calculated for each of the four groups for each day. Figure 5A shows the changes in accuracy (with 95% confidence intervals) across the 4 days of testing (x-axis). Participants performed above chance (.25) on Day 1 regardless of the training conditions (bar color) and demonstrated overall average daily improvements with each training/testing session. Figure 5B plots Day 4 results by stimulus (x-axis) and training conditions (bar color). Participants in the two single-talker training conditions had roughly equal mean accuracies across the four stimulus conditions. In contrast, participants in the two multitalker training conditions had the lowest mean accuracy for low-frequency low-probability (F−P−) targets and the highest mean accuracy for low-frequency high-probability (F−P+) targets.
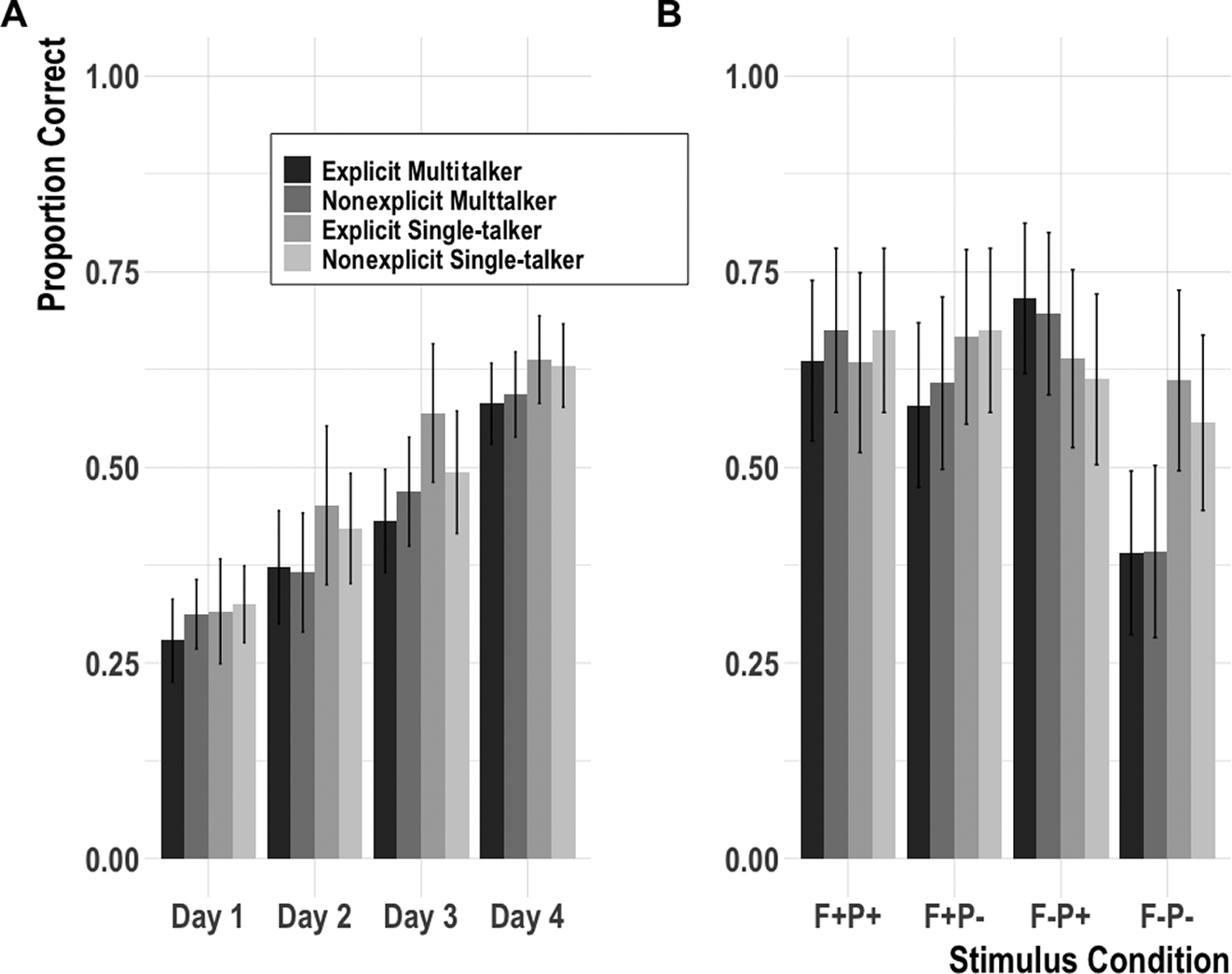
FIGURE 5. A: Mean daily 4-AFC accuracy by training conditions.
B: Mean Day 4 4-AFC accuracy by stimulus and training conditions. Error bars in both figures indicate 95% confidence intervals.
To test the effects of training conditions and stimulus conditions on the mouse-click accuracy, Day 4 (i.e., the final day of testing) mouse-click results were analyzed using mixed-effect logistic regression models with the lme4 package (Bates et al., Reference Bates, Mächler, Bolker and Walker2015) in R (version 3.6.1; R Core Team, Reference Core Team2019). The inclusion of fixed and random effects of all models in this article were evaluated by first building the maximally appropriate model with random intercepts and slopes: glmer(4-AFC accuracy ~ talker variability × syllable frequency × probability × instruction + (1 + instruction × talker variability|item) + (1 + syllable frequency × probability|subject), family = “binomial”). Next, effects that did not improve the model fit as assessed through the lmertest package (Kuznetsova et al., Reference Kuznetsova, Brockhoff and Christensen2017) were removed. See Supplementary Materials for power analyses carried out using the simr package in R (Green & MacLeod, Reference Green and MacLeod2016). All models in this article treated fixed effects as contrast coded variables (1, −1). The final 4-AFC logistic regression model did not include instructional manner as a main effect (χ2 = 0.01, p = .92) or as part of any interaction (χ2s < 2, ps > .1). Talker variability was not included as a main effect (χ2 = 2.42, p = .11), as a two-way interaction with syllable frequency (χ2 = 0.02, p = .88), or as a three-way interaction with syllable frequency and tonal probability (χ2 = 3.11, p = .07). See Table 1 for final model specification and output.
TABLE 1. Summary of mixed-effect regression on Day 4 4-AFC mouse-click accuracy
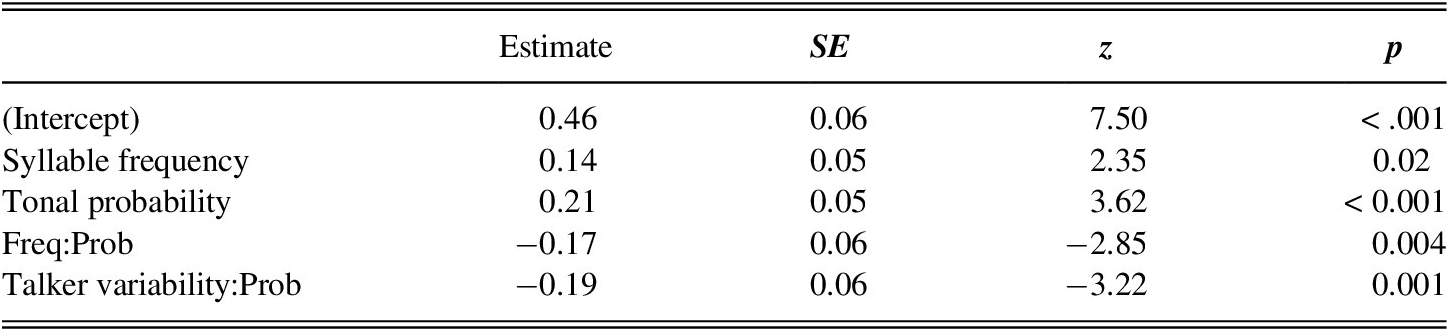
Note: High frequency, high probability, and single-talker were all coded as 1.
R code: glmer(accuracy ~ syllable frequency × probability + talker variability: probability + (1+variability|item) + (1+syllable frequency × probability|subject), family = “binomial”).
The model revealed (positive) main effects of syllable frequency and tonal probability and two separate (negative) two-way interactions between syllable frequency and probability, and between talker variability and probability. The difference in direction of these effects indicates that the main effects were not additive. For low-frequency (F−) items, there was an effect of probability and for low-probability (P−) items, there was an effect of frequency. In other words, no difference was found between probability conditions for F+ items (p = .24)3; for F− items, high-probability tones (P+) were identified more accurately than P− tones (p < .01). For P+ tones, no difference was found between frequency conditions (p = .12); for P− tones, F+ items were identified more accurately than F− items (p < .01). For single-talker speech, no difference was found between probability conditions (p = .74); for multitalker speech, P+ tones were identified more accurately than P− tones (p < .001).
To summarize, tonal probability was useful for the identification of low-frequency syllables only (F−P+, F−P−). No difference due to tonal probability was found for items containing high-frequency syllables (F+P+, F+P−). Moreover, this effect of tonal probability was primarily driven by participants exposed to multitalker input. Participants exposed to single-talker input did not identify P+ items more accurately than low-probability targets. These results suggest that participants unfamiliar with tone languages can learn syllable-tone statistical regularities (RQ1), but they rely on this information to a greater extent when the stimuli contain high talker variability (RQ2). Explicit instruction had no apparent effect on participants’ ability to extract statistical information (RQ3).
Eye Fixations
To examine the overall time course of shifts in eye fixations and to determine the sizes of analysis windows, we first plotted the loess-smoothed (Cleveland, Reference Cleveland1979) grand mean fixations to the target and competitors across all training and stimulus conditions on all 4 days (Figure 6). Looks to each visual candidate began at chance (.25) at the critical word onset and looks to the target and tonal competitor diverged from looks to the rhyme competitor and distractor at about 250 ms after that. In accordance with previous studies that report that approximately 200 ms (from critical auditory input) is required to plan and execute an eye movement (e.g., Matin et al., Reference Matin, Shao and Boff1993), the initial divergence reflects accurate detection of syllable onset. Looks to the tonal competitor peaked between 750 ms and 1,000 ms and dropped below chance (.25) by approximately 1,500 ms.
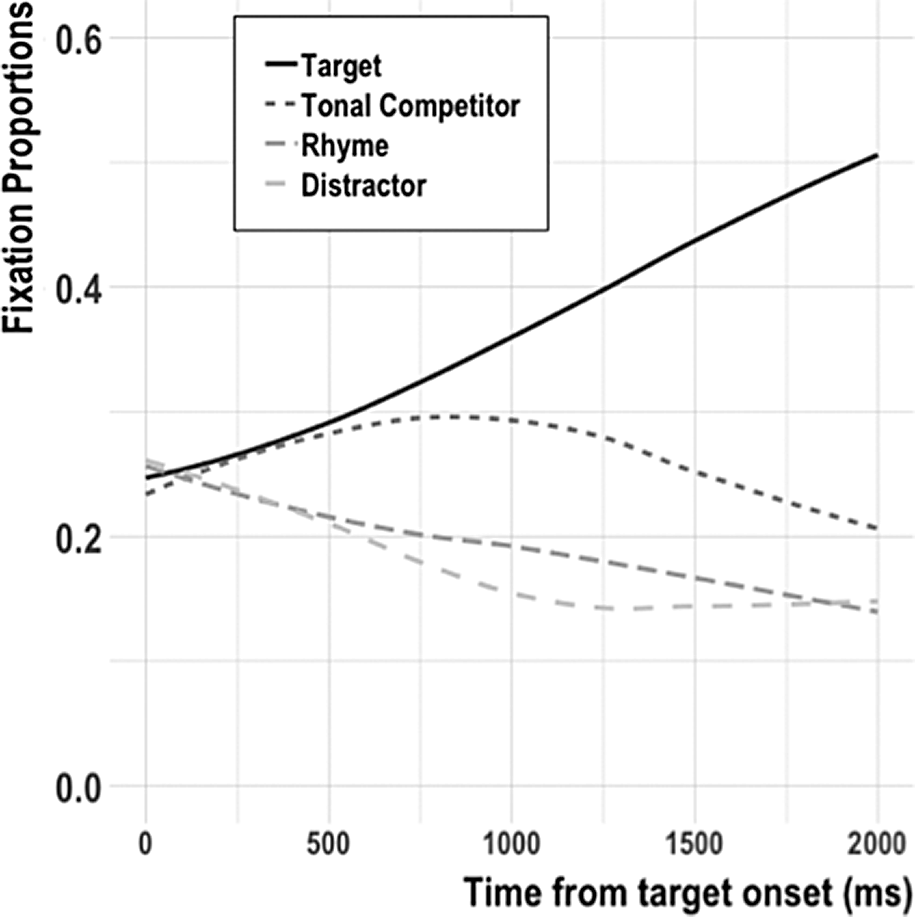
FIGURE 6. Loess-smoothed grand mean fixation proportions from the onset of the target word across 4 days of testing.
Figure 7 magnifies the 300–1,500 ms time window in which tonal competition was above chance. The figure plots the loess-smoothed fixation functions for the target and tonal competitor by training and stimulus conditions on Day 4. Both high-probability conditions (1st and 3rd columns) showed an early divergence between the target and tonal competitor, and the looks to the tonal competitor remained at or below chance after 300 ms, regardless of training condition. In these conditions where the target had the most probable tone, looks to the less probable tonal competitor did not exceed looks to the target at any point in time. In contrast, the two low-probability conditions (2nd and 4th columns) showed looks to the tonal competitor at or above chance from 300 ms until 900 ms. For the two multitalker conditions (1st and 2nd rows), looks to the tonal competitor exceeded looks to the target competitor from roughly 300 ms until 900 ms.
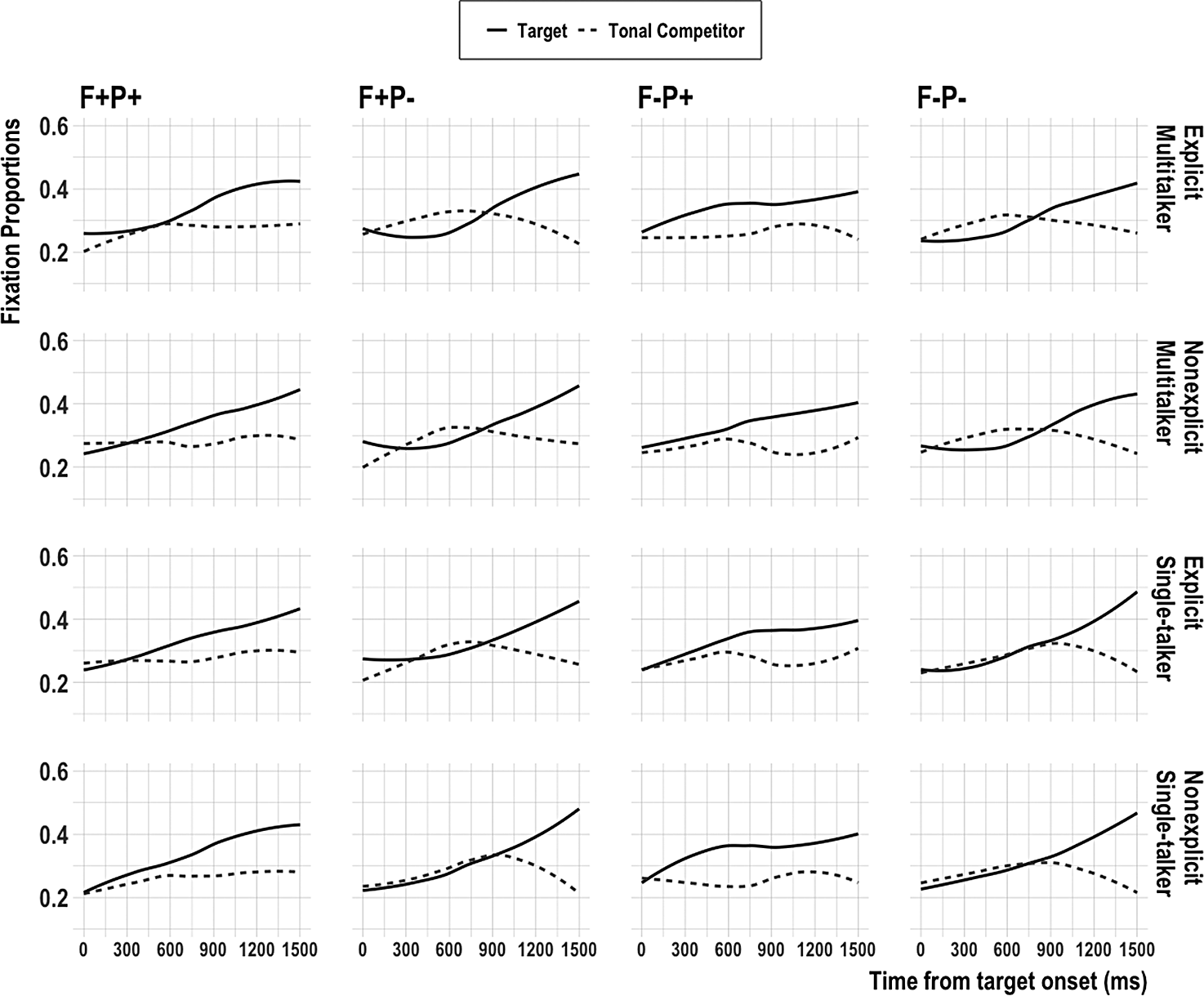
FIGURE 7. Loess-smoothed mean fixation proportions to Day 4 target and tonal competitor by stimulus and training conditions (0–1,500 ms).
To test whether looks to the target differed across training and stimulus conditions, the empirical logit (elogit) and the associated weights were calculated for 200 ms time intervals for each trial and participant. Four consecutive weighted mixed-effects models were tested from 300 ms until looks to the tonal competitor peaked at 1,100 ms. Model effect structure for each window followed the method outlined in the previous section by starting with the full model: lmer(elog target ~ talker variability × syllable frequency × probability × instruction + (1 + instruction × talker variability|item) + (1 + syllable frequency × probability|subject), weights = weight). The final model only contained tonal probability as a fixed effect. Syllable frequency, instructional method, and talker variability (and all interactions containing these variables) did not significantly improve the model’s fit at any time window (χ2s < 3, ps > .05). Given the number of models built, Bonferroni correction was used for all reported p-values. Table 2 reports the final model along with its output for each 200 ms window.
TABLE 2. Summary of weighted mixed-effect regressions on Day 4 target fixations
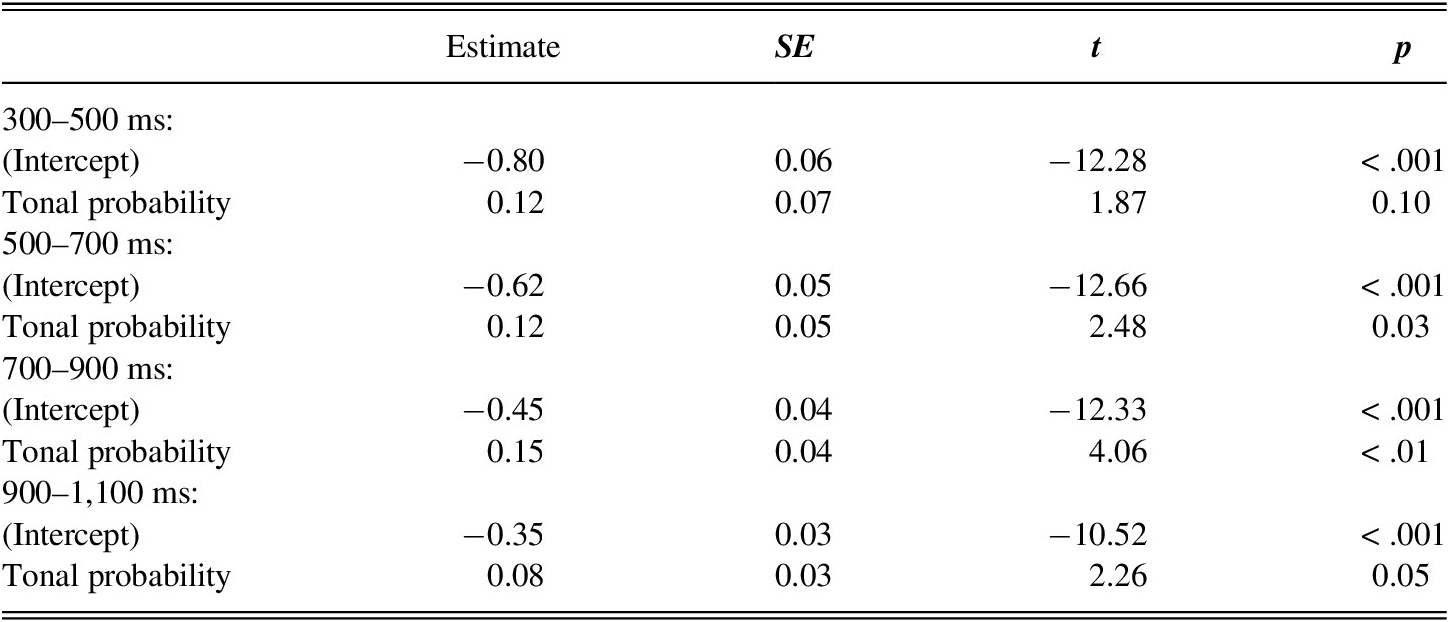
R code: lmer(elog target ~ probability + (1|item) + (1 + probability|subject), weights = weight)
From 300 to 500 ms, a marginal effect of tonal probability was found. Participants looked to targets with high-probability (P+) tones at a marginally greater proportion than to targets with low-probability (P−) tones. From 500 to 700 ms and 700 to 900 ms, a main effect of tonal probability was found. Participants looked to P+ targets at a significantly higher proportion than to P− targets. From 900 to 1,100 ms, P+ targets were looked to at a marginally greater proportion than P− targets.
To confirm whether this reduction in looks to the P− target was due to participants looking to the more probable P+ tonal competitor, the weighted elogit of the tonal competitor was analyzed following the same procedure as outlined for looks to the target (Table 3). Once again, neither syllable frequency nor instructional method nor talker variability (nor any interactions) significantly improved the model’s fit (χ2s < 3, ps > .05).
TABLE 3. Summary of weighted mixed-effect regressions on Day 4 tonal competitor fixations
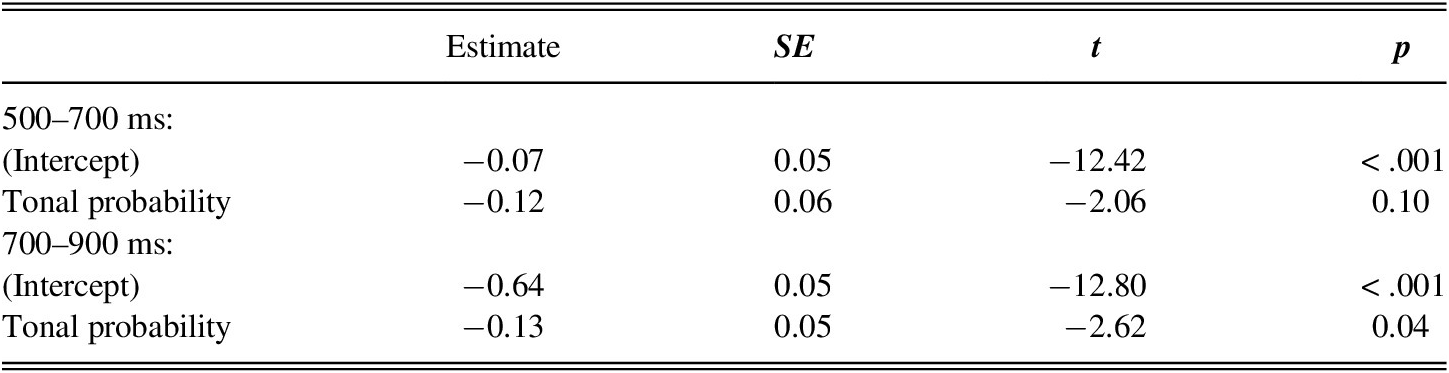
R code: lmer(elog competitor ~ probability + (1|item) + (1 + probability|subject), weights = weight)
No significant effects of predictor factors were found in the 300–500 ms model. From 500 to 700 ms, participants looked to P+ tonal competitors at a marginally higher proportion than they looked to P− tonal competitors. From 700 to 900 ms, a main effect of tonal probability was found: participants looked to P+ tonal competitors at a higher proportion than to P− tonal competitors irrespective of syllable token frequency. No significant effects were found in the 900–1,100 ms window.
To summarize the eye-fixation results, from 300 to 1,100 ms, participants looked to high-probability (P+) candidates at a higher proportion than to corresponding low-probability (P−) candidates. These looks were independent of syllable frequency, talker variability, and instructional method. From 500 to 900 ms, participants looked at P+ targets and P+ tonal competitors at significantly higher rates than P− targets and P− tonal competitors across all training conditions (RQ1). Talker variability in the input (RQ2) and explicit instruction (RQ3) did not appear to affect the use of syllable-tone statistical information during online spoken word recognition.
DISCUSSION
This study examined whether adult listeners unfamiliar with lexical tone are able to track statistical regularities in syllable-tone co-occurrences in a manner similar to L1 and intermediate L2 Mandarin listeners. We additionally explored whether multitalker input increases the reliance on this statistical information during spoken word recognition and whether explicit instruction to attend to specific tone contours facilitates this statistical learning. We trained participants for four consecutive days on our artificial tonal language and took Day 4 eye movements as our measure of online predictive processing.
Results from the Day 4 4-AFC task indicated that tonal probability information was useful for identifying items containing low-frequency (F−) syllables only. No differences were found for items containing high-frequency (F+) syllables. Moreover, this effect of tonal probability was primarily driven by participants exposed to multitalker input. Participants in the single-talker condition did not show a statistically significant advantage in their mouse-click results for high tonal probability (P+) over low-probability (P−) items. Analyses of the Day 4 eye movements revealed that participants across all training conditions looked to P+ targets and tonal competitors at a significantly greater proportion than corresponding P− targets and tonal competitors from 500 to 900 ms. These eye movements were independent of instructional method or talker variability.
Taken together, our mouse-click and eye-movement results indicate that learners unfamiliar with lexical tone were able to track the relative frequency of CV syllables and the probabilities of such syllables co-occurring with particular F0 contours. This dimension-based statistical learning occurred independently of the variability in the speech input or the instructions used to teach tone. Early-stage L2 acquisition thus appears to involve not only tracking nonnative speech cues’ distributions, such as phonetic continua and phonemic contrasts (e.g., Escudero et al., Reference Escudero, Benders and Wanrooij2011; Hayes-Harb, Reference Hayes-Harb2007; Hayes-Harb & Masuda, Reference Hayes-Harb and Masuda2008), but also multiple co-occurring cues that vary along acoustic-phonetic dimensions. Importantly, our results strengthen the claim that adult nonnative listeners can track tonal regularities at not just the phonetic level (e.g., Ong et al., Reference Ong, Burnham, Escudero and Stevens2017; Potter et al., Reference Potter, Wang and Saffran2017; Wang & Saffran, Reference Wang and Saffran2014) but also at the lexical level as in Mandarin.
Remarkably, this dimension-based statistical learning took place even without any explicit instruction of tone’s acoustic characteristics. Unlike Ong et al. (Reference Ong, Burnham and Escudero2015), we found statistical learning of syllable-tone co-occurrences independent of instructional method. Participants trained explicitly on visualized tone contours and a pinyin-like romanization did not differ in mouse-click accuracy or proportion of eye fixations as compared to those participants who were not explicitly trained. Previous instructional method studies on nonnative tone acquisition typically used small, evenly distributed sets of syllable-tones with pinyin-like text (e.g., Showalter & Hayes-Harb, Reference Showalter and Hayes-Harb2013), auditory-only stimuli devoid of visual cues (e.g., Godfroid et al., Reference Godfroid, Lin and Ryu2017), or tone-only discrimination tasks (e.g., Ong et al., Reference Ong, Burnham and Escudero2015). In our study, the artificial language contained a large number of items (including syllable-tone homophones) and nonce symbols more closely resembling Mandarin. Our design thus may have better simulated the challenges involved in L2 acquisition (see Ettlinger et al., Reference Ettlinger, Morgan-Short, Faretta-Stutenberg and Wong2016 for discussion of artificial languages’ external validity) by capturing the increased perceptual competition among new L2 sound categories and lexical candidates typically observed during spoken L2 word recognition (e.g., Escudero et al., Reference Escudero, Hayes-Harb and Mitterer2008; Weber & Cutler, Reference Weber and Cutler2004, Reference Weber and Cutler2006). We note that the present study’s Day 4 mean accuracy across training and stimulus conditions was approximately 60% with learners primarily making tonal mistakes. This is much lower than the posttraining ceiling performances typically observed with relatively small, symmetric stimuli syllable-tone sets (e.g., Wong & Perrachione, Reference Wong and Perrachione2007).
With respect to talker variability, our results did not support Wang et al.’s (Reference Wang, Spence, Jongman and Sereno1999) finding that multitalker input leads to improved tonal categorization among classroom Mandarin L2 learners already familiar with tone. Unexpectedly, we observed no difference in overall 4-AFC accuracy between the single-talker and multitalker groups. A difference, however, was observed for the low-frequency syllables; for participants trained and tested on multiple speakers, low-frequency syllables with low-probability tones (F−P−) had the lowest average accuracy while low-frequency syllables with high-probability tones (F−P+) had the highest average accuracy (Figure 5B). For participants trained and tested on the same speaker, no such difference was found. There are at least three factors that may have contributed to this two-way interaction between syllable frequency and talker variability.
First, for true beginner learners like the participants tested in the present study, talker variability may have hindered learning the low-frequency, low-probability tones. There may have been too much variation in the F0 contours across the four talkers and too few exemplars to learn from. Thus, the difference observed in 4-AFC results between the F− items may have reflected the challenge of learning highly varied tones with a limited number of exemplars. Under this account, as L2 learners accrue greater awareness of tonal categories and weigh F0 movement cues more heavily over time, F0 variability may prove to be more beneficial in tonal word learning (e.g., Barcroft & Sommers, Reference Barcroft and Sommers2014). For instance, intermediate and advanced learners may gradually encode more robust tone categories given more varied input, which is in line with Wang et al.’s (Reference Wang, Spence, Jongman and Sereno1999) results.
Second, greater variability in the input may have led to a greater tendency to rely on experience-based distributional processing as a means of accommodating the acoustic variability (e.g., Nixon & Best, Reference Nixon and Best2018; Nixon et al., Reference Nixon, van Rij, Mok, Baayen and Chen2016; Wiener et al., Reference Wiener, Ito and Speer2018). This probability-based account is in line with the eye-fixation results. Figure 8 plots the loess-smoothed mean fixations to the low-probability (P−) and high-probability (P+) tonal competitors across all trials, irrespective of syllable frequency. This figure demonstrates that upon hearing the P+ target, participants looked to the P− tonal competitor at nearly identical rates across variability training conditions (Figure 8, left). In contrast, upon hearing the P− target (Figure 8, right), participants exposed to multiple talkers looked proportionally more often to the P+ tonal competitor from about 300 to 700 ms. This increase in looks to the more probable tonal competitor demonstrates an emerging tendency for learners to rely greater on probability-based tone processing in the face of multitalker input.
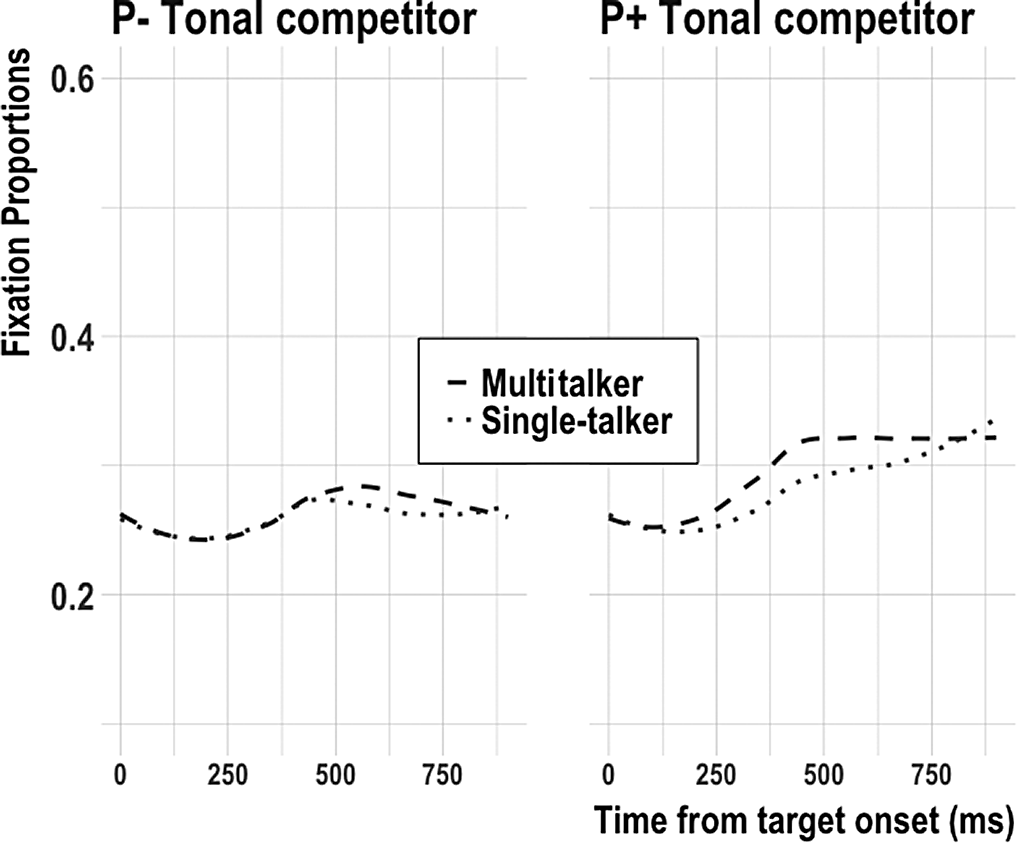
FIGURE 8. Day 4 loess-smoothed mean fixations to the tonal competitor across all high-probability P+ and low-probability P− trials by talker variability.
Further support for this claim was found in a post-hoc analysis4 involving participants’ overall 4-AFC rate of improvement from the first day of testing to the last day of testing (see Supplementary Materials for additional details including statistical analysis). This also acknowledges a third potential contributor to our results: individual differences in learning aptitude and/or perceptual abilities (among other factors; see Bowles et al., Reference Bowles, Chang and Karuzis2016; Chandrasekaran et al., Reference Chandrasekaran, Sampath and Wong2010; Theodore et al., Reference Theodore, Monto and Graham2019). Whereas none of the tested participants demonstrated a pitch deficiency, it remains possible that only participants with strong perceptual abilities benefited from the greater variability in the input while those participants with weak abilities saw no gains (e.g., Perrachione et al., Reference Perrachione, Lee, Ha and Wong2011; Sadakata & McQueen, Reference Sadakata and McQueen2014). Our post-hoc analysis revealed that participants with lower four-day improvement rates relied more heavily on tonal probability information, and that this effect was particularly robust for those tested on multitalker input. In particular, listeners with lower improvement rates were less certain of the intended tonal category of the incoming speech signal and therefore relied more heavily on their knowledge of syllable-tone co-occurrence probabilities. The difference between the early eye-movement data and the resulting mouse clicks may have reflected learners’ difficulties in recovering from their initial probability-based predictions, which led to the errors in the 4-AFC task (Figure 5B). In contrast, those learners with higher improvement rates may have been better at recovering from incorrect initial predictions and ultimately identifying the correct target. These preliminary results extend individual differences research (e.g., Perrachione et al., Reference Perrachione, Lee, Ha and Wong2011; Sadakata & McQueen, Reference Sadakata and McQueen2014) by demonstrating how learners with lower tonal improvement rates may use statistical knowledge to bootstrap their learning of tone. We note, however, that recent evidence suggests different levels of learner aptitude may not necessarily lead to better or worse learning from high (or low) talker variability training input (Dong et al., Reference Dong, Clayards, Brown and Wonnacott2019). Additional research in this domain is needed to clarify the role of individual differences in tone learning.
Finally, we acknowledge that the 4-AFC and eye-fixation results may also be accounted for by an error-driven learning mechanism (e.g., Nixon Reference Nixon2018, Reference Nixon2020) rather than a purely statistical learning mechanism. Similar to the present study, Nixon (Reference Nixon2020) trained Native English speakers on an artificial tonal language and demonstrated that the cue-outcome order of stimuli affects tone learning. Nixon presented participants with a control cue (e.g., VOT) or a critical cue (e.g., tone) and trained participants to associate the cue with an outcome (e.g., image). After this pretraining phase, the critical cue was presented with a second cue (e.g., nasal) in the main training phase. Testing involved only the second cue. Nixon found that when the critical cue was learned in the pretraining phase, it “blocked” learning of the second cue (e.g., Kamin, Reference Kamin, Campbell and Church1969). Nixon next manipulated the temporal order of cues and outcomes by presenting either a discriminative order (cues followed by images) or nondiscriminative order (images followed by cues). Nixon found that discriminative structures, which allow for feedback from prediction error, resulted in better learning of predictive cues, particularly for items with fewer exemplars (i.e., low token frequency). Additionally, discriminative structures allowed better down-weighting or unlearning of nondiscriminative cues.
In the present study, we varied the cue-outcome order in different ways. The first two daily tasks (Figure 4) involved the simultaneous presentation of the syllable-tone (cue) and symbol (outcome). The naming task presented the nonce symbol (i.e., the outcome) first and required participants to produce the syllable-tone cue—a task analogous to presenting Chinese learners with a written character and asking them to produce its syllable-tone combination (see Chung, Reference Chung2003). This may have allowed for feedback from prediction error in line with Nixon’s (Reference Nixon2020) design; however, all participants were given feedback irrespective of naming accuracy. It is unclear whether such feedback guided learning of incorrect responses (e.g., Arnon & Ramscar, Reference Arnon and Ramscar2012; Ramscar et al., Reference Ramscar, Yarlett, Dye, Denny and Thorpe2010; Reference Ramscar, Dye and McCauley2013). Moreover, we note that feedback from our naming production task may work in a fundamentally different way than that from a perceptual identification task. For beginner L2 learners especially, production abilities may lag behind perceptual abilities, which could influence learning (e.g., Baese-Berk & Samuel, Reference Baese-Berk and Samuel2016). Our fourth and final daily task involved simultaneous presentation of the cue and outcome. For this 4-AFC identification task, feedback was again provided after each mouse click irrespective of accuracy. To what degree this feedback influenced the mouse-click and eye-fixation data remains unclear. Our future work will aim to test how the cue-order outcome and discriminative structures in line with Nixon’s (Reference Nixon2020) proposal contribute to the present study’s observed learning of tone.
CONCLUSION
In this study we established that adult participants unfamiliar with lexical tone are able to track syllable conditioned tonal probabilities. This statistical learning occurs irrespective of the amount of talker variability in the speech signal or participants’ explicit instructed awareness of tone. Future research is needed to fully tease apart whether the underlying mechanism driving this learning is statistical in nature (e.g., Maye et al., Reference Myers2002; Saffran, Reference Saffran2003) or error driven (e.g., Nixon, Reference Nixon2020) and to what degree individual differences affect this learning. Finally, there is a need for future research to examine the pedagogical implications of this work and investigate how classroom Mandarin learners track the occurrence and co-occurrence regularities of syllables and tones and to what degree the timing of acoustic cues and semantic outcomes affect this learning.
SUPPLEMENTARY MATERIALS
To view supplementary material for this article, please visit http://dx.doi.org/10.1017/S0272263120000418.