Book contents
- Coarse Grained Simulation and Turbulent Mixing
- Coarse Grained Simulation and Turbulent Mixing
- Copyright page
- Dedication
- Contents
- Contributors
- Preface
- Prologue
- Part I Fundamentals
- Part II Challenges
- 5 Subgrid and Supergrid Modeling
- 6 Cloud Modeling
- 7 Verification, Validation, and Uncertainty Quantification for Coarse Grained Simulation
- Part III Complex Mixing Consequences
- Index
- Plate section
- References
7 - Verification, Validation, and Uncertainty Quantification for Coarse Grained Simulation
from Part II - Challenges
Published online by Cambridge University Press: 05 June 2016
- Coarse Grained Simulation and Turbulent Mixing
- Coarse Grained Simulation and Turbulent Mixing
- Copyright page
- Dedication
- Contents
- Contributors
- Preface
- Prologue
- Part I Fundamentals
- Part II Challenges
- 5 Subgrid and Supergrid Modeling
- 6 Cloud Modeling
- 7 Verification, Validation, and Uncertainty Quantification for Coarse Grained Simulation
- Part III Complex Mixing Consequences
- Index
- Plate section
- References
Summary
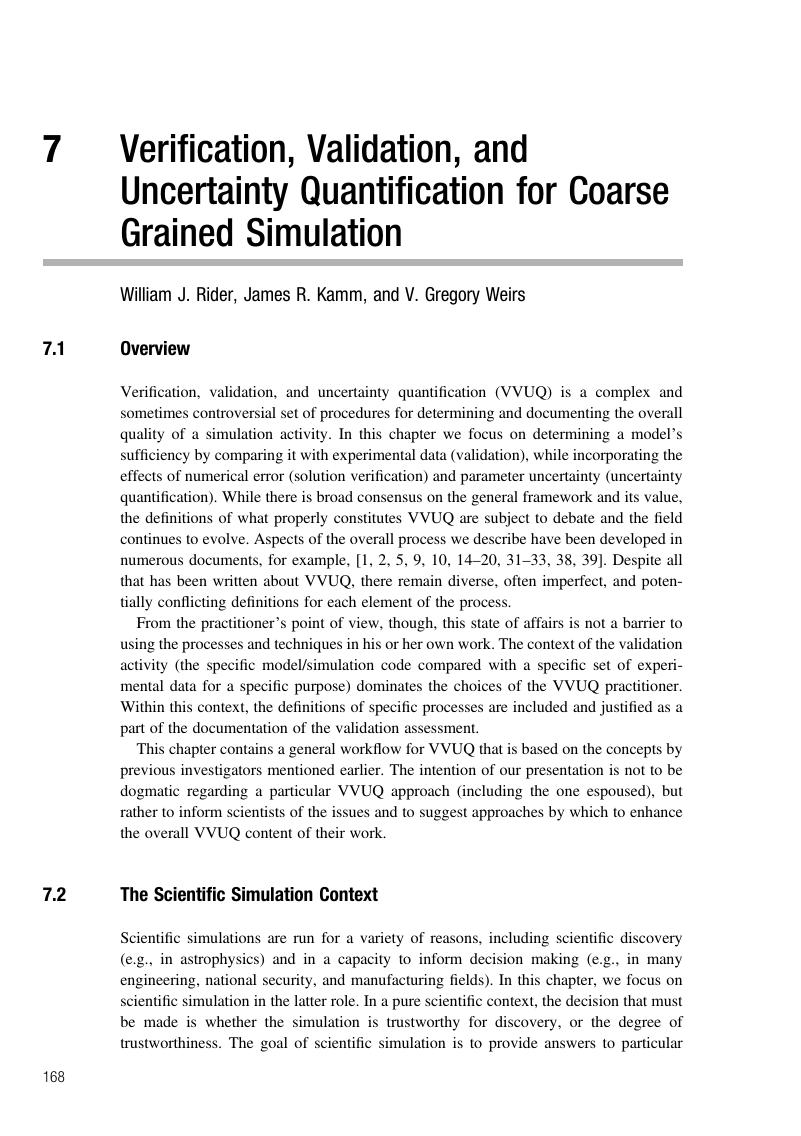
- Type
- Chapter
- Information
- Coarse Grained Simulation and Turbulent Mixing , pp. 168 - 190Publisher: Cambridge University PressPrint publication year: 2016
References
- 2
- Cited by