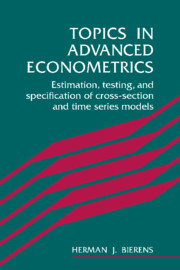
Book contents
- Frontmatter
- Contents
- Preface
- 1 Basic probability theory
- 2 Convergence
- 3 Introduction to conditioning
- 4 Nonliner parametric regression analysis and maximum likelihood theory
- 5 Tests for model misspecification
- 6 Conditioning and dependence
- 7 Functional specification of time series models
- 8 ARMAX models: estimation and testing
- 9 Unit roots and cointegration
- 10 The Nadaraya–Watson kernel regression function estimator
- References
- Index
6 - Conditioning and dependence
Published online by Cambridge University Press: 28 October 2009
- Frontmatter
- Contents
- Preface
- 1 Basic probability theory
- 2 Convergence
- 3 Introduction to conditioning
- 4 Nonliner parametric regression analysis and maximum likelihood theory
- 5 Tests for model misspecification
- 6 Conditioning and dependence
- 7 Functional specification of time series models
- 8 ARMAX models: estimation and testing
- 9 Unit roots and cointegration
- 10 The Nadaraya–Watson kernel regression function estimator
- References
- Index
Summary
Time series models usually aim to represent, directly or indirectly, the conditional expectation of a time series variable relative to the entire past of the time series process involved. The reason is that this conditional expectation is the best forecasting scheme; best in the sense that it yields forecasts with minimal mean square forecast error. The concept of a conditional expectation relative to a one-sided infinite sequence of “past” variables cannot be made clear on the basis of the elementary notion of conditional expectation known from intermediate mathematical-statistical textbooks. Even the more general approach in chapter 3 is not suitable. What we need here is the concept of a conditional expectation relative to a Borel field. We shall discuss this concept and its consequences (in particular martingale theory) in section 6.1. In section 6.2 we consider various measures of dependence as some, though rather weak, conditions have to be imposed on the dependence of a time series process to prove weak (uniform) laws of large numbers. These weak laws are the topics of sections 6.3 and 6.4.
Throughout we assume that the reader is familiar with the basic elements of linear time series analysis, say on the level of Harvey's (1981) textbook.
Conditional expectations relative to a Borel field
Definition and basic properties
In section 3.1 we have defined the conditional expectation of a random variable Y relative ∣ to a random vector X ε Rk as a Borel measurable real function g on Rk such that
E[Y–g(X)]ψ(X) = 0
for all bounded Borel measurable real functions ψ on Rk.
- Type
- Chapter
- Information
- Topics in Advanced EconometricsEstimation, Testing, and Specification of Cross-Section and Time Series Models, pp. 110 - 134Publisher: Cambridge University PressPrint publication year: 1994