Breast milk, either from an infant's own mother or from another woman, can be assumed to have been the sole source of nourishment for infants during most of human evolution, having evolved as an adaptation to transfer immune factors to offspring and to space births (Sellen, Reference Sellen2007). It has been suggested that breastfeeding duration in prehistoric times was of two–three years and that from the Middle Ages to the 19th century, most infants in Europe were typically weaned between the ages of one and two years (Schön & Silvén, Reference Schön and Silvén2007). It was not until the end of the 19th century, that is, in our recent history, that artificial feeding became a safe alternative to breast milk, and breastfeeding became dependent on the mother's choice in most milieus. Consequently, nowadays a large variability in this behavior can be observed both within and between countries, with global statistics showing that 37% of infants were exclusively breastfed until six months age in 2007 (World Health Organization, 2013), which is the current recommended minimum weaning age (World Health Organization & United Nations Children's Fund, 2003). This percentage varied considerably across countries; for example, 18.8% in the United States in 2011 (Centers for Disease Control and Prevention, 2014), 17.6% in Australia in 2011–2012 (Australian Bureau of Statistics, 2013) and 28.5% in Spain in 2011–2012 (Ministerio de Sanidad, Servicios Sociales e Igualdad, 2012). The instruments for data collection vary between countries, for example, in being explicit about the inclusion of expressed milk in exclusive breastfeeding, so some rates could be higher.
Given the positive impact of breastfeeding on infants’ and mothers’ health (Ip et al., Reference Ip, Chung, Raman, Chew, Magula, DeVine and Lau2009; World Health Organization & United Nations Children's Fund, 2003), there is a current interest in determining which factors are related to women's decisions regarding the method of feeding their infants, that is, the predispositions or situations that make them more likely to initiate breastfeeding or bottle feeding, and the duration of breastfeeding. Breastfeeding is a biocultural behavior (Holman & Grimes, Reference Holman and Grimes2003) and is influenced by multiple factors (Thulier & Mercer, Reference Thulier and Mercer2009), such as the action of prolactin and oxytocin (Heinig & Dewey, Reference Heinig and Dewey1997), pain from nipple trauma, mastitis, maternal exhaustion and perceived poor milk supply (Almqvist-Tangen et al., Reference Almqvist-Tangen, Bergman, Dahlgren, Roswall and Alm2012; Spencer, Reference Spencer2008), babies’ sucking dynamics (Sakalidis et al., Reference Sakalidis, Williams, Garbin, Hepworth, Hartmann, Paech and Geddes2013), body mass index (Wojcicki, Reference Wojcicki2011), level of education (Colodro-Conde et al., Reference Colodro-Conde, Sánchez-Romera, Tornero-Gómez, Pérez-Riquelme, Polo-Tomás and Ordoñana2011), working conditions (Calnen, Reference Calnen2010), psychological factors, personality, self-efficacy, anxiety (Brown, Reference Brown2014; Colodro-Conde et al., in press (a); Colodro-Conde et al., in press (b); De Jager et al., Reference De Jager, Hartley, Terrazas and Merrill2012; Li et al., Reference Li, Kendall, Henderson, Downie, Landsborough and Oddy2008; McFadden & Toole, Reference McFadden and Toole2006; Wagner et al., Reference Wagner, Wagner, Ebeling, Chatman, Cohen and Hulsey2006), support from partner, family and peers (Thulier & Mercer, Reference Thulier and Mercer2009), social norms (Swanson & Power, Reference Swanson and Power2005), and advice from health professionals (Brown et al., Reference Brown, Raynor and Lee2011).
The magnitude of the genetic and environmental sources of variance in breastfeeding behavior were explored in a previous study in a cohort of Spanish twins from the Murcia Twin Registry (Ordoñana et al., Reference Ordoñana, Perez-Riquelme, Gonzalez-Javier, Carrillo, Gomez-Amor and Martinez-Selva2006, Reference Ordoñana, Rebollo-Mesa, Carrillo, Colodro-Conde, García-Palomo, González-Javier and Pérez-Riquelme2013). The best-fitting models explained the observed variance through additive genetic and non-shared environmental factors for initiation and duration in the first-born child and the average for the complete offspring, with heritability ranging between 44% and 54% (Colodro-Conde et al., Reference Colodro-Conde, Sánchez-Romera and Ordoñana2013). It was hypothesized that among the genetic factors influencing breastfeeding behavior, those related to the regulation of hormone production could play a part in breastfeeding outcomes. In support of this hypothesis, Jonas et al. (Reference Jonas, Mileva-Seitz, Girard, Bisceglia, Kennedy, Sokolowski and Steiner2013) found that the single nucleotide polymorphism (SNP) rs2740210 in the oxytocin peptide gene was associated with exclusive breastfeeding at three and six months postpartum. Other authors, however, did not find a relationship between selected oxytocin receptor SNPs and breastfeeding (Tharner et al., Reference Tharner, Luijk, Raat, IJzendoorn, Bakermans-Kranenburg, Moll and Tiemeier2012). As breastfeeding has been suggested to be significantly influenced by genetic factors, it would be interesting to explore the genetic variants underlying this trait.
Several studies have concluded that body mass index is associated with breastfeeding behavior, so mothers who are overweight or obese are less likely to initiate lactation, and are prone to early cessation of breastfeeding (Jevitt et al., Reference Jevitt, Hernandez and Groër2007; Wojcicki, Reference Wojcicki2011). A study with health professionals showed that from their experience, initiating was more difficult than continuing breastfeeding for women with large breasts and/or obesity (Katz et al., Reference Katz, Nilsson and Rasmussen2010). The link between overweight, elevated serum testosterone concentration and pathologies such as the polycystic ovary syndrome could be underlying this relationship (Balen et al., Reference Balen, Conway, Kaltsas, Techatraisak, Manning, West and Jacobs1995; Barber et al., Reference Barber, McCarthy, Wass and Franks2006). Heritability of breast cup size has been estimated to be 56%, and one third of this variance was shared with body mass index (Wade et al., Reference Wade, Zhu and Martin2010). Some genetic variants associated with breast size also influence breast cancer risk (Eriksson et al., Reference Eriksson, Benton, Do, Kiefer, Mountain, Hinds and Tung2012).
This article has three main objectives. First, it aims to replicate previous findings related to heritability of breastfeeding by exploring the magnitude of the genetic and environmental influences in the variation of breastfeeding behavior in a sample of Australian twins. For that aim, we used a twin design, calculating twin correlations for breastfeeding and fitting an ACE variance components model. Second, in order to detect possible genetic variants related to breastfeeding behavior, we conducted a GWAS of this phenotype, using data collected from this large community-based cohort of Australian twins. Lastly, we report the results of a polygenic risk score analysis examining whether an individual's number of genetic variants predisposing to breast size are associated with breastfeeding.
Materials and Methods
Participants
Participants in this study were 3,364 female twin mothers from the QIMR health and lifestyle studies Cohorts I and II with data on breastfeeding. Women in Cohort I were born between 1892 and 1963 (n = 3,205) and women in Cohort II were born between 1,964 and 1971 (n = 419). At the time of the survey (1988–93), the mean age was 43.41 years (SD = 12.32, range = 25–86 years), for women in Cohort I and 24.81 years (SD = 1.87, range = 19–29 years) for women in Cohort II. Further details of the sample, data collection and zygosity determination are described elsewhere for cohort I (Heath et al., Reference Heath, Bucholz, Madden, Dinwiddie, Slutske, Bierut and Martin1997) and for cohort II (Knopik et al., Reference Knopik, Heath, Madden, Bucholz, Slutske, Nelson and Martin2004).
The sample comprised 992 complete twin pairs: 629 MZ and 363 DZ, and 1,380 individual twins from incomplete pairs (411 MZ and 347 DZ from female-female pairs, 621 from female-male pairs and 5 of unknown zygosity). Genome-wide genotypic data were available for 1,521 of the 3,364 individuals.
Procedure
In 1988–1993, participants of both cohorts completed a mailed questionnaire including information about their childbearing. Some of these who did not complete the mailed questionnaire were interviewed over the telephone in 1989–1992. A small subsample of participants of Cohort I (n = 341) was re-surveyed two years after their initial contact to establish the test-retest reliability of the interview measures.
As part of follow-up studies, blood samples were collected from the majority of participants in Cohorts I and II. DNA samples were genotyped using the Illumina 317, 370 and 610 SNP chips. Following quality control, the data were imputed using the subset of ~281,000 markers, which were available across all chips. The genotypes were phased using MACH, then imputed (including the X chromosome) using Minimac based on the 283 European reference individuals in the March 2013 release of phased haplotype from the 1000 Genomes project. In the analyses presented here, we used data from 6.59 M imputed SNPs that passed quality control (R 2 > 0.3 and minor allele frequency > 1%) for association analysis. Details of SNP typing, quality control, data cleaning (including ancestry exclusion) and analysis are given in detail elsewhere (Medland et al., Reference Medland, Nyholt, Painter, McEvoy, McRae, Zhu and Henders2009).
This study was approved by the Queensland Institute of Medical Research Human Research Ethics Committee and the storage of the data follows national regulations regarding personal data protection. All of the participants provided informed consent.
Measures
Data were based on retrospective self-reports. All the participants were asked about details of the birth of up to eight children, including birth complications, time of birth, birth weight, hours of labor, feelings of depression and type of feeding in the first month (see Appendix). The variable we analyzed was the mean number of months they breastfed each child, averaged across all live births and standardized to a Z-score.
Data Analysis
Twin modelling
Data preparation and descriptive and preliminary analyses were performed in SPSS v.19 (SPSS, 2010). Assumptions of the twin design were checked, including the homogeneity of the means and variances of first- and second-born twins and across zygosity groups. Further details of the twin design, including checking at assumptions, can be found elsewhere (Neale & Cardon, Reference Neale and Cardon1992; Posthuma et al., Reference Posthuma, Beem, de Geus, van Baal, von Hjelmborg, Iachine and Boomsma2003).
Statistical analyses employed full information maximum-likelihood modelling (FIML) procedures using the statistical package Mx (Neale et al., Reference Neale, Boker, Xie and Maes2006). In FIML, both complete and incomplete pairs of twins can be used in the analyses and the goodness-of-fit of a model to the observed data is distributed as chi-square (χ2). By testing the change in chi-square (Δχ2) against the change in degrees of freedom (Δdf), we can test whether dropping or equating specific model parameters significantly worsens the model fit. The best-fitting model was chosen in each case by deducting the residual deviance of the compared models and by comparing Akaike's information criterion (AIC).
In a first step, we determined twin pair correlations per zygosity group for breastfeeding. We tested whether MZ correlations were higher than those of DZ twin pairs, which would suggest a genetic influence on individual differences in this trait. Then, variance component model-fitting was conducted to partition the variation in breastfeeding into genetic, shared environmental and non-shared environmental influences. Observed MZ and DZ twin correlations generally reflect a combination of A, C, D, and E influences, and structural equation modeling determines the combination that best matches the observed data (Posthuma et al., Reference Posthuma, Beem, de Geus, van Baal, von Hjelmborg, Iachine and Boomsma2003). Age at the survey time was modelled as covariate in all the analyses.
SNP-based genome-wide association study
One thousand genome imputed dosage data were tested for association with breastfeeding using the additive family-based association test in Merlin-off line (Chen & Abecasis, Reference Chen and Abecasis2007). Correction for age at the survey time was performed by fitting the covariate in the regression model. We adopted a genome-wide significance level for the association between SNP and phenotype of 5×10−8 or smaller to correct for the total number of independent tests (Dudbridge & Gusnanto, Reference Dudbridge and Gusnanto2008).
Gene-based analysis
A gene-based test, Versatile Gene-based Association Study (VEGAS), designed for use with GWAS data with related individuals (Liu et al., Reference Liu, Mcrae, Nyholt, Medland, Wray, Brown and Martin2010), was conducted to determine the level of association across the gene while correcting for linkage disequilibrium (LD) and gene size. Details of this procedure are summarized elsewhere (Verweij et al., Reference Verweij, Zietsch, Medland, Gordon, Benyamin, Nyholt and Madden2010). In brief, this test explores association of each gene (including 50 Kb up and down of the coding region) taking into account the p values of all SNPs (after first pruning out those in high LD) (r 2 > 0.20)), and the LD between them. A p value below α = 2.8×10−6 was considered to be genome-wide significant as the gene-based association test included 17,585 genes (0.05/17,585 genes).
Polygenic study
To examine a potential shared genetic etiology between breastfeeding and breast size, we tested the effects of SNPs previously associated with breast size through a polygenic risk scoring approach. This took the top 2,079 SNPs associated with breast size in a previous GWAS in an independent sample (Eriksson et al., Reference Eriksson, Benton, Do, Kiefer, Mountain, Hinds and Tung2012) at a p value of ≤.0001. These were then clumped within PLINK (Purcell et al., Reference Purcell, Neale, Todd-Brown, Thomas, Ferreira, Bender and Daly2007) to correct for LD within the QIMR sample (R 2 < 0.2 in 250 kb windows), identifying 66 overlapping independent SNPs associated with breast size. A polygenic score was generated using PLINK weighting on the beta for each SNP. This score was then tested for association with breastfeeding phenotypes, correcting for covariates of five principal components. Variance explained by this score was derived as that of a model including the polygenic score and covariates minus the variance explained by a model including only covariates as predictors of breastfeeding.
Results
Descriptive Results
As stated before, our sample is entirely comprised of mothers who reported data about breastfeeding. The mean number of children was 2.52 (SD = 1.26, range = 1–12). Three out of four (75.2%) women breastfed for at least 1 month for each one of their births. The mean duration of breastfeeding was 5.31 months (SD = 4.69, range: 0–48), with slight changes according to child order (Table 1). Table 2 presents the correlation matrix for the duration of breastfeeding for the first five children. Breastfeeding reports showed a high test–retest reliability (r = 0.96, p < .001) and internal consistency among breastfeeding durations (Cronbach's α = 0.98; ICC = 0.85).
TABLE 1 Breastfeeding Duration (Months) According to Childbirth Order

TABLE 2 Correlations (N) for Breastfeeding Duration (Months) in the First Five Children

Spearman's rho (N). *p < .01.
Twin Correlations and Variance Component Model-Fitting
The MZ twin correlation for the mean breastfeeding duration (r MZ = 0.52, 95% CI 0.46–0.57) was larger than for DZ twins (r DZ = 0.35, 95% CI 0.25–0.43), which suggests the presence of genetic influences (see Figure 1). Lower correlations were found for the breastfeeding duration of the first-born child (r MZ = 0.41, 95% CI 0.34–0.47; r DZ = 0.27, 95% CI .17–0.37).
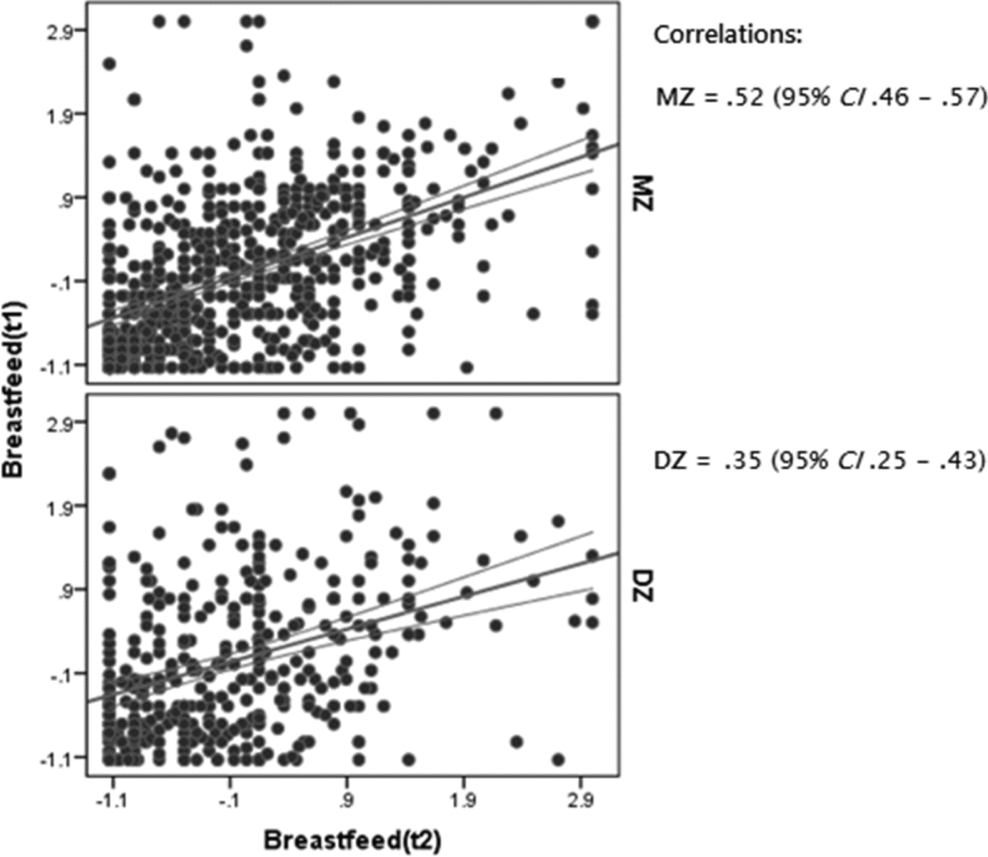
FIGURE 1 Scatter plot of twin correlations with 95% confidence intervals (CI) for breastfeeding duration.
Note. t1: twin 1, t2: twin 2
A univariate model was fit to disentangle the sources of variance of the mean breastfeeding duration (see Table 3). Since the DZ twin correlation was more than half the MZ twin correlation, C was estimated instead of D. The best-fitting model was the one that included additive genetic and non-shared environmental sources of variation — shared environmental factors did not account for a significant portion of variation. Additive genetic factors accounted for 53% in breastfeeding (95% CI 47%–58%) and the remaining 47% was due to unique environmental factors (95% CI 42%–53%). The reduced model fit well, as it did not differ significantly from the full ACE model. The model-fitting analysis revealed a similar structure of the underlying variance for the breastfeeding duration in the first-born child, though with lower heritability (0.42 vs. 0.53 for average duration).
TABLE 3 Model-Fitting Results for Univariate Models for Breastfeeding Mean Duration and Proportions of Variance Explained By Additive Genetic Influences (A), Common Environment (C) and Unique Environment (E)

A: additive genetic factors, AIC: Akaike's information criterion, BF: breastfeeding mean duration across all births, C: common environmental factors, CI: confidence interval, df: degrees of freedom, E: unique environmental factors, -2LL: twice negative log-likelihood, ∆χ2: difference in χ2 to saturated model, ∆df: difference in degrees of freedom to saturated model. Bold type indicates best-fitting model.
Genome-Wide Association Study
We conducted a GWAS of breastfeeding in 1,521 individuals from 1,073 Australian families. The average age of the genotyped sample was 46.46 years (SD = 11.38). Breastfeeding mean duration in this subsample was 5.52 months (SD = 4.75). We tested 6.59 M SNPs for association with breastfeeding, correcting for age at the time of survey.
The Manhattan plot of association p values for 6.59 M SNPs is shown in Figure 2. While no SNP achieved genome-wide significance (p < 6.6*10−8), regions of suggestive association signals, with the smallest p value of 1.2*10−7 obtained for a SNP (rs6950451) were observed on chromosome 7. Suggestive association signals were also detected in chromosomes 2 (SNP rs930421) and 18 (rs9807759), with p values of 1.2*10−6 in both cases.
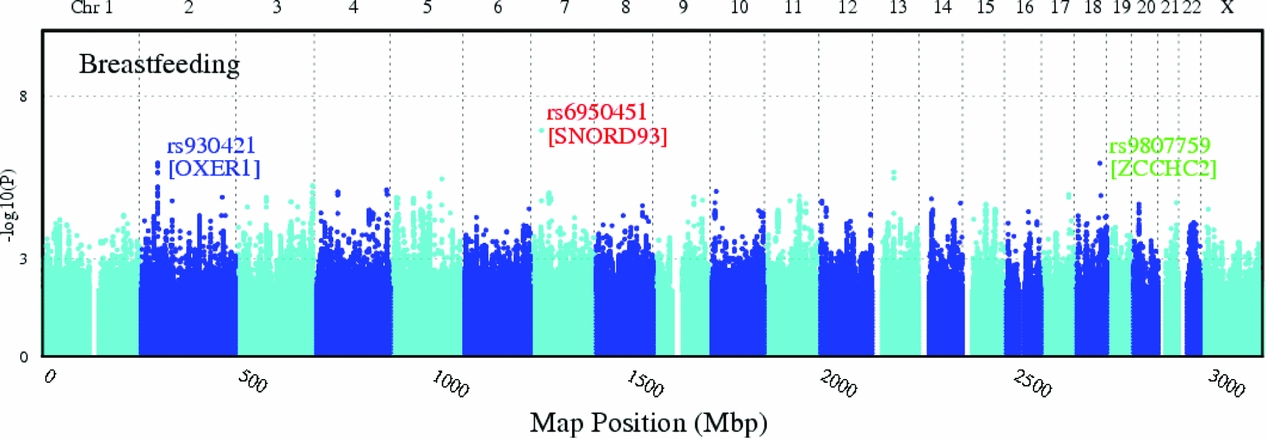
FIGURE 2 Manhattan plot showing the results of the genome-wide association analyses for breastfeeding. Genes at or nearby best SNPs are indicated. The vertical axis shows the -log10 of the associated p values and the horizontal axis shows the chromosome numbers divided into 22 autosomes and the X chromosome.
The quantile-quantile plot (Q-Q plot) of the observed versus expected (under the null-hypothesis of no association) log10 (p value) from the association analysis is presented in Figure 3. The genomic control l (1.007) was close to 1.0, indicating that there was no evidence for inflation of the test statistics or a bias because of possible population stratification in the results (Bacanu et al., Reference Bacanu, Devlin and Roeder2000) and that the family-based association model had correctly accounted for relatedness.

FIGURE 3 Quantile–quantile plot for breastfeeding mean duration. The horizontal axis shows the -log10 of expected p values of association from a 1 degree of freedom chi-square distribution and the vertical axis shows the -log10 of p values from the observed chi-square distribution. The colored dots represent the top hit SNPs. Genes at or nearby best SNPs are indicated.
The top SNPs and gene regions from GWAS analysis with the strongest association with breastfeeding are shown in Table 4.
TABLE 4 Top Ten SNPs and Potential Candidates and Their Gene Regions From GWAS Analysis Showing the Strongest Associations With Breastfeeding

Figure 4 shows the locus zoom plots of the unconditioned analyses for the top five SNPs, rs6950451, rs930451, rs9807759, rs17381960, rs7446359, and also for rs498793, since there are genes at or nearby this latter SNP that have been emphasized for their role in the composition of fatty acids in mothers’ milk (Glaser et al., Reference Glaser, Lattka, Rzehak, Steer and Koletzko2011; Standl et al., Reference Standl, Sausenthaler, Lattka, Koletzko, Bauer, Wichmann and Schaaf2012). Notably, although LD structure surrounding the chromosome 7 locus is rather sparse, rs6950451 was well imputed (R2 = 0.84). Conditional analyses on the top SNP were also performed, and there were no associated SNPs with p < .001.
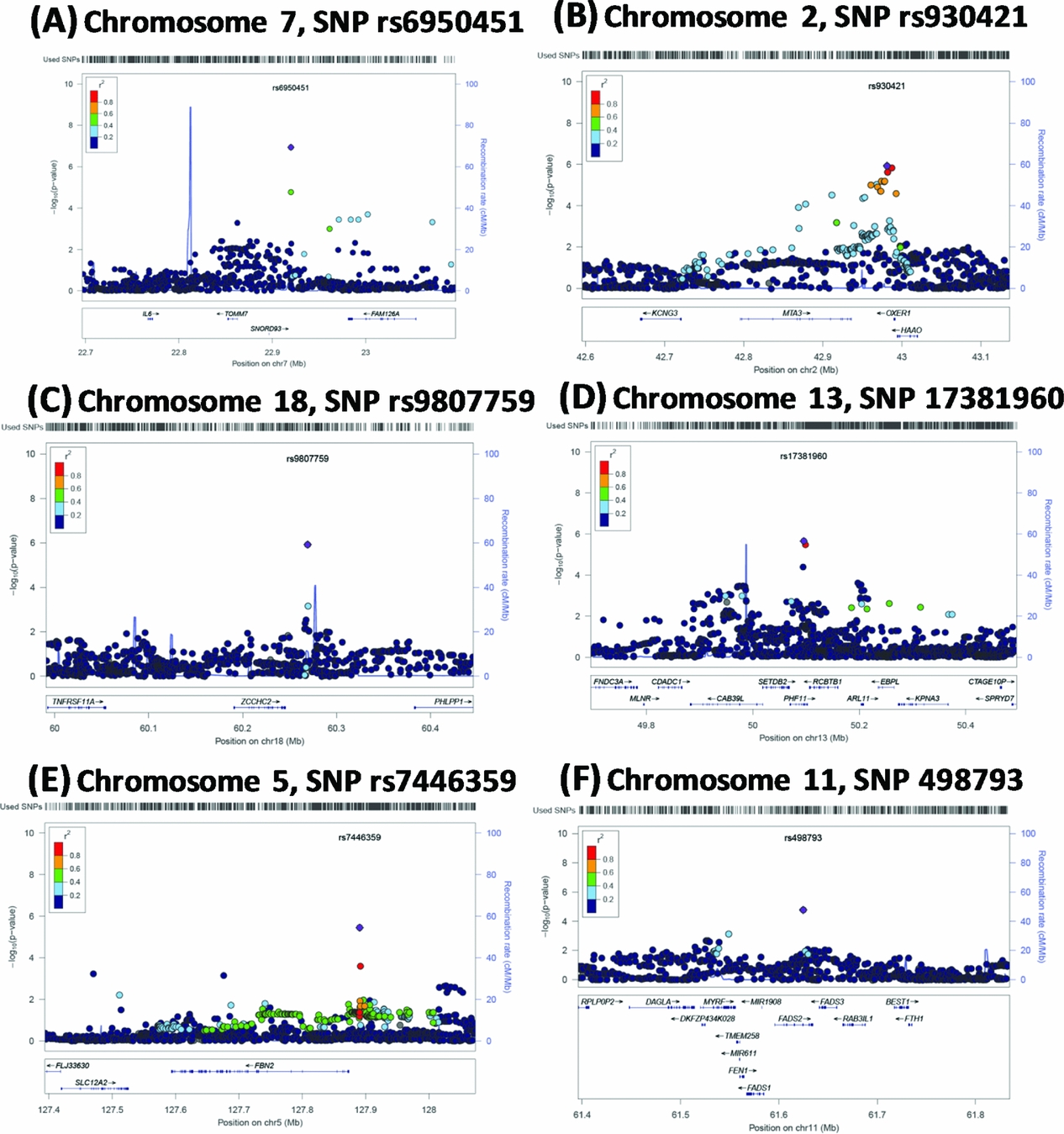
FIGURE 4 Regional association plots for breastfeeding. (A), showing the top associated SNP rs6950451 (p = 1.2×10−7) on chromosome 7; (B-F), showing the possible associated regions; on chromosomes 2, 5, 11, 13 and 18.
Gene-Based Analysis
Gene-based test results are shown in Table 5. Although none of the genes reached genome-wide significance, we list the top 20 genes. Two of these (MRAs and OXER1) have already been noted in the top SNP analysis (Table 4), but the other genes listed are all novel.
TABLE 5 Genes (p < 5×10−3) From VEGAS Gene-Based Analysis Showing the Strongest Associations With Breastfeeding

Polygenic Study
Next, we tested an association between the polygenic score for breast size and we found no significant associations between a polygenic score of genetic predisposition to breast size and breastfeeding among the 66 SNPs with p < 10−4 available (β = 0.011, r2 = 0.003, p = 0.67).
Discussion
In this investigation, we used a broad behavioral genetics approach to analyze individual differences in breastfeeding. The first objective was to explore the proportion of genetic and environmental sources in the variation of breastfeeding. Correlations were higher for MZ twins than for DZ twins, suggesting the presence of genetic factors implicated in this behavior. The results of the twin analysis showed that the best-fitting model was the one including additive genetics effects and unique environmental factors, explaining 53% (CI 95%: 0.47–0.58) and 47% (CI 95%: 0.42–0.53) of the variance in breastfeeding behavior, respectively. The results are also compatible with the full model, in which 17% (CI 95%: 0–0.35) of variance was accounted for by common environmental factors. The values obtained here are very similar to those found recently in a Spanish sample (Colodro-Conde et al., Reference Colodro-Conde, Sánchez-Romera and Ordoñana2013), where 54% of the variance of breastfeeding duration of all the offspring was due to additive genetic factors and the remaining 46% to unique environmental factors. Despite the samples coming from two distinct cultural backgrounds (Australia vs. Spain) and measures being different (quantitative vs. ordinal), the similar results obtained confirm that genetic factors are an important source of variation between women's breastfeeding behavior. While we have not been able to detect any significant effect of shared environmental factors, this may be because of a lack of power due to sample size and our results, which are compatible with a C2 as high as 35%.
Confirmation that genetic factors influence breastfeeding behavior justified our exploring the genetic variants underlying this trait by GWAS, as is discussed below.
The present study is, to our knowledge, the first to perform a GWAS on breastfeeding behaviors. No genome-wide significant SNPs were identified. However, although not significant, we found a promising genetic region on chromosome 7, around rs6950451. Likewise, the VEGAS gene-based analysis did not find any significant results. In addition, we detected suggestive associations of some genetic regions with plausible links to breastfeeding. Among them, the oxoeicosanoid (OXE) receptor 1 gene (p = 1.2*10−3) in chromosome 2 is a receptor for eicosanoids and polyunsaturated fatty acids (Rebhan et al., Reference Rebhan, Chalifa-Caspi, Prilusky and Lancet1997), which are present in breast milk (Koletzko et al., Reference Koletzko, Lien, Agostoni, Böhles, Campoy, Cetin and Forsyth2008).
It is well known that breast milk provides a unique supply of long chain polyunsaturated fatty acids and that their synthesis is controlled by key enzymes encoded by the FADS gene cluster (Marquardt et al., Reference Marquardt, Stöhr, White and Weber2000; Martin et al., Reference Martin, Benyamin, Hansell, Montgomery, Martin, Wright and Bates2011). In this work, we found a suggestive association of the FADS1 (p = 2.3*10−2), FADS2 (p = 3.9*10−2) and FADS3 (p = 4*10−2) genes, on chromosome 11 (Figure 4, panel F), with breastfeeding. Several studies have shown strong associations between the FADS gene cluster and fatty acid levels in breast milk (Glaser et al., Reference Glaser, Lattka, Rzehak, Steer and Koletzko2011; Standl et al., Reference Standl, Sausenthaler, Lattka, Koletzko, Bauer, Wichmann and Schaaf2012). Maternal genetic variants in the FADS gene cluster have previously been associated not only with higher colostrum levels of long chain polyunsaturated fatty acids but also with higher cognitive scores in their children (Morales et al., Reference Morales, Bustamante, Gonzalez, Guxens, Torrent, Mendez and Vrijheid2011).
Accordingly, changes in these gene regions may have an effect on breastfeeding duration. As no other study has explored the molecular genetic basis of breastfeeding, we cannot yet replicate our findings. The fact that we did not find genome-wide significant results is to be expected, considering the low power.
This article has some limitations that need to be taken into consideration. First, measures of breastfeeding were based on self-report and much of the data used was reported a long time after the breastfeeding took place. A larger sample is also required for the GWAS as we expect that breastfeeding is influenced by a large number of SNPs with very small effect size. Ideally, we seek to conduct a meta-analysis combining different samples.
In summary, we have confirmed that genetic factors are significant in the explanation of breastfeeding behavior variability. However, we could neither identify any breastfeeding-related SNP reaching conventional levels of genome-wide significance, nor any overlap with an individual's genetic predisposition towards breast size. This study provides replication of a previous one that explored the sources of variance of breastfeeding in a Spanish sample and, to our knowledge, is the first one to conduct a preliminary GWAS on breastfeeding.
Appendix Births
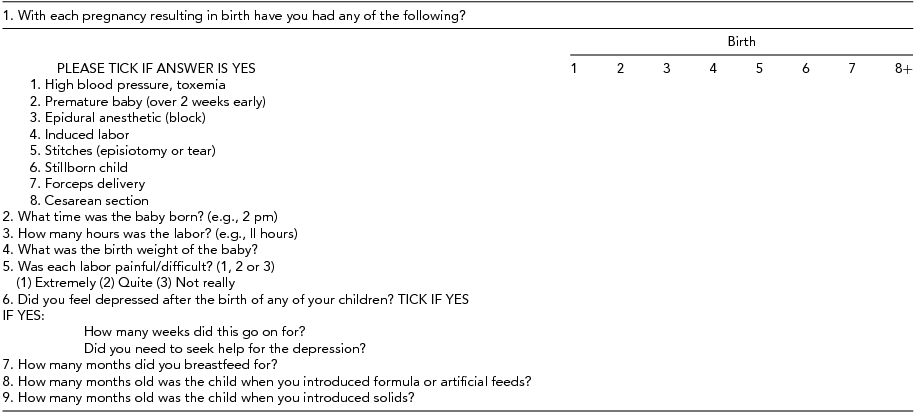
Acknowledgments
We are grateful to the twins for their participation and to the data collectors and data managers Anthony Conciatore, Richard Parker and Scott Gordon. We also thank Liz Rietschel, Jana Strohmaier, Brendan Zietsch, Ada Johansson, Patrick Jern, and Miguel Renteria for their comments on earlier drafts of this paper. Genome-wide genotyping in the Australian sample was funded by NIH Grants AA013320, AA013321, AA013326, AA011998 and AA017688. LCC was supported for a research visit at Genetic Epidemiology Laboratory, QIMR, and for a postdoctoral fellowship by Seneca Foundation — Regional Agency for Science and Technology, Murcia, Spain (12431/FPI/09 and 19151/PD/13).