Book contents
- Social Interactions in Virtual Worlds
- Social Interactions in Virtual Worlds
- Copyright page
- Contents
- Contributors
- Introduction
- Part I Individual Behaviors and Dyadic Relationships
- Part II Groups: Norms, Leadership, and Virtual Organizations
- Part III Understanding Culture with Games
- Part IV Techniques for Analyzing Game Data
- 12 The Power of Social Features in Online Gaming
- 13 Profiling in Games: Understanding Behavior from Telemetry
- 14 Using Massively Multiplayer Online Game Data to Analyze the Dynamics of Social Interactions
- References
13 - Profiling in Games: Understanding Behavior from Telemetry
from Part IV - Techniques for Analyzing Game Data
Published online by Cambridge University Press: 15 June 2018
- Social Interactions in Virtual Worlds
- Social Interactions in Virtual Worlds
- Copyright page
- Contents
- Contributors
- Introduction
- Part I Individual Behaviors and Dyadic Relationships
- Part II Groups: Norms, Leadership, and Virtual Organizations
- Part III Understanding Culture with Games
- Part IV Techniques for Analyzing Game Data
- 12 The Power of Social Features in Online Gaming
- 13 Profiling in Games: Understanding Behavior from Telemetry
- 14 Using Massively Multiplayer Online Game Data to Analyze the Dynamics of Social Interactions
- References
Summary
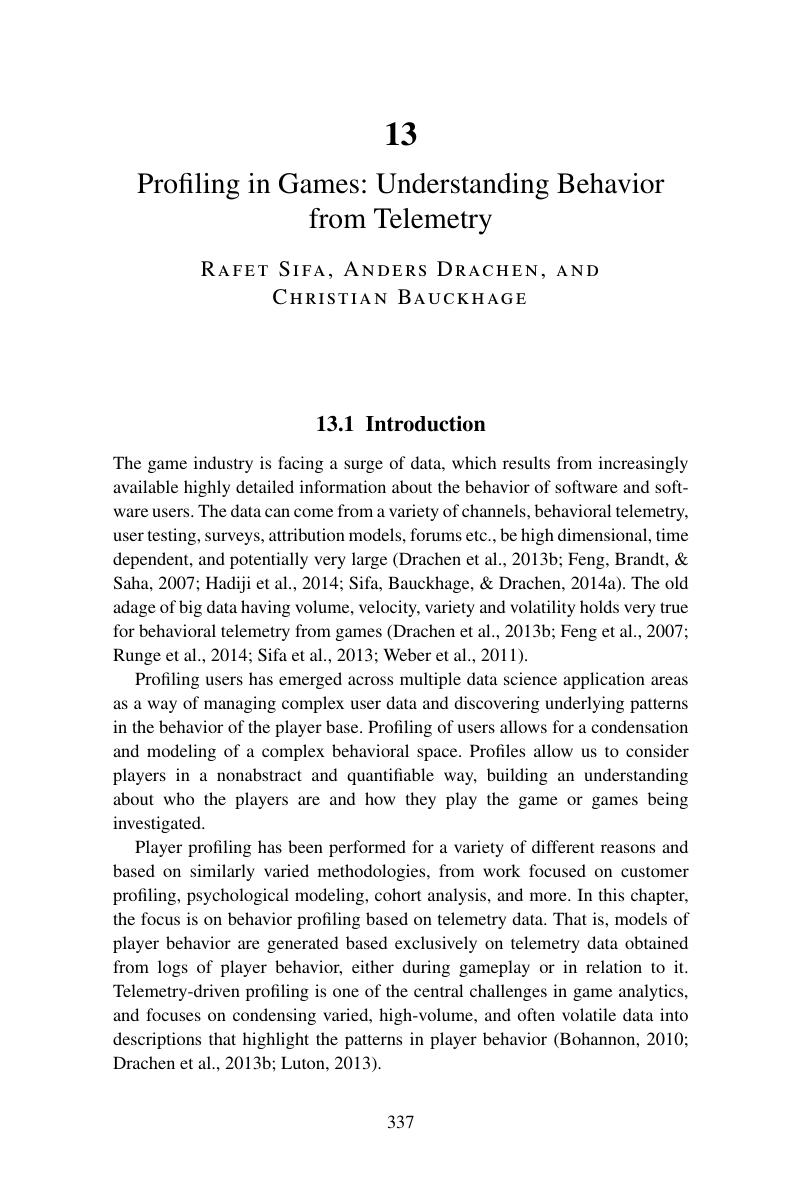
- Type
- Chapter
- Information
- Social Interactions in Virtual WorldsAn Interdisciplinary Perspective, pp. 337 - 374Publisher: Cambridge University PressPrint publication year: 2018
References
- 14
- Cited by