Book contents
- Frontmatter
- Dedication
- Contents
- Preface
- 1 Nonparametric Statistical Models
- 2 Gaussian Processes
- 3 Empirical Processes
- 4 Function Spaces and Approximation Theory
- 5 Linear Nonparametric Estimators
- 6 The Minimax Paradigm
- 7 Likelihood-Based Procedures
- 8 Adaptive Inference
- References
- Author Index
- Index
- References
References
Published online by Cambridge University Press: 05 December 2015
- Frontmatter
- Dedication
- Contents
- Preface
- 1 Nonparametric Statistical Models
- 2 Gaussian Processes
- 3 Empirical Processes
- 4 Function Spaces and Approximation Theory
- 5 Linear Nonparametric Estimators
- 6 The Minimax Paradigm
- 7 Likelihood-Based Procedures
- 8 Adaptive Inference
- References
- Author Index
- Index
- References
Summary
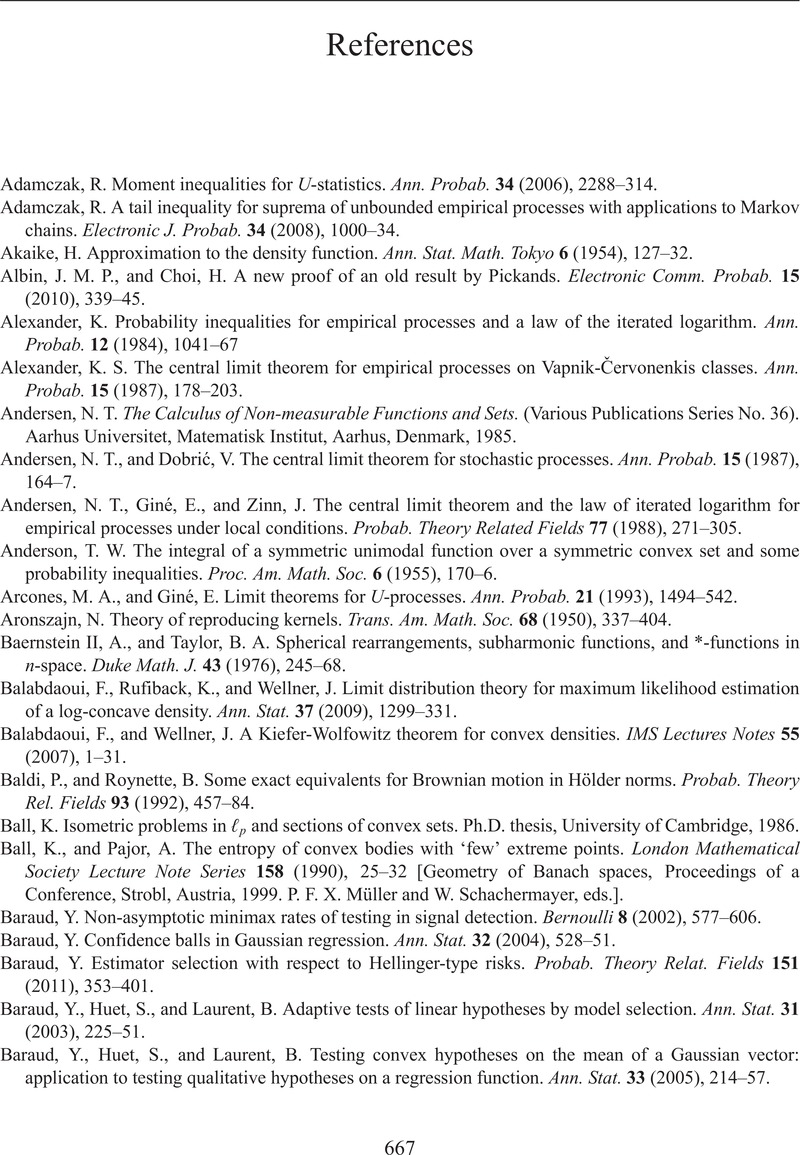
- Type
- Chapter
- Information
- Publisher: Cambridge University PressPrint publication year: 2015