LEARNING OBJECTIVES
After reading this article you will be able to:
• identify the neural networks involved in substance dependence and relapse
• appreciate how neuroimaging continues to characterise different aspects of substance dependence and treatment
• recognise how individualised therapy has potential to shape the future of treatment in substance dependence.
Addiction is a global health problem that places a huge burden on individuals and society. In the UK, it is estimated that addictions consume £15 billion of government resources yearly, and on average £4 billion of the annual National Health Service (NHS) budget is spent on alcohol and drug misuse. National Drug Treatment and Monitoring Statistics report that, for the year 2017 to 2018, approximately 270 000 adults were in contact with drug and alcohol services (Knight Reference Knight, Brand and van der Merwe2018). This is likely to be an underrepresentation of individuals who would benefit from service intervention, but nevertheless it reflects how a relatively small section of the population demand a great deal of resources and support.
Clinically, the chronically relapsing nature of addiction is a core problem, with few treatment options in abstinence maintenance. Relapse rates are high; despite usual treatment, over 20% of individuals will relapse within 1 month and a further 40% within 6 months. Longitudinal data suggest that at least 5 years of abstinence are required before recovery is well established, after which only a minority will relapse (White Reference White2012). Historically this has been a hugely neglected area of study and one with significant room for improvement and development, and the ability to predict relapse and the development of novel treatments continue to be major goals in addiction research. Understanding the neurobiological mechanisms underlying relapse will help us to identify therapeutic targets to reduce this risk, providing tools that we can use to achieve these goals.
Theories of addiction
Drug addiction has been conceptualised as a disorder that affects neural networks centred on reward, inhibitory control, stress, emotional processing and learning/memory. Interference with these networks supports the theoretical framework for the three-stage addiction cycle that promotes drug-seeking behaviour in the addicted state (Koob Reference Koob and Le Moal2008). There are also several eminent addiction theories: incentive salience (Robinson Reference Robinson and Berridge1993, Reference Robinson and Berridge2008), reward deficiency syndrome (Blum Reference Blum, Braverman and Holder2000, Reference Blum, Thanos and Gold2014), the allostatic hypothesis (Koob Reference Koob and Le Moal2005), habit formation theory (the shift from impulsive to compulsive choice) (Everitt Reference Everitt and Robbins2005, Reference Everitt and Robbins2016) and impaired response inhibition and salience attribution (Goldstein Reference Goldstein and Volkow2002).
There is overlap between all models with regard to impairments in reward processing and ‘hijacking’ of reward circuitry by drugs of misuse, such that the motivation to procure drugs overpowers the drive for non-drug-related goals. Koob's model describing the role of various brain regions in substance use and addiction is useful to understand which parts of the brain are involved in binge/intoxication, withdrawal/negative affect and preoccupation/anticipation (Fig. 1) (Koob Reference Koob and Volkow2016). For instance, the reward system in the striatum is key during binge/intoxication, with dysregulation in the amygdala mediating the low mood and emotional stress seen in withdrawal. The prefrontal cortex (PFC) is responsible for inhibitory control and a key modulator of striatal activity, i.e. reward processes. Impairments in this region manifest as impulsivity and contribute to the preoccupation/anticipation phase. In the early stages of drug misuse, positive reinforcement of drug consumption concurrent with impulsivity drives this cycle. Once the transition to substance dependence is more robust, drug-taking becomes more compulsive and negative reinforcement becomes the driver of drug-seeking and consumption (Koob Reference Koob and Volkow2010; Goldstein Reference Goldstein and Volkow2011).
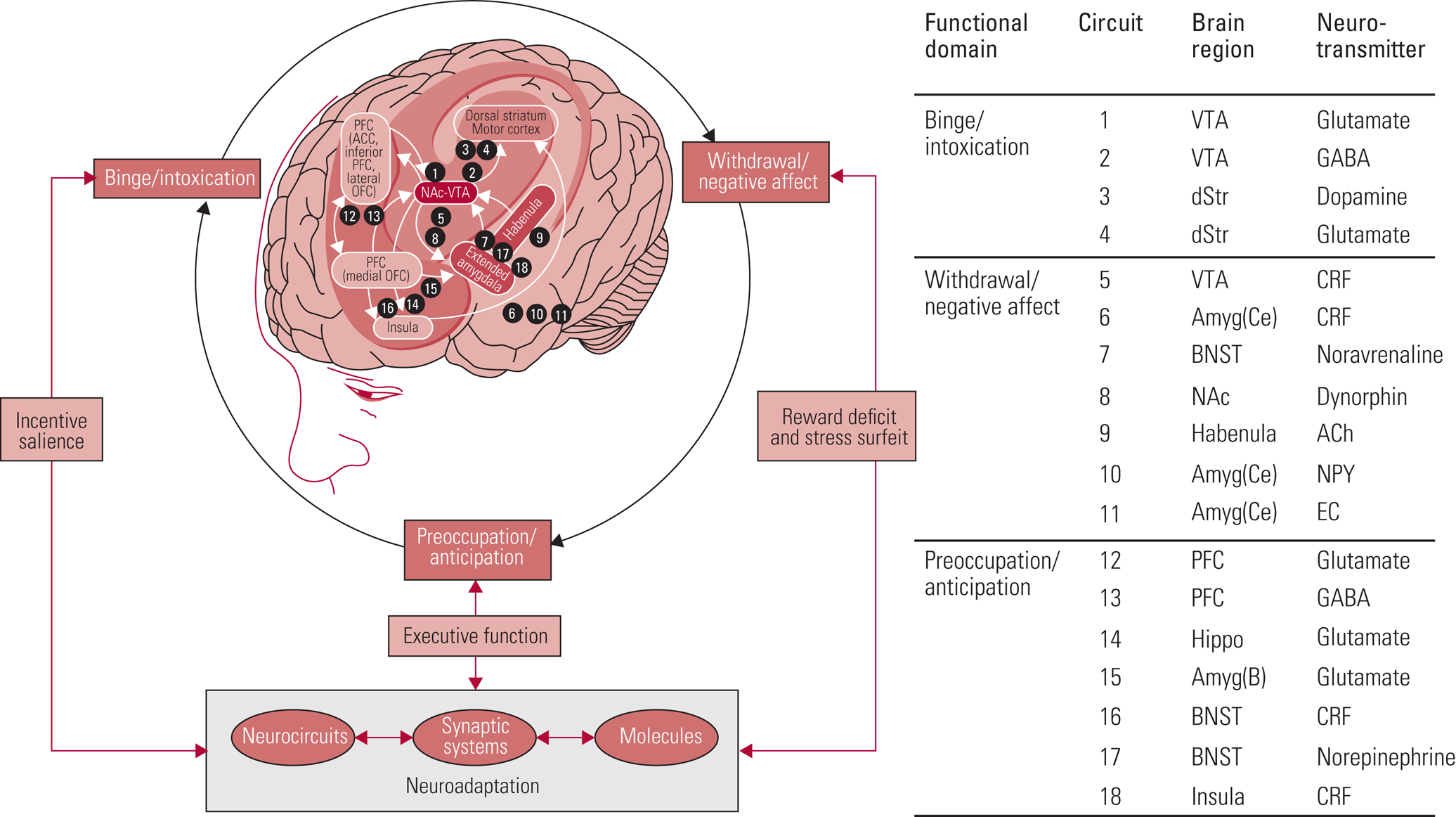
FIG 1 Brain regions involved in substance use and addiction (Koob Reference Koob and Volkow2016). PFC, prefrontal cortex; ACC, anterior cingulate cortex; OFC, orbitofrontal cortex; NAc, nucleus accumbens; VTA, ventral tegmental area; GABA, γ-aminobutyric acid; dStr, dorsal striatum; Amyg(ce), central nucleus of the amygdala; CRF, corticotropin-releasing factor; BNST, bed nucleus of the stria terminalis; ACh, acetylcholine; NPY, neuropeptide Y; EC, endocannabinoids; Hippo, hippocampus; Amyg(B), basolateral amygdala.
In the past few decades, neuroimaging has transformed our understanding of the neurobiology of addiction, the impact of substances, and vulnerability and resilience factors. The focus has been on improving treatments and clinical outcomes by predicting treatment response – which enables targeted individualised therapy – and improving early diagnosis and interventions. Definitions and clinical criteria that describe and classify addictive disorders have also been changing, and we begin our discussion with an overview of these.
Definitions: addiction versus dependence
Research in psychiatry generally classifies individuals on the basis of their diagnosis using one of the two main classification systems: the DSM, now in its 5th edition (DSM-5) (American Psychiatric Association 2013) is commonly used in research; the ICD is widely used clinically and is currently being released in its 11th revision (ICD-11) (World Health Organisation 2018). Although concordance between DSM-IV, ICD-10 and ICD-11 was high, this is not necessarily the case with DSM-5, particularly when diagnosing harmful or mild dependence, resulting in implications for interpreting and comparing studies using these different systems and versions (Saunders Reference Saunders, Degenhardt and Reed2019). DSM-5 considers substance use disorder as a continuum rather than separating misuse and dependence, whereas ICD-11 retains ICD-10 categories of ‘harmful use’ and ‘dependence’. It must be remembered that in pharmacological terms, dependence also refers to the neuroadaptations that underlie tolerance and withdrawal (e.g. in relation to prescribed opioids or benzodiazepines) but does not necessarily mean ‘addiction’, which is a complex behaviour involving drug-seeking and craving. Clinically this distinction must be clarified to inform appropriate management.
Functional neuroimaging in addictions
Much of what we understand of the neurobiology of addiction has been informed through research in animal models. Animals, particularly rodents, have been shown to repeatedly lever-press for drug administration, establishing that drugs of misuse act as reinforcers (for a review see Sanchis-Segura Reference Sanchis-Segura and Spanagel2006). Subsequent translational models of misuse liability, drug-taking, escalation and relapse have been developed using self-administration, conditioned place preference, drug discrimination and drug reinstatement paradigms. Such research has allowed us to identify structures and pathways that contribute to the neuropathology of addiction (Lynch Reference Lynch, Nicholson and Dance2010). However, there are limitations to animal models, such as modelling vulnerability factors and complex addictive behaviours. In an attempt to accelerate the development of drugs into the clinic, more translational approaches, such as functional magnetic resonance imaging (fMRI), have been utilised to better understand the relationship between brain mechanisms and behaviour in the human brain.
Functional neuroimaging techniques such as positron emission tomography (PET) and fMRI have been widely applied to relate biochemistry, neural substrates and networks to behaviour in humans. PET measures gamma rays emitted by radioactivity attached to a compound that binds to a known target, for example a neurotransmitter receptor or protein involved in inflammatory processes or glucose metabolism. PET is the only imaging modality to directly assess neurotransmitters such as dopamine. fMRI measures brain activity via the blood oxygenation level-dependent (BOLD) signal, providing an indirect measure of brain activity that can be examined at rest (i.e. resting-state fMRI) or in response to neurocognitive tasks. The more recently developed MRI-based techniques of magnetic resonance spectroscopy (MRS) and diffusion tensor imaging (DTI) are being applied to study addiction. MRS enables us to characterise cellular constituents such as N-acetyl aspartate or choline, as well as gamma-aminobutyric acid (GABA) and glutamate (although it reflects primarily metabolic rather than small neurotransmitter pools), and DTI reveals the function of white matter tracts.
More recently, fMRI research has focused on identifying neural ‘biomarkers’ of substance dependence, which would help clarify how and under what circumstances treatments are effective, allowing for more individualised and effective treatment of substance dependence (Moeller Reference Moeller and Paulus2018). Owing to the importance of reward processing in the manifestation of substance dependence, many fMRI paradigms have focused on delineating the neural correlates of drug and non-drug reward processing, using tasks such as cue reactivity. Nevertheless, it is important not to overlook that addiction also involves dysregulation in inhibitory control, stress and emotional processing.
Reward
The dopaminergic mesocorticolimbic pathway is commonly associated with reward and projects from the ventral tegmental area (VTA) to the striatum and PFC (Table 1). Conventional rewards such as food and sex activate this pathway to create pleasurable feelings and reinforcement of the reward. Hypofunction of this pathway underpins the ‘reward deficiency’ hypothesis of vulnerability to and perpetuation of addiction.
TABLE 1 Brain regions and their involvement in reward processing

As drug use develops into regular use and possible dependence, the dopaminergic mesocorticolimbic system becomes dysregulated. Drugs of misuse are able to ‘hijack’ the reward system by increasing dopamine in the striatum, particularly limbic or ventral striatum, more so than conventional rewards (Koob Reference Koob2013) (Table 2). For example, stimulants such as cocaine and amphetamines directly increase extracellular dopamine by blocking the presynaptic dopamine transporter (DAT), and amphetamines also increase the release rate from synaptic vesicles. In contrast, drugs such as opiates, alcohol, cannabis and nicotine indirectly increase extracellular dopamine by inhibiting GABA interneurons, which in turn increase VTA dopaminergic firing. A range of inhibitory receptors modulate inhibitory GABAergic interneurons, including mu opioid, nicotinic and cannabinoid CB1 receptors. It is important to remember, however, that, owing to the sensitivity limits of PET, findings on increases in dopamine from some substances of misuse as assessed using PET are limited and less robust (Reid Reference Reid and Lingford-Hughes2006; Nutt Reference Nutt, Lingford-Hughes and Erritzoe2015). Likewise, despite the prominent role of the dopaminergic system in addiction, neither dopaminergic agonists nor antagonists have been found to be clinically efficacious.
TABLE 2 Primary targets, acute and long-term effects of drugs of misuse (adapted from Lingford-Hughes Reference Lingford-Hughes, Watson and Kalk2010)

DAT, dopamine transporter; MAO, monoamine oxidase; Ach, acetylcholine; VTA, ventral tegmental area; GABA, γ-aminobutyric acid; NMDA, N-methyl-d-aspartate; GHB, γ-hydroxybutyric acid; CB, cannabinoid.
Aside from dopamine, other systems have also been implicated in addiction. For example, the endogenous endorphin system plays an important role in reward. PET imaging has shown that pleasure from alcohol or stimulants is associated with increased endogenous endorphin levels and stimulation of the mu opioid receptor (Colasanti Reference Colasanti, Searle and Long2012; Mitchell Reference Mitchell, O'Neil and Janabi2012; Turton Reference Turton, Myers and Mick2020). Through such mechanisms the cues that are associated with the drug become conditioned stimuli, gaining salience. Combined with a loss of top-down control, the motivational drive to seek and consume the drug of misuse becomes more intense, reinforcing drug-taking behaviour and craving (Beck Reference Beck, Schlagenhauf and Wüstenberg2009; Koob Reference Koob and Volkow2016).
Monetary incentive delay
The monetary incentive delay (MID) task is a validated task that involves periods of anticipated monetary loss, gain or a neutral outcome. fMRI measures the BOLD signal during both anticipation and outcome of monetary reward to enable examination of neural correlates of probabilistic non-drug reward and associated learning.
In a neuroimaging meta-analysis of the MID task, the BOLD response to reward anticipation showed significant activation clusters in striatal–thalamic regions of the brain (Oldham Reference Oldham, Murawski and Fornito2018). A meta-analysis comparing the BOLD response in substance-dependent populations and controls confirmed blunted striatal activation during reward anticipation tasks in substance and gambling addictions (Luijten Reference Luijten, Schellekens and Kühn2017). Interestingly, Büchel et al (Reference Büchel, Peters and Banaschewski2017) found that blunted response to anticipated monetary reward in the ventral striatum and dorsolateral PFC in novelty-seeking young teenagers was predictive of problematic drug use at age 16. This suggests a mechanism for predisposition that could be identified prior to exposure to drugs. Finally, reduced striatal activation in response to monetary reward anticipation has been shown to be predictive of relapse in individuals with cocaine dependence; this blunted response has been shown to ‘normalise’ with increasing time spent abstinent (Balodis Reference Balodis, Kober and Worhunsky2016). Together, these findings identify blunting of the reward pathway as a potential biomarker both for vulnerability to substance misuse and risk of relapse.
Cue reactivity
Although substance-dependent individuals demonstrate a hyporesponse to conventional rewards, studies have also shown hyperactivation in their neural response to drug-related stimuli, which as described gain salience during regular drug consumption.
Neuroimaging studies using cue-reactivity tasks typically demonstrate involvement of a range of brain regions encompassing reward/motivation and learning/memory-related circuitry. Meta-analyses of cue-reactivity tasks in fMRI and PET studies suggest that similar patterns of neural activation are seen across alcohol, nicotine and cocaine dependence, with increased BOLD responses observed in the ventral striatum, anterior cingulate cortex (ACC) and amygdala compared with healthy controls (Chase Reference Chase, Eickhoff and Laird2011; Kühn Reference Kühn and Gallinat2011). Greater responses to salient drug stimuli in the dorsal PFC, dorsal striatum, thalamus, ACC and posterior cingulate cortex (PCC) were associated with an increased risk of relapse across alcohol, nicotine, cocaine and opioid dependence (Courtney Reference Courtney, Schacht and Hutchison2016). There is a wealth of evidence indicating that cue reactivity is modulated by individual factors and can be predictive of substance dependence severity, risk of relapse and treatment outcomes (Jasinska Reference Jasinska, Stein and Kaiser2014) (biomarkers are discussed further below).
Impulsivity and inhibitory control
Impulsivity is characterised by a lack of inhibitory control and manifests as action in the absence of premeditation. There are several domains of impulsivity to consider, including choice, trait and impulsive action (Mitchell Reference Mitchell and Potenza2014). Increased impulsivity, either alone or as part of another disorder (e.g. attention-deficit hyperactivity disorder or bipolar disorder), is thought to contribute to the increased risk of substance dependence (Dalley Reference Dalley, Everitt and Robbins2011). Compared with dysregulation of the reward system, clinical intervention to attenuate impulsivity and improve inhibitory control is less prevalent.
Evidence from questionnaires such as the Barratt Impulsiveness Scale (BIS-11) and the Urgency, Premeditation (lack of), Perseverance (lack of), Sensation Seeking, Positive Urgency, Impulsive Behavior Scale (UPPS-P) consistently show increased trait impulsivity substance-dependent people compared with healthy controls (Taylor Reference Taylor, Murphy and Boyapati2016). Similarly, choice impulsivity assessed by the Kirby Delay-Discounting task is also higher in substance-dependent individuals compared with healthy controls, demonstrated by a preference for smaller, more immediate rewards over larger, delayed rewards (Gray Reference Gray and MacKillop2015). Interestingly, several studies have shown an association between blunted response in the ventral striatum during reward anticipation in the MID task and increased trait and choice impulsivity in substance-dependent individuals (Beck Reference Beck, Schlagenhauf and Wüstenberg2009; Taylor Reference Taylor, Murphy and Boyapati2016; Murphy Reference Murphy, Nestor and McGonigle2017). In line with this, substance dependence-related deficits in neural activation during choice impulsivity tasks have been located in regions associated with reward processing. These include the ventral striatum, ACC, orbitofrontal cortex (OFC) and dorsal PFC (Hester Reference Hester and Garavan2004; Hoffman Reference Hoffman, Schwartz and Huckans2008).
Motor inhibition can be measured with tasks such as the go/no-go and stop-signal tasks. In abstinent cocaine-dependent individuals increased BOLD signal has been observed in the right inferior frontal gyrus, the superior frontal gyrus and the medial prefrontal gyrus during successful response inhibition, whereas hypoactivation of these regions is found in active cocaine users (Hester Reference Hester and Garavan2004; Connolly Reference Connolly, Foxe and Nierenberg2012). This suggests that brain function underpinning inhibitory control may vary as a function of length of abstinence and could be used as a predictor of relapse.
Neuroadaptations to chronic substance exposure
Tolerance
As a drug is consumed regularly, a higher dose may be required to achieve a response equivalent to that experienced following initial consumption. This is termed tolerance and results from neuroadaptive changes in the brain to maintain homeostasis. These include processes such as receptor downregulation and desensitisation and changes in enzyme production and metabolism (for a review see Lüscher Reference Lüscher and Malenka2011). Owing to the wide range of pharmacological mechanisms of different drugs of misuse, such neuroadaptations may vary dependent on both the drug and the individual. It is also important to note that tolerance to behavioural and physiological effects of drugs may develop at different rates. For example, chronic opioid consumption is associated with reduced sensitivity of the mu opioid receptor – the key receptor mediating analgesia, pleasurable rewarding effects and respiratory depression (for a review see Allouche Reference Allouche, Noble and Marie2014). Although tolerance to the euphoric effects of opiates occurs rapidly, underpinning further drug-seeking behaviour, tolerance to the respiratory depression effects of opioids happens more gradually. This desynchrony can be life-threatening as an individual will seek more of the drug to achieve the euphoric effect while putting themselves at risk of fatal respiratory depression. Given the life-threatening potential of these effects it is important that we better understand the mechanism underpinning tolerance.
Withdrawal
Following these neuroadaptations, abrupt cessation of the drug leaves the brain unable to achieve homeostasis, resulting in withdrawal characterised by dysphoria, emotional stress and a decrease in cognitive ability. Just as neuroadaptive changes differ for each drug of misuse and individual, so do some withdrawal symptoms. Some drugs, such as alcohol and opiates, produce significant physiological withdrawal symptoms (e.g. alcohol: tremors, sweating, nausea, seizures, etc.; opiates: muscle aches, lacrimation, sweating, diarrhoea), whereas others more prominently cause emotional symptoms (e.g. stimulants: depression). Withdrawal often perpetuates drug use or leads to relapse as an individual attempts to overcome its negative effects. As the brain adapts to the absence of the drug, generally withdrawal diminishes over days to weeks.
Relapse
For those individuals who achieve abstinence, risk of relapse remains high and although this risk is reduced over time it never completely disappears. Relapse may occur years after achieving abstinence, in response to environmental stressors, low mood or stress during the preoccupation/anticipation phase of the substance dependence associated with enduring dysregulation in key brain processes such as reward and inhibitory control (see above). As described above, salient cues result in activation in several brain regions and are associated with experiences such as an urge to use or ‘craving’ which may lead to relapse. However, craving is not a unitary concept and also may reflect positive or negative reinforcement, may be difficult to measure clinically and does not correlate well with relapse. Owing to the high rate of relapse it is important that we better understand the mechanisms underpinning relapse in order to improve clinical management.
Modulation of brain indices and biomarkers
Psychosocial interventions
If brain indices identified by fMRI are to have clinical utility, then they should demonstrate modulation in the presence of an efficacious treatment, in a direction consistent with therapeutic benefit. Psychosocial interventions are the mainstay of treatment in substance use disorders, but there is limited literature investigating how these interventions modulate proposed biomarkers in addictions, despite the effectiveness of several of them. Motivational interviewing, for example, is an empathetic interview style that focuses on changing behaviours in relation to an individual's goals (Miller Reference Miller1983). Motivational interviewing in substance use disorders has been shown to have an impact on positive outcomes, but despite this only one study has investigated its effects on fMRI indices during a salient cue-reactivity task. For alcohol dependence, there was significant activation of the OFC, nucleus accumbens, insula, caudate and putamen in response to alcohol-related cues accompanied by ‘counter-change talk’ (language supporting the status quo), but there was no significant activation of these areas during the same cue-reactivity task when accompanied by ‘change talk’ (language relating to commitment to change) (Feldstein Ewing Reference Feldstein Ewing, Filbey and Sabbineni2011). This illustrates how fMRI could be used to provide biological support for the benefits of psychosocial interventions, and much more research could be conducted in this area.
Pharmacological interventions
Naltrexone
More commonly, pharmacological modulation of brain indices and biomarkers have been investigated, although it is worth noting that there is also limited evidence in this area. One medication that has been investigated using fMRI is naltrexone, a mu opioid receptor antagonist that is recommended as first-line treatment for relapse prevention in alcohol and opiate dependence. For alcohol dependence, it has been shown to reduce craving and alcohol consumption in some, but not all, putatively by blocking the positively reinforcing effects of alcohol. Use of an opioid antagonist also was consistent with PET studies showing greater levels of mu opioid receptors in early abstinence in a range of addictions (Gorelick Reference Gorelick, Kim and Bencherif2005; Heinz Reference Heinz, Reimold and Wrase2005; Williams Reference Williams, Daglish and Lingford-Hughes2007). A more recent study, however, found no difference in opioid receptors in longer-term abstinent alcohol-dependent individuals with blunted amphetamine-induced endogenous opioid release (Turton Reference Turton, Myers and Mick2020). Therefore, there appears to be variability in the status of the opioid system in alcoholism that may contribute to why naltrexone does not benefit all patients.
Naltrexone has been the most extensively studied pharmacotherapy in addiction and has been important in helping us understand the mechanisms of alcohol dependence as well as enabling us to understand more about the recovery and resilience of brain function in addiction to inform development of further treatments. For instance, naltrexone has been shown to attenuate alcohol cue-induced activation of the ventral striatum in alcohol dependence relative to placebo (Myrick Reference Myrick, Anton and Li2008). More recently, using a cohort from the PREDICT multicentre trial, abstinent alcohol-dependent individuals with a high baseline cue-elicited ventral striatum activation have been shown to have better outcomes (length of time to first relapse) on naltrexone compared with individuals from the same population who have low cue-elicited ventral striatum activation. In the same study, this finding was not observed for acamprosate, the alternative first-line treatment for relapse prevention in alcohol dependence (Mann Reference Mann, Vollstädt-Klein and Reinhard2014) (we will return to acamprosate in the section on Clinical management). A subsequent replication study showed that naltrexone reduced alcohol cue-elicited striatal activation after 2 weeks of treatment and reduced heavy drinking over 16 weeks. Those with the greatest modulation by naltrexone experienced the least heavy drinking during follow-up. Interestingly, naltrexone was only superior to placebo among smokers in this cohort, suggesting that both smoking and the extent of modulation in cue-elicited brain activation may predict treatment response (Schacht Reference Schacht, Randall and Latham2017). The latest study investigating naltrexone in early abstinence has found that naltrexone attenuated the striatal response to alcohol cues and reduced risk of relapse into heavy drinking within 3 months of treatment. Additionally, increased cue-elicited activation in the left putamen (and its progressive increase in the weeks following abstinence) predicted both naltrexone response and risk of relapse, supporting a potential role of neural cue reactivity as a biomarker in the development of precision medicine approaches (Bach Reference Bach, Weil and Pompili2019).
The effects of naltrexone have also been studied in the context of modulating the neural correlates of non-drug-related reward, providing further insight into the mechanism underlying its efficacy in relapse prevention. Using a MID fMRI paradigm, a collaboration between Imperial College London, the University of Cambridge and the University of Manchester (the ICCAM consortium: for further details see Paterson Reference Paterson, Flechais and Murphy2015) showed that both the abstinent alcohol-dependent and abstinent polydrug-dependent (alcohol plus opiate and/or cocaine) groups had a blunted response to non-drug reward anticipation compared with neutral anticipation. The polydrug-dependent group also had a blunted reward anticipation response in the left OFC compared with the alcohol-dependent and control groups. This effect was not ameliorated by naltrexone, suggesting that an alternative mechanism of neural modulation may contribute to its efficacy (Nestor Reference Nestor, Murphy and McGonigle2017).
DRD3 antagonists
The ICCAM consortium have also implemented the same fMRI paradigm to examine the effects of the D3 dopamine receptor (DRD3) antagonist GSK598809 on the MID task in abstinent alcohol-dependent, abstinent polydrug-dependent and healthy control groups. Compared with placebo, GSK598809 was shown to enhance the blunted ventral striatal response to monetary anticipation in the polydrug-dependent group, suggesting that DRD3 antagonism may restore reward deficits in substance dependence (Murphy Reference Murphy, Nestor and McGonigle2017).
Prediction of relapse
As observed in the cue-reactivity literature, neuroimaging markers of non-drug reward processing may also have utility in predicting clinical outcome. For example, in a non-drug reinforcement-learning task, methamphetamine users who had relapsed were observed to exhibit lower striatal activation in response to task outcomes relative to abstainers at 1 year (Stewart Reference Stewart, May and Poppa2014). Reduced striatal activation in response to monetary reward anticipation was similarly shown to be predictive of relapse in a longitudinal study involving participants with cocaine dependence and, interestingly, this blunted response was shown to ‘normalise’ with increasing time spent abstinent, highlighting the potential of this biomarker to track progress during recovery (Balodis Reference Balodis, Kober and Worhunsky2016).
Using a similar approach but with decision-making tasks, a role for the insula in decision-making deficits observed in addiction has been demonstrated (Gowin Reference Gowin, Mackey and Paulus2013). In an early study, baseline insula activation correctly predicted the relapse status of >90% of methamphetamine-dependent individuals (Paulus Reference Paulus, Tapert and Schuckit2005). In follow-up studies using a monetary reward task that incorporates a decision-making component, differential insula and dorsal striatal response to winning large risky, relative to small safe rewards could reliably predict relapse with better sensitivity and specificity than clinical variables (Gowin Reference Gowin, Harlé and Stewart2014, Reference Gowin, Ball and Wittmann2015).
Together these data suggest that attenuated response to non-drug reward and risk processing in striatal and/or insula areas may increase vulnerability to relapse. With further validation, such markers could potentially serve as targets for future therapeutic interventions and could help identify those most susceptible to relapse or those in most need of early intervention or additional resources to improve outcomes.
Clinical management: neurobiological translation and current issues
In the UK, effective treatments for substance dependence include psychosocial interventions and pharmacological therapy. Recommended management involves a combination of these, with multidisciplinary team involvement. Pharmacological therapies vary depending on the drug of dependence but can be broadly categorised for use during acute intoxication, substitution, detoxification (detox) or relapse prevention. Table 3 summarises the current treatment options and their mechanisms. For more information about prescribing, see the British Association of Pharmacology (BAP) guidelines (Lingford-Hughes Reference Lingford-Hughes, Welch and Peters2012).
TABLE 3 Current pharmacological interventions and their mechanisms of action for drugs of dependence

GABA, γ-aminobutyric acid; NMDA, N-methyl-d-aspartate; GHB, γ-hydroxybutyric acid; GBL, γ-butyrolactone; nAChR, nicotinic acetylcholine receptor.
Pharmacological treatment should be seen as adjunctive to psychosocial therapy for most substance use disorders. However, for specific substances, pharmacological therapy is highly likely to be required, such as benzodiazepines for medically assisted alcohol detoxification to reduce the symptoms of withdrawal and prevent complications of seizures, or management of opioid dependence with opiate substitution therapy. It should be noted that for stimulants (cocaine, amphetamine, etc.), cannabinoids, nitrous oxide, methylenedioxymethamphetamine (MDMA), hallucinogens and other novel psychoactive substances, there are no specific pharmacological therapies, but treatment during any phase of intoxication, detoxification or withdrawal should be supportive and medication for symptomatic relief (i.e. antipsychotics, anxiolytics, etc.) can be administered if it is safe to do so.
As previously described, substance dependence is multifactorial and extremely complex, with environmental, genetic, social and physical factors all playing a role in an individual's susceptibility and treatment needs. Moreover, abstinence rates remain low and relapse rates high for all substances of dependence, with a lack of specific and evidence-based relapse prevention options available. Randomised controlled trials (RCTs) investigating pharmacotherapy in addictions produce mixed results, or efficaciousness cannot be generalised to all patient subsets even for individuals using the same psychoactive substance, owing to likely heterogeneity of underlying neurobiology.
As mentioned above, alcohol consumption leads to the release of endogenous opioids that bind to mu opioid receptors, which in turn interact with the dopamine system of the brain, causing pleasurable and reinforcing effects. Naltrexone competitively binds to the mu opioid receptor and modulates the dopaminergic cell firing in the VTA, which would be consistent with it reducing the rewarding effects of alcohol. Several meta-analyses of RCTs comparing naltrexone have found a small but significant reduction in drinks per day, days of heavy drinking and reduction in craving, but there is no effect on improving continuous abstinence rates. Therefore, naltrexone appears to benefit dependent drinkers who want to remain abstinent but lapse and may also be beneficial for those who are non-dependent heavy drinkers who want to reduce their consumption.
In comparison, acamprosate, a glutamatergic N-methyl-d-aspartate (NMDA) receptor antagonist, attenuates the hyperglutamatergic state resulting from chronic alcohol use. Unlike naltrexone, there are limited neuroimaging studies of acamprosate. However, in a study using MRS, Umhau et al (Reference Umhau, Momenan and Schwandt2010) reported that acamprosate reduced glutamate levels in alcohol dependence, supporting the preclinical data. Several systematic reviews of RCTs conclude that, compared with placebo, acamprosate moderately increases duration of abstinence after detoxification. However, studies that included individuals with lower severity of dependence have found no effect on duration of abstinence, indicating that acamprosate might only be beneficial for those with severe dependence. Other factors, such as delay in starting acamprosate after detoxification, high physiological dependence at baseline, negative family history of alcoholism and serious anxiety or craving at baseline may predict that a response to acamprosate is less likely (for a review see Lingford-Hughes Reference Lingford-Hughes, Welch and Peters2012).
The dearth of clinically useful predictors for treatment and the limited range of medications highlight the substantial gaps that remain in our knowledge and ability to identify alcohol-dependent individuals who would respond best to different relapse prevention medications. Indeed, arguably the situation is worse for other substance dependences with fewer, or no, clinically efficacious medications available (for a review see Lingford-Hughes Reference Lingford-Hughes, Welch and Peters2012). In addition, being able to identify the best treatment and response for individuals dependent on more than one substance or with a coexisting psychiatric disorder would improve abstinence rates and quality of life, while decreasing the cost and burden of addictions on society as a whole.
Future directions
Functional neuroimaging has played a key role in delineating the neurobiology of addiction in humans, as it has done more broadly within psychiatric research. Our understanding of fMRI, higher spatial and temporal resolution, better image processing and statistical modelling, along with an increase in number of participants in studies, have resulted in greater confidence in interpretation and replicability of findings. Many of these issues also apply to PET; however, the critical limiting factor here is the lack of available robust tracers to probe targets of interest such as glutamate receptors. There is also now an emphasis on preregistration of neuroimaging studies, which involves committing to an analysis plan prior to the research being conducted. This avoids what are known as ‘fishing expeditions’, where hypotheses are formulated after results are known. Preregistration enables better quality of analysis and transparency of results. Important for future functional neuroimaging studies is a focus on the ‘three Rs’: replication, reliability and reproducibility.
Most fMRI studies currently use a region of interest (ROI) approach to test hypotheses concerning brain regions. Regarding addiction as a problem involving single brain regions greatly oversimplifies what is known to involve a complex interaction of multiple brain circuits. Recently, analysis techniques have evolved to include modelling of networks, requiring greater computational power and mathematical expertise, but with the potential to regard addiction as a problem of dysregulated networks rather than isolated brain regions. Future research in this area is likely to involve the formation and growth of multicentre cohort studies to provide insight into these questions, such as ENIGMA (http://enigma.ini.usc.edu/) and IMAGEN (https://imagen-europe.com/).
Although fMRI continues to provide insight into the underlying neural mechanisms involved and how these are modulated with treatment, several questions remain unanswered. There is growing evidence that neural circuitry plays a role in one's predisposition to addiction, and this needs to be explored in more detail. Additionally, many changes in neural activity associated with chronic substance use are not well characterised. For example, direct causality and effect of such neural changes and the involvement of environmental and genetic factors are still to be determined. It is also unknown whether changes in neural activity ‘normalise’ during abstinence and whether there is a role for pharmacotherapy to facilitate this process. Addressing these questions may confer a therapeutic benefit.
The frequency of polysubstance use is a factor that is likely to continue to be a barrier to the investigation of individual substances and their isolated effects. Continued investment in large-cohort longitudinal studies is likely to aid in answering these questions, in particular those that include well-defined addiction outcomes, as historically there have been inconsistencies in alcohol and substance use data collected by large multicentre studies, making research with these resources more difficult to employ.
Finally, in the UK the present segregation of addiction services from the NHS and systematic cuts in funding are ongoing barriers to successful clinical management that should not be overlooked (Bowden-Jones Reference Bowden-Jones, Sinclair and Lingford-Hughes2020). With most services currently being run by third-sector organisations, this has eliminated training posts for psychiatrists, including academic trainees, and inhibits general interaction with healthcare trusts and research bodies. The adverse impact of this on research must be countered if we are to optimise development of novel treatment and prediction tools to improve outcomes.
Author contributions
All four authors contributed to drafting and preparing the final version of the manuscript.
Declaration of interest
None.
ICMJE forms are in the supplementary material, available online at https://doi.org/10.1192/bja.2020.68.
MCQs
Select the single best option for each question stem
1 Which of the following substances of misuse directly increases extracellular dopamine?
a Alcohol
b Stimulants
c Opiates
d Nicotine
e Cannabis.
2 Different substances of misuse cause varying neuroadaptations following chronic consumption. Chronic use of which substance primarily causes a decrease in GABAA receptor function while increasing the function of NMDA receptors?
a Nicotine
b Cocaine
c Opiates
d Alcohol
e Cannabis.
3 fMRI paradigms are commonly used to probe the neurocircuitry of addiction. Which of the following regarding neural responses in reward circuitry (e.g. ventral striatum) to a stimulus is correct?
a In response to drug-related stimuli (cues), the BOLD response is blunted in abstinent substance-dependent individuals compared with healthy controls
b In response to anticipation of winning money, the BOLD response is greater in abstinent substance-dependent individuals compared with healthy controls
c In abstinent substance-dependent individuals, a blunted BOLD response to monetary reward anticipation is associated with increased likelihood of relapse
d In adolescents, a heightened response to monetary reward anticipation is seen in those who go on to problematic drug use compared with those who do not
e The length of abstinence does not influence the BOLD response to drug- or non-drug-related stimuli.
4 Naltrexone is a first-line treatment for relapse prevention in alcohol dependence and several studies have investigated its underlying neurobiology and effects. Which of the following is a correct finding related to alcohol dependence?
a Naltrexone commonly increases craving in abstinent alcohol-dependent individuals
b Naltrexone attenuates heightened BOLD signal activation in response to salient alcohol cues compared with placebo
c Naltrexone increases ventral striatum activation in response to salient alcohol cues compared with placebo
d Naltrexone is an agonist at the delta opioid receptor
e No association has been found between naltrexone modulation of brain responses and clinical outcomes.
5 For which of these substances are benzodiazepines (e.g. diazepam, chlordiazepoxide) not to be used to aid detox?
a Opiates
b Benzodiazepines
c Alcohol
d Ketamine
e GHB/GBL.
MCQ answers
1 b 2 d 3 c 4 b 5 a
eLetters
No eLetters have been published for this article.