References
Published online by Cambridge University Press: 07 November 2024
Summary
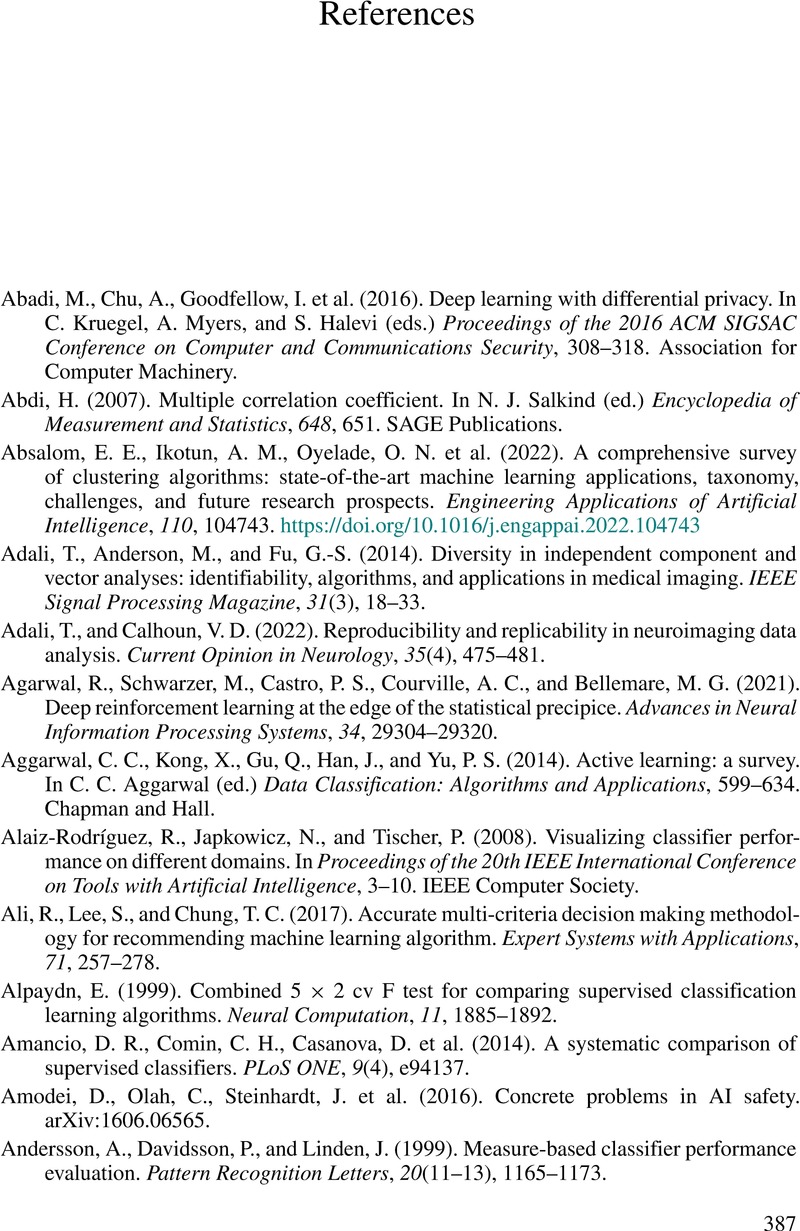
- Type
- Chapter
- Information
- Machine Learning EvaluationTowards Reliable and Responsible AI, pp. 387 - 402Publisher: Cambridge University PressPrint publication year: 2024