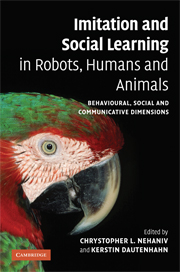
Book contents
- Frontmatter
- Contents
- List of plates
- List of figures
- List of tables
- List of contributors
- Introduction: the constructive interdisciplinary viewpoint for understanding mechanisms and models of imitation and social learning
- Part I Correspondence problems and mechanisms
- Part II Mirroring and ‘mind-reading’
- Part III What to imitate?
- Part IV Development and embodiment
- Part V Synchrony and turn-taking as communicative mechanisms
- Part VI Why imitate? – Motivations
- Part VII Social feedback
- Part VIII The ecological context
- Index
- Plate section
Part III - What to imitate?
Published online by Cambridge University Press: 10 December 2009
- Frontmatter
- Contents
- List of plates
- List of figures
- List of tables
- List of contributors
- Introduction: the constructive interdisciplinary viewpoint for understanding mechanisms and models of imitation and social learning
- Part I Correspondence problems and mechanisms
- Part II Mirroring and ‘mind-reading’
- Part III What to imitate?
- Part IV Development and embodiment
- Part V Synchrony and turn-taking as communicative mechanisms
- Part VI Why imitate? – Motivations
- Part VII Social feedback
- Part VIII The ecological context
- Index
- Plate section
Summary
Social learning and behaviour matching are prevalent and important in learning by animals and humans. But as we saw in the section on correspondence problems and mechanisms, there are many possibilities for what aspects of an observed behaviour an individual may attempt to match. This is the problem of ‘what to imitate?’ and has been identified as amongst the big five problems for the study of social learning (Dautenhahn and Nehaniv, 2002). This is of course closely linked to the question ‘why imitate?’ as it potentially requires discussion of the internal motivational states that cannot be directly observed by the social learner (see Part VI, Why imitate? – Motivations, this volume).
In this section, three very different constructive approaches toward solving this problem are put forth by ethologists Malinda Carpenter and Josep Call, roboticists Sylvain Calinon and Aude Billard, and artificial intelligence researchers Tony Belpaeme, Bart de Boer and Bart Jansen.
Malinda Carpenter and Josep Call approach the problem of what to imitate in artificial constructed systems from an ethologist's viewpoint. With reference to studies of primates, especially human children, and other animals including birds and dolphins, they present an illuminating survey of several different ways in which an observer might infer the goals or sub-goals of an observed behaviour.
- Type
- Chapter
- Information
- Imitation and Social Learning in Robots, Humans and AnimalsBehavioural, Social and Communicative Dimensions, pp. 131 - 134Publisher: Cambridge University PressPrint publication year: 2007