Background
Sepsis is an abnormal host response to infection, resulting in organ and tissue damage (Singer et al., Reference Singer, Deutschman, Seymour, Shankar-Hari, Annane, Bauer and Angus2016). Septic shock is the most severe form of sepsis, with a significantly higher mortality rate due to profound circulatory and metabolic abnormalities (Singer et al., Reference Singer, Deutschman, Seymour, Shankar-Hari, Annane, Bauer and Angus2016). The annual worldwide incidence of sepsis in adults has been estimated at 31.5 million cases per year, of which 5.3 million instances are fatal (Fleischmann et al., Reference Fleischmann, Scherag, Adhikari, Hartog, Tsaganos and Schlattmann2016). Sepsis poses a high financial burden on the economy, with US in-hospital costs for each episode averaging US$22,100 (Angus et al., Reference Angus, Linde-Zwirble, Lidicker, Clermont, Carcillo and Pinsky2001).
Individuals vary widely in terms of their susceptibility to sepsis and their prognosis, and this heterogeneity is thought to be, in part, due to host genetic factors (Sørensen et al., Reference Sørensen, Nielsen, Andersen and Teasdale1988). A genetic basis for susceptibility to sepsis has long been suspected because epidemiological studies found that adopted individuals had markedly increased risk of mortality from infection when a biological parent had died prematurely from infection, but no corresponding increase risk in mortality when an adoptive parent had died from infection (Sørensen et al., Reference Sørensen, Nielsen, Andersen and Teasdale1988). However, previous candidate gene and genomewide association studies (GWAS) of sepsis have had limited success in identifying genetic loci robustly associated with the disease and outcomes (Rautanen et al., Reference Rautanen, Mills, Gordon, Hutton, Steffens and Nuamah2015; Scherag et al., Reference Scherag, Schöneweck, Kesselmeier, Taudien, Platzer, Felder and Brunkhorst2016; Srinivasan et al., Reference Srinivasan, Page, Kirpalani, Murray, Das and Higgins2017). This is possibly due to lack of statistical power caused by a combination of small sample sizes and heterogeneity of both the patient population and the phenotype (Rautanen et al., Reference Rautanen, Mills, Gordon, Hutton, Steffens and Nuamah2015; Scherag et al., Reference Scherag, Schöneweck, Kesselmeier, Taudien, Platzer, Felder and Brunkhorst2016; Srinivasan et al., Reference Srinivasan, Page, Kirpalani, Murray, Das and Higgins2017).
We designed the ADRENAL Genome-Wide Association Study (ADRENAL-GWAS) to investigate the genetic influences on susceptibility to, resolution of and mortality from septic shock. We performed a GWAS on a cohort of critically ill patients who were enrolled into a randomized controlled trial into the efficacy of corticosteroid therapy in septic shock (ADRENAL; Venkatesh et al., Reference Venkatesh, Finfer, Cohen, Rajbhandari, Arabi and Bellomo2018). We sampled individuals suffering from septic shock, as opposed to sepsis, on the rationale that focusing on extreme cases will increase statistical power to detect genetic associations as has been done successfully in the case of many other diseases and complex traits (Barnett et al., Reference Barnett, Lee and Lin2013).
To improve our understanding of the genetic basis of sepsis, we performed additional analyses with increased power to complement the knowledge gained from the GWAS. These included gene and pathway-based analysis and evaluation of polygenic risk scores. To our knowledge, these approaches have not been used in the evaluation of sepsis or septic shock.
Methods
Study Participants
The ADRENAL-GWAS is a substudy within the main ADRENAL trial (ClinicalTrials.gov number, NCT01448109) designed to investigate the genetics and genomics of septic shock. All ADRENAL participants who were admitted to 27 participating hospital sites in three countries (Australia, New Zealand and the UK) were eligible for the ADRENAL-GWAS substudy. The inclusion/exclusion criteria were similar to the original ADRENAL study and are summarized in Supplementary Methods S1 (Venkatesh et al., Reference Venkatesh, Finfer, Cohen, Rajbhandari, Arabi and Bellomo2018). Blood samples from ADRENAL-GWAS participants (N = 578) were collected at the time of randomization, prior to administration of corticosteroids/placebo. Blood was collected into 2 × 2.5 ml EDTA, 2 × 2.5 ml serum blood collection vacuettes (Interpath; Cat. No. 455071), and 1 × 2.5 ml PAXgene RNA Vacutainer (Becton Dickinson; Cat. No. 762165).
An unpublished genotyped cohort consisting of 3624 individuals collected at the QIMR Berghofer Medical Research Institute (QIMRB) was used as a control group in the case–control GWAS analyses. These unselected controls were drawn from the controls (i.e., ‘healthy’ individuals) used in studies of reproductive health or melanoma risk factors. DNA was extracted from either blood or saliva samples. Notably, the controls, while drawn from an unselected cohort, were deliberately chosen as they were genotyped using the same array, and had similar underlying ancestries to the ADRENAL participants.
Genotyping and Quality Control
ADRENAL-GWAS genomic DNA extractions were performed on 200 μl of whole blood using the QIAsymphony SP instrument according to the manufacturer’s protocol (QIAsymphony DSP DNA Mini Kit, Cat. No. 937236). Genomic DNA was eluted in 100 μl of Buffer ATE and quantified using the Trinean Dropsense 96. Samples were genotyped on the Illumina Infinium Global Screening Array-24+ v1.0 (20005136). The arrays were scanned on an Illumina iScan system, and the raw fluorescence intensity data were normalized and clustered for each sample using Illumina Genome Studio (v 2.0.3). Genotypes were called using the standard Illumina GSA-24v1-0_A6 Cluster File.
In the QIMRB cohort, DNA was extracted from either blood or saliva samples, and genotyped using the Illumina Infinium Global Screening Array-24+ v1.0. Genotype data were screened for genotyping quality (GenCall < 0.7), single-nucleotide polymorphism (SNP) and individual call rates (<0.95), Hardy–Weinberg Equilibrium (HWE) failure (p < 10–6), and minor allele frequency (MAF < 0.01). As these samples were genotyped in the context of a larger project, the data were integrated with the larger QIMRB genotype project and the data were checked for pedigree, sex and Mendelian errors, and for non-European ancestry.
The PLINK v1.90b3.31 software package was used to carry out a number of standard quality control (QC) procedures (Chang et al., Reference Chang, Chow, Tellier, Vattikuti, Purcell and Lee2015). A detailed breakdown of QC procedures can be found in the Supplementary Methods S3.
Outcomes
The primary outcome was death from any cause at 90 days, and the secondary outcomes were death at 28 days, shock resolution and susceptibility to septic shock. For diagnostic criteria and definitions of these outcomes, see Supplementary Methods S1 and S2.
Statistical Power Analyses
We investigated power to detect variants at genomewide levels of significance (α = 5 × 10–8) and also power to replicate variants reported in previous GWAS of susceptibility to sepsis and 28-day mortality (α = .05) using the Genetic Association Study Power Calculator (Johnson & Abecasis, Reference Johnson and Abecasis2017). The relationship between heterozygous relative risk (RR; a measure of genetic effect size) and statistical power is presented in the Supplementary Methods S4. We assumed a 1% lifetime risk of septic shock, a multiplicative model of disease risk (on the odds scale), that the risk locus had been genotyped (r 2 = 1), and we matched the GWAS sample sizes (see Supplementary Methods S4).
The susceptibility to septic shock GWAS had the most statistical power of all the GWAS, where for genomewide association analyses (α = 5 × 10–8), we expect 80% power to detect variants with a heterozygous RR of 1.55 and 2.52 for a risk allele frequency of .50 and .05, respectively. For replication of previous findings (α = .05), there is 80% power to detect variants with a heterozygous RR of 1.22 and 1254 for a risk allele frequency of .50 and .05, respectively. Power calculations for the other analyses are presented in Supplementary Figure S3.
Genomewide Association Analyses
We performed logistic regression analysis assuming an underlying additive genetic model (on the log scale) as implemented in PLINK across the genome on septic shock patients (ADRENAL-GWAS) and healthy controls (QIMRB). The first five principal components (PCs) from a PC analysis of the cleaned merged GWAS dataset were used as covariates. Furthermore, we performed logistic regression analysis, on ADRENAL-GWAS participants only (no controls), assuming an underlying additive genetic model using PLINK for 28-day mortality, 90-day mortality, and shock resolution. Covariates included sex and the first five PCs.
Look Up of Previously Reported Variants
Suggestive associations (p ≤ 1 × 10–5) reported in analyses of 28-day survival/mortality in sepsis (Rautanen et al. survivors = 1194, nonsurvivors = 359; Scherag et al. survivors = 2803, nonsurvivors = 667) were queried in our 28-day mortality GWAS summary statistics (Rautanen et al., Reference Rautanen, Mills, Gordon, Hutton, Steffens and Nuamah2015; Scherag et al., Reference Scherag, Schöneweck, Kesselmeier, Taudien, Platzer, Felder and Brunkhorst2016). Likewise, SNPs that reached suggestive significance in a susceptibility GWAS of premature infants (Srinivasan et al. cases = 351, controls = 406) and two GWAS from the UK Biobank (UKBB) were also queried in our susceptibility to septic shock GWAS summary statistics (Sudlow et al., Reference Sudlow, Gallacher, Allen, Beral, Burton, Danesh and Collins2015; Srinivasan et al., Reference Srinivasan, Page, Kirpalani, Murray, Das and Higgins2017; Neale, Reference Neale2018). The UKBB analyses were originally performed on cohorts defined as having ‘other septicaemia’ (Phecode_A41, cases = 1096, controls = 360,098) and ‘septicaemia/sepsis’ (Phecode_20002_1657, cases = 238, controls = 360,956).
Gene- and Pathway-Based Analyses
A number of gene-/pathway-based analyses were performed on the GWAS data for each outcome to identify genes or pathways that were enriched through combining statistical information across many markers within a gene, or within multiple genes in a pathway, and testing for association with the outcome. The Complex-Traits Genetics Virtual Lab (CTG-VL) implemented version of FastBAT was used to perform gene-based association analyses on the summary-level results (Bakshi et al., Reference Bakshi, Zhu, Vinkhuyzen, Hill, McRae, Visscher and Yang2016; Cuéllar-Partida et al., Reference Cuéllar-Partida, Lundberg, Fang Kho, D’Urso, Gutiérrez-Mondragón, Thanh Ngo and Hwang2019). Data-Driven Expression-Prioritized Integration for Complex Traits (DEPICT v.1 beta) was used to identify enriched genes, gene-sets/pathways and cell/tissue types from independent lead variants (r 2 = .2, MAF > 0.05, clump-kb 1000), which reached suggestive significance (p ≤ 1 × 10–5; Pers et al., Reference Pers, Karjalainen, Chan, Westra, Wood, Yang and Franke2015).
Polygenic Risk Score Analyses
We downloaded GWAS summary statistics for phenotypes with suspected shared genetic etiology with sepsis, or requested the data from the authors (Sudlow et al., Reference Sudlow, Gallacher, Allen, Beral, Burton, Danesh and Collins2015; Zheng et al., Reference Zheng, Erzurumluoglu, Elsworth, Kemp, Howe, Haycock and Neale2017; Neale, Reference Neale2018). These included large-scale GWAS meta-analyses for hematocrit, diastolic blood pressure (DBP), granulocyte count, white blood cell count (WBC), coronary artery disease, type-2 diabetes (T2D), C-reactive protein levels (CRP), ‘septicaemia/sepsis’ (Phecode_20002_1657) and ‘other septicaemia’ (Phecode_A41) (International Consortium for Blood Pressure Genome-Wide Association Studies et al., Reference Ehret, Munroe, Rice, Bochud, Johnson and Johnson2011; Sudlow et al., Reference Sudlow, Gallacher, Allen, Beral, Burton, Danesh and Collins2015; Astle et al., Reference Astle, Elding, Jiang, Allen, Ruklisa, Mann and Soranzo2016; Scott et al., Reference Scott, Scott, Mägi, Marullo, Gaulton and Kaakinen2017; Ligthart et al., Reference Ligthart, Vaez, Võsa, Stathopoulou, de Vries, Prins and Alizadeh2018). Polygenic risk scores (PRS) for height were also constructed as a negative control (Yengo et al., Reference Yengo, Sidorenko, Kemper, Zheng, Wood and Weedon2018). Independent SNP signals from each set of summary statistics were identified using PLINK (r 2 = .1, clump-kb = 1000).
Genomewide PRS for each of the above diseases/traits were generated for the 493 septic shock cases as well as for the 2442 control individuals that passed QC. Individuals were scored on the number of risk alleles they carried for each variant (at the thresholds p < 5 × 108, 5 × 10–6, .01, .5, 1), weighted by regression coefficients from the respective GWAS (Evans et al., Reference Evans, Brion, Paternoster, Kemp, McMahon, Munafò and Smith2013). To investigate relationships between septic shock and the various traits, 28-day mortality was regressed on the PRS generated for each septic shock individual using logistic regression, with age, sex and the first five PCs from the GWAS as covariates. Disease status (logistic regression) was regressed against the scores generated for the case/control cohort, with the first five PCs used as covariates.
Results
From May 2014 through April 2017, 578 patients were enrolled into the GWAS substudy at 27 hospital sites. The intensive care units were in Australia (18 sites), New Zealand (4) and the UK (5). Of the 578 patients enrolled, 300 were assigned to receive hydrocortisone and 278 to receive placebo. A total of 493 septic shock cases passed QC, and of the 3624 QIMRB controls, 2442 individuals passed (Supplementary Methods S3). All individuals passing QC were of European descent (Supplementary Figure S1). A breakdown of the outcomes, sample sizes and characteristics in ADRENAL-GWAS cases and QIMRB controls can be found in Table 1. Treatment had no significant effect on 90-day mortality, 28-day mortality or shock resolution (p =.35, .62, .09) and therefore had no confounding effect on the GWAS. GWAS were performed for all outcomes; however, we focused the latter analyses on 28-day mortality and susceptibility to septic shock to replicate past GWAS of sepsis, to be consistent, and reduce the burden of multiple testing correction.
Table 1. Characteristics of ADRENAL-GWAS patients prior to randomization and administration of corticosteroids, and QIMRB controls

Note: Reported is the ratio and percentage for dichotomous characteristics, and the mean and standard deviation (SD) for quantitative traits. Scores on the Acute Physiology and Chronic Health Evaluation (APACHE) II assess the severity of disease, ranging between 0 (low risk for death) and 71 (high risk). A Student’s t-test was used to test for differences in the mean quantitative traits between 90-day survivors and nonsurvivors. A chi-squared test of independence was used to test for difference in dichotomous traits between 90-day survivors and nonsurvivors.
a The susceptibility to septic shock analyses were the only analyses containing QIMRB controls.
b There was some missing data. QIMRB, QIMR Berghofer Institute of Medical Research.
Genomewide Association Studies
GWAS were performed for susceptibility to shock (493 cases, 2442 controls), 28-day mortality (90 nonsurvivors, 403 survivors), 90-day mortality (112 nonsurvivors, 381 survivors) and shock resolution (34 unresolved, 459 resolved). The Manhattan plots for each GWAS are presented in Figure 1. The T-allele of the genotyped SNP rs9489328 was the only genetic variant to be significantly associated with any of the outcomes. This SNP sits within a noncoding RNA gene AL589740.1 (Supplementary Figure S4), and was associated with decreased risk of septic shock (p = 1.05 × 10–10; Table 2). Three genetic variants, rs11167801, rs7698838, and rs17128291, were associated with shock resolution at suggestive levels of significance (p < 1 × 10−6). Quantile–quantile plots (Supplementary Figure S5) and genomic inflation factors (Supplementary Table S2) suggest that the GWAS results were not systematically inflated.
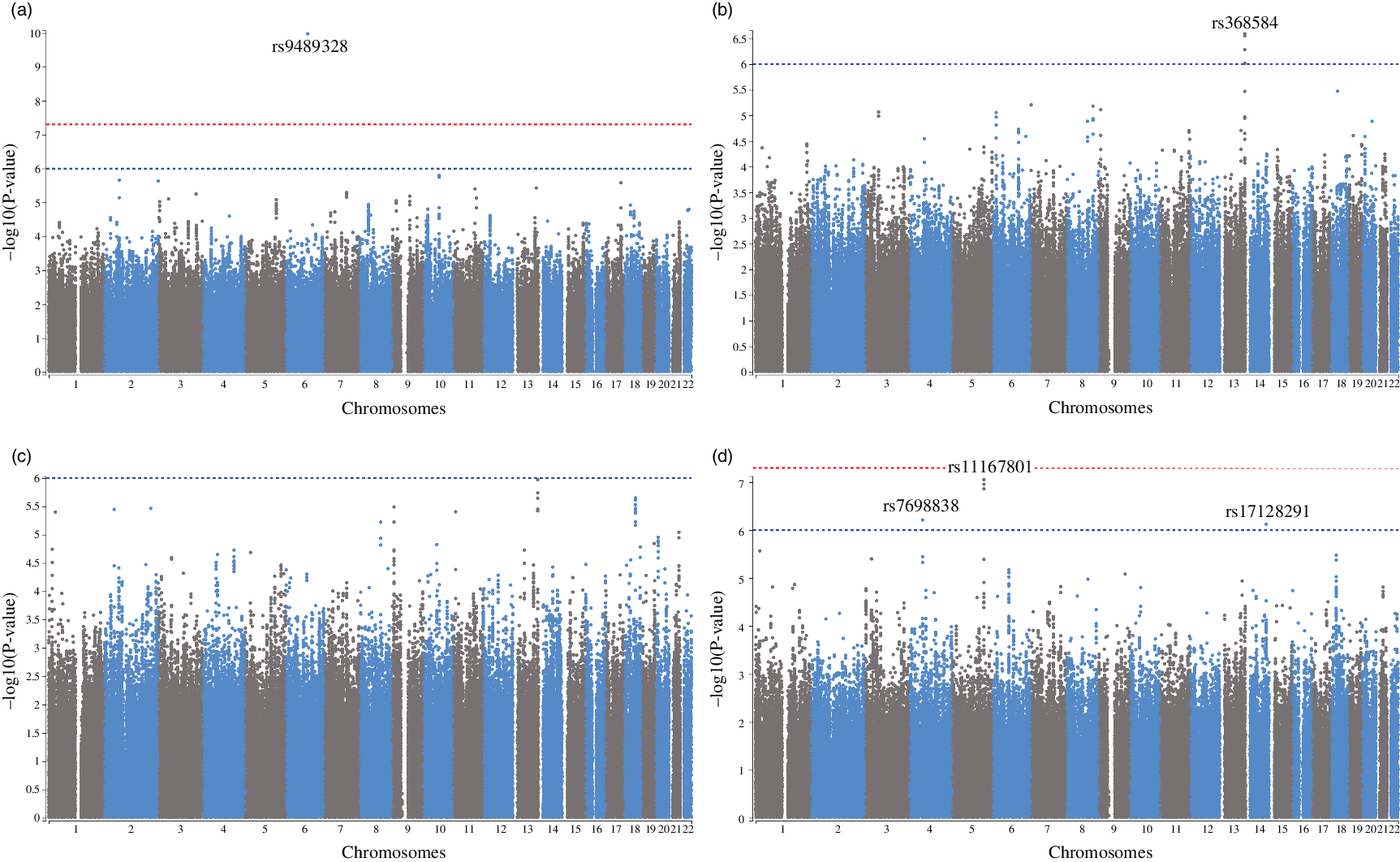
Fig. 1. Manhattan plots for (A) susceptibility to septic shock, (B) 28-day mortality, (C) 90-day mortality and (D) resolution of shock genomewide association studies. Plots were generated using the Complex-Traits Genetics Virtual Lab (Cuéllar-Partida et al., Reference Cuéllar-Partida, Lundberg, Fang Kho, D’Urso, Gutiérrez-Mondragón, Thanh Ngo and Hwang2019). The blue dotted line denotes the ‘suggestive significance’ threshold of p < 1 × 10–6, and the red line denotes the ‘genomewide significance’ threshold of p < 5 × 10–8, and the lead single nucleotide polymorphisms surpassing these thresholds are annotated. Only genetic variants with a minor allele frequency greater than 5% are shown.
Table 2. Lead SNPs with p-value < 1 × 10−6 from the GWAS

Note: Lead single-nucleotide polymorphisms (SNPs) with minor allele frequency (MAF) < 5% were removed. The MAF, odds ratio and standard error (SE) correspond to the minor allele. The hg38 human genome build was used.
a Genomewide significance.
Replication Studies
This study failed to replicate (p < .05) any SNPs previously associated (p ≤ 1 × 10–5) with 28-day sepsis mortality/survival and susceptibility to sepsis (Supplementary Tables S3 and S4). SNPs that reached suggestive significance (p ≤ 1 × 10–5) from the UKBB sepsis-related phenotypes also failed to replicate in the present septic shock GWAS (Supplementary Table S4; Rautanen et al., Reference Rautanen, Mills, Gordon, Hutton, Steffens and Nuamah2015; Scherag et al., Reference Scherag, Schöneweck, Kesselmeier, Taudien, Platzer, Felder and Brunkhorst2016; Srinivasan et al., Reference Srinivasan, Page, Kirpalani, Murray, Das and Higgins2017; Neale, Reference Neale2018).
Gene- and Pathway-Based Analyses
The top five gene associations from FastBAT analyses for each outcome are summarized in Table 3. No genes reached the significance threshold (p ≤ 2.5 × 10–6). DEPICT did not identify any genes, pathways, cells or tissue types to be significantly enriched across all the GWAS at false discovery rate <5%; however, the top five genes, pathways and tissues can be found in Supplementary Tables S5, S6 and S7.
Table 3. FastBAT results for the outcomes (A) susceptibility to septic shock, (B) 28-day mortality, (C) 90-day mortality and (D) shock resolution

Note: The top five genes associated with the primary outcomes are tabulated, along with the number of single-nucleotide polymorphisms (SNPs) within the gene region, the gene-based test p-value, the most significant SNP within the region, and the respective p-value from the genomewide association study (GWAS), interesting gene ontology annotations, and previous associations listed within the GWAS Catalog (MacArthur et al., Reference MacArthur, Bowler, Cerezo, Gil, Hall, Hastings and Parkinson2017). The FastBAT analyses were carried out using the Complex-Traits Genetics Virtual Lab (Bakshi et al., Reference Bakshi, Zhu, Vinkhuyzen, Hill, McRae, Visscher and Yang2016; Cuéllar-Partida et al., Reference Cuéllar-Partida, Lundberg, Fang Kho, D’Urso, Gutiérrez-Mondragón, Thanh Ngo and Hwang2019).
Polygenic Risk Score Analysis
PRS derived from GWAS summary statistics for 10 different traits were calculated in the septic shock 28-day survivors and nonsurvivors, as well as in all septic shock patients and controls (Table 4). The p-value threshold for statistical significance after Bonferroni correction for multiple testing is 5.0 × 10–3 (10 phenotypes). There were three significantly associated PRS in the expected direction. PRS for higher hematocrit and granulocyte count were negatively associated with 28-day mortality (p = 3.04 × 10–3 and 2.29 × 10–3). PRS for higher CRP levels were positively associated with susceptibility to septic shock (p = 1.44 × 10–3). For all significant phenotypes, PRS constructed using SNPs that reached the less conservative p-value thresholds were more predictive than PRS constructed using only SNPs that met more conservative thresholds. There were no significant associations with the negative control height.
Table 4. PRS analysis results
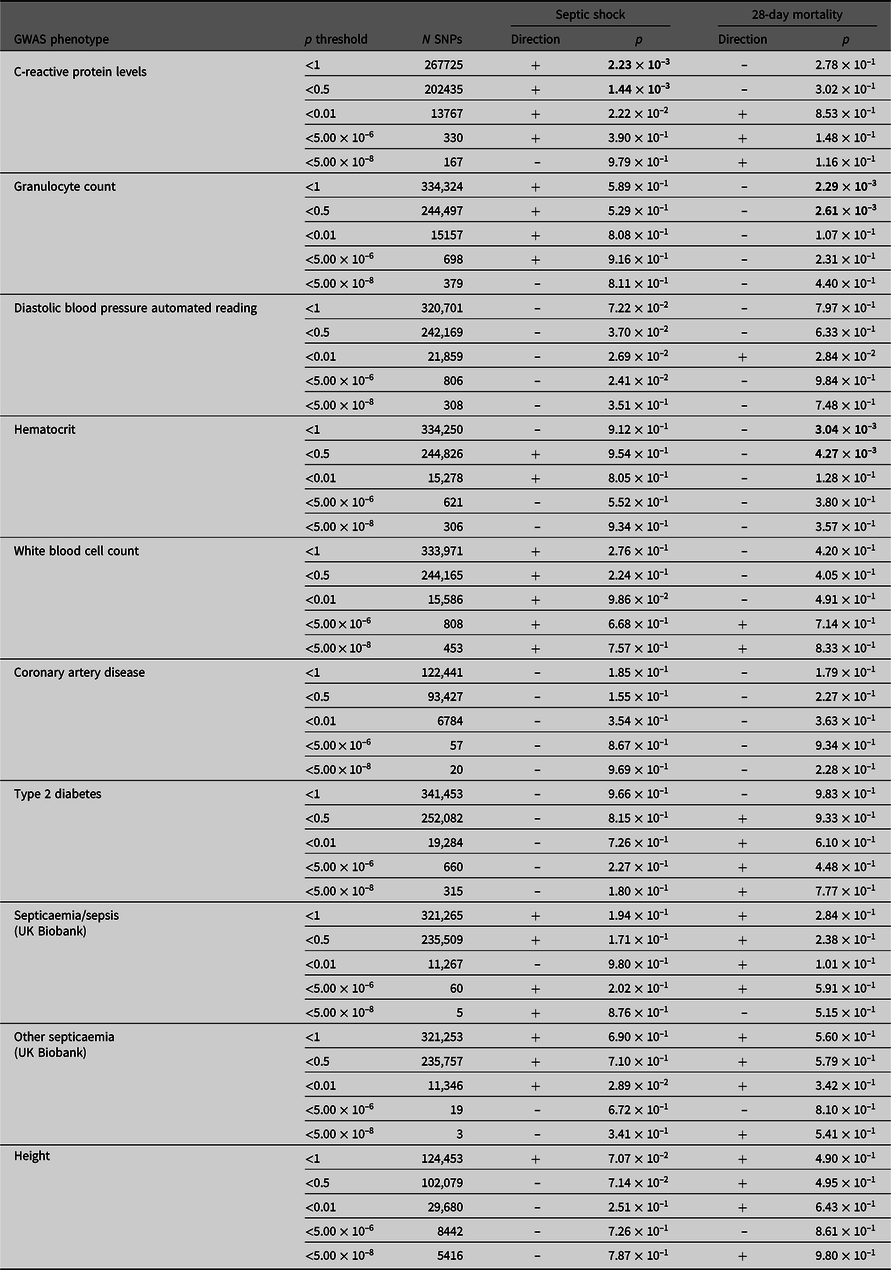
Note: Septic shock patients (ADRENAL-GWAS) and population controls (QIMRB) were scored on their genotypes at single-nucleotide polymorphisms (SNPs; weighted by the reported effect sizes) that reached various p-value thresholds (< 5 × 10–8, 5 × 10–6, 0.01, 0.5 and 1) in nine genomewide association studies (GWAS) for traits of interest. The number of SNPs (N SNPs) included in each analysis is tabulated. Regressions for septic shock and 28-day mortality were performed, with standardized PRS as a predictor, and the top five principal components as covariates (as well as age and sex for 28-day mortality). The direction of the beta coefficient is noted, where a positive coefficient indicates a positive association with the tested phenotype (i.e., increased propensity to septic shock and/or increased risk of mortality at 28 days), and vice versa for negative coefficients. Bolded p-values indicate statistically significant results after Bonferroni × correction for multiple testing (p ≤ 5 ×10−3).
Discussion
The current study presents, to our knowledge, the first report of genetic association analyses and the use of a polygenic risk score analytical approach in an exclusive cohort of patients with septic shock. The robust clinical outcome data were collected systematically in the context of a randomized controlled trial, and the analyses focused on patient-centered outcomes. Comparable genetic studies consist only of small cohorts of patients with sepsis (Rautanen et al., Reference Rautanen, Mills, Gordon, Hutton, Steffens and Nuamah2015; Scherag et al., Reference Scherag, Schöneweck, Kesselmeier, Taudien, Platzer, Felder and Brunkhorst2016; Srinivasan et al., Reference Srinivasan, Page, Kirpalani, Murray, Das and Higgins2017). We attempted to increase the power of the current study by focusing our efforts on the most severe form of sepsis, septic shock, which may involve alleles of larger effect. In addition, we performed a suite of gene-based, pathway-based and polygenic risk score analyses, which have greater statistical power than single locus tests of association.
One SNP, rs9489328, was genomewide significantly associated with susceptibility to septic shock. The rs9489328 SNP lies physically within an uncharacterized noncoding RNA (AL589740.1), with no known biological functions. This variant has not been previously significantly associated with any traits. The SNPs that are in linkage disequilibrium with it are not associated with the phenotype (Supplementary Table S8), hence the lack of ‘peak’ typical of true associations. Although rs9489328 passed all QC steps, it is possible that the association reflects a false-positive and may be a genotyping artifact or a product of batch effects and should be validated with methods such as minisequencing or Taqman. A discussion of the four SNPs (rs368584, rs11167801, rs7698838 and rs17128291), which reached suggestive levels of significance, can be found in the Supplementary Discussion S1. The results from the present GWAS have not identified common variants of large effect contributing to susceptibility to, mortality from and resolution of septic shock.
Despite being adequately powered, we failed to replicate previous SNP associations (which were notably rare variants; see Supplementary Tables S3 and S4) with sepsis in this study. This could be due to variability in the phenotype definitions; although this may be unlikely considering the variants contributing to the sepsis phenotype would likely be enriched in a cohort of septic shock individuals. In addition, the previous studies used controls who had been exposed to sepsis risk factors; while this is ideal, we consider the reduction in power we experienced due to using population controls to be minimal, given the low incidence of sepsis in the population (and likely in our controls) — and not the reason we were unable to replicate previous findings. Instead, the genetic variants prioritized in previous sepsis GWAS may be spurious associations arising from small cohort sizes.
Gene-based analyses failed to identify any genes significantly associated with the tested phenotypes. However, the top results for each analysis consisted of a number of cardiovascular and immune-related genes. Variants within KLB, NINJ2 and SS18 have been previously associated with cardiovascular-related phenotypes (Astle et al., Reference Astle, Elding, Jiang, Allen, Ruklisa, Mann and Soranzo2016; Giri et al., Reference Giri, Hellwege, Keaton, Park, Qiu, Warren and Edwards2019; Ikram et al., Reference Ikram, Seshadri, Bis, Fornage, DeStefano, Aulchenko and Wolf2009; Kanai et al., Reference Kanai, Akiyama, Takahashi, Matoba, Momozawa, Ikeda and Kamatani2018; Kichaev et al., Reference Kichaev, Bhatia, Loh, Gazal, Burch, Freund and Price2019). Likewise, NINJ2 and CMTM7 have been associated with immune phenotypes (Astle et al., Reference Astle, Elding, Jiang, Allen, Ruklisa, Mann and Soranzo2016; Jonsson et al., Reference Jonsson, Sveinbjornsson, de Lapuente Portilla, Swaminathan, Plomp, Dekkers and Stefansson2017). Given the previous associations of variants within these genes with cardiovascular and immune disorders, and observed increased risk of sepsis in individuals with chronic medical conditions, a connection with the pathophysiology of septic shock may be biologically plausible, and may provide possible therapeutic targets given replication and functional follow-up (Wang et al., Reference Wang, Shapiro, Griffin, Safford, Judd and Howard2012).
This study was the first to conduct PRS analyses in a cohort of patients with septic shock, and the results indicate that correlation between septic shock and a number of clinically relevant phenotypes is not only observed at the phenotypic level, as seen in observational studies, but also reflected at the genetic level. Future Mendelian randomization analyses in larger genetic cohorts may help ascertain whether the PRS associations reflect a genetic overlap or a causal relationship (Davey Smith & Ebrahim, Reference Davey Smith and Ebrahim2003). This may overcome confounding if the assumptions are met, and may provide valid targets for therapeutics.
The PRS analyses found increased CRP levels were predictive of increased susceptibility to septic shock. CRP is an acute-phase protein synthesized predominantly by liver cells in response to inflammatory cytokines, mainly interleukin 6, and therefore levels rise during inflammation (Ligthart et al., Reference Ligthart, Vaez, Võsa, Stathopoulou, de Vries, Prins and Alizadeh2018). One interpretation of a significant association between PRS for CRP and septic shock is that increased CRP levels are causally related to an increased risk of septic shock. However, we consider this explanation unlikely to be true because PRS from the most strongly CRP-associated SNPs (i.e., PRS consisting of only genomewide significant variants for CRP) were not associated with septic shock, despite previous research showing genomewide significant SNPs explain more variance in CRP levels than PRS, including SNPs reaching less stringent thresholds (Ligthart et al., Reference Ligthart, Vaez, Võsa, Stathopoulou, de Vries, Prins and Alizadeh2018; Evans et al., Reference Evans, Brion, Paternoster, Kemp, McMahon, Munafò and Smith2013). More likely, the result could reflect a genetic overlap with potential underlying genetic inflammatory disorders that contribute to increased circulating CRP levels, and also increased risk of septic shock (Muller et al., Reference Muller, Gorter, Hak, Goudzwaard, Schellevis, Hoepelman and Rutten2005; Wang et al., Reference Wang, Shapiro, Griffin, Safford, Judd and Howard2012). For example, individuals with a genetic predisposition to increased CRP levels (likely actually reflecting underlying genetic inflammatory disorders which increase CRP levels) may respond to a severe infection with a more pronounced inflammatory response, resulting in progression into shock.
PRS for granulocyte count were negatively associated with 28-day mortality. The direction of the association suggests a genetic predisposition to higher granulocyte count is associated with decreased patient mortality, possibly because of a better immune response and successful clearing of the infection, which is supported in the literature (Bermejo-Martín et al., Reference Bermejo-Martín, Tamayo, Ruiz, Andaluz-Ojeda, Herrán-Monge and Muriel-Bombín2014).
PRS for decreased hematocrit levels were associated with increased 28-day mortality. Although the primary role of red blood cells is the transport of oxygen, they also mediate innate immunity through binding chemokines, pathogens and nucleic acids (Anderson et al Reference Anderson, Brodsky and Mangalmurti2018). Sepsis patients present with high hematocrit due to capillary leak syndrome, and this could be related to an adverse outcome (van Beest et al., Reference van Beest, Hofstra, Schultz, Boerma, Spronk and Kuiper2008). However, the PRS reflect hematocrit in ‘healthy’ individuals, and due to their immune role, a genetic predisposition to decreased red blood cell count (and thus lower hematocrit) could alter the host’s ability to mount an effective immune response.
The main limitation of the current study was a lack of statistical power, primarily due to small sample size caused by the logistical difficulty of collecting biological samples in intensive care settings in a timely manner from a large number of sepsis patients. In addition, power may have been reduced due to disease heterogeneity and misclassification bias. As sepsis is triggered by an environmental cue (i.e., infection), it is possible that some controls would, in fact, have been cases had they been exposed to the relevant environment, or may have already survived a sepsis episode; however, this reduction in power is small given the low incidence of sepsis. While we were underpowered to detect loci of small effect at genomewide levels of significance, our results show common variants of large effect (e.g., variants in the major histocompatibility region that are known to contribute to many immune-mediated diseases) do not contribute to susceptibility to or mortality from septic shock (Evans et al., Reference Evans, Spencer, Pointon, Su, Harvey and Kochan2011).
Although extensive molecular studies into sepsis and septic shock have been performed, genetic analyses have been limited, despite longstanding evidence of a strong genetic component to the disease. Sepsis is likely a complex trait, and hence will be influenced by many genetic variants with small effect. A large meta-analysis would be better powered to detect genetic variants with smaller effects that likely contribute to septic shock mortality or susceptibility, and may permit calculations using LD score regression, and place a lower bound on sepsis heritability (Bulik-Sullivan et al., Reference Bulik-Sullivan, Loh, Finucane, Ripke, Yang and Neale2015).
In conclusion, our polygenic risk score analyses identified several associations between genetic risk scores for clinically relevant variables and septic, shock indicating shared underlying genetic etiology with comorbid traits. This report in an exclusive cohort of patients with established septic shock represents a key step in understanding the genetic basis of septic shock and may inform the debate on future therapeutic targets for the condition.
Supplementary material
To view supplementary material for this article, please visit https://doi.org/10.1017/thg.2020.60.
Acknowledgments
The authors thank participants and their families for their contributions and ongoing support of research. They acknowledge the extensive work carried out by current and former QIMRB staff, particularly the current QIMRB Sample Processing facility (formerly Molecular Epidemiology Lab) for sample processing; former interviewers, IT and project staff for recruitment and data collection; and the use of the QIMRB High Performance Computing facility for data storage and analysis. The authors thank the NHMRC for funding that supported the genotyping of QIMRB controls (grants NHMRC APP1031119 and APP1084325).
Author contributions
D.M.E., M.A.B., B.V., J.M., S.F., J.P. and J.C. conceived the study. J.M., S.F., J.C. and B.V. oversaw the clinical trial, and nurses at the various hospital sites collected the data. E.P., D.R., B.V., D.E. and J.C. managed the ADRENAL-GWAS subset of the project. M.A.B. and E.D.G. performed the ADRENAL-GWAS genotyping. S.E.M., S.D.G. and N.G.M. were involved in sample preparation of the control cohort. S.D. and G.C.P. cleaned the data and performed the statistical analyses. S.D. performed the literature search and prepared all tables and figures. The CHARGE Inflammation Working group and S.L. managed and provided C-reactive protein analyses and aided in interpretation of the results alongside D.M.E., G.C.P., A.B., B.V., J.C. and S.D. D.M.E., G.C.P. and A.B. supervised the work. S.D. prepared the manuscript for submission. All authors reviewed the article and approved the final version.
Financial support
This work was supported by an NHMRC project grant (GNT1085159 to D.M.E., B.V., S.F., J.M. and J.C.). D.M.E. is supported by an NHMRC Senior Research Fellowship (APP1137714). J.P. is supported by a NHMRC Career Development Fellowship (APP1107599). S.D. received a University of Queensland Diamantina Honours scholarship. S.E.M. is supported by an NHMRC Senior Research Fellowship (APP1103623). J.M. is supported by a Level 2 NHMRC Practitioner Fellowship. B.V. is supported by a MRFF Practitioner Fellowship. The funder of the study had no role in study design, data collection, data analysis, data interpretation or writing of the report.
Conflict of interest
The authors declare no competing interests.
Ethical standards
The authors assert that all procedures contributing to this work comply with the ethical standards of the relevant national and institutional committees on human experimentation and with the Helsinki Declaration of 1975, as revised in 2008.