Book contents
- Half title page
- Title page
- Copyright page
- Contents
- Preface
- Book part
- 1 Introduction
- 2 Statistical models and methods
- 3 Decision analysis
- 4 Spatial modeling
- 5 Value of information in spatial decision situations
- 6 Earth sciences applications
- 7 Problems and projects
- Appendix: selected statistical models and sampling methods
- References
- Index
- References
References
Published online by Cambridge University Press: 05 November 2015
- Half title page
- Title page
- Copyright page
- Contents
- Preface
- Book part
- 1 Introduction
- 2 Statistical models and methods
- 3 Decision analysis
- 4 Spatial modeling
- 5 Value of information in spatial decision situations
- 6 Earth sciences applications
- 7 Problems and projects
- Appendix: selected statistical models and sampling methods
- References
- Index
- References
Summary
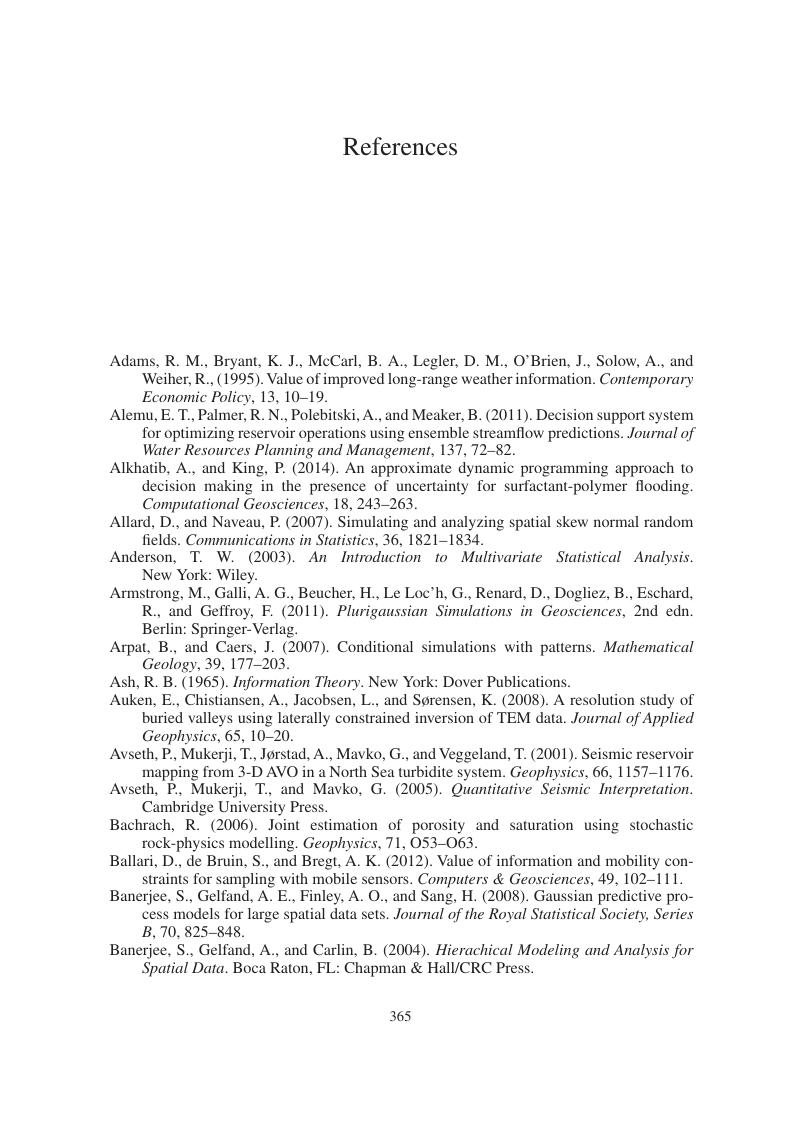
- Type
- Chapter
- Information
- Value of Information in the Earth SciencesIntegrating Spatial Modeling and Decision Analysis, pp. 365 - 377Publisher: Cambridge University PressPrint publication year: 2015