1. Introduction
Design success is heavily dependent on the interpretation of information, reasoning, and decision-making, making understanding the factors that influence these actions important (Pahl & Beitz Reference Pahl, Beitz and Wallace1996; Eppinger & Ulrich Reference Eppinger and Ulrich2004; Linsey & Viswanathan Reference Linsey and Viswanathan2010). One factor is bias: the tendency to lean in favor or against someone or something and the absence of a neutral viewpoint on the particular situation (Boysen & Vogel Reference Boysen and Vogel2009; Haselton, Nettle, & Andrews Reference Haselton, Nettle and Andrews2015; De Houwer Reference De Houwer2019). Bias is known to influence the interpretation of information, decision-making, reasoning and interpersonal relationships (Hewstone, Rubin, & Willis Reference Hewstone, Rubin and Willis2002; Boysen & Vogel Reference Boysen and Vogel2009; Haselton et al. Reference Haselton, Nettle and Andrews2015; Plews-Ogan et al. Reference Plews-Ogan, Bell, Townsend, Canterbury and Wilkes2020a). Therefore, a better understanding of bias and its impacts is key to achieving a deeper understanding of engineering design itself.
Bias exists in many forms: implicit, explicit and cognitive (Hewstone et al. Reference Hewstone, Rubin and Willis2002; Croskerry Reference Croskerry2003; Boysen & Vogel Reference Boysen and Vogel2009; Haselton et al. Reference Haselton, Nettle and Andrews2015; De Houwer Reference De Houwer2019). Cognitive bias surfaces during the various stages of cognition or mental action, including but not limited to decision-making, reasoning and interpretation of information (Croskerry Reference Croskerry2003). Different types of cognitive biases have been described, such as confirmation bias and hindsight bias. Croskerry (Reference Croskerry2003) details 32 different types of cognitive biases that may lead to diagnosis errors in the medical field (Croskerry Reference Croskerry2003). The process of making a medical diagnosis involves considering numerous factors systematically to make a decision (Wagner Reference Wagner1993; de Haes & Bensing Reference de Haes and Bensing2009; Chandiok & Chaturvedi Reference Chandiok and Chaturvedi2016). Similarly, cognitive activities used in the engineering design process involve systematical considering factors to develop a solution via brainstorming or reflective processes (Seidel & Fixson Reference Seidel and Fixson2013). These similarities suggest that identified cognitive biases in medicine may exist in engineering design as well. Implicit and explicit bias are seen in the behaviors or actions of the entity or individual demonstrating the bias (Boysen & Vogel Reference Boysen and Vogel2009; De Houwer Reference De Houwer2019). Implicit bias is an unacknowledged action by the individual demonstrating bias (De Houwer Reference De Houwer2019). Explicit bias is expressed as overt, intentional actions or expressions by an individual as opposed to the subconscious nature of implicit bias. (Hewstone et al. Reference Hewstone, Rubin and Willis2002; Boysen & Vogel Reference Boysen and Vogel2009).
Bias can negatively influence decision-making, suggesting that it also negatively influences key processes in engineering design tasks such as idea generation, concept selection and collaborative decision-making (Boysen & Vogel Reference Boysen and Vogel2009; Croskerry Reference Croskerry2003; Hewstone et al. Reference Hewstone, Rubin and Willis2002; Howard, Culley, & Dekoninck Reference Howard, Culley and Dekoninck2008; Pahl & Beitz Reference Pahl, Beitz and Wallace1996). A detailed investigation into bias is crucial to understanding how bias may negatively influence engineering design outcomes. (Croskerry Reference Croskerry2003; Moss-Racusin et al. Reference Moss-Racusin, Sanzari, Caluori and Rabasco2018; Parks Reference Parks2018; Plews-Ogan et al. Reference Plews-Ogan, Bell, Townsend, Canterbury and Wilkes2020a, Reference Plews-Ogan, Bell, Townsend, Canterbury and Wilkes2020b) It is more known for bias to have a negative impact; however, understanding and implementing bias can have a positive impact (Xi, Fu, & Yang Reference Xi, Fu and Yang2013). Xi et al. (Reference Xi, Fu and Yang2013) explored how leveraging bias into predictive models can improve design outcomes (Xi et al. Reference Xi, Fu and Yang2013). Similarly, exploring heuristics and biases has played a positive role in idea generation and collaborative decision-making (Kahneman & Tversky Reference Kahneman and Tversky1974). Heuristics are defined as the cognitive pathways or frameworks used to reach a solution (Griffin, Daniel, & Gilovich Reference Griffin, Daniel and Gilovich2002). Biases, as defined previously, are the tendency to lean in favor or against someone or something and the absence of a neutral viewpoint on the particular situation (Boysen & Vogel Reference Boysen and Vogel2009; Haselton et al. Reference Haselton, Nettle and Andrews2015; De Houwer Reference De Houwer2019). In relation to heuristics, biases are the errors that influence the heuristics. The scope of this review focuses on biases influencing design outcomes. In addition to design outcomes, bias, both implicit and explicit, can affect interpersonal relationships (Croskerry Reference Croskerry2003; Moss-Racusin et al. Reference Moss-Racusin, Sanzari, Caluori and Rabasco2018; Parks Reference Parks2018; Plews-Ogan et al. Reference Plews-Ogan, Bell, Townsend, Canterbury and Wilkes2020a, Reference Plews-Ogan, Bell, Townsend, Canterbury and Wilkes2020b).
Design is heavily dependent on interpersonal relationships because design is conducted by heterogeneous teams with a variety of roles, skills and perspectives, making it important to understand how interpersonal bias may impact design teams (Clemmensen, Ranjan, & Bødker Reference Clemmensen, Ranjan and Bødker2018; Elena & Summers Reference Elena and Summers2019; Green Reference Green2006; Prabhu et al. Reference Prabhu, Miller, Simpson and Meisel2020; Vestergaard, Hauge, & Hansen Reference Vestergaard, Hauge and Hansen2016). The exploration and study of the impacts on interpersonal relationships in design can enable designers to explore mitigation strategies to counter or avoid negative impacts and achieve positive outcomes. However, bias has not been studied and reported extensively in the design literature. Therefore, we turn to medical practice, a field in which bias has been studied in-depth, to provide clues for its presence and influence in design. In the medical field, biases affecting diagnosis, treatment, disease prevention and medical research are investigated to mitigate negative health outcomes and improve human health (McGauran et al. Reference McGauran, Wieseler, Kreis, Schüler, Kölsch and Kaiser2010; Saposnik et al. Reference Saposnik, Redelmeier, Ruff and Tobler2016; Baker et al. Reference Baker, Smith, Jacobs, Houmanfar, Tolles, Kuhls and Piasecki2017; Plews-Ogan et al. Reference Plews-Ogan, Bell, Townsend, Canterbury and Wilkes2020a). As the actions, tasks and events in the field of medicine parallel those in the field of engineering design, the similarities enable the use of existing bias research in medicine to guide bias research in engineering design.
The objective of this study is twofold. Aim one is to systematically review the literature on bias in the field of medicine. Aim two is to leverage knowledge gained from existing research on bias in medicine to highlight potential areas where bias may exist in the field of engineering design. The overarching goal of this work is to provide a resource to the engineering design community to support future research investigating the influence of bias, and so this begins with a systematic review of bias research in engineering design to identify gaps. As previously stated, bias can be explicit, implicit or cognitive and show up in interpersonal relations. For this study, different biases that may potentially exist in engineering design are identified from the field of medicine and grouped into three classes: interpersonal, cognitive and other, as seen in Figure 3. The following section details why bias research in the medical field is useful for highlighting potential bias in engineering design and elaborates on the scope of this review. After the background section, the following section details the approach used to collect and synthesize publications on bias in the medical field. The results section connects the medical field to engineering design to pinpoint potential bias in engineering design. The final section summarizes the findings and proposes future research areas and questions regarding bias and its role in engineering design.
2. Background: analogies between the field of medicine and engineering design
Bias is known to influence a person’s thought processes and actions. Both explicit and implicit cognitive bias can lead to misinformed decisions and affect interpersonal relationships (Kahneman & Tversky Reference Kahneman and Tversky1974; Lehner et al. Reference Lehner, Seyed-Solorforough, O’Connor, Sak and Mullin1997). Engineering design utilizes highly cognitive and qualitative processes (e.g., decision-making, design thinking and interpersonal relationships in the form of team collaboration), making the process vulnerable to bias. Bias has been explored for decades in psychology and other social sciences (Baron & Hershey Reference Baron and Hershey1988; Hewstone et al. Reference Hewstone, Rubin and Willis2002; Boysen & Vogel Reference Boysen and Vogel2009; Marshall et al. Reference Marshall, Trimmer, Houston and McNamara2013; De Houwer Reference De Houwer2019). Medical research has leveraged the field of psychology’s bias research to understand the role bias plays in social interactions and decision-making in the medical field (Aberegg, Arkes, & Terry Reference Aberegg, Arkes and Terry2006; Levy & Hershey Reference Levy and Hershey2008; Stone & Moskowitz Reference Stone and Moskowitz2011; Saposnik et al. Reference Saposnik, Redelmeier, Ruff and Tobler2016). The large body of work on bias in medicine can be used as a guide to investigate bias in engineering design to improve design outcomes.
Though the tasks involved in engineering and medicine are not explicitly the same, the themes in medical research, education and practice parallel those in engineering design research, education and practice. Researchers have previously used events in engineering to investigate similar events in medicine and vice versa (Frey & Dym Reference Frey and Dym2006; Stripe et al. Reference Stripe, Best, Cole-Harding, Fifield and Talebdoost2006; Zeltser & Nash Reference Zeltser and Nash2010). For example, Zeltser and Nash (Reference Zeltser and Nash2010) highlighted teamwork training programs in aviation to provide a conceptual framework for evaluating the effectiveness of teamwork training in medicine (Zeltser & Nash Reference Zeltser and Nash2010). Aviation teams, design teams and medical teams share similar characteristics in their approaches to risk reduction and the importance of interpersonal skills, making the field of medicine an ideal tool for exploring bias in engineering design.
To date, the concept of bias as a potential variable in engineering design is under-recognized and understudied. The similarities between engineering design and the medical domain allow for existing work in the field of medicine to act as a roadmap for a deeper exploration of bias in engineering design (Frey & Dym Reference Frey and Dym2006). High-level similarities between the field of medicine and engineering design can be seen in Figure 1 and are subsequently discussed in further detail.

Figure 1. High-level similarities between engineering design and the field of medicine.
The history of the fields of medicine and engineering design may be explicitly different in context, but both fields are rooted in the practice of problem-solving. The primary purpose of medicine is to diagnose, treat and prevent diseases and medical ailments. Diagnosis, treatment and prevention are executed in daily practice but also understood and taught via medical research, and education and training of medical providers. The goal is to ultimately provide solutions to health problems using informed techniques and practices. The central goal of the field of medicine parallels the goal of engineering design but in a different context. The goal of engineering design is to provide solutions to technological and social problems using informed engineering techniques and practices. To meet this goal, designers also utilize design in practice, design research and design education and training to understand and further improve the field of design.
In addition to common objectives, both fields have similar core relationships; the patient–provider relationship in medicine and the designer–end-user relationship in engineering design. The medical provider, whether a physician, nurse or physician assistant, processes the information provided by the patient and then looks to provide a potential solution. This echoes the interaction that occurs between the designer and the end-user (Frey & Dym Reference Frey and Dym2006). The end-user provides information on their needs, or the designer collects information based on the problem identified and then processes that information to provide a designed solution. In addition to similar approaches to collecting information, medical providers and design engineers have similarities in how they handle the information once collected (de Haes & Bensing Reference de Haes and Bensing2009). For instance, to provide solutions to group health problems, instead of individual patients, medical providers use datasets to identify trends and investigate solutions that can be applied to larger groups. This occurs in engineering design when designers develop solutions that can be applied to groups of diverse end-users. Finally, both fields share problem-solving techniques. Figure 2 illustrates the parallels between the medical diagnosis and the design process. The medical diagnosis process is based upon a description provided in the 2015 National Academics Press (Balogh, Miller, & Ball Reference Balogh, Miller, Ball, Balogh, Miller and Ball2015). The engineering design process description is based on the Howard et al. (Reference Howard, Culley and Dekoninck2008) framework for the engineering design process. Actions and Tasks that Occur in Phase are defined as events that occur in that phase or the information that may be collected in that phase. For example, Clinical history represents an information type that may be collected by a medical practitioner from their patient.
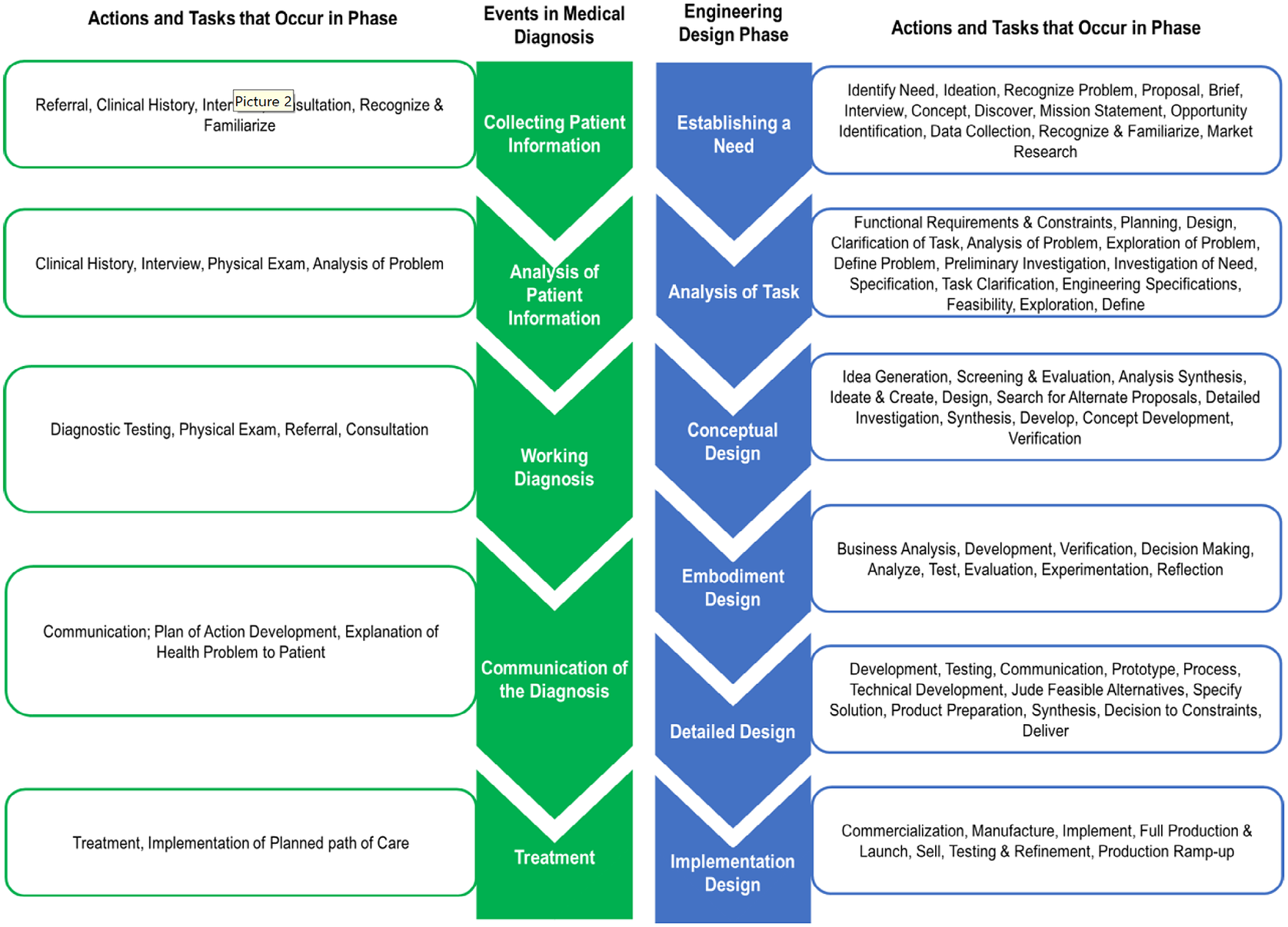
Figure 2. Conceptual process model comparison of medical diagnosis process and the engineering design process (Howard et al. Reference Howard, Culley and Dekoninck2008; Balogh et al. Reference Balogh, Miller, Ball, Balogh, Miller and Ball2015).
As seen in Figure 2, the engineering design process can be divided into subsequent phases. These phases include Establishing the need, analysis of task, conceptual design, embodiment design phase, detailed design and implementation design. Many actions and tasks in medical practice parallel ones in engineering design especially with regard to the process physicians use to come to a medical diagnosis. The establishing the needs phase is equivalent to when the physician collects information from the patient and other available resources. The analysis of task phase equates to when physicians identify both positive and negative findings from the information provided by the patient or the available resources. The conceptual design phase is when the project needs and problem space are interpreted, and the designer begins to generate and identify concepts that can be potential solutions. The embodiment design phase is when the concepts established are developed into tangible prototypes or solutions. The detailed design phase is the phase where the final solution is developed. These phases are not as evidently related to the medical diagnosis process. However, these phases parallel when medical professionals synthesize the findings established from the prior phases into a clinical diagnosis and then use that diagnosis to adopt a plan of action. The final stage, the implementation design phase, is when the final design solution is deployed, and the commercialization or application of the solution is executed and monitored. The final phase is similar to when physicians apply their plan of action in response to their diagnosis, most often when the treatment plan is formulated and implemented.
Beyond the methods used in practice, similarities also exist in the education and training approaches used to establish problem-solving methods. Medical providers are trained to address society’s health needs (Bloom Reference Bloom1988). Medical providers and design engineers solve problems using skills and techniques that parallel each other. The education and training to learn those problem-solving skills also have similarities, starting with the trajectory of the curriculum. In most curriculums, engineering design is taught at the upper-level undergraduate level, building on previously taught engineering concepts (Emami, Bazzocchi, & Hakima Reference Emami, Bazzocchi and Hakima2020). Some engineering schools introduce engineering design early in the undergraduate curriculum to complement other engineering courses and to familiarize students with engineering design early. Overall, engineering design is intended to enable students to integrate and use engineering skills to solve design problems. In the medical field, students major in STEM or related fields and then integrate and use those skills in medical school. To integrate and use skills, both medical students and students in engineering design participate in training, coursework and projects that have a mixture of cooperative, inquiry and integrated learning pedagogical approaches.
Though there are several similarities between the field of medicine and engineering design, there are differences between the fields that need to be mentioned. In practice, a medical practitioner is more likely to apply evidence-based, standard treatments to develop a diagnosis (Brush, Sherbino, & Norman Reference Brush, Sherbino and Norman2017; Corazza, Lenti, & Howdle Reference Corazza, Lenti and Howdle2020; Cusimano Reference Cusimano1996). In design, a systematic approach is used to collect and deduce information for a design solution (Pahl & Beitz Reference Pahl, Beitz and Wallace1996). However, a key difference between the practices applied to determine a diagnosis and develop a design solution is that designers are more likely to implement unconventional solutions. This may be due to another key difference between the fields that the medical field is explicitly higher risk than engineering design. Another key difference is the type of information medical professionals consider when making a diagnosis. Medical professionals collect contextual information, just as designers do to make a design solution. However, medical professionals systematically are more inclined to consider information on emotions and respond to those emotions(de Haes & Bensing Reference de Haes and Bensing2009). This may be due to the need for them to have a bedside manner with patients. The differences between engineering design and the field of medicine still allow for the comparison of biases in both fields. However, in comparing and identifying biases in engineering based on biases in the field of medicine, it is important to recognize the differences in context to identify how biases may show up.
Other fields more closely related to engineering design, such as software engineering, have studied bias within their field (Mohanani et al. Reference Mohanani, Salman, Turhan, Rodriguez and Ralph2020). Mohanani et al. (Reference Mohanani, Salman, Turhan, Rodriguez and Ralph2020) systematically reviewed bias in software engineering and identified what bias has been researched. Though Mohanani’s work shows bias has been explored in a field that is more closely related to engineering design, he also finds that there have been limited studies on ways to mitigate bias (Mohanani et al. Reference Mohanani, Salman, Turhan, Rodriguez and Ralph2020). The goal of this work is not only to pinpoint potential bias but to drive future work to find ways to mitigate that bias. Bias research in the field of medicine was used as an analogy for bias in engineering design because some studies in medicine have identified ways to mitigate bias (Benoit et al. Reference Benoit, Travis, Swan Sein, Quiah, Amiel and Gowda2020). In addition to providing ways to mitigate bias, bias research in the field of medicine also explores bias in three foundational areas of medicine: medical research, medical practice and medical education. Limited research exists in niche areas of engineering that cover education or instructional bias, research bias and bias that occurs as a practicing engineer in that specialty. Cognitive bias and bias have been studied extensively in psychology (Hotopf Reference Hotopf1958). However, bias in the field of medicine was chosen for this comparison because it has been used as an analogy to engineering design (Frey & Dym Reference Frey and Dym2006) as well as bias research has been studied in three distinct domains; education, research and practice (Capers et al. Reference Capers, Clinchot, McDougle and Greenwald2017; Green et al. Reference Green, Carney, Pallin, Ngo, Raymond, Iezzoni and Banaji2007; Mendel et al. Reference Mendel, Traut-Mattausch, Jonas, Leucht, Kane, Maino, Kissling and Hamann2011). Engineering design applies to many fields of engineering, so leveraging and encompassing fields such as medicine will highlight the reach of potential bias within the field of engineering design.
3. Review of bias in engineering design
The ultimate goal of this paper is to further drive research on bias in engineering design, driving research to investigate bias, understand its impacts and develop approaches to mitigate bias. To do so, the first part of this review examines bias research in engineering design to paint a picture of how bias has been explored in engineering design. The engineering design space encompasses many fields of engineering. Our review of the existing literature on bias research in engineering design has yielded only a few studies, with the majority of the focus on tool bias and designer bias with concept selection or evaluations (Anderson & Gilbride Reference Anderson and Gilbride2007; Gopsill Reference Gopsill2018; Gweon et al. Reference Gweon, Jun, Finger and Rosé2017; Hallihan & Shu Reference Hallihan and Shu2013; Howard & Borenstein Reference Howard and Borenstein2018; Jansson & Smith Reference Jansson and Smith1991; Khoje et al. Reference Khoje, Günay, Kremer, Park, Jackson and Wu2018; Mueller et al. Reference Mueller, Melwani, Goncalo, Mueller, Melwani and Gonçalo2012; Nelius et al. Reference Nelius, Doellken, Zimmerer and Matthiesen2020; Ostafichuk & Sibley Reference Ostafichuk and Sibley2019; Savage et al. Reference Savage, Miles, Moore and Miles1996; Stacey et al. Reference Stacey, Petre, Rzevski, Sharp and Buckland1996; Toh, Strohmetz, & Miller Reference Toh, Strohmetz and Miller2016; Zheng, Ritter, & Miller Reference Zheng, Ritter and Miller2018). Zheng et al. (Reference Zheng, Ritter and Miller2018) investigated the impacts of a concept selection tool on promoting creative ideas and decision-making among designers (Zheng et al. Reference Zheng, Ritter and Miller2018). The findings indicate that designers are inclined to select ideas that were highly ranked by design tools only if the concept met their expectations, hinting at the existence of a cognitive bias in decision-making (Zheng et al. Reference Zheng, Ritter and Miller2018). This study suggests that bias should be considered when investigating the effects of design tools. Jansson & Smith (Reference Jansson and Smith1991) investigated if design fixation is measurable (Jansson & Smith Reference Jansson and Smith1991). The findings suggest design fixation is measurable and may be influenced by cognitive factors measurable with cognitive science research techniques. Design fixation is an extension of cognitive research that is explored in engineering design (Vasconcelos & Crilly Reference Vasconcelos and Crilly2016; Crilly Reference Crilly2019) and may benefit from design bias. Mueller et al. (Reference Mueller, Melwani, Goncalo, Mueller, Melwani and Gonçalo2012) investigated why people reject creative ideas and found that a participants’ bias against creativity may have interfered with their ability to recognize a creative idea (Mueller et al. Reference Mueller, Melwani, Goncalo, Mueller, Melwani and Gonçalo2012). Mueller’s study demonstrated that participants exhibited a negative bias against creativity when they were uncertain of the final design solution (Mueller et al. Reference Mueller, Melwani, Goncalo, Mueller, Melwani and Gonçalo2012).
Similar to uncertainty, Savage (Reference Savage, Miles, Moore and Miles1996) explored the impacts of cognitive overload and bias on engineering design and found that there is roughly a 50% chance certain information such as time and cost can influence the design outcome (Savage et al. Reference Savage, Miles, Moore and Miles1996). Similar to Savage’s study, Gopsill (Reference Gopsill2018) looked at how factors may bias design outcomes when it comes to construction kits (Gopsill Reference Gopsill2018). These studies point out how design constraints drive solutions but also bias solutions, making it important to understand the extent and impact they bias solutions. Hallihan and Shu (Reference Hallihan and Shu2013) found that confirmation bias influences the evaluation of design research and can contribute to deviation from scientifically accurate conclusions (Hallihan & Shu Reference Hallihan and Shu2013). Nelius et al. (Reference Nelius, Doellken, Zimmerer and Matthiesen2020) also explored confirmation bias in engineering design by investigating its impact on reasoning and visual attention. The findings from this study show that confirmation bias plays a role in the interpretation of the problem and solution space. These studies show ways bias has been investigated in engineering design but suggest more aspects of engineering design bias exists. Concept selection has been at the forefront of bias research in design due to the importance of selecting the best solutions (Stacey et al. Reference Stacey, Petre, Rzevski, Sharp and Buckland1996; Toh et al. Reference Toh, Strohmetz and Miller2016). Stacey et al. (Reference Stacey, Petre, Rzevski, Sharp and Buckland1996) explored bias from design tools used in concept selections by investigating the use of a design tool that strategically leverages design tool bias for concept selection (Stacey et al. Reference Stacey, Petre, Rzevski, Sharp and Buckland1996). Toh et al. (Reference Toh, Strohmetz and Miller2016) investigated ownership bias in concept selection as a result of the designer’s gender (Toh et al. Reference Toh, Strohmetz and Miller2016). Though it is important to understand the bias impacting concept selection, it is also important to understand where else within the design process and design tasks bias is occurring.
Bias has been explored in feedback in engineering design (Anderson & Gilbride Reference Anderson and Gilbride2007; Gweon et al. Reference Gweon, Jun, Finger and Rosé2017; Khoje et al. Reference Khoje, Günay, Kremer, Park, Jackson and Wu2018; Ostafichuk & Sibley Reference Ostafichuk and Sibley2019). Ostafichuk and Sibley (Reference Ostafichuk and Sibley2019) confirmed the presence of self-bias and gender bias in student peer evaluations when students evaluated women students more favorably (Ostafichuk & Sibley Reference Ostafichuk and Sibley2019). Other research in engineering has looked at gender bias in engineering, but there are still a lot of unknowns (Khoje et al. Reference Khoje, Günay, Kremer, Park, Jackson and Wu2018). Anderson and Gilbride (Reference Anderson and Gilbride2007) explored the influence of outreach programs on gender bias and female student consideration for engineering careers (Anderson & Gilbride Reference Anderson and Gilbride2007). Anderson’s study shows that outreach does bias female students, ultimately improving consideration for engineering careers (Anderson & Gilbride Reference Anderson and Gilbride2007). Though gender bias was found to exist, statistical methods were used to identify bias instead of validated tests that measure the students’ bias (Ostafichuk & Sibley Reference Ostafichuk and Sibley2019) (Anderson & Gilbride Reference Anderson and Gilbride2007). To combat gender bias, Khoje et al. (Reference Khoje, Günay, Kremer, Park, Jackson and Wu2018) developed an evaluation framework to evaluate the appropriateness of the design projects (Khoje et al. Reference Khoje, Günay, Kremer, Park, Jackson and Wu2018). Khoje’s framework is a questionnaire intended to be used by engineering educators to determine the appropriateness of design projects and determine the level of gender bias in the design project (Khoje et al. Reference Khoje, Günay, Kremer, Park, Jackson and Wu2018). This questionnaire was developed from a literature review of studies that identify gender bias from a statistical standpoint. Though this framework is beneficial, it would benefit if it tackled bias that were measured from validated models. Gweon et al.’s (Reference Gweon, Jun, Finger and Rosé2017) study also highlights how bias from an instructor may have a negative impact. Gweon et al. (Reference Gweon, Jun, Finger and Rosé2017) explored bias in engineering design differently by looking at bias from instructor feedback in project-based learning and ways to mitigate bias from the instructor (Gweon et al. Reference Gweon, Jun, Finger and Rosé2017). Leveraging bias to improve design outcomes was explored by Xi et al. (Reference Xi, Fu and Yang2013) in computation design (Xi et al. Reference Xi, Fu and Yang2013). Xi et al. (Reference Xi, Fu and Yang2013) explored an approach to correct model predictions used in reliability-based design based on characterized model bias (Xi et al. Reference Xi, Fu and Yang2013). This study demonstrates how bias can be leveraged to improve design outcomes in the computational design space. Several studies in engineering design have focused on debiasing methods to reduce bias in engineering design (Cheong & Shu Reference Cheong and Shu2013; Emmons et al. Reference Emmons, Mazzuchi, Sarkani and Larsen2018; Kinsey et al. Reference Kinsey, Kinateder, Gwynne and Hopkin2021; Hancock et al. Reference Hancock, Klotz, Shealy, Johnson, Weber, Stenger and Vuppuluri2022). But there are limited studies that leverage bias to benefit engineering design outcomes outside of computational design.
The existence and influence of bias on engineering design outcomes have been understudied. The engineering design studies reviewed highlight under-researched aspects of bias in engineering design making the need to understand where bias exists that much more important. The lack of bias research in areas outside of designer bias regarding the generated solution and the bias exhibited by design tools highlights the need to improve the understanding of the extent to which bias potentially exists in engineering design. Though existing work is limited, it is demonstrated that bias exists in engineering design. A lot of work has explored bias in the design tools. Work that has explored gender, race or other factors that influence bias used trends in data to support the existence of bias (Anderson & Gilbride Reference Anderson and Gilbride2007; Khoje et al. Reference Khoje, Günay, Kremer, Park, Jackson and Wu2018; Ostafichuk & Sibley Reference Ostafichuk and Sibley2019). However, few studies measure bias with validated tools to identify its existence. Engineering design research in bias shows that understanding what bias exists can help understand how it can be beneficial to design outcomes. This suggests that understanding the existence of bias more extensively from statistical measures of gender, and race, but measuring bias with a validated tool may allow engineers to develop frameworks that mitigates the negative impacts of bias. The field of medicine may act as a blueprint for engineering design to mitigate bias because they use validated measures in addition to statistical measures to identify bias and develop ways to mitigate bias.
Bias has been investigated in engineering design, but there is room to further drive its investigation in engineering design to understand the influence and impacts of bias. No framework and general concept of potential bias research in engineering design exist to help drive bias research in the field. To fill this gap, the next portion of this review intends to leverage bias research in medicine to fill this void and understand potential bias research in engineering design. This review focuses on bias in the field of medicine to then identify if the same bias in engineering design may exist. A systematic review of bias in design was not conducted; only a high-level review of bias in design was conducted. The following sections detail the approach used to provide a summary of bias in the medical field while highlighting potential areas in engineering design that might be specifically affected by bias, providing a roadmap for future exploration of bias in engineering design.
4. Literature review approach: leveraging bias research in the field of medicine to pinpoint potential bias in engineering design
The aim of this study is twofold; systematically review the literature on bias in the field of medicine, and then use the bias research to highlight potential areas where bias may exist in the field of engineering design. To address both aims of our study two reviews of the literature were conducted:
-
1. Studies investigating the presence, context and mitigation of bias in the field of medicine were reviewed and findings were extrapolated to the field of engineering design.
-
2. Studies of bias in engineering design were selected to summarize existing research and highlight potential areas where bias may exist.
To meet the overarching goal of the study of providing a framework for future bias research in engineering design, it is important to conduct a review of existing bias research in engineering design. This highlights what research has been conducted in engineering design to not only establish what bias is known in engineering design but also use research in medicine to pinpoint areas that may be unknown gaps. This allows one to indicate the gaps in bias research in engineering design. To outline existing bias in engineering design, a qualitative review of bias research in the field of medicine is conducted. Using bias research in the field of medicine, the potential bias in engineering design was highlighted by aligning tasks, actions and events that are similar in both fields. Research publications on bias in the field of medicine were collected and reviewed for the study. In the medical field, bias can be exhibited by the physician(s) to the patient(s), among physicians and medical providers, and by the patient(s) to the medical provider(s). For the scope of this study, only bias demonstrated by medical physicians, medical professionals and/or medical resources were considered. The following section details the study approach used. The following sections detail the systematic search, screening, selection and review process.
4.1. Literature search
The first aim of this study was to systematically review publications on bias in the field of medicine by pinpointing publications that documented events and tasks in the field of medicine where bias is present. To collect published literature on bias in medicine, the Penn State University Libraries and PubMed research database search engines were used. Several search terms were used to search for publications within the databases. The search teams used included “bias in medicine”, “bias in medical decision-making”, “bias in medical diagnosis”, “medical bias”, “bias”, “medical research bias” and “physician bias”. The PubMed search resulted in over 60,000 results, but the Penn State Libraries broad database search resulted in over 1 million hits.
4.2. Screening of literature
The resulting journal publications were screened for the study in the order they resulted from the search, based on the default “relevance” order in the search engine. The first screening of the literature consisted of screening study titles. The titles of the resulting journal articles were screened to ensure that they indicated a specific type of bias and a specific context in which the bias occurred. Titles that mentioned the bias at the focus of the study, as well as the action, event or task where the bias occurs, were selected. From there, the abstracts of the selected publications were screened for study specifications. The criteria for screening abstracts were that the abstract needed to (1) identify that the objective of the study was to investigate bias, (2) indicate that bias was measured in some way in the study, (3) describe the context, including the action, task and/or event, where the bias investigated in the study was identified, and (4) state the impact(s) the investigated bias has on an identified area within the field. Publications that did not fit these criteria were omitted. These criteria were established to ensure that the literature included in the review contained sufficient information to support subsequent comparison and analysis. Due to the large number of studies resulting from the search, studies that investigated the same bias in similar medical events, actions and/or tasks were not considered. This was done to allow for unique occurrences of bias to be highlighted while not over-saturating the data reviewed in the analysis. The remaining publications were screened by reading the full publications.
4.3. Data extracted from publications on bias in the field of medicine
For publications that satisfied the title and abstract criteria, data was extracted to provide context and address the first aim of the study as well as to begin to address the second aim of the study. The types of extracted data are enumerated and defined in Table 1. In addition to this information, the citation information, such as the author, publication year and publication entity, was collected.
Table 1. Definition of data extraction categories.

The terms listed in the study were used by the author to provide a quasi-framework for each of the studies reviewed in the literature survey. For the scope of this review, Investigated Bias or Used Bias to Interpret Data is defined as whether the study investigated bias and the impacts of bias or used bias to interpret data findings. Investigated Bias means that the study investigated if bias exists in given situations, scenarios or cases. Oftentimes, these studies used validated tools to measure bias. These studies also may have looked at the outcomes of different biases. Used Bias to Interpret Data is defined, in the scope of this review, which means the study used known factors that lead to bias, such as race, gender and experience, to identify any trends in data. The Bias Agent is used to identify the entity performing the bias and the Bias Recipient is used to identify the entity impacted by the bias.
4.4. Data analysis: identifying potential bias in engineering design
Using data from Table 1, which was extracted from medical publications, paralleling scenarios were established for the field of engineering design. This was done using the “Field” data, the “Context” data, and the “Event, Action, and/or Task Investigated” data. The “Field” category from Table 1 was used as a high-level category to identify scenarios in engineering design. The three high-level Fields used were medical research, medical education and medical practice. These fields parallel engineering design research, design education and design in practice domains. The data extracted as “Context” and “Event, Action, and/or Task Investigated” were then used to identify similar scenarios in the field of engineering design education, research and engineering design in practice. The events, actions and tasks in engineering design education and research that paralleled the cases in medical education and research were identified. Once identified, the context for where this bias may occur in engineering design education and research was also identified. To address the second focus of the study, the gaps in current engineering design research, a search of engineering design research was conducted to establish the extent to which the bias has been investigated and summarized.
The second aim of the paper is to leverage knowledge gained from existing research on bias in medicine to highlight potential areas where bias may exist in the field of engineering design. The overarching goal of the paper is to provide a resource to the engineering design community that helps researchers understand the state of bias research in engineering design and the potential for furthering the understanding of that phenomenon. Toward that goal, the final section of this paper identifies where bias may exist in engineering design and subsequently generates strong hypotheses for future engineering design research to investigate bias for understanding and mitigation.
5. Results
5.1. Selected literature on bias in the field of medicine
For the systematic review of bias in the field of medicine, 30 unique publications were identified. Details for each publication, including journal, title, author and date, domain (education, research and/or in practice), bias agent, bias recipient, context, event, action and/or task investigated, and study design are listed in Table 2 in the Appendix. Given the parallel relationship between engineering design and medicine, these studies were analyzed to identify where and what bias may exist in the field of engineering design.
5.2. Potential bias in engineering design
The evaluation of selected publications on bias in the field of medicine was organized within three domains of reported bias studies: medical education, medical practice and medical research. Findings from the selected publications were categorized within at least one of these three domains based on the context of the study and the event, action and/or task investigated. These three domains served as a framework to place and investigate potential bias in engineering design. Any action, event or task occurring in the process of education and training of medical professionals was considered when extracting bias in medical education. Bias occurring in the following areas of medical education was considered for this study: textbooks and training tools, the recruitment and admissions process, as well as the educational curriculum. The medical practice domain encompasses any duties and tasks the medical providers utilize while executing their role as a provider. Lastly, the medical research domain includes tasks involved in funding, conducting, presenting and publishing research. Based on the described intersection of the medical field and engineering design, some tasks may be reflected in multiple domains. Data in these three domains are presented as parallels to engineering design to highlight reported bias.
Figure 3 depicts bias present in education, practice and research for both engineering design and medicine fields based on the articles reviewed in the literature survey. Bias presence is divided into three categories, interpersonal, cognitive and other. Indicators in the graphic highlight where bias is known to occur in medicine and where bias potentially exists in similar areas in engineering design. It is also indicated where bias may potentially exist in engineering design based on known bias in the field of medicine because this review compared the two fields. Known bias, reported in a publication is indicated by a solid, filled circle in Figure 3. An empty circle does not mean bias does not exist; it means the bias was not identified in the comparison made in this review. A hatched circle indicates that the bias was identified and reported in a publication in the field of medicine and, based on a similar context, potentially exists in the field of engineering design. The subsequent sections summarize these findings in further detail. Specifically, comparisons are made in terms of education, practice and research.

Figure 3. Conceptual diagram of bias present in education, practice and research for the field of medicine and engineering design. (The different colors are to differentiate the column).
5.2.1. Comparing medical education & engineering design education
Education, in the scope of medical education and engineering design education, includes tasks from admission into an educational program to acting as a faculty member in educational programs. Bias has been described and identified in medical school admissions (Griffin & Wilson Reference Griffin and Wilson2010; Capers et al. Reference Capers, Clinchot, McDougle and Greenwald2017). Prompted by a lack of diversity in medical schools, Capers et al. (Reference Capers, Clinchot, McDougle and Greenwald2017) investigated racial bias in medical school admissions and found that admissions committee members demonstrated an implicit white preference (Capers et al. Reference Capers, Clinchot, McDougle and Greenwald2017). The admission committee members included faculty and students. The findings showed that regardless of gender or role, all committee members demonstrated this white preference bias implicitly but not explicitly. While the format of the admission process in engineering design differs from medical school admissions, bias may also exist in the application and admittance process of engineering programs. An implicit racial bias may exist in the admissions procedures or admissions committees of engineering programs, impacting the diversity of engineering designers. The implicit white preference racial bias was found to be one of the factors that influence diversity in medical school. Capers et al. (Reference Capers, Clinchot, McDougle and Greenwald2017) noted that the academic class admitted after the Implicit Association Test (IAT) intervention was the most diverse in the school’s history, supporting the influence of racial bias on diversity in education.
The reported white preference by admission committees is reflected in the interviews conducted for admission into medical programs (Griffin & Wilson Reference Griffin and Wilson2010). During the admissions process for medical school, applicants undergo multiple mini-interviews. Griffin & Wilson (Reference Griffin and Wilson2010) found that the interviewers show leniency interviewer bias toward interviewees based on the personality and gender of the interviewee (Griffin & Wilson Reference Griffin and Wilson2010) by being more permissive and sparing toward the interviewee (Griffin & Wilson Reference Griffin and Wilson2010). This bias is reflected in the interviewer’s evaluations of the interview even though the bias demonstrated was small. Evaluations such as these may occur in interviews of applicants for engineering programs. Though interviewing may not occur in some programs, leniency bias can also show up in the review of applicant materials. Interviewer leniency bias for interviewees of similar gender and personalities can cause engineering programs to lack diversity based on gender as well as mindsets. Engineering and STEM programs are known for a lack of diversity and inclusion (Briggs Reference Briggs2017; Jones et al. Reference Jones, Williams, Whitaker, Yingling, Inkelas and Gates2018; Botella et al. Reference Botella, Rueda, López-Iñesta and Marzal2019). For 2019, the American Society of Engineering Education reported that 18.1% of tenured/tenure-track STEM faculty positions were held by women and 2.5% were held by African Americans (Roy Reference Roy2020). Accounting for leniency interviewer bias and implicit preference bias may shine a light on ways to achieve a more diverse population of engineers (Wulf Reference Wulf1999; Du & Kolmos Reference Du and Kolmos2009; Daly et al. Reference Daly, Seifert, Yilmaz and Gonzalez2016). Biases in the engineering programs not only impact the students but also the faculty (Moss-Racusin et al. Reference Moss-Racusin, Sanzari, Caluori and Rabasco2018). Gender and racial bias, as well as leniency bias toward a specific gender, impact women and racially marginalized engineers, affecting their sense of belonging and readiness for participation (Moss-Racusin et al. Reference Moss-Racusin, Sanzari, Caluori and Rabasco2018). Therefore, it is important to be aware of any biases that occur in the admissions process of engineering design programs, but also any bias that occurs among faculty in those programs.
Another area in education where bias may exist is the curriculum (Dijkstra, Verdonk, & Lagro-Janssen Reference Dijkstra, Verdonk and Lagro-Janssen2008; Schofferman Reference Schofferman2015; Benoit et al. Reference Benoit, Travis, Swan Sein, Quiah, Amiel and Gowda2020). Dijkstra et al. (Reference Dijkstra, Verdonk and Lagro-Janssen2008) recognized that gender bias existed in the field of medicine, and therefore investigated if it was reflected in medical textbooks (Dijkstra et al. Reference Dijkstra, Verdonk and Lagro-Janssen2008). It was found that medical textbooks show gender bias, lacking gender-specific body and social environment information relevant to good medical practice. Textbooks act as resources for learning, but they also act as datasets used to make informed medical decisions and conclusions. In engineering design, bias may exist in the design textbooks used. Textbooks are used to teach engineering design and also act as a resource that provides tools for executing engineering design (Pahl & Beitz Reference Pahl, Beitz and Wallace1996; Otto & Wood Reference Otto and Wood2000; Dieter & Schmidt Reference Dieter and Schmidt2013). A bias in textbooks could lead to biased design practices and a limited perspective when conducting design. A gender bias may exist in engineering design textbooks as well, and this should be assessed in further detail.
In addition to textbooks, Benoit et al. (Reference Benoit, Travis, Swan Sein, Quiah, Amiel and Gowda2020) demonstrated that bias exists in medical training (Benoit et al. Reference Benoit, Travis, Swan Sein, Quiah, Amiel and Gowda2020). When discussing normal and healthy bodies in medical training, white bodies and features were often used which could imply that different bodies are not healthy. For example, in a lecture on gum health, pink gums were provided as an example of healthy gums. However, healthy gums can also be brown or purple in people with darker skin tones. Benoit et al. (Reference Benoit, Travis, Swan Sein, Quiah, Amiel and Gowda2020) sought to provide a framework on how to remove such implicit biases from the curriculum. Though engineering design may not use human bodies as the basis of healthy and unhealthy in the way it is used in the medical field, similar scenarios may occur in the engineering design curriculum when referring to customers or end-users of a design solution. When teaching students to design for customers or intended users, it is important to not bias their approaches by providing example design solutions that reflect certain populations. For example, the curriculum should not only provide example design problems and solutions that reflect traditional engineering space because that may bias designers to focus solely on providing a specific and familiar solution but may not fit well within the intended user’s environment or expectations. This was the case with crash test dummies for car collision safety testing (Gupta Reference Gupta2021). The test dummy represented an average size white male, resulting in safety data that was inaccurate for drivers of different body types, particularly women. This may show up in methods taught to collect information on the end-user, or methods taught to evaluate the final design, making it important to investigate implicit bias present in design methods. Design methods are crucial in guiding the designer in the design process, so understanding the bias present in the methods can highlight if and why bias exists in design outcomes.
Schofferman (Reference Schofferman2015) also demonstrated that bias may exist in medical education with the source of bias being industry-funded medical education curriculums (Schofferman Reference Schofferman2015). Continuing medical education (CME) programs that are financially supported by industries have the potential to subconsciously bias leadership professionals and educators. This bias can be caused or enhanced by marketing strategies used because industry participants funded by CME programs are more likely to use the sponsoring company’s product in training. This can occur at CME programs as well as at CME conferences. Being exposed to specific products in training impacts the participant’s or attendee’s choice of drugs and devices used in practice, potentially affecting patient care. A similar scenario may occur in engineering design education regarding industry-funded engineering design programs, projects or courses. Industry-funded programs, courses and projects often use content and tools provided by the industry funder. The educational institution may have limited control of the project focus, materials taught, and the design tools and equipment used. Though students often seek out these industry-funded programs or projects, this involvement can unintentionally bias the student designers. Pinzur (Reference Pinzur2020) also addressed bias in industry-sponsored educational programs, specifically looking at a specialized society that educates medical professionals: the American Orthopaedic Foot & Ankle Society (AOFAS) (Pinzur Reference Pinzur2020). Pinzur detailed that within AOFAS educational program presentations, presenters avoided highlighting competitors’ products, showing a selection bias in the educational information presented. Before material was presented, there was also selection bias in the papers or presentations accepted, with research being rejected if the committee members disagreed with the treatment advocated, or the device or implant used. Pinzur’s study highlights how different education avenues can be biased as well as unintentionally teach bias.
In engineering design education, this bias may occur at industry-sponsored conferences or educational programs. Stakeholders may limit sharing content that does not align with the industry funder, limiting the shared knowledge within the large engineering design community. Conferences and education programs offered at conferences are often the places in the engineering design community where students, researchers and educators can network. If the places that act as collaborative educational spaces for engineering design are prone to be biased, this may influence existing biases designers have as well as unintentionally limit the expansion of engineering design. It is important to understand that bias exists in industry-sponsored education programs, on both the small and the large scale. As detailed before, in engineering design education, bias has not been explored as extensively as in medical education. Bias studied in medical education highlights what may influence or induce bias in engineering design education as well as the gaps in engineering design education bias research.
5.2.2. Comparing medical practice & engineering design practice
The second domain explored is the practice of medicine or engineering design. The scope of this study covers actions, tasks and events that occur when practicing medicine or executing engineering design. The process of engineering design can be divided into subsequent phases as seen in Figure 1. Using the established phases in Figure 1, bias in design practice was pinpointed. The establishing the need phase is when the designers begin to collect information on the intended user or intended design space to identify the need, problem or scope of the project. In this stage, a lot of information searching, collection and processing occurs, similar to when medical providers take a past medical history or search for information to diagnose a disease. Medical research has identified many biases that may also be found to influence actions and tasks that may show up in the first stage of the design process. In the establishing needs phase, the designer is oftentimes interacting directly with the intended end-user, or indirectly by collecting information from resources on the end-user. Bias research in the field of medicine shows that provider bias influences this similar interaction in medical practice: physician–patient interaction and treatment recommendations (Sieverding et al. Reference Sieverding, Schatzkin, Shen and Liu2018). Sieverding et al. (Reference Sieverding, Schatzkin, Shen and Liu2018) found that fertility care medical providers exhibited a bias toward patients with certain marital statuses, interfering with the approach used to collect patient information and recommended management, and ultimately affecting the quality of care (Sieverding et al. Reference Sieverding, Schatzkin, Shen and Liu2018). This bias may be seen in engineering design as a designer bias toward the intended end-user. Designers may have preconceived notions when collecting information on the intended user directly or indirectly, affecting the interpretation of the information collected as well as their perceived design solution. In addition to designers exhibiting bias because of the end-users’ demographic status, designers may exhibit bias because of the end-users’ race (Laidley et al. Reference Laidley, Domingue, Sinsub, Harris and Conley2019).
Laidley et al. (Reference Laidley, Domingue, Sinsub, Harris and Conley2019) found that providers’ racial bias influences their diagnosis of the patient (Laidley et al. Reference Laidley, Domingue, Sinsub, Harris and Conley2019). Despite patients being siblings or having similar backgrounds, their skin tone was found to be a predictor of hypertension, with darker skin tone patients having a greater likelihood of being diagnosed with hypertension (Laidley et al. Reference Laidley, Domingue, Sinsub, Harris and Conley2019). While the intended users in engineering design efforts usually have a shared aspect that groups them as the target customers, projects with racial differences among target users may shine a light on any racial biases held by designers. For example, designers may be attempting to provide a design solution to end-users of a community or organization with similar racial identities as their community. However, if these designers were attempting to provide the same design solution to a community or organization of end-users with racial identities that were vastly different from their community, would their approaches change? When designers interview the end-users or use techniques to uncover end-users’ needs, there may be a bias that the designer holds that drives how the needs and expectations of the end-user are interpreted, their understanding of the problem and potentially the solution the designer suggests.
In the field of medicine, racial bias refers to reoccurring bias that negatively impacts the provider’s interpretation of the patient’s needs as well as the prescribed care (Hoffman et al. Reference Hoffman, Trawalter, Axt and Oliver2016; Plews-Ogan et al. Reference Plews-Ogan, Bell, Townsend, Canterbury and Wilkes2020a). One very important example is that racial bias exhibited by medical providers has caused these providers to inaccurately assess the patient’s level of pain (Hoffman et al. Reference Hoffman, Trawalter, Axt and Oliver2016). Racial bias plays a role in the interpretation of information as well as the synthesis of this information. In all stages of engineering design, but particularly in the establishing needs phase, racial bias impacts how the designers assess information shared by the intended user or information collected from the intended user and the design space can lead to the development of design solutions that fail. Being knowledgeable and aware of the racial bias by the designer that exists in engineering design can shine a light on how racial bias influences the first stage of the design process, which is a crucial design stage.
Another cognitive bias that can occur in the first stage of the engineering design process is utilization bias. Aberegg et al. (Reference Aberegg, Arkes and Terry2006) identified that physicians demonstrate a bias leading them to forgo new beneficial information because of their need to not abandon their current practices even if their practices are not as beneficial (Aberegg et al. Reference Aberegg, Arkes and Terry2006). Though the design process is iterative, and designers gather new information throughout the design process, a bias like this can be harmful if exhibited in the early stage of gathering information and establishing the needs. In design, this may be expressed as a hindrance to designers when learning new methods to collect information or new approaches to evaluating information, or even when discovering new areas to collect information that can benefit their understanding of the end-user, the needs and project scope. It is important to understand if this bias exists among designers, how it is expressed and what effects it has on a successful design.
Two biases that exist in medical practice that may surface in the analysis of task phase are hindsight bias and weight bias (Persky & Eccleston Reference Persky and Eccleston2010; Arkes Reference Arkes2013). Hindsight bias is the tendency to exaggerate the extent to which a past event could have been predicted (Arkes Reference Arkes2013; Saposnik et al. Reference Saposnik, Redelmeier, Ruff and Tobler2016). Arkes’ (Reference Arkes2013) literature review on the consequences of hindsight bias in medical decision-making found that hindsight bias affects learning and also leads to overconfidence when practicing medicine, which can result in malpractice (Arkes Reference Arkes2013). Medical decision-making and the analysis of task phase have several paralleling tasks. Hindsight bias and overconfidence in the analysis of task phase can lead to the designer’s misinterpretation of the design problem and poor execution of design. Interpersonal biases may also influence medical decision-making. Persky and Eccleston (Reference Persky and Eccleston2010) found that physician recommendations differed based on the weight of the patient, suggesting that physicians demonstrate a weight bias (Persky & Eccleston Reference Persky and Eccleston2010). Though the weight of a patient is assumed to be correlated to a person’s health in the medical field, in design, weight or any visual appearance of the target user may impede the designer’s decision-making. Designers may unconsciously exhibit an interpersonal bias toward their end-users based on the end-user’s appearance or specific features, essentially an appearance or ability bias. This bias may be noted in the questions used to solicit information for the design project as well as in how the designer interprets that information. The bias may also show up when developing a product based on biased data sets such as weight and height data of a population (Nordqvist Reference Nordqvist2022). Weight is an important factor to consider when developing an ergonomic change, but if BMI tables are used as a standard to determine chair limits and constraints based on user weight, it will result in a biased design solution because BMI tables are inherently biased. An interpersonal bias that stems from someone’s appearance can hinder the execution of the analysis of task phase if the designer is not aware that they hold such bias. This bias prevents the designer from understanding the design space or user needs fully.
The last four design phases are the conceptual design phase, embodiment design phase, detailed design phase and implementation design phase. All four phases have different objectives, but each phase shares similar tasks such as ideation, evaluation, selection, documentation, analysis and other cognitive tasks. One bias that occurs in medical design, testing, regulation and product use that may also occur in engineering design is gender bias. Hutchison (Reference Hutchison2019) found that there was a gender bias in the design and testing of hip implants due to the consideration and use of only male bodies and male-centered kinesiology (Hutchison Reference Hutchison2019). The gender-biased hip implant design led to increased implant failures for female patients (Hutchison Reference Hutchison2019). This bias directly relates to engineering design in practice because medical practitioners participated in designing the hip implant. In engineering design, gender bias may unknowingly occur if the metrics used to evaluate, test and demonstrate designs only use male participants. It is important to take into account the different gender demographics of the indented users when designing, to minimize design failure. Gender bias may be seen in the metrics used to evaluate the performance and functions of the design solution. Taking into account any gender biases in the final design stages can ensure the functionality of the final design solution for all the intended users.
In addition to design, testing and implementation, gender bias can also inform the selection of design concepts. Stålnacke et al.’s (Reference Stålnacke, Haukenes, Lehti, Wiklund, Wiklund and Hammarström2015) study identified that males had a significantly higher chance of being prescribed physiotherapy and radiological examination than female patients when presenting with chronic musculoskeletal pain (Stålnacke et al. Reference Stålnacke, Haukenes, Lehti, Wiklund, Wiklund and Hammarström2015). This task is similar to the designer collecting information and selecting the best concept for the intended user or customer. Designers need to be aware of any gender bias or other interpersonal bias that exists when selecting concepts. One way designers can explore if a gender bias exists in this regard is to assess past projects for any trends that may suggest a gender bias.
Another bias that can influence selection or decision-making in the design process is racial bias. Green et al.’s (Reference Green, Carney, Pallin, Ngo, Raymond, Iezzoni and Banaji2007) study on the decision to treat patients found that physicians’ implicit racial bias plays a significant role in the likelihood of not treating black patients (Green et al. Reference Green, Carney, Pallin, Ngo, Raymond, Iezzoni and Banaji2007). This implicit bias may show up in engineering design unknowingly, especially when designing for users of different racial backgrounds which often occurs in humanitarian design or global development (Avgerou Reference Avgerou2010). Engineering design is primarily conducted by white males, so there is a possibility that design thinking and decision-making may be biased when designers are designing with or designing for people of marginalized racial groups.
Medical research suggests confirmation bias may exist in these design stages (Mendel et al. Reference Mendel, Traut-Mattausch, Jonas, Leucht, Kane, Maino, Kissling and Hamann2011). Confirmation bias is the tendency to confirm a favored hypothesis (Tschan et al. Reference Tschan, Semmer, Gurtner, Bizzari, Spychiger, Breuer and Marsch2009; Elston Reference Elston2020; Nelius et al. Reference Nelius, Doellken, Zimmerer and Matthiesen2020). Mendel et al. (Reference Mendel, Traut-Mattausch, Jonas, Leucht, Kane, Maino, Kissling and Hamann2011) found that psychiatrists and medical students exhibited confirmation bias in their information search leading to diagnostic inaccuracies (Mendel et al. Reference Mendel, Traut-Mattausch, Jonas, Leucht, Kane, Maino, Kissling and Hamann2011). This situation in medicine is similar to the establishing the needs phase as well as the conception selection phase in engineering design. Confirmation bias in a designer may impact the information the designer searches trickling down to the methods used to develop and implement the design solutions. Confirmation bias may also influence the designer’s ability to change their selection, decision or understanding, all being important actions throughout the design process.
In the final design phase, the implementation phase, the final design solution is transferred to the intended user by producing the product to scale, commercialization and mass production if needed. Documentation associated with the final design is also developed as a supplemental resource for users as well as other designers. Phillips, Wassersug, & McLeod (Reference Phillips, Wassersug and McLeod2012) study identified that documentation bias in supplemental pharmaceutical literature was linked to patients being less informed on drug side effects (Phillips et al. Reference Phillips, Wassersug and McLeod2012). Some of the drivers for this documentation bias were due to the funding sources for the work. Documentation bias can unknowingly occur in engineering design as funding sources or stakeholders may unintentionally limit the information included in the final documentation. This bias can systematically influence what information is conveyed, available or made explicit to the end-user and other designers. Potential conflicts of interest may exist and unknowingly bias the content in the resulting documentation or supporting literature.
Though the design process can be divided into six different phases, most tasks are not exclusive to a single design phase. For example, decision-making, selection and even interviewing can occur in multiple phases of the design process. Biases that occur during medical decision-making are biases that can occur in all phases of the engineering design process. Hershberger et al. (Reference Hershberger, Markert, Part, Cohen and Finger1996) investigated cognitive bias in medical decision-making and found that both physicians and medical students demonstrate cognitive bias, but physicians were less susceptible (Hershberger et al. Reference Hershberger, Markert, Part, Cohen and Finger1996). Student and professional designers may also exhibit cognitive bias in design decision-making. Decision-making tasks in design include selection, synthesis, evaluation, production, specification and many additional tasks that require design thinking. It is important to understand if cognitive bias plays a role in these tasks as it does in the field of medicine. It is also important to understand the variance of cognitive bias based on experience level or other designer traits.
Elston (Reference Elston2020) provided a review of cognitive bias, specifically confirmation bias, in medical decision-making (Elston Reference Elston2020). Confirmation bias in the medical context is the tendency to give greater weight to data that supports a preliminary diagnosis while neglecting contradictory evidence (Elston Reference Elston2020). This bias may occur in all stages of the design process. When collecting project or end-user information in engineering design, a confirmation bias may impede the designer’s judgment if they are refusing to collect any information that may not support their findings. This may also occur with concept selection or even evaluation and synthesis of new information regarding conceptual design. The designer may disregard any information that suggests that the selected concept is not the best solution or fail to even consider such information due to a confirmation bias. Age plays a role in exhibiting confirmation bias, with older individuals being more likely to exhibit this bias. It is important to understand where confirmation bias is seen in design, its impacts, as well as the variance among designers based on experience as well as age, gender and race.
Saposnik et al.’s (Reference Saposnik, Redelmeier, Ruff and Tobler2016) systematic review of the cognitive bias research in medical decision-making highlighted common cognitive biases that occur during management, treatment, diagnosis and/or prognosis (Saposnik et al. Reference Saposnik, Redelmeier, Ruff and Tobler2016). These biases include anchoring, availability bias, blind obedience, commission bias, confirmation bias, diagnostic bias/premature closing, framing effect, omission bias, overconfidence, tolerance to risk and satisfying bias (Saposnik et al. Reference Saposnik, Redelmeier, Ruff and Tobler2016). Though these biases occur and were studied in medical scenarios, they may also occur in engineering design scenarios. Management refers to how tasks within the scope are handled and regarded and is directly comparable to the management of tasks throughout the engineering design process. How is information handled? How is work within design teams distributed and executed? All these are ways where bias in the management of the design process can come into play. Management can be influenced by outcome bias, framing effect, anchoring or confirmation bias. It is important to understand this concept in the management of and within design projects. Medical decision-making, such as treatment and diagnosis, can be extrapolated to various design stages. Diagnosis is the interpretation of information to determine the presence or absence and quality of a disease in a patient. In engineering design, this can be compared to data interpretation from the end-user, in the early stages or prototype testing results and feedback from the final design stages. Overconfidence, outcome bias, anchoring or confirmation bias, and information bias have all been described in medical diagnosis, and may potentially occur in similar engineering design cognitive tasks. Treatment is the action that follows diagnosis. The emphasis is how the medical professional decides to provide a solution for their diagnosis. This may mimic the engineering design route the designer selects to use in the early design stages or even the approach used to produce and implement the final design. Framing effect, feedback bias and outcome biases can impact treatment in medical decision-making, so it likely impacts similar tasks in engineering design. Prognosis is the medical decision-making task of predicting the likelihood of a certain outcome of a disease or ailment. Though not as evident, prognosis is comparable to designers predicting the success or feasibility of a concept based on knowledge or with the use of tools to aid design. Simply, prognosis is the action of predicting an outcome. Though there are many design tools to assist in the design process and provide indicators that help make decisions, often design progresses to the next stages because of a designer’s prediction of a solution or concept’s success. If not successful, the designers investigate the problem and implement changes accordingly. The iterative nature of the design process partially relies on the prediction of the designers. Though tools and external resources have been developed to mitigate the human error of prediction, it is important to understand how cognitive bias in prediction shows up in engineering design.
Biases influencing decision-making not only are exhibited by individuals but can also be demonstrated by teams (Tschan et al. Reference Tschan, Semmer, Gurtner, Bizzari, Spychiger, Breuer and Marsch2009; Helzer et al. Reference Helzer, Myers, Fahim, Sutcliffe and Abernathy2020). Tschan et al.’s (Reference Tschan, Semmer, Gurtner, Bizzari, Spychiger, Breuer and Marsch2009) study suggests that confirmation bias exists in collaborative diagnosis and explicit reasoning within medical teams occurred more in groups that made the correct diagnosis (Tschan et al. Reference Tschan, Semmer, Gurtner, Bizzari, Spychiger, Breuer and Marsch2009). Collaborative diagnosis in medicine parallels team ideation and concept selection in engineering design. Confirmation bias may exist in collaborative ideation or concept selection if design teams are not explicitly stating their reasoning, potentially leading to design solutions that fail. Bias in collaborative teams can also stem from team members’ gender bias. Helzer et al.’s (Reference Helzer, Myers, Fahim, Sutcliffe and Abernathy2020) study highlighted that participants relied more on treatment advice delivered by a male physician versus a female physician (Helzer et al. Reference Helzer, Myers, Fahim, Sutcliffe and Abernathy2020). It was also demonstrated that participants’ reliance on the advice from female physicians increased based on the physician’s experience, and this was not the case for male physicians (Helzer et al. Reference Helzer, Myers, Fahim, Sutcliffe and Abernathy2020). This gender bias may occur when team members contribute information and even when instructors or managers offer advice to design teams. Designers may have a bias that prevents them from accepting helpful information due to the gender of the person providing the information. It may also be worth investigating if the experience of the person providing information influences how designers accept and use the information.
Research on bias in the medical field shows that medical providers can demonstrate bias, but some work also suggests the patient can exhibit bias. Levy and Hershey (Reference Levy and Hershey2008) study explored patient bias and found that in close-call medical decisions, patients may exhibit value-induced bias (Levy & Hershey Reference Levy and Hershey2008). Value-induced bias is when someone distorts relevant probabilities to justify decisions, ultimately wishful thinking (Levy & Hershey Reference Levy and Hershey2008). Though in engineering design, most projects are not risky close calls like in some medical scenarios, end-users may exhibit this bias when tasked with decision-making when interpreting information conveyed by the stakeholder. The motivation to justify one’s decision and preference may lead people to distort their perception of relevant possibilities. Levy’s findings suggest that it is important to investigate the bias not only among the designers but also among the end-users. Research on bias in the practice of medicine highlights potential biases when executing engineering design that has not been investigated.
Bias research in engineering design has primarily explored biases that impact the practice or execution of design (Stacey et al. Reference Stacey, Petre, Rzevski, Sharp and Buckland1996; Toh et al. Reference Toh, Strohmetz and Miller2016; Nelius et al. Reference Nelius, Doellken, Zimmerer and Matthiesen2020). Stacey et al. (Reference Stacey, Petre, Rzevski, Sharp and Buckland1996) explored design tool bias in concept selection with the implementation of a design tool that mitigates and utilizes design tool bias to aid in concept selection (Stacey et al. Reference Stacey, Petre, Rzevski, Sharp and Buckland1996). This study highlights that design tools can be biased but that also bias can be beneficial to design outcomes. Toh et al. (Reference Toh, Strohmetz and Miller2016) explored the variance of ownership bias in concept selection based on the designer’s gender (Toh et al. Reference Toh, Strohmetz and Miller2016). Though gender bias was not explored, Toh found that male designers are more likely to demonstrate ownership bias than female designers (Toh et al. Reference Toh, Strohmetz and Miller2016).
5.2.3. Comparing medical research & engineering design research
One final domain of engineering design where bias may show up is in the process of engineering design research. Many aspects of engineering design are investigated in engineering design research such as designer protocols, effects of design solutions, tools that aid design, patterns in the design process, creativity and ideation, design thinking, and even documentation during the design process(Cardella, Atman, & Adams Reference Cardella, Atman and Adams2006; Maier & Fadel Reference Maier and Fadel2009; Caldwell Reference Caldwell2011; Linsey et al. Reference Linsey, Clauss, Kurtoglu, Murphy, Wood and Markman2011; Van Bossuyt & Dean Reference Van Bossuyt and Dean2016; Kannengiesser & Gero Reference Kannengiesser and Gero2017). As previously mentioned, Hutchison (Reference Hutchison2019) identified a gender bias in the design, testing, regulation and use of hip replacement (Hutchison Reference Hutchison2019). Though this is considered a bias in design practice, it also highlights a bias that may exist in engineering design research because of the research that goes into product design. Research of design solutions consists of the development, testing and implementation of design solutions (Paleta, Pina, & Santos Silva Reference Paleta, Pina and Santos Silva2014; Pinto et al. Reference Pinto, Wong, Fennesy, Tang and Compere2016). If a gender bias exists within these stages, developing and testing with a specific user in mind can lead to failed design solutions such as the hip replacement in Hutchison’s case. This lack of inclusion highlights another bias that can show up in design research: participation bias.
Participation bias is when the participants of clinical trials fail to reflect the demographics of the patient populations (Murthy, Krumholz, & Gross Reference Murthy, Krumholz and Gross2004). Murthy et al. (Reference Murthy, Krumholz and Gross2004) found that elderly and racially and gender-minoritized groups were less likely to enroll in cooperative group cancer trials than white participants, men and younger patients (Murthy et al. Reference Murthy, Krumholz and Gross2004). Though clinical trial studies differ from engineering design studies, a participation bias can be a factor in design research. Many engineering design studies are conducted at the institutional level, using undergraduate or graduate-level engineers as participants in those studies. The demographics of students enrolled in these engineering departments need to be considered to understand if a participation bias occurs. Most students enrolled in these engineering departments are white, male students, thus enabling the researcher to only collect data on design thinking that reflects the perspectives of younger white males (Roy Reference Roy2019, Reference Roy2020). It is important to understand how participation in clinical studies plays a role in biasing the data we used to understand design. Race and age-based disparities in medical research participation may be the result of social and economic disparities or other systematic factors such as racism; however, one’s race is not a health risk factor. In design, particularly design research, race and sex disparities may influence design outcomes as these factors may influence decision-making in the design context (Helzer et al. Reference Helzer, Myers, Fahim, Sutcliffe and Abernathy2020).
Another research bias that occurs in the medical field is reporting bias. Reporting bias refers to any bias that impedes what and how research findings are reported and published (McGauran et al. Reference McGauran, Wieseler, Kreis, Schüler, Kölsch and Kaiser2010). Some examples of reporting bias include publication bias, time-lag bias, multiple publication bias, location bias, citation bias, language bias and outcome reporting bias (McGauran et al. Reference McGauran, Wieseler, Kreis, Schüler, Kölsch and Kaiser2010). McGauran et al.’s (Reference McGauran, Wieseler, Kreis, Schüler, Kölsch and Kaiser2010) review of reporting bias in medical research identified that many manufacturers and regulatory agencies withheld study data or attempted to suppress publications (McGauran et al. Reference McGauran, Wieseler, Kreis, Schüler, Kölsch and Kaiser2010). McGauran also found that reporting bias oftentimes revolved around the overestimation of efficacy and the underestimation of safety risks of interventions (McGauran et al. Reference McGauran, Wieseler, Kreis, Schüler, Kölsch and Kaiser2010). This study highlights how the nature and direction of the results can bias how the findings are reported. Reporting bias may occur in design research if the results go in a certain direction. Oftentimes, failed design efforts are not reported, which highlights a reporting bias in unsupportive findings. It may be beneficial to highlight how often studies go unreported because of the nature of the results. In addition to no reporting based on the nature of the results, reporting bias may also inform how research is documented. Researchers may oversell findings or withhold findings to achieve publication. This is an important phenomenon because reporting and publishing research findings are the primary way research is shared within the design community. Publishing is important for designers to understand what research is being conducted in the field but is also important for the expansion of engineering design as a field.
Delgado-Rodríguez and Llorca ( Reference Delgado-Rodríguez and Llorca 2004 ) reviewed the bias that impacts research in the medical field, identifying an extensive list of biases that influence medical research (Delgado-Rodríguez & Llorca Reference Delgado-Rodríguez and Llorca2004). These biases and the biases mentioned previously can be explored in engineering design by investigating the presence in the research pipeline. For the context of this study, the research pipeline refers to the funding, stakeholders, researchers, participants, data sets, tools, conferences, publications and other entities involved. Exploring these biases in these spaces will begin to close the gap in bias research in engineering design research.
6. Conclusion
Although the fields of medicine and engineering design have numerous similarities, the understanding of the role of bias in engineering design tasks and events is not as well understood. Bias research in the medical field highlights many potential opportunities for parallel bias research in engineering design. Bias may occur during end-user interviews, ideation, brainstorming, concept selection, decision-making or admissions, and even in funded conferences and educational courses. A summary of the comparison of actions and events where bias has been investigated in the fields of medicine and engineering design is depicted in Figure 4.

Figure 4. Comparison of actions, areas and events investigated for bias in the fields of engineering design and medicine.
Using the context identified for bias in the medical field creates avenues for bias research in engineering design. In addition to identifying potential bias and engineering design, findings also identified potential sources for bias in engineering design to be investigated. It is important to understand where and what bias exists in engineering design, but it is also important to understand the potential causes for bias in engineering design. Listed below are the potential sources of bias based on the findings from the review of bias in the medical field.
6.1. Potential sources for bias in engineering design education
In the scope of this review, engineering design education encompasses the tasks involved to receive and provide engineering design education. This includes events such as the admission process into an engineering program that teaches design as well as events involved directly in engineering design instruction. Based on bias known in medical education, there are many scenarios in engineering design education where bias is having a potential impact. Starting with the admissions process, bias may occur during interviews for admission into engineering programs. In addition to the admissions process, bias may also influence the material and resources used in engineering design education, including textbooks and course curriculums. The content in the materials may contain racial and gender bias as seen in the example stated previously with medical textbooks (Benoit et al. Reference Benoit, Travis, Swan Sein, Quiah, Amiel and Gowda2020). White bodies and features were often used when discussing normal and healthy bodies in medical training unknowingly biasing medical students when they practice on patients that were not white. In addition to the content of course materials, the selection of those materials may be impacted by funding bias and/or information bias. The latter may also stem from educational partnerships, which have some benefits but still bias the curriculum. It is therefore important to take note of the potential impact of educational partnerships that fund the curriculum and/or filter the curriculum provided. The potential sources of bias in engineering design education may therefore be summarized as follows:
-
• Leniency bias , racial bias and gender bias may impact admission into engineering programs that teach design programs and engineering design programs.
-
• Racial and gender bias may show up in engineering design textbooks and course materials. Based on findings highlighting the presence of bias in medical textbooks.
-
• Funding and information bias may show up in the curriculum used in engineering design education programs and be a result of educational funding and partnerships.
6.2. Potential sources for bias in engineering design in practice
Engineering design is in practice a variety of processes and tasks, including but not limited to understanding the user’s needs, collecting information and transferring that information into the development of design solutions that meet the user’s needs. Bias may be introduced in each of these stages. For instance, during customer interviews, designers may exhibit racial bias, leniency bias, weight bias and appearance bias. Bias can also influence cognitive tasks in engineering design such as collaborative decision-making. Cognitive biases may also influence the surveys and questionnaires developed by designers to collect user information. In addition to the tools used to collect user data, the data sets themselves may be biased as well, exuding racial, gender and information bias. Similar to bias in engineering design education, funding bias, information bias and outcome bias may stem from the influence of project funding and stakeholders. The potential sources of bias in engineering design practice may therefore be summarized as follows:
-
• Racial bias , leniency bias and appearance bias may potentially influence the collection of user needs, particularly in customer interviews.
-
• Cognitive bias and interpersonal bias may show up during decision-making.
-
• Cognitive bias may influence survey and questionnaire development for customer data.
-
• Racial bias and gender bias may have biased the data available in data sets if the tools used to collect the data and the designers are exhibiting this bias.
-
• Information bias , outcome bias and funding bias may stem from project stakeholders, funders and outlets for research.
6.3. Potential sources for bias engineering design research
Research in engineering design involves a range of tasks used to study all aspects and parts that make up engineering design. Like the biases that may occur in engineering design education and in practice, bias in design research may stem from both the design researchers and the stakeholders. Information bias, outcome bias and funding bias may also play a role in engineering design research and outcomes. Engineering design research can be viewed almost as a pipeline, from research funding and participation to research publications. Research on bias in medical research suggests there may be bias in engineering design research due to the similar contextual factors between engineering design research and medical research. The potential sources of bias in engineering design research may therefore be summarized as follows:
-
• Cognitive biases and interpersonal biases among researchers may lead to biased research.
-
• Biased data sets can influence the research outcomes.
-
• Information bias can result from limitations and representations at conferences can bias the work in the field of engineering design. Also limiting the methods and tools used in engineering design research.
-
• Publication bias from publication entities may influence what information can and cannot be published.
-
• Participation bias caused by a lack of diversity in the research participant population can bias research outcomes. A lack of diverse target end-users or customers can also bias the protocols that are established in engineering design.
7. Next steps for bias in engineering design
It is important to explore the various sources of bias to begin to understand and mitigate it. Research in the medical field has extensively identified sources of bias as well as methods for studying bias, including both qualitative and quantitative modalities. In this work, we turned to the field of medicine as an analog for engineering design, and through this comparison identified potential sources of bias in engineering design itself. Identifying the bias in engineering design can help understand the impact of bias on design education, projects and research. It is only through understanding these sources and influences that future work can begin to explore strategies for mitigation.
The three domains studied in this work – education, research and practice – act as three future research areas for bias in engineering design. In large part, this work has introduced hypotheses for where bias may occur in engineering design. Therefore, future work needs to investigate if the identified biases truly exist in the identified scenarios. In addition to the scenarios identified here, researchers should also investigate other scenarios in engineering design that may not have explicit parallels in medicine but are still crucial to engineering design. To do so, future work should begin investigating the aspects of engineering design that are known as vital for effective and successful engineering design. For example, bias is known to influence interpersonal relationships in medicine and interpersonal relationships are crucial to executing engineering design. Future work can explore if and how bias affects interpersonal relationships between designers and end-users or within design teams. Effective characteristics in education, in research and when practicing design can shine a light on areas to investigate the influence of bias. Future work should identify not only the bias that exists but also ways to mitigate or utilize the bias for the benefit of the design and the intended users. This can be done by exploring existing debiasing approaches in and out of the field of engineering design as well as the opportunities to expand the debiasing approaches. An understanding of bias that exists and ways to mitigate bias enables designers to understand bias and its impacts holistically.
Appendix
Table 2. Publication details and data (Aberegg et al. Reference Aberegg, Arkes and Terry2006; Arkes Reference Arkes2013; Benoit et al. Reference Benoit, Travis, Swan Sein, Quiah, Amiel and Gowda2020; Capers et al. Reference Capers, Clinchot, McDougle and Greenwald2017; Dijkstra et al. Reference Dijkstra, Verdonk and Lagro-Janssen2008; Elston Reference Elston2020; Green et al. Reference Green, Carney, Pallin, Ngo, Raymond, Iezzoni and Banaji2007; Griffin & Wilson Reference Griffin and Wilson2010; Hall et al. Reference Hall, Chapman, Lee, Merino, Thomas, Payne, Eng, Day and Coyne-Beasley2015; Helzer et al. Reference Helzer, Myers, Fahim, Sutcliffe and Abernathy2020; Hershberger et al. Reference Hershberger, Markert, Part, Cohen and Finger1996; Hoffman et al. Reference Hoffman, Trawalter, Axt and Oliver2016; Hutchison Reference Hutchison2019; Laidley et al. Reference Laidley, Domingue, Sinsub, Harris and Conley2019; Levy & Hershey Reference Levy and Hershey2008; McGauran et al. Reference McGauran, Wieseler, Kreis, Schüler, Kölsch and Kaiser2010; Mendel et al. Reference Mendel, Traut-Mattausch, Jonas, Leucht, Kane, Maino, Kissling and Hamann2011; Murthy et al. Reference Murthy, Krumholz and Gross2004; Persky & Eccleston Reference Persky and Eccleston2010; Phillips et al. Reference Phillips, Wassersug and McLeod2012; Pinzur Reference Pinzur2020; Plews-Ogan et al. Reference Plews-Ogan, Bell, Townsend, Canterbury and Wilkes2020b, Reference Plews-Ogan, Bell, Townsend, Canterbury and Wilkes2020a; Saposnik et al. Reference Saposnik, Redelmeier, Ruff and Tobler2016; Schofferman Reference Schofferman2015; Sieverding et al. Reference Sieverding, Schatzkin, Shen and Liu2018; Stålnacke et al. Reference Stålnacke, Haukenes, Lehti, Wiklund, Wiklund and Hammarström2015; Stone & Moskowitz Reference Stone and Moskowitz2011; Tripepi et al. Reference Tripepi, Jager, Dekker and Zoccali2010; Tschan et al. Reference Tschan, Semmer, Gurtner, Bizzari, Spychiger, Breuer and Marsch2009).
