Transmission of genetic information is carried out by two sets of genomes, nuclear and mitochondrial. The majority of genetic information that is contained in the nucleus follows a Mendelian pattern of inheritance. In contrast, the relatively small (16.5 kbp), circular, double-stranded DNA (mtDNA) that functions as the mitochondrial genome is primarily maternally transmitted and randomly distributed into daughter cells during cell division. mtDNA encodes for 13 peptide subunits, 22 tRNAs, and 2 rRNAs that are essential for mitochondrial protein synthesis and partake in several essential functions. These include energy production, cell signaling, calcium homeostasis, reactive oxygen species production, and apoptosis (Kujoth et al., Reference Kujoth, Leeuwenburgh and Prolla2006). Ultimately, they may affect energy reserves, mortality, development and aging, and contribute to disease risk.
The mitochondrial genome is packaged as a double-membraned organelle in the cytoplasm, an individual mitochondrion may carry multiple copies of mtDNA depending on the cell type, which can range on average from 10s to 1000s (Filograna et al., Reference Filograna, Mennuni, Alsina and Larsson2021), and a cell normally contains multiple mitochondria. What determines this organization is not fully understood. What is known, however, is that variable copies of the mitochondrial genome (mtDNA-CN) in a cell, together with any mtDNA mutation(s) (heteroplasmy), affect a cell’s overall function (Wallace & Chalkia, Reference Wallace and Chalkia2013). To this end, mtDNA-CN establishes the quantity of mitochondrial DNA, while heteroplasmy (HP), which captures the quality, refers to the ratio of mutated to normal mtDNA, and represents a crucial threshold, which may lead to pathological outcomes (Kopinski et al., Reference Kopinski, Janssen, Schaefer, Trefely, Perry, Potluri, Tintos-Hernandez, Singh, Karch, Campbell, Doan, Jiang, Nissim, Nakamaru-Ogiso, Wellen, Snyder, Garcia and Wallace2019; Stefano & Kream, Reference Stefano and Kream2016). Specifically, mtDNA-CN has been shown to have a negative correlation with age and is higher on average in women than men (Ashar et al., Reference Ashar, Moes, Moore, Grove, Chaves, Coresh, Newman, Matteini, Bandeen-Roche, Boerwinkle, Walston and Arking2015; Mengel-From et al., Reference Mengel-From, Thinggaard, Dalgård, Kyvik, Christensen and Christiansen2014). On the other hand, heteroplasmy increases with age, which reflects mutational burden (López-Otín et al., Reference López-Otín, Blasco, Partridge, Serrano and Kroemer2013; Mengel-From et al., Reference Mengel-From, Thinggaard, Dalgård, Kyvik, Christensen and Christiansen2014; Zhang et al., Reference Zhang, Wang, Ye, Picard and Gu2017). Interestingly, mtDNA-CN and heteroplasmy have been shown to be associated with a large number of complex multifactorial diseases (Ashar et al., Reference Ashar, Moes, Moore, Grove, Chaves, Coresh, Newman, Matteini, Bandeen-Roche, Boerwinkle, Walston and Arking2015; Ashar et al., Reference Ashar, Zhang, Longchamps, Lane, Moes, Grove, Mychaleckyj, Taylor, Coresh, Rotter, Boerwinkle, Pankratz, Guallar and Arking2017; Hong et al., Reference Hong, Longchamps, Zhao, Castellani, Loehr, Chang, Matsushita, Grove, Boerwinkle, Arking and Guallar2020). There is emerging interest in assessing the involvement of mitochondria in an ever-increasing number of complex diseases, including schizophrenia.
Schizophrenia (SZ) is a severe mental disorder with psychotic symptoms that include delusions and social withdrawal. Psychosis does not usually start until late adolescence and is associated with a reduction of 15−30 years in life expectancy (Olfson et al., Reference Olfson, Gerhard, Huang, Crystal and Stroup2015; Thornicroft, Reference Thornicroft2011). SZ, a complex disease, has high heritability (∼80%), and reduced concordance (∼50%) in monozygotic twins (Gejman et al., Reference Gejman, Sanders and Duan2010), a pattern that is compatible with a complex polygenic etiology influenced by environmental factors (Cardno & Gottesman, Reference Cardno and Gottesman2000; Sullivan et al., Reference Sullivan, Kendler and Neale2003). Attempts to identify genes and genetic and environmental determinants of the disease have been challenging. The results show that genetic, epigenetic, and environmental insults contribute to this devastating disorder. A recent large-scale genomewide association study (GWAS) has found common variants associated with SZ at 270 unique loci in 69,369 SZ patients and 236,642 controls. The study also highlights 19 genes based on protein-coding or UTR variation, and 130 genes in total, using fine-mapping and functional genomic data (Schizophrenia Working Group of the Psychiatric Genomics Consortium et al., Reference Ripke, Walters and O’Donovan2020; Trubetskoy et al., Reference Trubetskoy, Pardiñas, Qi, Panagiotaropoulou, Awasthi, Bigdeli, Bryois, Chen, Dennison, Hall, Lam, Watanabe, Frei, Ge, Harwood, Koopmans, Magnusson, Richards, Sidorenko and van Os2022). Yet, additional novel experimental approaches and technologies are needed to resolve the causes of schizophrenia. Here we will consider the potential involvement of the mitochondrial genome in SZ in a novel experimental design that uses monozygotic twins discordant (MZD) for the disease (Castellani et al., Reference Castellani, Melka, Gui, Gallo, O’Reilly and Singh2017; Tang et al., Reference Tang, Fan, Li, Xiang, Zhang, Li, He, Liao, Wang, He, Zhang, Shugart, Liu, Tang, Chan, Wang, Yao and Chen2017).
Monozygotic twins (MZ), also referred to as identical twins, have historically been used for studies assessing genetic and environmental contributions to diseases. Due to the unique characteristic of a single zygote splitting into two embryos with a shared genome, differences in a disease phenotype in MZ pairs can be reasoned to be a source of difference from environmental or de novo events independently operating on each individual. As a result, MZ twins have been used to ascertain the genetic and environmental contributions to complex diseases, including SZ (Castellani et al., Reference Castellani, Laufer, Melka, Diehl, O’Reilly and Singh2015; Li et al., Reference Li, Bi, Fan, Wu, Tang, Li, He, Zhou, Tang, Chen and Yao2017; Melka et al., Reference Melka, Castellani, O’Reilly and Singh2015; Singh et al., Reference Singh, Castellani and Hill2020; Tang et al., Reference Tang, Fan, Li, Xiang, Zhang, Li, He, Liao, Wang, He, Zhang, Shugart, Liu, Tang, Chan, Wang, Yao and Chen2017; Wrede et al., Reference Wrede, Mengel-From, Buchwald, Vitiello, Bamshad, Noonan, Christiansen, Christensen and Watson2015). Over the years, these studies have focused on the nuclear genome. More recently, there has been some attempt to assess the involvement of mtDNA between MZD twins for a number of disorders. These have included sleep, multiple sclerosis, SZ, obesity, and childhood intelligence, to name a few (Avital et al., Reference Avital, Buchshtav, Zhidkov, Tuval Feder, Dadon, Rubin, Glass, Spector and Mishmar2012; Bijnens et al., Reference Bijnens, Derom, Weyers, Janssen, Thiery and Nawrot2019; Heinonen et al., Reference Heinonen, Buzkova, Muniandy, Kaksonen, Ollikainen, Ismail, Hakkarainen, Lundbom, Lundbom, Vuolteenaho, Moilanen, Kaprio, Rissanen, Suomalainen and Pietiläinen2015; Li et al., Reference Li, Bi, Fan, Wu, Tang, Li, He, Zhou, Tang, Chen and Yao2017; Maeda et al., Reference Maeda, Kawai, Sanada, Terashima, Ogawa, Idehara, Makiishi, Yasuda, Sato, Hoshi, Yahikozawa, Nishi, Itoh, Ogasawara, Tomita, Indo and Majima2016; Souren et al., Reference Souren, Gerdes, Kümpfel, Lutsik, Klopstock, Hohlfeld and Walter2016; Wang et al., Reference Wang, Zhu, Zhang, Bian, Lu and Li2015; Wrede et al., Reference Wrede, Mengel-From, Buchwald, Vitiello, Bamshad, Noonan, Christiansen, Christensen and Watson2015). For example, decreased mtDNA-CN was observed with sleep efficiency, childhood intelligence, and obesity, in blood mtDNA-CN, placental mtDNA content, and adipose tissue mtDNA respectively (Bijnens et al., Reference Bijnens, Derom, Weyers, Janssen, Thiery and Nawrot2019; Heinonen et al., Reference Heinonen, Buzkova, Muniandy, Kaksonen, Ollikainen, Ismail, Hakkarainen, Lundbom, Lundbom, Vuolteenaho, Moilanen, Kaprio, Rissanen, Suomalainen and Pietiläinen2015; Wrede et al., Reference Wrede, Mengel-From, Buchwald, Vitiello, Bamshad, Noonan, Christiansen, Christensen and Watson2015). However, association studies on multiple sclerosis and SZ involving mtDNA heteroplasmy have shown little to no discordance for mtDNA heteroplasmy calls between discordant MZ twins. Current findings remain to be validated by larger sample sizes and greater sequencing depth, and a role for mitochondria in neurodevelopment and manifestation of SZ is an avenue for exploration. In fact, a link to developing brain function in a rat model of SZ has shown the importance of mitochondrial dynamics to this disease through mitochondrial transplantation (Ene et al., Reference Ene, Karry, Farfara and Ben-Shachar2023). In addition, mitochondrial dysfunction in synaptic and metabolic pathways have been implicated but not well established in the pathophysiology of SZ (Hjelm et al., Reference Hjelm, Rollins, Mamdani, Lauterborn, Kirov, Lynch, Gall, Sequeira and Vawter2015; Park & Park, Reference Park and Park2012; Stork & Renshaw, Reference Stork and Renshaw2005). The objective of this report is to evaluate the distribution of mitochondrial DNA copy number (mtDNA-CN) and heteroplasmy in schizophrenia by assessing MZ twins discordant for SZ, and their parents where possible, using microarrays (Affymetrix Human SNP Array 6.0), complete genome sequences (WGS), and quantitative polymerase chain reaction (qPCR). The findings argue that even with a powerful experimental model involving MZD twins, relatively large numbers of well-characterized patients will be needed to establish any direct role for SZ-related mitochondrial aberrations in humans.
Materials and Methods
Monozygotic Twin Subjects and Parents
All human subjects were diagnosed and recruited by Dr Richard O’Reilly under a grant from the Canadian Institutes of Health Research. They provided written consent to participate in the study, which has been described previously (Castellani et al., Reference Castellani, Laufer, Melka, Diehl, O’Reilly and Singh2015; O’Reilly et al., Reference O’Reilly, Torrey, Rao and Singh2013). In brief, genomic DNA from whole blood was extracted from six pairs of MZ twins discordant for SZ and two sets of parents using the 5 Prime Perfect Pure DNA Blood kit, following manufacturer’s protocol (N = 16). A summary table of MZ twins and their respective parents with age, sex, and technology applied are listed in Table 1. Participants ranged from 20 to 82 years of age with an equal ratio of males and females.
Table 1. Summary of participant twin pairs discordant for schizophrenia and their respective parents
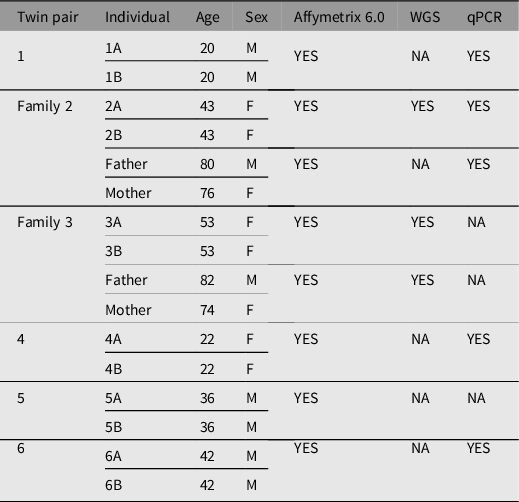
Note: A, affected twin; B, unaffected twin; NA, not available; WGS, whole genome sequencing; qPCR, quantitative polymerase chain reaction.
Microarray mtDNA-CN Estimation
DNA from whole blood of all MZ twins discordant for SZ and their respective parents (N = 16) was hybridized to the Affymetrix Human SNP Array 6.0. The array results were used to assess mtDNA-CN, defined as the ratio of total autosomal reads to total mtDNA reads. The java-based program Genvisis was used to generate mtDNA-CN estimates adjusted for principal components, age, and sex (Yang et al., Reference Yang, Newcomb, Battle, Hsieh, Chapman, Côté and Arking2021). Genvisis involves using log R ratios (LRR) to obtain population-normalized intensity values for each SNP on the Affymetrix microarray. Mitochondrial and autosomal markers are then selected for inclusion in the analysis. The method involves several quality filters and corrections, including correcting LRRs for GC content and removing mitochondrial markers that do not have a perfect match to the annotated location or have off-target matches. The resulting estimates capture batch effects, quality metrics, and latent confounding variables. The difference in mtDNA-CN estimates between MZD twins and their respective mothers (mother-child), and unrelated individual comparisons were generated. In the case of unrelated individuals, the difference in mtDNA-CN estimates for the unrelated group was compared between each individual to individuals outside of their respective families; therefore, comparisons did not have any familial relations—for example, twin 1A to twin 2B.
Whole Genome Sequencing, Alignment, mtDNA-CN Estimation, and Heteroplasmy Calling
Whole genome sequencing for a subset of six subjects comprising two MZ twin pairs discordant for SZ and one set of respective parents was generated from Complete Genomics Inc. (Mountain View, CA). CGA tools version 1.8.0 build 3 was used to align each individual genome sequence with hg19 as reference. The revised Cambridge Reference Sequence (NC_012920.1) was used as reference for mitochondrial genome alignment (Andrews et al., Reference Andrews, Kubacka, Chinnery, Lightowlers, Turnbull and Howell1999). The haplogroup of each individual was determined with Haplogrep 3 version 3.2.1 (Schönherr et al., Reference Schönherr, Weissensteiner, Kronenberg and Forer2023). Two distinct programs, Mutect2 and Mutserve, were used for heteroplasmy calling (Diroma et al., Reference Diroma, Modi, Lari, Sineo, Caramelli and Vai2021; McKenna et al., Reference McKenna, Hanna, Banks, Sivachenko, Cibulskis, Kernytsky, Garimella, Altshuler, Gabriel, Daly and DePristo2010). Mutect2 and Mutserve were run and mitochondrial heteroplasmic variants were called at 1%, 3% and 5% threshold level and an output variant call format (VCF) file for each heteroplasmy level were generated as text files (Weissensteiner et al., Reference Weissensteiner, Forer, Fuchsberger, Schöpf, Kloss-Brandstätter, Specht, Kronenberg and Schönherr2016). Mutect2 filtering criteria included setting: -initial-tumor-lod to 0, --tumor-lod-to-emit to 0, --af-of-alleles-not-in-resource to 4e-3, and --pruning-lod-threshold to -4, where LOD represents the logarithm of the odds (mitochondria mode). The alignment, base, and mapping quality scores cutoff was set to a minimum of 10 for both programs. fastMitoCalc was used to estimate mtDNA-CN from whole genome-sequencing data and is based on the proportion of sequencing coverage to underlying DNA copy number for autosomal and mitochondrial DNA (Qian et al., Reference Qian, Butler, Opsahl-Ong, Giroux, Sidore, Nagaraja, Cucca, Ferrucci, Abecasis, Schlessinger and Ding2017). mtDNA-CN was defined as the average coverage of mtDNA divided by the average coverage of autosomal DNA, multiplied by 2.
Quantitative Polymerase Chain Reaction mtDNA-CN Estimation
qPCR-based estimates of mtDNA-CN for a subset of twins comprising four discordant twin pairs and one set of respective parents were generated. A modified multiplexed real-time qPCR assay from a previously described method was used (Hsieh et al., Reference Hsieh, Budd, Deng, Gadawska and Côté2018; Yang et al., Reference Yang, Newcomb, Battle, Hsieh, Chapman, Côté and Arking2021). More specifically, the mtDNA target was the mtDNA control region (D-loop), a noncoding region in the mtDNA genome. The nuclear DNA (nDNA) target was the albumin gene, which is a single-copy gene present in all human cells. This method uses 20 ng of DNA per sample as input for the qPCR reaction. The Viia7 qPCR machine (ThermoFisher Scientific) alongside the provided Viia7 Software version 1.2.2 was used to determine Ct values. All samples were run in triplicates and deltaCt was calculated using the R software.
Statistical Analysis
Unpaired t tests were used to determine differences in mtDNA-CN means between groups. The Shapiro-Wilk test was used to test for normality of all variables before performing t tests to ensure that the assumptions of normality were met. All statistical analyses were performed using R (version 4.1.2). Additionally, using the known correlate of mitochondrial relatedness to mtDNA-CN, the effectiveness of different mtDNA-CN estimation techniques was compared with a linear regression of mtDNA-CN estimates for each technology to relatedness. The WGS mtDNA-CN estimates were not used in this regression analysis due to the small sample size which could undermine the validity of the comparison.
Results
Monozygotic Twins Discordant for Schizophrenia Differ for mtDNA-CN
The demographics of MZD twins and parental samples are summarized in Table 1. In this cohort, we identified differences in mtDNA-CN using three different technologies: Affymetrix, WGS and qPCR. The results generated by the Affymetrix microarray, for which we have results for all twin pairs, highlights the difference across individuals of differing relationships of MZ twins, mother-child and unrelated individuals (Figure 1). However, it is important to note that these conclusions are drawn from a study with a limited sample size of 16 individuals, comprising 6 twin pairs and 2 sets of parents. It shows that the familial comparisons display smaller differences while the unrelated individuals show extensive variability, as expected. Here, the MZ within-pair differences were smaller than unrelated individual comparisons (N = 16, p = .03, effect size = .60). Additionally, the mother-child to unrelated group comparison also showed a significant difference (n = 4, p = .01, effect size = .95). Further, a trend was seen in mother-child to MZD twin comparison where a closer similarity was seen in mtDNA-CN estimates between a mother and child as compared to within MZD twins (n = 4, p = .23, effect size = .81), though this difference was not statistically significant. More interesting are the comparisons that specifically relate to within MZD pair comparisons. Interestingly, the comparison varies across the six twin pairs studied (Figure 2). In all twin pairs, Affymetrix mtDNA-CN estimation resulted in a difference in mtDNA-CN between each pair. In the case of qPCR (n = 10) and WGS (n = 6), where only a subset of the MZD twins was run, the results also showed a similar trend, whereby identical twins displayed discordant mtDNA-CN measures.
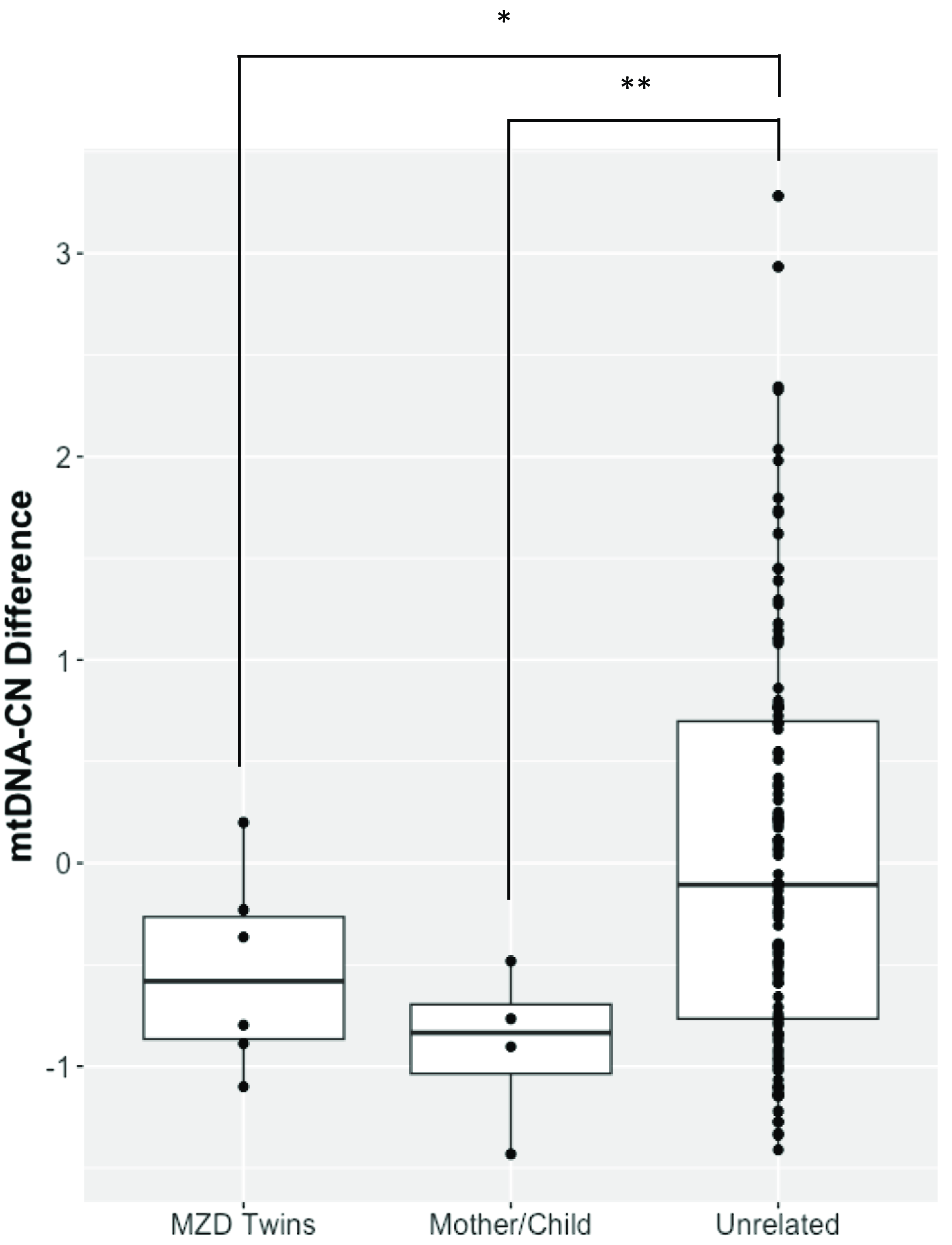
Figure 1. Affymetrix array mtDNA-CN estimates difference between individuals. Mean comparison of the groups showed significant difference for MZD Twins (*p < .05) and Mother/Child (**p < .01) when compared to unrelated samples.
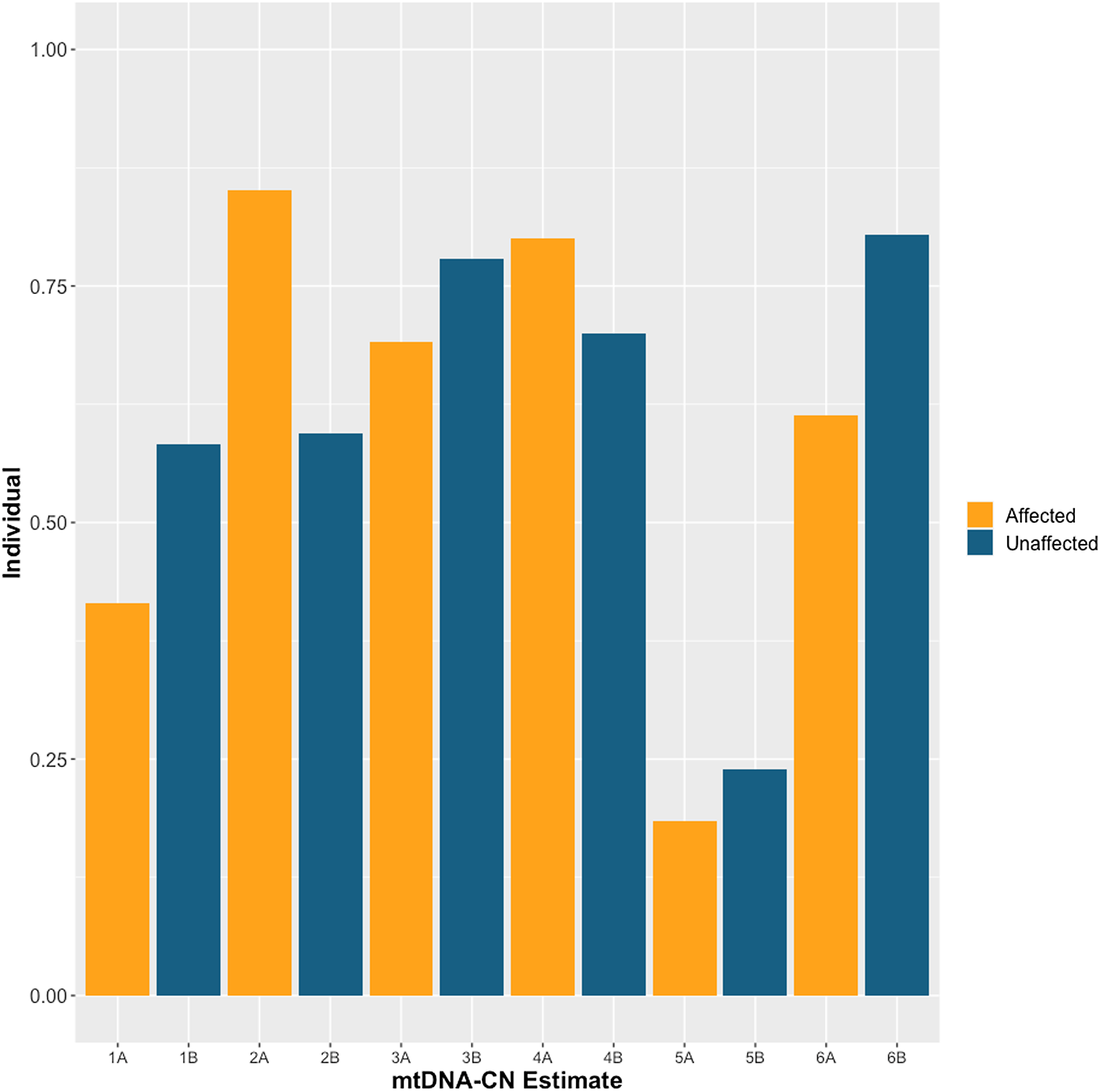
Figure 2. Affymetrix array mtDNA-CN estimates for each individual twin across 6 monozygotic discordant (MZD) twin pairs. SZ affected co-twin (A), Unaffected (B).
Direction of mtDNA-CN Differences is Consistent Across Array, WGS and qPCR Technologies
MZD twins exhibit shared directionality in mtDNA-CN estimation across three different mtDNA-CN estimation techniques (Figure 3). In some cases, the magnitude of the mtDNA-CN estimate showed numerical differences across different technologies; however, the direction of mtDNA-CN estimation between MZD twins was consistent. As an example, twin pair 2, for which mtDNA-CN estimates from all three technologies were generated, a lower mtDNA-CN estimate in the affected twin was observed compared to the unaffected co-twin. Also, for twin pairs 3, 5 and 6, where more than one technology was available, there is a similar trend, and unlike twin pair 2, the affected twin displays higher mtDNA-CN than the unaffected co-twin. Such results may argue for the potential involvement of mtDNA-CN in twin discordance for SZ.

Figure 3. Alluvial plot indicating the directionality of the mtDNA-CN differences between affected and unaffected twins as estimated by Affymetrix, qPCR and WGS. Directionality of mtDNA-CN differences was concordant across all available technologies. Line thickness reflects the magnitude of the mtDNA-CN difference between co-twins.
qPCR Outperforms Affymetrix in Estimating mtDNA-CN
Using the expected correlate for mtDNA-CN of relatedness, we found expected trends of mtDNA-CN with mitochondrial relatedness (Twin pairs = 1, Unrelated =0; Figure 4). The effectiveness of qPCR and Affymetrix technologies in estimating mtDNA-CN was determined by assessing the strength of these known relationships. Specifically, we found that as relatedness increases, mtDNA-CN concordance decreases. As outlined in the methods, WGS was not utilized in this part of the analysis due to the small sample size for estimating expected trends. qPCR performed best at mtDNA-CN estimation based on this relatedness metric (qPCR R2 = 0.74, Affymetrix R2 = 0.07).
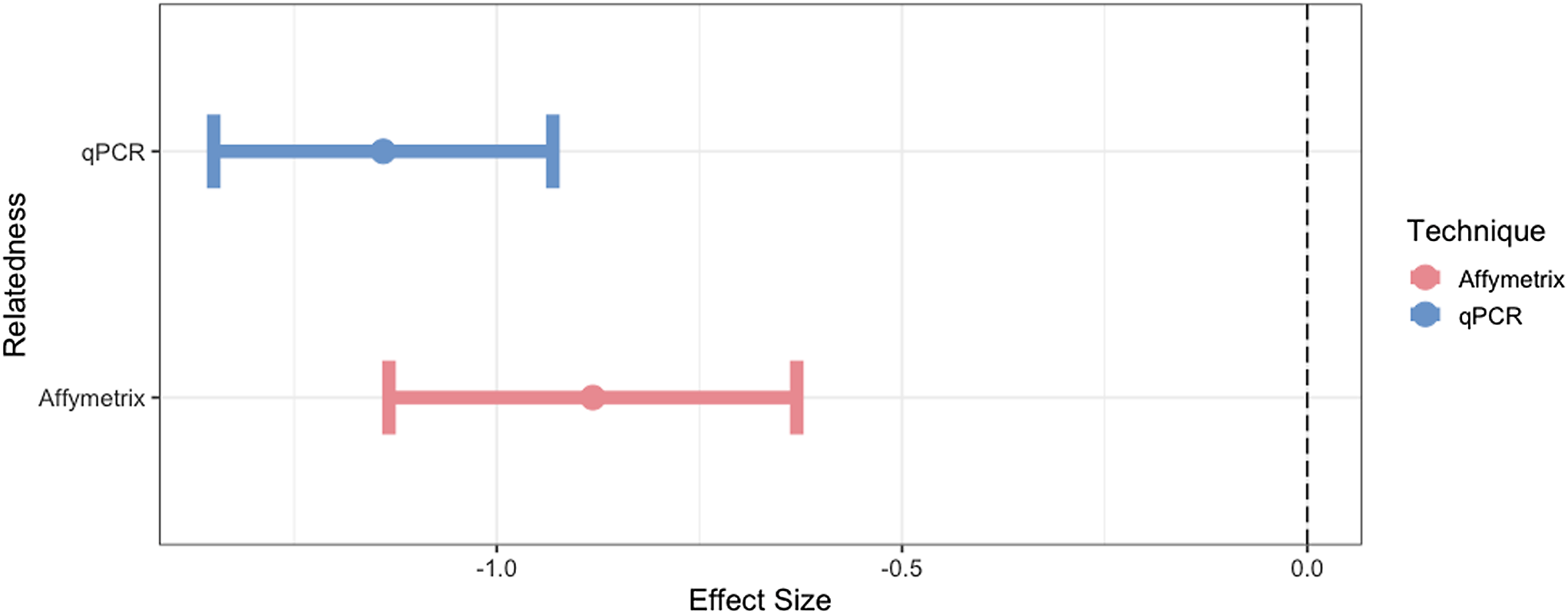
Figure 4. The effect size and 95% confidence interval for the association of mtDNA-CN with its known correlate, relatedness. Only unrelated individuals and identical twins are included in this analysis.
Absence of mtDNA Heteroplasmy Differences Between MZD Twins
Heteroplasmy calling was performed using WGS data for two twin pairs (Twin 2 and 3), using two independent programs, Mutserve and Mutect2. The cut-off criteria for calling a mutation was a call that was discordant from reference and had a base quality above 10. Mutect2 did not identify any heteroplasmic calls at the specified thresholds of 1%, 3% and 5%. In Mutserve analysis, eight heteroplasmic sites were identified in twin pair 2 and no sites were identified in twin pair 3. In twin pair 2, four heteroplasmy calls were identified by Mutserve to be unshared between twins, three of the heteroplasmies were unique to the affected twin (75%) and only one (25%) was unique to the unaffected twin (Table 2). Heteroplasmic calls at 1%, 3% and 5% thresholds were generated, and the results are available in Table 3. However, with further in-depth review of the raw sequencing reads at these locations through IGV visualization (Robinson et al., Reference Robinson, Thorvaldsdóttir, Winckler, Guttman, Lander, Getz and Mesirov2011), all unique heteroplasmy calls between co-twins called by either program could be attributed to sites lost during quality control steps in one twin but not in the co-twin and calls in the co-twin that were just below the heteroplasmic threshold (Figure 5). We conclude that these unique calls reflect differences in filtering/calling criteria rather than true unique heteroplasmies between identical twins. The haplogroup for twin pair 2 was determined to be U4b1a3a and the haplogroup for twin pair 3 was determined to be L3. Both individuals from twin pair 2 have at least one heteroplasmic call that is not found in their major haplogroup. Given that these heteroplasmies are shared between both the affected and unaffected twin and display the same level of heteroplasmic load in both twins, it is most likely that these variants represent biological variation that arose prior to the twinning event.
Table 2. Mutserve mitochondrial heteroplasmy calls for unique and shared heteroplasmy in discordant twin pair 2 aligned to reference rCRS with calls found in the affected twin in bold type and unaffected twin in roman type. Unique heteroplasmies are shown first followed by shared heteroplasmies. Heteroplasmy calls not found in the major Haplogrep assigned haplogroup (U4b1a3a) are indicated with ‘X’.
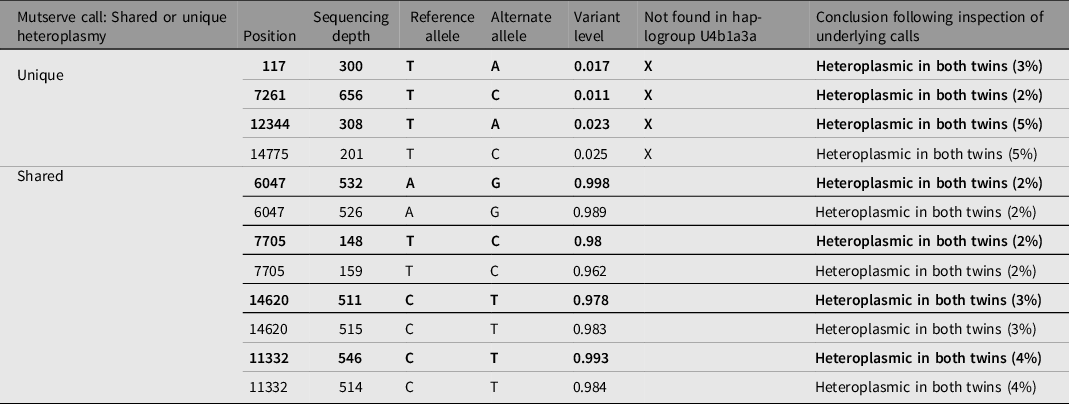
Table 3. Total heteroplasmy call count for Mutserve at a heteroplasmy calling threshold of 1%, 3% and 5% for each individual. Unshared call counts between twins are indicated in brackets.


Figure 5. IGV software screenshot of WGS alignments at mitochondrial location 12,344 for affected (left) and unaffected (right) twin pair 2 aligned to the rCRS reference genome. The affected twin had 95% T, 2% G, 1% C, 3% A base pair calls (330 T, 6G, 2C, 10A), a read depth of 348, and mean base and mapping quality of 17.9 and 37.5, respectively. The unaffected twin had 95% T, 2% G, 1% C, 2% A base pair calls (277T, 6G, 3C, 6A), a read depth of 567, and mean base and mapping quality of 19.1 and 37.9, respectively.
Discussion
The etiology of SZ is complex, with additional heterogeneity in clinical presentation. Although the nuclear genome of MZ twins discordant for SZ would theoretically be near identical, with rare postzygotic changes involving somatic mutations (Singh et al., Reference Singh, Castellani and Hill2020), it is possible that non-Mendelian genetic factors could contribute to the phenotypic outcome of SZ, including the contribution of the mitochondrial genome (Bi et al., Reference Bi, Tang, Zhang, Li, Chen, Yu, Chen and Yao2016). Furthermore, since the mitochondrial genome is inherited maternally, studies of mothers and their respective MZ offspring provides a prospect to trace the emergence of variation in the mitochondrial genome while controlling for variation in the nuclear genome. This study used next generation sequencing, genotyping arrays, and qPCR to assess mtDNA-CN and heteroplasmy variation in MZ twins discordant for SZ with their respective parents in a subset of twins.
mtDNA-CN has been shown to be associated with age, cardiovascular disease risk, diabetes and all-cause mortality, among other diseases (Ashar et al., Reference Ashar, Moes, Moore, Grove, Chaves, Coresh, Newman, Matteini, Bandeen-Roche, Boerwinkle, Walston and Arking2015; Ashar et al., Reference Ashar, Zhang, Longchamps, Lane, Moes, Grove, Mychaleckyj, Taylor, Coresh, Rotter, Boerwinkle, Pankratz, Guallar and Arking2017; Su et al., Reference Su, Li, Liu, Jiao, Shen, Yang, Yuan and Yao2022). More specifically, in discordant MZ twin studies, mtDNA-CN variation has been shown to be associated with sleep quality, obesity, and childhood intelligence (Avital et al., Reference Avital, Buchshtav, Zhidkov, Tuval Feder, Dadon, Rubin, Glass, Spector and Mishmar2012; Bijnens et al., Reference Bijnens, Derom, Weyers, Janssen, Thiery and Nawrot2019; Detjen et al., Reference Detjen, Tinschert, Kaufmann, Algermissen, Nürnberg and Schuelke2007; Wrede et al., Reference Wrede, Mengel-From, Buchwald, Vitiello, Bamshad, Noonan, Christiansen, Christensen and Watson2015). Therefore, mtDNA-CN could also be an important avenue for establishing non-Mendelian factors to SZ pathophysiology. Using MZ twins discordant for SZ, the results included in this report indicate that MZ twins discordant for SZ may display differences in mtDNA-CN and the direction of these differences involving affected and unaffected co-twins is independent of the technology used. Interestingly, a trend with a stronger concordance in mother-twin mtDNA-CN estimates for each twin compared to within-twin differences is noteworthy. However, this result must be taken with caution due to the small sample size and marginal statistical difference; therefore, it is critical to interpret these findings with caution and acknowledge the potential impact of the small sample size on the generalizability of the results. A possible reason for this trend could be explained by the fact that throughout the lifespan of the affected SZ twin, environmental and random genetic factors could have accumulated with inherited familial risk leading to the discordant SZ phenotype (Singh et al., Reference Singh, Castellani and Hill2020). With that, larger variation in mtDNA-CN between discordant MZ twins compared to mother-child could suggest a role for mtDNA-CN as one of the factors that causes a threshold ‘push’ towards the phenotypic SZ outcome (Singh et al., Reference Singh, Castellani and Hill2020). Given the complex nature of SZ, which involves multiple familial genetic, epigenetic and environmental factors, caution should be taken when interpreting these results (Hjelm et al., Reference Hjelm, Rollins, Mamdani, Lauterborn, Kirov, Lynch, Gall, Sequeira and Vawter2015; Pardiñas et al., Reference Pardiñas, Holmans, Pocklington, Escott-Price, Ripke, Carrera, Legge, Bishop, Cameron, Hamshere, Han, Hubbard, Lynham, Mantripragada, Rees, MacCabe, McCarroll, Baune, Breen and Walters2018; Singh et al., Reference Singh, Castellani and Hill2020). It is also probable that the degree of effect of mtDNA-CN on SZ is relatively small compared to other established genetic risk factors, which strongly requires that the sample size for future studies be expanded upon to control for these aforementioned factors.
Additionally, although WGS, microarray and qPCR provided a reliable measure of mtDNA-CN, our results favor qPCR over the Affymetrix technology for mtDNA-CN estimation. Further, to accurately quantify mtDNA-CN, it is crucial to consider the impact of DNA extraction methods on estimation. It has been shown that different extraction methods can lead to variations in mtDNA-CN measurements, likely arising from nonuniform changes in nDNA and mtDNA ratios. Studies utilizing different extraction methods have demonstrated that mtDNA-CN estimated from phenol-chloroform-isoamyl alcohol-based methods are significantly less variable than those isolated from traditional silica-based column selection methods (Guo et al., Reference Guo, Jiang, Bhasin, Khan and Swerdlow2009; Longchamps et al., Reference Longchamps, Castellani, Yang, Newcomb, Sumpter, Lane, Grove, Guallar, Pankratz, Taylor, Rotter, Boerwinkle and Arking2020). Additionally, repeated freeze-thaw cycles of samples has been found to decrease overall mtDNA-CN estimates, while whole blood samples yielded higher mtDNA-CN estimates as compared to buffy coat samples (Andreu et al., Reference Andreu, Martinez, Marti and García-Arumí2009). Taken together, optimizing these factors are crucial for obtaining reliable and consistent measurements of mtDNA-CN. Due to low sample sizes, we did not assess WGS in this comparison. As a result of differing sample size availability between measured mtDNA-CN techniques of microarray and qPCR and contrasting performance results to previous studies with larger sample sizes, these current findings should be interpreted with caution. In this study we identified consistent mtDNA-CN differences with shared directionally between MZD twins across different technological measures. Further understanding of factors that contribute to the differences in mtDNA-CN between discordant twins will be expected to provide insights into the etiology of SZ while identifying novel genetic and environmental factors that influence MZD twin dynamics. One such feature may be mitochondrial heteroplasmy, which we observed in this subset of MZ twins discordant for SZ to show complete concordance; however, we were also limited by both sample size and read depth.
The results included in this report show no clear unique heteroplasmy calls in the affected SZ twin compared to the unaffected co-twin. Our findings are consistent with earlier research on the relationship between mtDNA heteroplasmy in discordant SZ MZ twins (Li et al., Reference Li, Bi, Fan, Wu, Tang, Li, He, Zhou, Tang, Chen and Yao2017). The high concordance in mtDNA heteroplasmy between the discordant MZ twins may point to a relatively equal distribution of mtDNA during embryonic segregation and similar modulating effect from the nuclear genetic background. Additionally, this may also suggest other environmental and/or epigenetic factors are contributing to the discordance in SZ between MZ twins (Brown, Reference Brown2011; Dempster et al., Reference Dempster, Pidsley, Schalkwyk, Owens, Georgiades, Kane, Kalidindi, Picchioni, Kravariti, Toulopoulou, Murray and Mill2011; Fraga et al., Reference Fraga, Ballestar, Paz, Ropero, Setien, Ballestar, Heine-Suñer, Cigudosa, Urioste, Benitez, Boix-Chornet, Sanchez-Aguilera, Ling, Carlsson, Poulsen, Vaag, Stephan, Spector, Wu and Esteller2005). However, due to the complex nature of mtDNA heteroplasmy in SZ, interaction with the nuclear genome, and postzygotic genetic contributions to SZ, a multifaceted approach to the role of mtDNA heteroplasmy in SZ is required (Pardiñas et al., Reference Pardiñas, Holmans, Pocklington, Escott-Price, Ripke, Carrera, Legge, Bishop, Cameron, Hamshere, Han, Hubbard, Lynham, Mantripragada, Rees, MacCabe, McCarroll, Baune, Breen and Walters2018; Singh et al., Reference Singh, Castellani and Hill2020). Contamination can also be a significant factor leading to the presence of heteroplasmic mutations in individuals. In studies involving small sample sizes or employing high-throughput sequencing technologies, the risk of contamination increases (Yao et al., Reference Yao, Bandelt and Young2007). As a result, when analyzing the heteroplasmic mutations specific to a predetermined haplogroup variation may appear as a result of natural biological variation. The four non-U4b1a3a heteroplasmies shared by both twins do not contribute to a shared alternative haplogroup, further supporting the unlikelihood that they represent contamination. Implementing rigorous quality control measures and carefully assessing lineage and phylogenetic relationships aids in interpretation. In the case of this report, the existence of variants in both co-twins increases the likelihood that they represent true biological variation, though the potential for contamination cannot be exclusively eliminated.
In conclusion, we did not find a discernible difference in mtDNA heteroplasmy between MZ twins discordant for SZ based on our cut-off criteria using whole genome sequencing technology. However, we identified differences in mtDNA-CN that may or may not be related to twin discordance for SZ. Future research looking at mtDNA-CN and heteroplasmy in a larger cohort of MZ twin discordant for SZ and their families would further our understanding on how unique, shared, or changing heteroplasmy, and mtDNA-CN variation affects SZ risk.
Data availability statement
The data set supporting the results of this article is available at the Gene Expression Omnibus (GEO) repository, [GSE33598,http://www.ncbi.nlm.nih.gov/geo/query/acc.cgi?acc=GSE33598].
Acknowledgments
The authors would like to thank Dr Richard O’Reilly for the diagnosis and recruitment of the participants. Additionally, we would like to thank Amy Li for her computational assistance.
Financial support
This work was supported by funding from the Canadian Institutes of Health Research (CIHR, grant number R2258A15) and the Natural Sciences and Engineering Research Council of Canada (NSERC, grant number R2258) to S.S. as well as support from the Department of Pathology and Laboratory Medicine at Western University to C.C. P.W. is supported by the Ontario Graduate Scholarship (OGS).
Competing interests
The authors do not have any conflicts to disclose.
Ethical standards
The authors assert that all procedures contributing to this work comply with the ethical standards of the relevant national and institutional committees on human experimentation and with the Helsinki Declaration of 1975, as revised in 2008.